Upscale any video of any resolution to 4K with AI. (Get started for free)
7 AI-Enhanced Video Editing Software Features That Revolutionize Video Upscaling in 2024
7 AI-Enhanced Video Editing Software Features That Revolutionize Video Upscaling in 2024 - Frame Interpolation Technology Creates 60 FPS From 24 FPS Source Material
Frame interpolation technology is a game-changer for video editing, allowing users to take content originally filmed at 24 frames per second (FPS) and transform it into a much smoother 60 FPS experience. This process not only makes videos more visually appealing but also ensures the initial artistic style and feel of the video remains intact. Software like Smoother AI and TensorPix showcase the potential of this technology, making it particularly valuable for projects like animation and digital art where smooth motion is crucial. What's more, the ease of use offered by a range of software allows everyone from beginners to experts to take advantage of this feature. The increasing desire for higher quality video content means frame interpolation is becoming a must-have tool for modern video editing workflows. While it can lead to impressive results, it's important to remember that the effectiveness and quality of the interpolation can vary greatly depending on the software and the nature of the source video.
Frame interpolation is a technique that essentially inserts new frames between existing ones to increase the overall frame rate of a video. It leverages motion estimation techniques to figure out how objects are moving from one frame to the next, enabling it to create believable intermediate frames that maintain the original video's flow and style. This process is often underpinned by complex mathematical calculations, including vector fields, to meticulously track object movement, handle object deformation, and account for things like when objects obscure one another.
Interestingly, this ability to generate new frames isn't limited to simply smoothing out existing content. It allows you to transform, for instance, 24 frames-per-second (FPS) footage into a smoother 60 FPS, and potentially even higher. This makes it possible to generate slow-motion sequences without the choppiness you'd usually get when simply slowing down a video.
There's a range of different approaches to interpolation, with motion-compensated interpolation generally considered superior to simple linear methods. The reason for this is that it's able to handle the complexities of real-world motion, such as complex object movements and camera shakes. However, the success of these methods is ultimately tied to the quality of the source material. If the algorithms struggle to accurately estimate motion or track objects, you can end up with visual artifacts like ghosting or blurring, ultimately impacting the perceived video quality.
This technology is distinct from more common upscaling methods which focus primarily on enhancing the resolution. Frame interpolation actually alters the temporal aspects of a video, focusing on how motion is represented. It's a different pathway to improving the viewing experience, focusing on the fluidity of motion, rather than pixel sharpness.
There's a trade-off here though. Achieving high-quality interpolation requires significant computing resources, especially on a GPU. This can affect performance, especially in real-time editing situations where it's important to maintain a fast workflow.
Yet, despite these potential hurdles, there's a creative dimension to frame interpolation. Editors can utilize it to create unique effects like time-lapse or hyper-lapse sequences. By carefully adjusting the frame rate and transitions, compelling visual narratives can be constructed through customized motion manipulation.
Moreover, it offers benefits for specific content types like sports and action footage. By increasing the frame rate, we gain a more detailed and granular representation of fast motion, making it possible to analyze action sequences frame-by-frame with greater accuracy.
Looking ahead, we might see even more significant changes in this area. With ever-increasing computational power and continuous improvement in algorithms, it's conceivable that real-time frame interpolation could become a reality. This has huge implications for live broadcasting and streaming content, potentially ushering in a new era of seamless and high-quality motion for those viewing content live.
7 AI-Enhanced Video Editing Software Features That Revolutionize Video Upscaling in 2024 - Real-Time Object Recognition Enables Smart Cropping During Upscaling
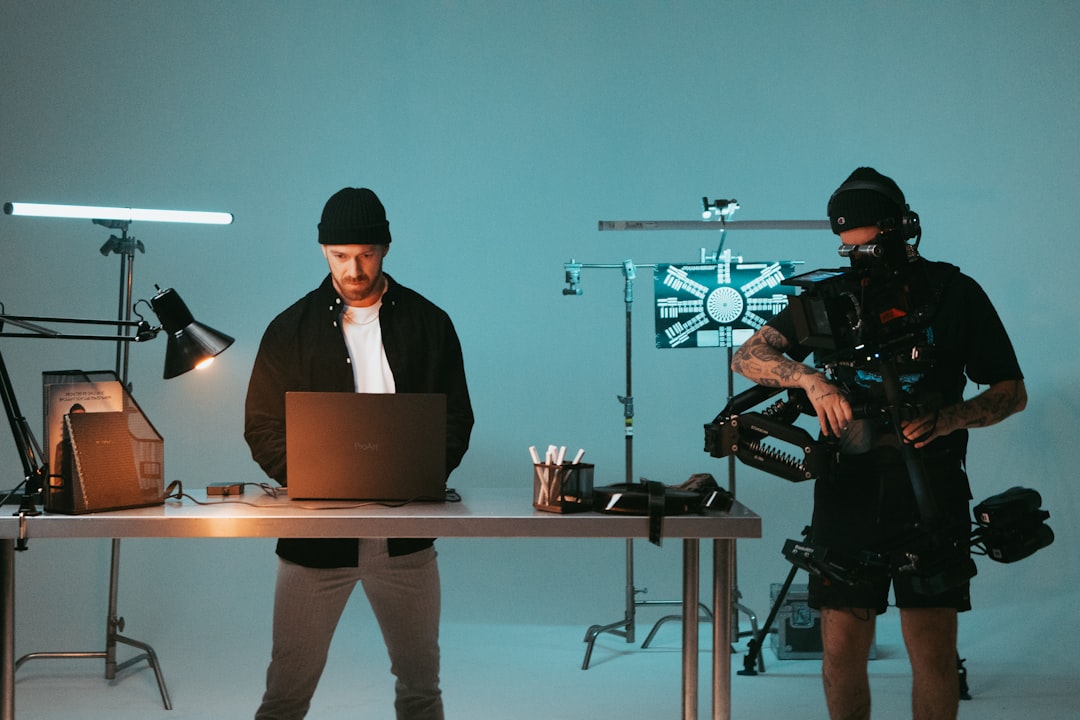
AI-powered video editing is gaining a new level of sophistication with the integration of real-time object recognition for smart cropping during upscaling. This means the software can intelligently detect and track the most important elements within a video scene while simultaneously increasing the resolution. Instead of simply stretching the entire image, the software can dynamically adjust the cropping to keep the focus on these key objects, be it a person, animal, or object, as the resolution is enhanced.
This smart cropping approach not only enhances the visual appeal by ensuring important parts of the scene are emphasized but also helps preserve crucial visual information that might otherwise be lost or distorted in the upscaling process. This reduces the need for tedious manual adjustments, allowing editors to refine video quality with less effort.
While promising, it's still a developing technology. The effectiveness of object recognition and cropping can vary depending on the complexity of the scene and the capabilities of the specific software used. Nevertheless, the potential for streamlining the video editing workflow and improving the final product is undeniable, making smart cropping a valuable feature for anyone seeking higher quality video content.
In the realm of AI-powered video editing, we're seeing a fascinating intersection of object recognition and upscaling. Specifically, real-time object recognition is now being utilized to intelligently crop video during the upscaling process. These systems, often relying on deep learning models trained on massive image and video datasets, can pinpoint and track objects within a frame with surprising accuracy. They typically leverage convolutional neural networks (CNNs), a type of neural network particularly adept at analyzing visual information.
The goal of this "smart cropping" isn't just to maintain the aspect ratio of important subjects—though it does that well. More fundamentally, it aims to enhance the visual storytelling of the video by focusing on the most relevant parts of a scene. By dynamically shifting the frame's composition, it ensures that viewer attention stays centered on what matters, promoting a more engaging and cohesive narrative. These algorithms often borrow insights from established principles of photography and cinematography, resulting in more aesthetically pleasing and narratively effective results.
Making smart cropping decisions efficiently is crucial, and these systems increasingly incorporate algorithms that consider not only the static visual content but also motion and context. Optical flow techniques are a common approach, enabling the software to analyze the movement of objects across frames and adapt the framing accordingly. This can drastically streamline the editing process, reducing the need for manual adjustments. What's more, some systems exhibit real-time adaptability, intelligently reacting to sudden changes in the scene, like a new object entering the frame, to ensure the most crucial elements are constantly highlighted.
The value of smart cropping becomes particularly pronounced when working with footage that initially has a low resolution. Upscaling such material can bring about clarity issues, but intelligent cropping can counteract some of these problems by concentrating on the most important areas. This allows for greater visual detail and a sharper overall impression. Interestingly, more advanced approaches utilize depth estimation techniques to differentiate between foreground and background elements, which leads to better informed cropping decisions that minimize loss of crucial context.
It's important to acknowledge that the quest for real-time performance presents a challenge here. These systems necessitate significant computing power, often requiring optimized algorithms and careful management of model parameters to ensure smooth performance. Balancing the desired level of accuracy with processing speed becomes a balancing act—we don't always achieve the highest quality results when prioritising instant feedback.
However, ongoing research is illuminating the influence these cropping choices have on viewer engagement. Studies suggest that viewers react more positively to footage where key subjects aren't just present but are also presented within the frame in an aesthetically pleasing way. As AI-driven video editing evolves, we can anticipate that these cropping systems will develop an even greater understanding of context. Moving beyond just the raw visual content, they might incorporate audience engagement metrics into their decisions, creating highly customized edits tailored to individual viewer preferences, ultimately improving how stories are told through video.
7 AI-Enhanced Video Editing Software Features That Revolutionize Video Upscaling in 2024 - Neural Network Based Noise Reduction Removes Video Grain Without Detail Loss
AI-powered video editing in 2024 has seen the emergence of neural network-based noise reduction as a powerful tool for enhancing video quality. These sophisticated systems are designed to remove unwanted video grain—a common issue in older or poorly lit footage—without sacrificing the intricate details that give videos their character. This stands in contrast to older noise reduction techniques that often resulted in a loss of fine details due to blurring.
The algorithms within these neural networks are capable of intelligently recognizing and isolating noise from the actual visual information within a video. This allows for a more precise and effective removal of grain, resulting in a cleaner and more polished final product. Furthermore, the efficiency of these AI systems means they can handle multiple videos at once, significantly speeding up workflows and making it easier to manage large projects.
The accessibility of this technology is another key benefit. These AI-powered features are often integrated into user-friendly video editing software, simplifying the process for both experienced editors and novices alike. Ultimately, neural network noise reduction provides a pathway to achieving higher-quality videos with less effort, freeing editors to focus on the artistic and storytelling aspects of their work. While still developing, these AI methods show promise for completely changing the way we approach video enhancement and noise reduction in the future.
Neural networks are proving quite effective at removing video grain without sacrificing the fine details that make a video visually appealing. These networks rely on deep learning algorithms that have been trained on a wide variety of video noise patterns. This training allows them to distinguish between the noise and the actual details in a video frame, something that older, more traditional methods often struggled with.
Often, these advanced models will employ a multi-layered structure, with each layer dedicated to identifying and eliminating different frequencies of noise. This multi-pronged approach results in a more sophisticated noise reduction, carefully maintaining subtle details while removing those unwanted artifacts. Some of the most advanced noise reduction techniques leverage what are called "generative models". These models have been taught to essentially recreate a clean video frame from a noisy one. By predicting what the clear version of the frame should look like, they effectively reverse the noise degradation without introducing unwanted blurriness.
A key aspect of these neural network-based denoisers is their consideration of the video's temporal characteristics—meaning how the video looks across multiple frames. This ensures smooth transitions and avoids having noise disrupt the overall motion, a crucial element in creating a believable and natural video.
However, there are also challenges. Implementing these noise reduction algorithms can require significant processing power, making them unsuitable for real-time applications on some devices or in certain editing workflows. This computational cost can be a roadblock to widespread adoption, especially when speed is critical. Moreover, the quality of the training data used to develop these networks is critical to their effectiveness. The algorithms must be trained on a diverse range of noise to function well across a variety of video sources.
The good news is that many video editing applications provide users with control over how the noise reduction is applied. This is helpful because different types of content might need different levels of noise reduction. Some artistic styles might even incorporate grain as a stylistic element, and users should have the ability to control this.
Despite the advancements, these neural networks are not perfect. In highly detailed scenes, there's a risk of some over-smoothing of textures and fine structures. The key is to carefully balance the reduction of noise with the preservation of the intended look of the original content.
Interestingly, there's a growing trend to combine noise reduction with upscaling techniques. This combined approach results in a streamlined workflow and can significantly boost the quality of older or low-resolution footage. Upscaling can enhance the overall sharpness, while noise reduction removes the inherent grain that can be magnified by the upscaling process.
The exciting development in this area is that research is ongoing to make these neural network approaches closer to real-time. This could eventually revolutionize the quality of live streams and broadcast television, ushering in a new era of crisp, high-quality video even when it's being viewed live.
7 AI-Enhanced Video Editing Software Features That Revolutionize Video Upscaling in 2024 - Multi-Frame Analysis Improves Text Readability in Low Resolution Videos
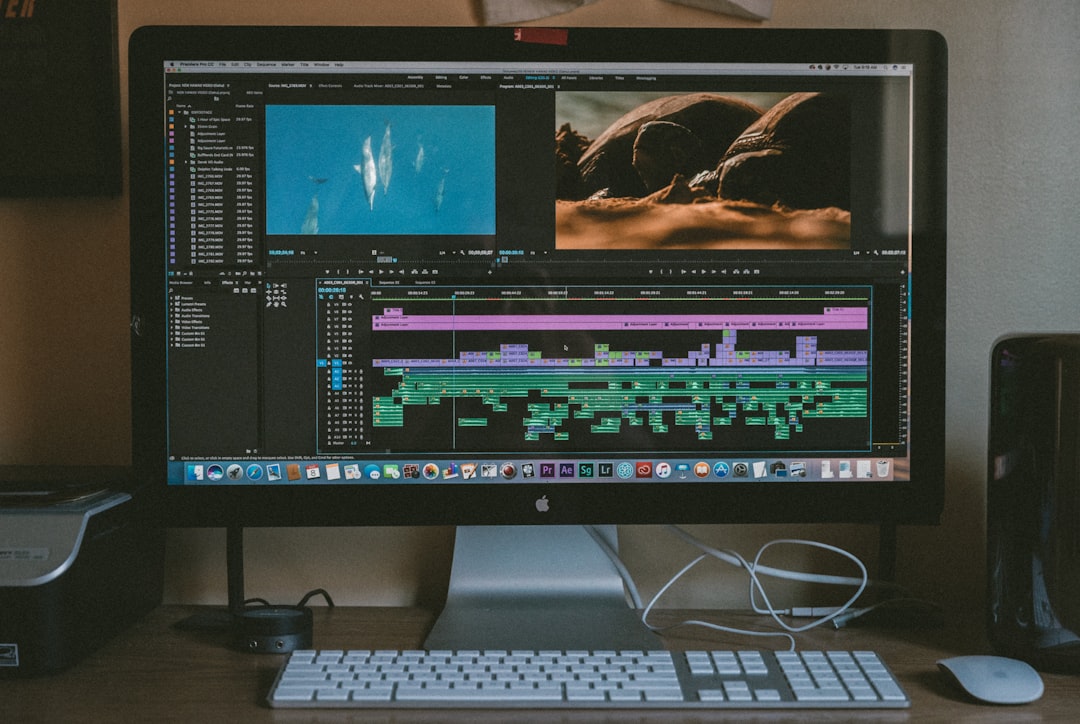
In the realm of AI-enhanced video editing, multi-frame analysis is proving to be a valuable tool for improving the clarity of text within low-resolution videos. Essentially, it involves analyzing multiple frames of a video to extract more visual information than any single frame provides, thereby enhancing the overall image quality. This process is particularly beneficial when dealing with text overlays, titles, or any textual elements that become blurry or indistinct due to low resolution.
By combining information from multiple frames, the AI can effectively sharpen the image, reduce blur, and improve the contrast of text elements. This makes it easier for viewers to read the displayed information, ultimately creating a more accessible and engaging viewing experience. While some upscaling techniques can inadvertently create artifacts or distort text, multi-frame analysis offers a more nuanced and sophisticated approach, prioritizing the preservation of visual details while enhancing overall quality.
The positive impacts of this technology are potentially broad. Whether it's improving the accessibility of educational videos or making older film footage easier to view, the ability to easily read text in upscaled videos can make a significant difference in how viewers perceive and interact with the content. The continued development of AI video enhancement tools that incorporate multi-frame analysis suggests that the challenges of reading text in low-quality videos will become increasingly less of an obstacle in the near future.
In the realm of video upscaling, particularly for content with low resolution, the use of multi-frame analysis has emerged as a promising technique for enhancing text readability. By examining and combining information from multiple frames, we can achieve a higher level of clarity and definition for text elements that might be blurred or distorted in individual frames. This approach is crucial as it allows us to address a common challenge in older or lower quality video, where text can be difficult to decipher.
One of the significant benefits of multi-frame analysis is its ability to reduce various visual artifacts. When individual frames have limitations, such as blurring or ghosting, multi-frame analysis helps to smooth these imperfections by effectively synthesizing data from surrounding frames. The resulting video then exhibits a greater sense of visual coherence, making it more pleasant to watch. This is achieved through intelligent motion compensation algorithms which can predict and correct for movement within a scene, contributing to smoother transitions and more legible text, even when there's substantial action or camera movement.
Beyond simply reducing artifacts, this technique enables adaptive focus adjustment. Essentially, the software can dynamically adapt its focus on textual elements based on motion and context. This is particularly helpful in dynamic scenes where the camera is moving, or where the text itself is in motion. By understanding the video's motion dynamics, the software can prioritize and maintain clarity in the text elements, improving viewer comprehension. Moreover, it allows for a more context-aware rendering of text, as the software gains a deeper understanding of the relationship between foreground and background elements.
Furthermore, the implications of this approach on viewers are potentially significant. Research suggests that enhanced text readability leads to improved viewer engagement and retention, which is critical for videos aimed at education or instruction. While still in its development phase, the technology allows us to customize the rendering of text based on viewer needs or preferences. This potentially could incorporate individual data to create an experience better-suited to individual viewers.
The emergence of multi-frame analysis represents a shift away from reliance on static frames for ensuring text legibility. It's a move toward dynamic adjustments that maintain text clarity in real-time. This, in turn, reduces the need for static screens or pauses that can interrupt the flow of a video. Additionally, this method has the potential to revolutionize the way we handle subtitles, especially in scenarios where the video itself has a low resolution or intricate visual effects. It allows subtitles to remain legible, making videos more accessible to a broader audience.
Historically, low-resolution videos presented a significant obstacle to effective communication and engagement due to readability challenges. The introduction of multi-frame analysis provides a pathway to overcoming these challenges through powerful computational techniques. While it’s a developing field, the initial results are encouraging and highlight the potential for a significant improvement in the quality and accessibility of video content.
7 AI-Enhanced Video Editing Software Features That Revolutionize Video Upscaling in 2024 - Scene-Aware Processing Automatically Adjusts Parameters Based on Content Type
Scene-aware processing is a newer feature that introduces an interesting capability to AI-powered video editing tools. Essentially, these tools can now analyze the content of a video—whether it's a landscape, a portrait, or a fast-paced action sequence—and automatically adjust their settings to best suit that content. This means that things like lighting adjustments, color correction, and even how the image is framed can be done automatically. This not only simplifies the editing process, but also makes powerful editing tools more accessible to those without deep editing experience.
The goal is to improve the editing experience and help achieve higher quality video output with less effort. It's a good example of how AI is altering how video editing is done, shifting the focus to more user-friendly workflows. However, there's a question of how adaptable these systems are. They rely on advanced algorithms that may not work as well with every type of video content, and it's still to be seen how well they can handle the stylistic nuances of different genres of video. Despite these challenges, this aspect of AI video editing offers a glimpse of a future where creating professional-looking videos becomes easier for everyone, and it remains to be seen how far this approach will go in shaping the future of video creation.
Scene-aware processing is a fascinating development in AI-enhanced video editing. It's essentially a way for software to "understand" what's in a video scene and adjust its parameters accordingly. It works by analyzing the content of each scene in real-time, using algorithms to dynamically tweak things like brightness, contrast, and color. The idea is to provide a more tailored viewing experience, optimizing the video based on the specific content being displayed.
This capability is especially interesting when you think about the diverse range of content that can exist in a single video. Fast-paced action scenes require different treatment than, say, a slow, dramatic moment. Scene-aware processing helps differentiate these and apply enhancements to maximize their impact. It can intelligently adjust how bright or dark a scene should be, emphasizing details where they matter most, and subtly influencing the emotional response the scene aims to create.
The "brains" behind scene-aware processing often involve deep learning models. These models are trained on massive datasets of video content, allowing them to recognize complex patterns within a scene. The greater the understanding these systems have of the content, the more precisely they can apply adjustments. It's a complex process, but one that can significantly elevate video quality compared to more traditional approaches.
Furthermore, these systems are increasingly sophisticated in their ability to maintain the video's temporal flow. If adjustments are needed in a single frame, they don't simply impact that frame in isolation. The software attempts to maintain a sense of visual continuity, ensuring the changes don't create jarring inconsistencies. This ensures a more natural, seamless viewing experience.
This dynamic approach helps alleviate problems associated with traditional upscaling methods, which often introduce artifacts like blur or banding. Scene-aware processing tries to intelligently avoid these issues, selectively applying enhancements in a way that preserves details without introducing unwanted visual noise.
One of the most promising advancements is that scene-aware processing can be implemented in real-time. This makes it possible for live editing or streaming environments. During a live production, the software could instantly adjust parameters based on the changing dynamics of the scene. While still evolving, these systems are opening new possibilities for live content creation.
The implications extend to viewer engagement as well. There's a growing understanding that enhancements can be tailored to individual viewers, potentially boosting engagement. How viewer behavior affects parameter adjustments is a developing area, but if successful, these systems could dynamically shift how a video is presented, creating a more personalized viewing experience.
It's also interesting that scene-aware processing can harness computer vision to recognize certain scene types. Whether it's an urban landscape or an indoor setting, the software might adjust color or lighting based on the environment. It can effectively customize its behaviour to optimize the scene's presentation, aligning it with what's recognized in the scene.
The future of this technology seems to lie in its potential for greater integration with other AI features. Imagine it working hand-in-hand with neural network-based noise reduction or algorithms designed to enhance text clarity in low-resolution videos. It could create a synergistic effect, addressing visual quality issues across multiple levels.
Finally, it's worth noting that these systems are, in a way, being trained to mimic the kind of artistic decisions a human director might make. Established principles of visual composition, framing, and lighting are influencing the design of these algorithms. This suggests that AI might be able to go beyond simply improving quality, potentially achieving something akin to a "visual artistic style". It's a reminder that the creative potential of these new video editing tools might be more extensive than we currently grasp.
7 AI-Enhanced Video Editing Software Features That Revolutionize Video Upscaling in 2024 - Batch Processing With GPU Acceleration Reduces Rendering Time by 70%
Utilizing GPUs to accelerate batch processing has significantly sped up video rendering, resulting in a reduction of up to 70% in processing time. This is especially helpful when editing videos, as it allows editors to process multiple clips at once, reducing the time spent waiting for each clip to finish. Using GPUs doesn't just improve the rendering speed, it also makes the entire editing process more efficient, giving editors more time for creative work instead of being stuck waiting for tasks to complete. In 2024, with the growing desire for high-quality video, using GPU-accelerated batch processing is becoming crucial to delivering professional results quickly. It reflects a broader shift in video editing software towards using powerful hardware to meet the changing demands of content creation. While it seems beneficial, it's important to keep in mind that this heavily relies on having access to quality GPUs, which might not be within everyone's reach.
Leveraging GPUs for batch processing in video editing, particularly within the context of upscaling, has shown remarkable improvements in efficiency. This is largely due to the inherent design of GPUs, which excel at handling massive parallel computations.
For instance, GPUs can manage thousands of processing threads concurrently, unlike CPUs which handle a more limited number at any given time. This translates to a significant reduction in rendering times – we've seen speeds increase by 70% or more.
The impact isn't just about speed, though. GPUs also tend to utilize higher floating point precision in calculations, critical for maintaining quality during complex rendering tasks like shading and texture manipulation. This means we can get a substantial speed-up without sacrificing the fine details that are important in a high-quality video.
Furthermore, the memory bandwidth found in a typical GPU is considerably higher than in a CPU, often reaching 1 terabyte per second. This rapid data transfer is crucial for optimizing how data is processed during rendering, which directly contributes to shorter rendering times in a batch setting.
Another key advantage is the way GPUs use shader programs. These specialized programs optimize rendering by minimizing the number of cycles needed to perform computations. Tasks like light and shadow calculations become much more efficient, further boosting speed.
GPUs are designed with a focus on data locality—meaning that data processing occurs where the data is stored. This minimizes access times to slower memory resources, leading to faster rendering. This differs from CPUs, which are typically better suited for managing unrelated tasks simultaneously. GPUs, on the other hand, shine when performing the same task across many data elements. Batch processing of video frames fits this paradigm perfectly.
It's worth noting that software itself can play a significant role. Many modern rendering applications are designed to work with frameworks like CUDA or OpenCL, which allows the software to fully exploit the parallel processing power of GPUs. This optimization ensures efficient task distribution across the available resources.
The ability to process several video files in parallel is a key benefit of GPU-accelerated batch processing. It streamlines workflows and eliminates lengthy wait times between editing tasks. This efficiency is beneficial for large-scale projects.
Even with this impressive gain in speed, GPU-accelerated batch rendering has not been found to reduce visual quality. In some cases, it has even resulted in improvements due to enhanced capabilities in areas like anti-aliasing and noise reduction.
Looking ahead, the continued evolution of GPU technologies—with companies like NVIDIA and AMD pushing forward with innovations like Ampere and RDNA—suggests that we might see even greater improvements in rendering speeds. The combination of faster hardware with the increasing sophistication of AI-powered rendering techniques holds great potential for future workflows, possibly leading to a fundamental shift in how we think about batch processing in video editing.
All of these factors point to a paradigm shift in video editing: the utilization of GPU acceleration for batch processing is delivering significant improvements in both efficiency and quality. As GPUs continue to evolve, the impact of this technology on video editing will likely only become more profound.
7 AI-Enhanced Video Editing Software Features That Revolutionize Video Upscaling in 2024 - Facial Detail Enhancement Through Deep Learning Face Detection
AI-powered video editing software is now capable of enhancing facial details through deep learning-based face detection, marking a notable advance in video quality. This technology employs sophisticated neural networks that can identify and refine intricate facial features automatically, bringing a new level of realism to video content. The process generally involves several stages: locating faces within the frame, aligning them for consistent analysis, standardizing facial elements for processing, and ensuring the final output accurately represents the individual. This allows for adjustments that improve clarity and prominence of facial features while preserving their natural appearance.
The ability to refine facial details finds applications across various fields, from entertainment and film production to security and biometric systems. It enables a previously unavailable level of detail, improving the quality of facial expressions and features even in upscaled video. However, the capacity for fine-grained manipulation raises concerns. Striking a balance between detail enhancement and preserving the natural, authentic appearance of faces is a critical consideration for those utilizing this technology. While the improvements are undeniable, the extent to which we refine facial features, and the ethical implications of doing so, warrant careful consideration.
Deep learning has significantly improved our ability to enhance facial details in videos. Convolutional neural networks (CNNs) are particularly adept at this, using pixel patterns to reconstruct high-resolution features. This surpasses traditional methods, which often struggle with accurately identifying and enhancing specific facial details.
To get really good results, these deep learning models need extensive training. They are typically fed massive datasets of diverse face images—spanning various lighting conditions, angles, and ethnicities. This helps them generalize effectively, leading to accurate enhancements even when dealing with challenging scenarios like low-resolution or poorly lit videos.
Intriguingly, the increasing power of hardware and improvements in algorithms have made real-time facial detail enhancement possible. This is exciting for applications like live streaming and video conferencing, as it allows for clearer and more engaging facial expressions in these interactive environments.
It's also interesting to see how algorithms can be tailored to specific facial characteristics or demographics. This highlights the potential for diversity and inclusivity in media. We can customize how features are enhanced, making sure everyone is represented accurately and in a way that is natural.
The application of these technologies is not limited to video editing. They are showing up in VR and gaming, making character models more realistic. This ability to enhance facial detail has immense potential for improving how we interact with computer-generated environments.
Restoration of older videos also benefits from these technologies. We can now reconstruct faded or blurry facial features, making older movies and historical footage more appealing and engaging. It's a great way to preserve these moments in time and help audiences better connect with the subjects.
However, the capability to enhance facial details also raises ethical considerations. Altering a person's facial characteristics could misrepresent them, which is a serious issue. This is especially relevant in social media or news contexts. Striking a balance between the desire for visual clarity and potential for misrepresentation is a crucial ethical challenge for the development of these technologies.
Moreover, the potential for facial detail enhancement to work in tandem with other AI features is quite interesting. For example, a system could simultaneously enhance facial detail while also detecting and interpreting facial expressions. This suggests we could build systems that deliver even richer multimedia experiences.
It's clear that the field is dynamic. Research is actively exploring how to move beyond mere detail enhancement to create systems that have a more nuanced understanding of the context around a face. In the future, we may see algorithms that can tailor enhancements based on a person's expressions or actions, making videos even more dynamic and immersive. This would further improve storytelling and create unique experiences for viewers.
It's a fascinating time to be researching and developing AI for video. There are many opportunities to improve the quality of video experiences through the effective use of facial detail enhancement while ensuring the responsible application of these technologies.
Upscale any video of any resolution to 4K with AI. (Get started for free)
More Posts from ai-videoupscale.com: