7 AI Video Enhancement Techniques to Elevate Your Business Explainer Videos in 2024
7 AI Video Enhancement Techniques to Elevate Your Business Explainer Videos in 2024 - Frame Rate Optimization Using Deep Learning Motion Analysis
Improving frame rates using deep learning can significantly refine how motion is presented in videos, especially when dealing with quick movements that traditional techniques often struggle to capture cleanly. Deep learning approaches can analyze the motion between frames and create new, intermediate frames, effectively boosting the frame rate without losing detail – a process called frame interpolation.
Some research suggests that deep learning can double or even quadruple frame rates in specific video scenarios, enabling a transformation from a standard 30 frames per second (fps) to a smoother 60 fps or even higher. The application of convolutional neural networks (CNNs) for motion analysis has opened up possibilities for real-time frame rate adjustments. This means the frame rate can be fine-tuned dynamically based on the video's content, rather than relying on a fixed setting.
However, a major hurdle in using these methods has been the demanding computational resources. Thankfully, developments in GPU technology have helped make it more feasible to use these AI models for enhancing videos in real-time for many commercial applications.
Intriguing model types like Generative Adversarial Networks (GANs) have shown impressive results in generating high-quality intermediate frames. These innovations continue to improve the visual fidelity we achieve during frame rate upscaling.
We can even consider replacing or improving traditional video encoding's motion vector estimation with deep learning methods. This can lead to a deeper understanding of motion, encompassing complex shifts and objects partially hidden from view.
Assessing how well frame rate optimization techniques work can be difficult. Sometimes, what a viewer prefers doesn't completely align with standard, objective measurements. There have been findings showing viewers may favor videos enhanced with deep learning methods, even when older methods achieve better scores on traditional assessment methods.
Newer neural rendering techniques utilized in frame rate optimization don't just enhance image quality. They also have the potential to be integrated with other video aspects, such as color correction and sound syncing, allowing for a more holistic video upgrade.
The use of self-supervised learning for frame rate enhancement is emerging as a potential game-changer. This approach allows models to learn from unlabeled data, leading to significant reductions in the training time and resources usually needed. This accessibility to advanced video enhancement technology could have a democratizing impact on video editing and creation.
7 AI Video Enhancement Techniques to Elevate Your Business Explainer Videos in 2024 - Resolution Upscaling Through Neural Network Processing
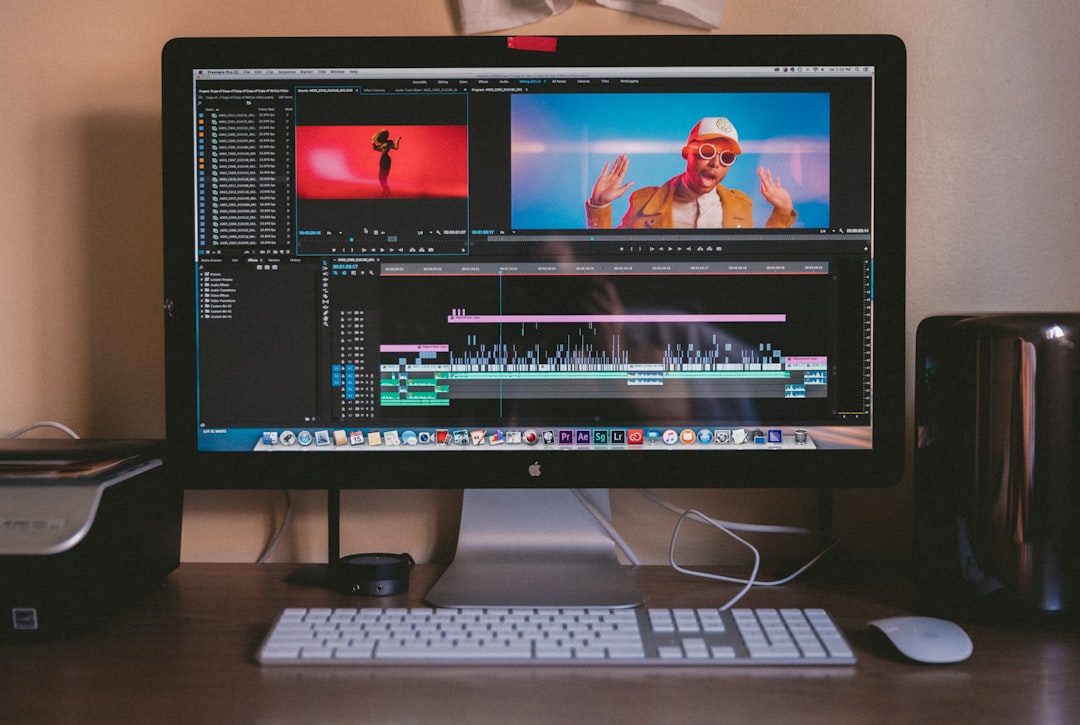
AI-powered resolution upscaling is a leading-edge video enhancement method. It leverages advanced neural networks to intelligently infer and fill in missing image information in low-resolution videos, effectively boosting the overall quality and detail. This means older, lower resolution videos can be transformed into higher definition versions, like converting standard definition (SD) to high definition (HD) or even 8K. Furthermore, neural networks can also address blurriness or pixelation, leading to a cleaner and more refined visual presentation. The accessibility of these techniques is improving through tools like Adobe Premiere Pro's AI capabilities and dedicated AI video enhancement services. This suggests a move toward more automated, efficient processes for video upscaling, offering businesses greater control and quicker workflows for improving their explainer videos. However, as these techniques continue to evolve, the increasing reliance on AI for video content creation raises interesting considerations about the future role of human creativity in this field.
Resolution upscaling through neural network processing offers a compelling approach to enhancing video quality. These networks, often built on convolutional neural networks (CNNs), excel at reconstructing missing image details that are lost during traditional upscaling methods. Instead of just stretching pixels, they learn from vast amounts of data to intelligently fill in textures and edges, producing remarkably sharper results.
Many of these advanced neural networks leverage a technique called "super-resolution" which goes beyond simple resolution increases. They also refine aspects like contrast, sharpness, and noise reduction, resulting in a perceptual improvement over the original content. However, the choice of neural network architecture is important because different ones achieve different outcomes. More complex models, such as ESRGAN (Enhanced Super Resolution GAN), integrate techniques like residual learning and adversarial training to significantly boost upscaled image clarity. These models often demonstrate enhanced capabilities over simpler networks.
The processing power needed for real-time upscaling using neural networks remains a significant hurdle. It often demands considerable GPU memory and processing time. While hardware advancements have made this more manageable for some applications, ongoing research into more efficient algorithms is crucial. It is intriguing to observe that even with sophisticated upscaling, artifacts can sometimes occur. These visual blemishes can detract from the viewer experience, so carefully tuning the models is needed to balance detail enhancement with retaining the original image's natural appearance.
Neural networks can be trained to specialize in specific content types, improving their ability to tailor enhancement to the particular nature of the video. This means they can be optimized for specialized applications, like explainer videos, leading to better results. Though resolution upscaling primarily focuses on visual enhancement, it can sometimes unintentionally alter the original video's artistic character. This has sparked discussions about "image authenticity" and how these enhancements can influence viewer perceptions and potentially impact the intended message.
Researchers are increasingly using self-supervised learning approaches to train resolution upscaling models. These methods utilize large datasets without labels, drastically reducing the training time and resources needed to develop effective solutions. This has implications for democratizing access to sophisticated video editing technology for smaller organizations. Furthermore, integrating resolution upscaling with other video processing methods, like background blurring or color grading, presents exciting opportunities to create innovative content. This type of integrated approach gives video creators greater control over refining the aesthetics of their work.
It's important to note that viewer preferences for upscaled content can vary greatly. While some viewers value the clarity of ultra-high definition, others might prefer the nostalgic look of lower-resolution formats. This highlights the complex interplay between technology, aesthetics, and user experience within the field of video content.
7 AI Video Enhancement Techniques to Elevate Your Business Explainer Videos in 2024 - Smart Color Grading With Pattern Recognition
AI-powered color grading, specifically using pattern recognition, is a recent development that's changing video editing. These systems use machine learning to analyze video content, recognizing patterns to automatically adjust color balance, brightness, and saturation. The aim is to refine the visual look of videos in a way that matches the creator's desired aesthetic. This automated approach not only speeds up the editing process but also promotes consistency across projects.
The benefit is clear: easier access to professional-looking results. However, there's a potential pitfall. If not carefully managed, the AI could over-correct the colors, making them appear overly saturated or unnatural. This can actually diminish the video's impact by taking away from the artistic vision the filmmaker originally had in mind. As this technology matures, the challenge will be to strike a balance between enhancing video quality and preserving the original intent of the content. Essentially, it's about using the technology as a tool, not as a replacement for artistic judgment.
AI-powered color grading, often referred to as smart color grading, is becoming increasingly sophisticated by incorporating pattern recognition techniques. These techniques involve training algorithms on vast collections of videos, allowing them to learn the nuances of color palettes that are often associated with specific genres, moods, and even viewers' preferences. This learned knowledge then informs the AI's ability to suggest or even automatically apply color grading effects that align with a desired aesthetic or narrative.
One of the fascinating aspects of this approach is the potential to optimize color grading for different platforms. AI algorithms can analyze the color reproduction capabilities of various display technologies, whether it be a smartphone screen, a television, or a cinema projector. This allows the color grading to adapt to the unique characteristics of each platform, ensuring a more consistent viewing experience across various contexts.
Moreover, AI can leverage insights from color psychology. Research suggests that particular color combinations evoke specific emotional responses in viewers. By recognizing these patterns, smart color grading algorithms can intelligently adapt color palettes to create the desired tone for a video.
The application of these algorithms has also shown the potential to expedite the color correction process. By automating certain color adjustments, AI can free up creators to focus on other aspects of video production. While traditional color correction can be a time-intensive process, smart color grading has shown the capacity to decrease the time needed by as much as 50%. However, we need to carefully consider the potential ramifications of automating this creative process. It raises questions about whether there's a risk of homogenizing video aesthetics as AI systems become more ubiquitous and refine their recommendations.
It's important to highlight that smart color grading isn't limited to basic color adjustments. Advanced techniques can refine skin tones, which is crucial for maintaining realism and naturalness when working with human subjects. AI can also address issues like uneven lighting and inconsistency across different scenes, creating a more unified look and feel for the entire video.
While the technology has a lot of potential for positive impact, it's not without its drawbacks. As AI algorithms learn from datasets, they might be trained in a way that results in a certain 'look' that becomes dominant. A growing reliance on these algorithms could unintentionally lead to creative stagnation or reduce the diversity of artistic styles. We must continue to carefully observe how viewers respond to the subtle changes created by smart color grading and critically assess the potential influence of AI-driven color choices on our perceptions of video content. Balancing the need for standardization and consistency with the desire for unique and expressive visuals will be an ongoing discussion in the coming years.
7 AI Video Enhancement Techniques to Elevate Your Business Explainer Videos in 2024 - Background Noise Reduction Through Audio Separation
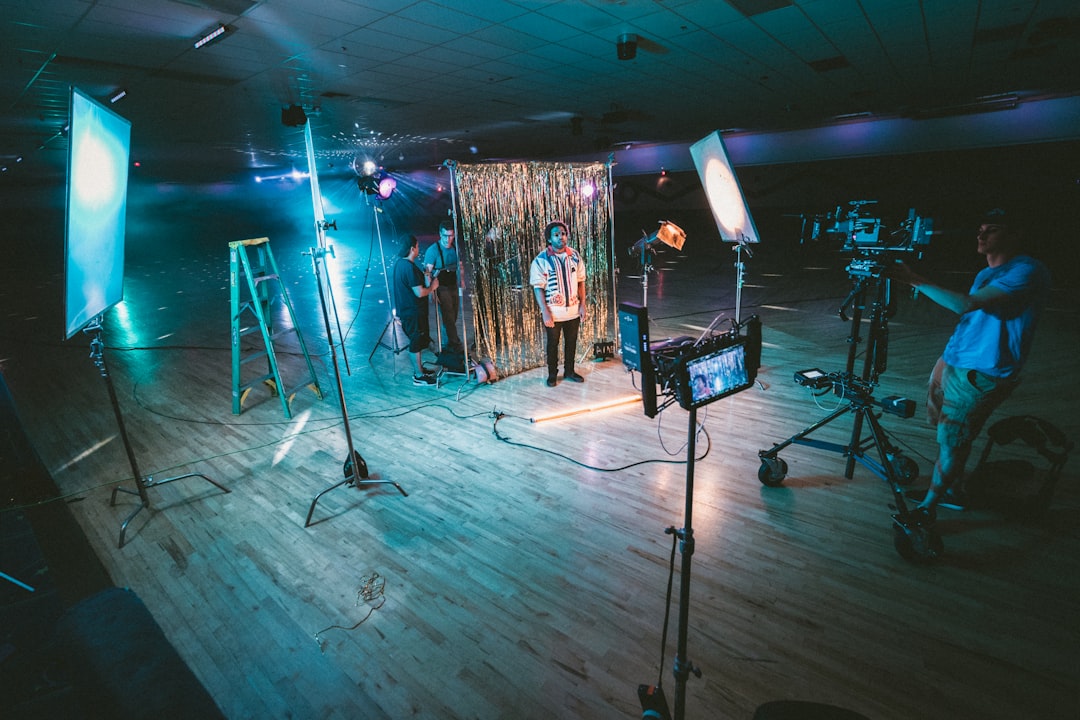
In 2024, effectively eliminating background noise is crucial for making professional-quality explainer videos. While traditional methods often struggle with complex audio scenarios, AI-powered audio separation offers a new approach. These AI techniques can isolate and remove unwanted background noise, like chatter or ambient sounds, without degrading the primary audio. Tools are becoming increasingly common that can quickly separate the desired audio from the noise using machine learning models, which greatly speeds up the process.
While the ability to rapidly clean up audio is a huge advantage, there are some potential caveats. Over-reliance on automated solutions can sometimes introduce subtle distortions or unwanted artifacts that detract from the intended quality. It is important to exercise some caution and judgment when applying these tools to ensure the desired outcome. A balance between the convenience of AI solutions and a mindful approach to audio quality is key for achieving professional audio in explainer videos. This nuanced approach can be the difference between a polished final product and something less than ideal.
Complex audio environments, like those often encountered in business explainer videos, necessitate effective background noise reduction. This isn't simply a matter of muffling unwanted sounds; it increasingly involves disentangling audio into its separate components. This separation, often termed source separation, allows us to isolate specific elements like voices amidst a symphony of background sounds. This is particularly beneficial when capturing meetings, tutorials, or even social media content where clear communication is key.
Techniques like Non-Negative Matrix Factorization (NMF) and, more recently, deep learning methods, enable this decomposition of audio into its component parts. This means even intricate audio mixtures can be painstakingly analyzed, allowing us to either amplify or remove specific sound sources without sacrificing the overall sonic landscape. This capability holds enormous potential for podcasters or anyone looking to enhance their audio's clarity.
The ability to perform background noise reduction in real-time is a recent development with significant implications for live-streaming and video conferencing, where maintaining clarity is paramount. This capability has been made possible through advancements in algorithm design.
However, the efficacy of these techniques hinges heavily on the availability of high-quality training data. Training AI models on extensive datasets containing both pristine and noisy audio allows them to better understand the characteristics of each and improve the overall effectiveness of their noise cancellation performance.
Interestingly, reducing background noise can paradoxically affect how we perceive the primary audio source. While algorithms strive for crystal-clear audio, they might inadvertently generate audible artifacts if they aren't precisely tuned. These artifacts can detract from the listener's experience, demonstrating the need for careful calibration.
Modern algorithms can now intelligently adjust to fluctuating noise levels in real-time. Instead of relying on static noise profiles, these systems are adept at learning and adjusting as the acoustic landscape shifts. This adaptability significantly improves noise reduction's reliability when faced with varied conditions.
Intriguingly, research is exploring the potential for audio separation to work synergistically with the visual components of videos. For example, if a speaker is prominently featured in the frame, systems might be trained to selectively prioritize noise reduction around their voice, resulting in a richer audio-visual experience.
Some systems allow users to tailor noise reduction to specific settings or personal preferences, moving beyond the limitations of a one-size-fits-all approach. This offers greater flexibility and can further refine the end result, improving user satisfaction.
Working with multi-channel audio adds a layer of complexity to noise reduction compared to processing a simple mono signal. Fortunately, sophisticated techniques now exist to simultaneously process multiple channels, allowing us to more effectively disentangle and clarify sounds across a broader spectrum. This capability enhances the overall listening experience by reducing the interference of overlapping sound sources.
Research suggests that enhanced audio clarity can reduce the cognitive load experienced by listeners, resulting in improved understanding and information retention. This has significant implications for explainer videos, where conveying complex information in an easily understood way is crucial. This underscores how noise reduction not only enhances audio aesthetics but also plays a key role in facilitating effective communication.
7 AI Video Enhancement Techniques to Elevate Your Business Explainer Videos in 2024 - Automated Video Stabilization With Motion Tracking
Shaky footage can significantly detract from the quality of a video, especially for professional business explainers. Automated video stabilization with motion tracking helps solve this problem by using artificial intelligence to analyze and adjust video frames. These AI algorithms can track the movement within the video and make corrections, helping to keep important subjects steady and in the center of the frame. This is particularly useful when you have footage captured using a handheld camera, where shaking is more common. While these AI-powered tools can definitely make editing faster and improve the look of a video, there's always the possibility that the over-use of such features can make the final video feel too polished or artificial. In essence, there's a balance to strike. This balance is crucial to maintain the organic and natural quality of the content. As AI-powered video stabilization continues to improve, it's likely to become a key component of crafting professional-looking and engaging explainer videos. This is especially important in 2024, where clear and effective video communication plays a pivotal role in how businesses connect with their audience.
Automated video stabilization using motion tracking is a fascinating area of AI video enhancement. It essentially tackles the common issue of shaky footage by using algorithms to analyze how the camera moves frame by frame. These algorithms are able to make real-time corrections, resulting in smoother, more watchable videos. Methods like optical flow, which help estimate the movement of objects in a scene, are becoming increasingly important in providing detailed information for stabilization.
One of the key benefits of these automated systems is their ability to address jitter, that annoying slight shaking that can come from handheld cameras. While this might seem like a minor issue, it can be quite distracting to viewers. Thankfully, these systems are designed to minimize jitter by ironing out minor inconsistencies between frames.
But stabilization can go beyond simply smoothing out the entire frame. More advanced techniques integrate motion tracking, which allows the system to understand the motion of the subject within the video. This means the system can tell the difference between a shaky camera and purposeful subject movement, leading to a more refined output where the main subject is highlighted while the background is stabilized.
Historically, stabilization was typically done in post-production, requiring extra editing steps. However, advancements in real-time stabilization technology allow filmmakers to create stable footage on the fly, even during live streams. This ability to stabilize instantly opens up a wide array of new possibilities for capturing dynamic scenes without having to rely on specialized equipment.
The computational burden of these processes can be a hurdle. Powerful algorithms require significant processing power. Researchers are constantly working on ways to make these algorithms more efficient so they can run smoothly on existing hardware. This ensures businesses and individuals can leverage these benefits without requiring massive computational resources.
Interestingly, studies have shown that stabilized video actually improves the perception of narrative flow. When the viewer isn't distracted by shaking, they can more easily focus on the content and the story being presented. This leads to improved comprehension and retention of the message in explainer videos and other types of content.
Modern stabilization technologies usually include controls that allow users to fine-tune the degree of stabilization and the focal point of the stabilization. This flexibility gives video creators the ability to tailor the stabilization to the specific needs of each project and aesthetic goal. For example, sometimes a slightly shaky camera can enhance a sense of immediacy or authenticity.
Further advancements integrate machine learning models that analyze scene depth, alongside motion. This allows the stabilization to account for the three-dimensional structure of the environment. The results are often more natural-looking and viewers are less likely to notice the adjustments that were made.
The great thing about AI stabilization is that it can be applied in a variety of different video situations, from sports to documentaries. This adaptability makes it a valuable tool for a broad range of businesses and content creators.
Finally, the growing trend of designing user-friendly interfaces for stabilization tools is fantastic. This makes these techniques more accessible to users with varying levels of technical expertise. It's a great example of how technological advancements are making professional video production techniques available to more people, opening doors for small businesses and individuals to create compelling content with ease.
7 AI Video Enhancement Techniques to Elevate Your Business Explainer Videos in 2024 - Dynamic Light Adjustment Using Scene Analysis
Dynamic light adjustment, powered by scene analysis, is a newer AI method that's especially valuable for enhancing explainer videos in low-light situations. AI can analyze the video's scene in real-time and make intelligent decisions about how to adjust the lighting. This helps to improve clarity and make sure important parts of the video are visible, even if the original footage was shot in less-than-ideal conditions. A key benefit is that these methods can minimize or even eliminate the flickering artifacts that can sometimes be a problem with other video enhancement techniques.
The technique relies on understanding the changes in lighting from one frame to the next. By analyzing the temporal information across frames, AI can more accurately adjust the color balance and manage noise. This prevents viewers from missing out on vital information due to poor lighting conditions.
Despite its promise, there are challenges with this type of dynamic light adjustment. If the AI changes the lighting too much, the enhanced video might not look natural. It needs to be subtle and careful so that the original intent of the video creator isn't lost in the process. Moving forward, refining these systems to create an enhancement that doesn't distort the original video's aesthetic will be essential for ensuring the effectiveness of business explainer videos. The goal is to make sure videos are easier to watch while still retaining their natural character.
Real-time adjustments to video lighting, often referred to as dynamic light adjustment, can leverage scene analysis to create a more visually appealing and engaging viewing experience. It's a concept that moves beyond static lighting adjustments, adapting brightness and contrast on a frame-by-frame basis. While traditional methods often struggle to maintain a consistent look across varied lighting conditions, these AI-driven techniques analyze the scene in real-time, distinguishing elements like foreground subjects and backgrounds to intelligently optimize lighting.
One of the primary advantages of this approach is the ability to instantaneously react to changes in the environment or the subject within the scene. For example, if the camera moves from a brightly lit outdoor space into a dimly lit indoor environment, a dynamic light adjustment system can quickly adapt the video's lighting, ensuring consistent visibility without jarring the viewer. It's like having a virtual cinematographer making constant subtle lighting adjustments in real time.
Researchers have discovered that, when properly implemented, these adjustments can improve contrast sensitivity in viewers. By emphasizing differences between subtly varying color shades, these techniques can enhance the impact of videos intended for instructional or detailed presentations. We can also strive for more consistent lighting across the entire video. Even if scenes were captured under vastly different lighting conditions, the system can analyze average brightness and color temperature, creating a more uniform experience.
But, some approaches are even more ambitious. Some research explores using eye-tracking data. The idea is that these systems can focus lighting adjustments on the parts of the video the viewer is focusing on. This adaptive approach could lead to greater viewer engagement and potentially better information retention.
The machine learning angle is also fascinating. AI algorithms can adapt to viewer preferences over time. This could mean the lighting styles that are applied will evolve, becoming progressively better tailored to the intended audience. The idea is that by learning from viewer interactions, the AI can offer a more refined experience.
Interestingly, these techniques aren't limited to any particular type of video. Whether it's a marketing video, a tutorial, or anything else, the tools can be applied. For businesses that produce multiple kinds of video content, this can mean a more consistent visual style across their output.
A notable benefit is the potential reduction in the time spent correcting videos in post-production. With automated lighting corrections built into the filming process, video creators might spend less time with fine-tuning adjustments later. In the fast-moving digital media landscape, that's a big deal.
Furthermore, advanced algorithms can separate the subjects within a scene. This means the main subjects of a video can be given enhanced lighting while dimming the background. It's like automatically drawing the viewer's attention to the key elements without losing the context of the entire scene.
These techniques have the ability to simulate artistic effects as well. For instance, it might be possible to create the gentle softening of light at sunset or replicate the flickering of candlelight. This has the potential to give a more impactful quality to business explainer videos, without necessarily needing extensive manual manipulation.
However, the journey of these AI-driven technologies is ongoing. A major challenge involves effectively leveraging scene understanding to provide high-quality, natural-looking lighting, without resorting to overly stylized or artificial effects. The future of video lighting appears to involve a careful balance between intelligent automation and the preservation of artistic intent.
7 AI Video Enhancement Techniques to Elevate Your Business Explainer Videos in 2024 - Smart Object Detection For Clearer Text Overlays
AI video enhancement is increasingly sophisticated, and one of the newer techniques, "Smart Object Detection for Clearer Text Overlays," focuses on improving the readability of text within videos. It uses AI algorithms to identify objects within a video scene, enabling text overlays to be positioned and styled in a way that makes them easy to read, even against complex or changing backgrounds. This intelligent placement of text, coupled with real-time adjustments, not only makes the text itself more visible but also helps reduce visual distractions, enhancing the viewer's experience.
However, achieving the right balance is key. As with other AI video enhancement techniques, there's always a risk of making the overlays appear artificial or overly precise if the technology isn't used carefully. This can detract from the video's original message or intended style. The goal is to use this technology as a tool to make the text clearer without fundamentally altering the video's look or feel.
If successfully implemented, smart object detection has the potential to greatly simplify the process of adding text to videos, particularly for creating professional and engaging explainer videos. By making the text more readable and less visually disruptive, these techniques can make videos more accessible and impactful for viewers.
Smart object detection is a relatively new technique that's enhancing how we add text overlays to videos, particularly for explainer videos. It uses AI to precisely map pixels and understand the context of the video, allowing text to be placed in a way that's clear and doesn't interfere with the visual content. Essentially, it's a more sophisticated approach to ensuring text remains readable even in busy scenes or when the background elements change rapidly.
One intriguing aspect is the use of deep learning models, often convolutional neural networks (CNNs), for tasks like optical character recognition (OCR). These models can distinguish text from complex backgrounds, which is crucial for placing overlays in a way that extracts the essential information and presents it cleanly to the viewer. Another benefit is the capability for real-time processing, making it suitable for scenarios like live broadcasts where overlays need to adjust dynamically. The AI algorithms can also figure out how overlays should interact with objects in the foreground, using depth information to determine when text should be sharper or have transparency, giving a better visual experience.
There's an interesting interplay between recognizing objects and actually interpreting their significance. While these systems are quite good at finding objects, they still struggle with the nuances of context. For example, if a text overlay ends up in a cluttered section of the video, it can become less noticeable. So, ongoing research aims to refine these algorithms so they can better balance visual appeal and conveying the necessary information.
We're also seeing the development of better feedback mechanisms within these systems, with user input influencing how text is placed. This learning approach can potentially make overlays more effective over time based on how viewers engage with the content. It's quite fascinating to see how machine learning is being used to guide these overlays.
Aesthetics also play a significant role. Modern systems often use layers and effects like gradients or shadows to improve the look of text overlays. This is important because readability and visual appeal work together to provide a polished and professional presentation.
Another aspect worth noting is the integration of smart object detection into user interfaces that make it easier to control text overlays. This makes these powerful techniques more accessible to people of different skill levels, a trend we're seeing across many aspects of video editing.
It's noteworthy that the field is experimenting with incorporating other inputs like audio. This multimodal approach allows the overlay behavior to adapt to audio cues within the video, helping to improve synchronicity across different communication channels.
While the current state of smart object detection shows great promise for clearer and more effective text overlays, there's still work to be done in terms of fully grasping the subtleties of content and context. As researchers continue to refine these systems, we'll likely see even more powerful tools that elevate the quality of explainer videos and other visual media.
More Posts from ai-videoupscale.com: