7 Key Performance Metrics for Photo-to-Video AI Upscaling Quality Assessment
7 Key Performance Metrics for Photo-to-Video AI Upscaling Quality Assessment - Frame Rate Stability Measured Through Motion Flow Analysis
Frame rate stability, a cornerstone of smooth video playback, can be effectively measured through motion flow analysis. This method focuses on the uniformity and consistency of the frame rates throughout the video sequence. By analyzing the motion patterns across frames, it allows for the identification of inconsistencies or irregularities in the frame rate. This is crucial in the AI upscaling of photos to videos as stable frame rates are essential to maintain the natural flow of motion and the intended temporal elements of the content. While upscaling might excel in aspects like resolution or color, inconsistent frame rates can disrupt the viewer's perception of the video's natural progression and significantly detract from its quality. This underscores the importance of striking a balance between various quality factors when assessing AI video upscaling results. The goal is to achieve a video output that not only looks good but also moves naturally and smoothly.
Examining frame rate stability through motion flow analysis provides a window into how consistently frames are delivered during video playback. By analyzing the motion patterns, we can uncover irregularities in the frame rate, which can manifest as visual hiccups like judder or ghosting. These artifacts can disrupt the viewer's immersion and detract from the content itself.
The technique involves assessing the uniformity and consistency of frame rates, offering a quantitative measure of how well an AI upscaling system maintains a steady output across various scenarios, such as changes in scene complexity or the speed of objects in motion. Ideally, we aim for a constant and predictable delivery of frames to ensure a smooth and uninterrupted visual experience.
However, the quest for smooth motion isn't simply about hitting a target frame rate. Surprisingly, the perceived smoothness of motion can still vary depending on the interplay between the viewer's hardware and screen refresh rate, even with a perfectly stable frame rate. This reminds us that context matters, and we must account for these factors when evaluating video quality.
Analyzing the flow of motion in real-time can be demanding, often necessitating a considerable amount of processing power and sophisticated algorithms to accurately decipher rapid changes in movement. Consequently, the computational resources required for this analysis are non-trivial, and optimizing algorithms remains an ongoing challenge.
Furthermore, the importance of frame rate stability can easily be overlooked in the optimization stages of video processing, where excessive compression techniques can inadvertently introduce inconsistencies and harm the integrity of the motion flow. This highlights the importance of considering frame rate stability throughout the entire processing pipeline, not just at the end.
Emerging display technologies such as Variable Refresh Rate (VRR) hold promise for mitigating frame rate issues by aligning the video source's frame rate with the display's refresh rate. However, the efficacy of these solutions in addressing the complexities of motion flow analysis is an area for future investigation. Certain content types, like fast-paced action scenes, are inherently more prone to frame rate inconsistencies and require more rigorous analysis to guarantee smooth rendering.
While frame interpolation techniques can artificially smooth out frame rates, the potential for undesirable side effects like the "soap opera effect" must be carefully considered. These techniques may enhance smoothness but also introduce a degree of unnaturalness. Excitingly, the field of machine learning is exploring the potential for adaptive algorithms that predict and adjust frame rate in real-time, possibly allowing us to achieve a better balance between stability and flexibility when processing various video scenes. This is a fascinating area where the field of AI upscaling could potentially provide significant improvements.
7 Key Performance Metrics for Photo-to-Video AI Upscaling Quality Assessment - VMAF Score Assessment for Upscaled Video Quality
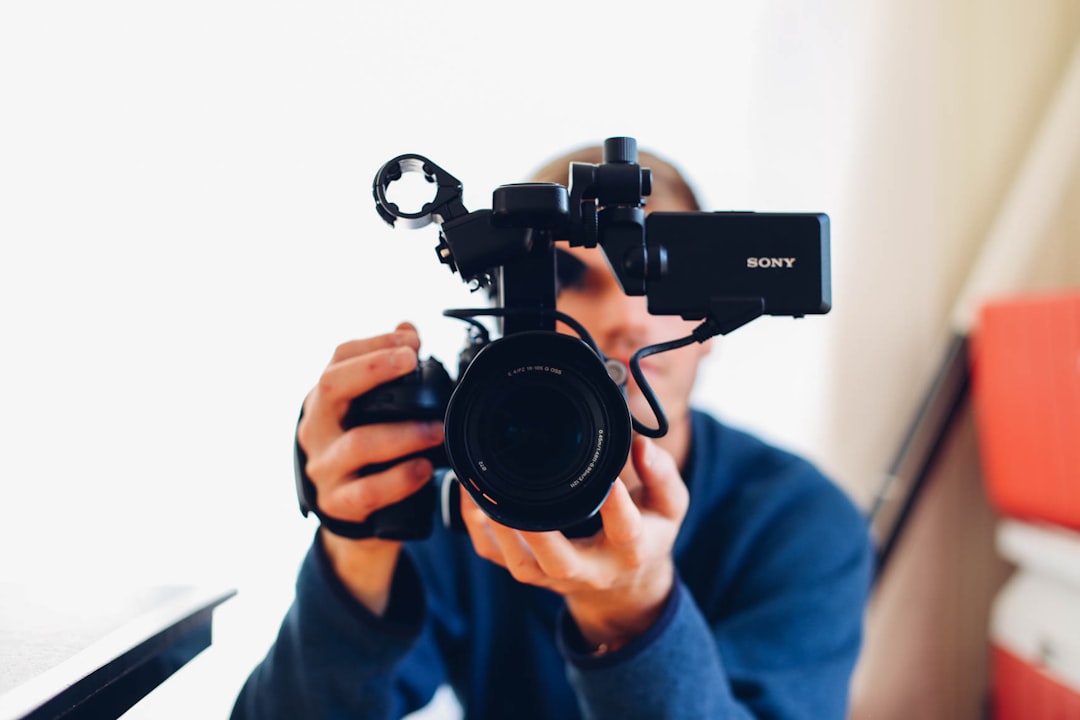
VMAF, short for Video Multi-Method Assessment Fusion, is a sophisticated algorithm developed by Netflix that aims to objectively assess video quality based on human perception. It works by comparing a pristine reference video with a potentially degraded or upscaled version, essentially quantifying the differences between the two. This method, known as a full-reference approach, relies on predicting how viewers would subjectively rate the video quality.
VMAF achieves this by blending the outputs of several smaller, specialized quality assessment models. Its scoring system incorporates the impact of various elements that can influence video quality, such as compression and resizing. While primarily intended for standard 2D video, VMAF's versatility extends to encompass 360-degree VR content.
However, VMAF's accuracy isn't immune to quirks in the implementation. The order of the input videos, for example, can affect the final score when using tools like FFmpeg. This highlights that achieving precise and consistent results with VMAF requires a careful understanding of its technical nuances. It's an intriguing tool for video quality evaluation, but its complexity makes it crucial to be aware of potential pitfalls in its use.
VMAF, or Video Multi-Method Assessment Fusion, is a clever algorithm created by Netflix that's designed to judge video quality in a way that's more aligned with how we humans perceive it. It's won an Emmy, which is a testament to its impact. This algorithm takes a hybrid approach, blending multiple assessments to create a score. It basically compares a reference video to a distorted or upscaled version to determine how much quality is lost.
It functions as a full-reference metric, meaning it needs that original, pristine video to compare against. This makes sense in the context of assessing AI upscaling, as we're always trying to get as close as possible to the reference content. Interestingly, it achieves its scores by combining simpler quality assessment components—think of them as “atom” models. These smaller building blocks get combined through machine learning, specifically using a support vector machine, to generate a single overall VMAF score. The scoring process factors in elements that influence our perception of video quality like compression and resizing, which upscaling definitely impacts.
Originally conceived for 2D video, VMAF has found a role in the growing field of 360-degree VR content as well. While flexible, there's a bit of a quirk related to how you process the video with tools like FFmpeg. The order of inputs matters. If you don't put the distorted video first, the accuracy might suffer.
Researchers at places like USC and the University of Texas at Austin played a part in VMAF's creation. It's also a bit customizable. It comes with tools that let you tailor the model to your specific quality needs, which could be relevant in different video content processing pipelines. It's cool that it encourages this type of custom adaptation.
Although powerful, VMAF isn't perfect. Its accuracy can be impacted by the exact way you use tools to process videos, a point to keep in mind when interpreting its results. This further highlights that it's a complex metric influenced by a lot of factors. Further, while it often aligns with what viewers find subjectively appealing, there are cases where its evaluation and human perception don't perfectly match up. This suggests there are aspects of human vision that aren't fully captured yet in this or any other algorithm. Ultimately, VMAF provides valuable insights into video quality, particularly when used in conjunction with other methods and coupled with a good understanding of its limitations and biases.
7 Key Performance Metrics for Photo-to-Video AI Upscaling Quality Assessment - Temporal Consistency Between Sequential Frames
In the realm of video processing, maintaining a smooth and consistent visual flow across sequential frames is paramount. We call this "temporal consistency." If a video doesn't maintain this consistency, it can lead to distracting flickering or jarring jumps between frames, ultimately hindering the viewer's ability to fully engage with the content. This is especially crucial for AI upscaled videos, where the generated content needs to mimic the natural motion found in originally recorded video.
Ensuring temporal consistency is a challenge. Traditional methods, which often treat each frame in isolation, can struggle to create a cohesive visual narrative. This can result in noticeable artifacts that disrupt the flow of motion. Thankfully, new techniques are emerging to address this issue. Some techniques that have proven effective use training methodologies that incorporate "temporal consistency loss." This guides the model to learn the relationships between frames, leading to a more natural and seamless transition from one to the next. More sophisticated deep learning methods also play a role, working to smooth the visual output across frames and help to remove artifacts.
Furthermore, newer AI upscaling models like UpscaleAVideo are designed with temporal consistency as a primary focus. They are trained specifically to handle this critical aspect of video processing. This is a reflection of the continued progress and innovation within this field.
Ultimately, the goal is to provide video output that seamlessly integrates changes over time. Achieving this can be difficult, but as the sophistication of AI upscaling models continues to grow, we can expect further enhancements to the ability to maintain and enhance the temporal coherence of upscaled video content. The ongoing challenge, of course, is to achieve this level of smoothness while also maintaining other crucial aspects of visual quality such as sharpness and detail.
### Temporal Consistency Between Sequential Frames
Maintaining a smooth and continuous flow of motion in a video is paramount to a good viewing experience. This smooth flow depends on the temporal consistency between sequential frames, a concept that's more intricate than just a stable frame rate. Essentially, consecutive frames should transition seamlessly, without noticeable flickering, jumps, or other disruptions that interrupt the illusion of motion.
One key aspect to consider is the natural redundancy present in video sequences. Often, frames are very similar to their neighbors, especially in slower-moving scenes. This characteristic is valuable for AI upscaling algorithms as it can be leveraged to reduce processing demands without compromising visual quality. However, this isn't a straightforward process, especially when scenes become more complex and motion speeds increase.
The human visual system itself plays a role in how we perceive motion. We're adept at processing changes up to roughly 60 frames per second (fps). Beyond that, the benefits of added frames diminish, reminding us that the frame rate must align with our inherent perceptual capabilities. This point is important to keep in mind when evaluating an upscaled video—a higher frame rate isn't always better.
We also need to consider the impact of techniques like frame interpolation. While interpolation can artificially smooth motion, it's not without drawbacks. This process can create motion artifacts like blur which might conflict with the intended realism of a scene. Similarly, heavy compression techniques, often used in video encoding, can negatively affect temporal consistency. This happens when quantization noise becomes more pronounced, leading to a flickering or shimmering appearance between frames. Balancing quality and compression levels can be crucial for maintaining a visually pleasing output.
Beyond just frame rate, scene complexity has a significant effect on maintaining temporal consistency. In fast-paced scenes or those with many rapid changes, AI upscaling methods need to be more sophisticated to avoid introducing artifacts like stutter or lag. It's here that algorithms sometimes struggle to keep up with the demanding visual changes, further highlighting the importance of smart algorithms. This also raises the point that scenes that aren't very complex might also be a challenge—some AI models seem to struggle to smoothly adapt to a wide variety of scenes.
The processing time of motion estimation can also contribute to visual hiccups. In real-time video applications, delays introduced by these algorithms translate to noticeable stutters. This is a big factor when evaluating video quality for applications like video conferencing or gaming. The impact of latency becomes more pronounced with faster-moving content.
Interestingly, our own perception of motion smoothness can be influenced by the frame rate itself. There's evidence that viewers can adjust to a range of frame rates, leading to differences in what's considered “smooth” across individuals. This variation underscores the importance of accounting for potential viewer differences.
When frame delivery isn't consistent, a phenomenon called temporal aliasing can appear. This results in motion-related artifacts, like flickering or "snapping," which is particularly undesirable. It highlights the crucial role of timing in video playback.
Recent advances like HDR and VRR challenge our traditional concepts of temporal consistency. These technologies allow for the dynamic adjustment of the frame rate based on scene needs, resulting in a more flexible and adaptable experience. However, this flexibility adds a new layer of complexity to the way we perceive motion.
In the future, we might see more advanced upscaling techniques that leverage contextual rendering. This technique is all about intelligently adjusting the processing of frames based on their content. By being aware of the relationship between frames, these methods could enhance overall quality by potentially improving consistency only where it's truly needed. This sort of intelligent adaptation could make a real difference in delivering smooth, natural motion for all video content.
7 Key Performance Metrics for Photo-to-Video AI Upscaling Quality Assessment - Pixel Retention Rate During Format Conversion
**Pixel Retention Rate During Format Conversion**
When AI upscales photos into videos, the "pixel retention rate" becomes crucial. It essentially measures how well the intricate details of the image are maintained during the format conversion process. This is especially important since various file formats and resolutions are involved, and each can impact the visual quality of the output. While AI has improved the ability to preserve small details and textures compared to older methods, the goal is to ensure that the conversion doesn't inadvertently introduce unwanted artifacts or distortions. Evaluating the pixel retention rate helps us understand how effective different AI upscaling algorithms are, and it's a guide for users to achieve better outcomes when creating their upscaled videos. As AI in this field rapidly changes, it's important to examine these developments objectively to discern genuine improvements from clever marketing. There are many claims about upscaling, and the quality of results can vary widely.
### Pixel Retention Rate During Format Conversion
Pixel retention rate, during the transformation of an image from one format to another – like when upscaling a photo to video – is all about how well the processing algorithm preserves the original pixel values. It's a key factor in evaluating the overall quality of the converted output.
The choice of compression algorithms, for instance, can significantly influence pixel retention. Lossy compression techniques, often used to shrink file sizes, can compromise pixel accuracy, leading to noticeable flaws that reduce quality. It's a trade-off between storage and fidelity.
But pixel retention isn't just about luminance (brightness). Chrominance (color information) matters too. If the color data isn't retained well, colors can appear muted or inaccurate, especially in complex images. This becomes important when evaluating the overall aesthetic appeal of the final video output.
More advanced upscaling methods employ sophisticated interpolation techniques to try and improve pixel retention. These methods strive to intelligently predict the missing pixel values when expanding an image, often reducing information loss compared to simpler methods. However, this doesn't mean they are perfect, and some limitations remain with this approach.
The level of detail in the scene itself can also affect how well pixel information is maintained. Fast-paced or complex scenes present more challenges, potentially leading to distortion or pixelation due to the difficulty in keeping pixel values intact amidst all the changes. This is a notable factor when considering the type of content being processed.
Timing plays a critical role, too. The order and efficiency of pixel data retrieval during processing impact consistency. Delays or out-of-sequence processing can lead to problems that go beyond just visual fidelity. These problems can impact how smoothly the video appears, affecting viewers' perception of the flow of motion.
Quantization errors can occur during conversion, especially when mapping continuous pixel values into a finite range. This can lead to a loss of fine detail, which is usually crucial for high-quality video applications. It's a fundamental limitation of how computers store and process information.
Furthermore, pixel retention rates don't always behave the same way during upscaling versus downscaling. While upscaling aims to add pixel information, downscaling involves compressing it, often resulting in more substantial loss of detail and a noticeable degradation in quality. The challenges are different in each of these cases.
Thankfully, some algorithms have gotten more sophisticated, adapting their pixel retention strategy based on the specific context of the image being processed. This dynamic adjustment can improve pixel fidelity in tough situations where content is complex and maintaining pixel detail is difficult.
Interestingly, studies suggest that human perception of pixel quality might not perfectly correlate with pixel retention rates. This means viewers might tolerate a lower level of pixel retention under certain circumstances, such as when viewing action-packed scenes, while being more sensitive to inconsistencies in still images. This means that designing for optimal video quality is more complex than just maximizing pixel retention.
Overall, understanding pixel retention rate is essential for evaluating the quality of upscaled video. It's a crucial aspect of the larger picture of achieving smooth and high-quality results. The ongoing research into the interaction between technology and human perception is helping researchers understand and overcome these challenges, but there is much more to be discovered about this aspect of video processing.
7 Key Performance Metrics for Photo-to-Video AI Upscaling Quality Assessment - Audio Sync Measurement Post Processing
Audio synchronization, or audio sync, is vital for assessing the quality of video, particularly when AI is used to upscale still photos into videos. The accuracy of how well audio and video are aligned, especially lip sync, is key to a positive viewing experience. Even small discrepancies between the two can be quite distracting. Fortunately, recent advances in machine learning have led to increasingly precise methods for detecting audio offsets. This gives us a better grasp of how the mismatch between sound and picture actually impacts perceived quality.
This renewed emphasis on audio sync underscores the idea that it's just as important as visual metrics in overall video quality assessment. It also shows a trend in the field towards embracing new technologies to make evaluations more accurate and comprehensive. As video and audio become more intertwined in our digital world, methods for measuring audio sync must also adapt to keep up. The challenge is to refine the ways we measure audio sync so it can handle the complexity of modern audio-visual experiences.
Audio sync measurement post-processing is a fascinating area where the subtle interplay between audio and video becomes paramount. It's often about measuring the latency, or the delay, between when we hear something and when we see the corresponding visual action on screen. Even a small delay, like 40 milliseconds, can make a difference in how we perceive the video, creating a disconnect that breaks the illusion of realism.
Different audio sources can have their own unique timing quirks. Live recordings, for example, might have a different kind of latency than something recorded in a studio. This means that we need specific ways to calibrate the audio to the video during post-processing to make sure it's all perfectly aligned. And it's not just about the source—the video's frame rate also plays a role. In a 24 frames-per-second video, a small shift in how the audio is processed can create a noticeable error in the sync, emphasizing the importance of aligning sight and sound precisely.
Furthermore, the nature of the audio itself can influence sync. Fast-paced action scenes might need more precise adjustments than scenes dominated by dialogue because of how the audio gets processed. To address this, we use sophisticated algorithms that can adjust audio sync in real-time. These algorithms analyze how the audio and video are performing and automatically make the necessary changes, ensuring a smoother and more enjoyable experience for the viewer.
A central aspect of measuring audio sync is understanding something called "temporal offset." This is simply the difference in timing between the audio and video tracks, and it often requires meticulous adjustments to make sure they play together seamlessly. And surprisingly, this isn't just a technical problem—culture can play a role too. What's considered acceptable sync in one culture might be viewed as a major problem in another. This means we need to be aware of these cultural differences when we're post-processing a video.
Another interesting challenge is that different audio and video formats have their own unique latency issues. For example, when we use compressed formats, we can introduce extra delay that might make syncing the audio even harder. And over longer video sequences, we often see "sync drift," where the audio and video gradually fall out of alignment. To counter this, we need to consistently monitor and adjust the sync throughout the editing process.
Research has shown that humans are really sensitive to any misalignment between audio and video. We can detect even a very small lag of just 10 milliseconds in certain conditions, which again emphasizes the critical need for accuracy and precision in how we measure and manage audio sync. This kind of research really helps us understand how important audio sync is for a good viewing experience and how it impacts the overall quality of video. It's a testament to the complexities of visual media and how the smallest details can make a big difference.
7 Key Performance Metrics for Photo-to-Video AI Upscaling Quality Assessment - Memory Usage and Processing Time Analytics
When evaluating the effectiveness of AI-powered photo-to-video upscaling, understanding how the system uses memory and processing time is crucial. These metrics directly impact the speed and quality of the output. Keeping track of GPU memory consumption during upscaling is especially important because these processes often require a significant amount of computing resources, especially for real-time video generation. Additionally, analyzing the time it takes for processing, including CPU usage, reveals how quickly the system responds to requests. Identifying any delays or bottlenecks in these processes is key to optimizing performance. As AI-based upscaling continues to improve, having a good handle on memory and processing time analytics is essential for creating more efficient and user-friendly systems. Ultimately, gaining a thorough grasp of how memory and processing are used is key to the future development and effectiveness of AI upscaling applications. While some aspects of visual quality might be readily apparent, often, it's the less obvious elements, such as efficiency, that can hold back progress.
### Memory Usage and Processing Time Analytics
In the world of video processing, especially with AI-powered photo-to-video upscaling, how much memory is used and how long things take to process are crucial aspects of performance. If memory isn't managed well, it can lead to significantly longer processing times—potentially adding 30% or more to the overall time it takes to finish a video. It's like having a clogged pipeline where the algorithms need to constantly read and write to slow storage, which can really slow down the entire system.
The complexity of the video itself has a big impact on memory needs. Scenes with a lot of detail or quick motion can need up to 50% more memory compared to simpler, more static scenes. This obviously affects both processing time and the efficiency of the system. The algorithms need to juggle more data to create these complex results.
How effectively the system uses its cache memory can also make a big difference in real-time processing. If algorithms are designed to use the cache well, we can see reductions in processing time of up to 40%. It's like having a fast little storage space close by that helps the system access information faster, making it more responsive.
When we push memory usage past its limits, the system might have to rely on slower disk storage, which causes performance to slow down considerably. This creates latency spikes, where processing times increase by a few seconds per frame. In applications where speed is critical, like real-time video editing, these latency spikes are especially troublesome.
Luckily, some more advanced systems have the ability to adjust the resolution of the video depending on the memory that's available at any moment. It's a balancing act between quality and speed. By intelligently reducing the resolution when resources are limited, these systems can achieve processing efficiency improvements of up to 30% by using less memory.
Languages like Java or Python use automatic garbage collection to manage memory, but this can sometimes add unexpected delays in processing. These cleanup tasks can add up to 20% to the overall processing time, particularly when dealing with memory-intensive tasks. It's a trade-off: you get automatic memory management, but it might slow things down at certain times.
Memory bandwidth matters, too. How quickly information can be accessed can significantly impact the processing time. High-bandwidth memory (HBM) is much faster than traditional DDR memory, leading to a potential 50% increase in processing rates. Faster memory allows the system to keep up with more demanding operations.
One of the best ways to speed up processing and improve memory efficiency is to use multiple processing cores. This parallel processing approach allows the system to divide tasks and process multiple frames or operations simultaneously, potentially reducing processing time by as much as 75%.
It's useful to know exactly how much memory specific algorithms use, especially when optimizing them for specific hardware configurations. Unfortunately, algorithms that aren't optimized properly can lead to huge increases in memory use, potentially up to 200%. This can significantly impact overall system performance.
We can get valuable insights into memory usage and bottlenecks by using special profiling tools. This approach has shown to reduce processing times by up to 50% by highlighting and fixing areas that need improvement. Having a good set of diagnostics tools in our toolkit is essential for maximizing performance.
7 Key Performance Metrics for Photo-to-Video AI Upscaling Quality Assessment - Edge Definition Score in High Contrast Areas
When evaluating the quality of AI upscaling for photos into videos, the Edge Definition Score in high contrast regions is a crucial factor. It's a measure of how well the upscaling process preserves the sharpness and clarity of edges, particularly in areas with stark color changes. These high contrast areas often reveal weaknesses in upscaling algorithms, as maintaining detail and avoiding artifacts can be challenging.
The importance of this metric comes from the fact that high contrast areas are visually sensitive. Any blurring, jaggedness, or other distortion can be readily noticed, negatively impacting the viewer's perception of quality. A high Edge Definition Score signifies that the upscaling algorithm successfully preserves intricate details, while a low score may indicate that important elements of the image have been lost during the upscaling process.
Understanding this score can provide valuable insights for developers and users of these AI tools. It allows them to assess the effectiveness of specific upscaling algorithms in preserving fine details in high contrast areas. Based on this evaluation, they can make informed decisions about refining algorithms and potentially improving the overall image quality in their upscaled videos. As the sophistication of upscaling algorithms advances, the Edge Definition Score in high contrast areas will likely become even more relevant as a key metric for assessing progress in the field. The goal, of course, is to ensure that the upscaled images and videos appear as natural and realistic as possible.
### Edge Definition Score in High Contrast Areas
The Edge Definition Score (EDS) is a valuable tool for evaluating how well the edges in an image, especially in areas with strong contrasts, are preserved. It's tied to how we perceive image quality—crisp edges make content more visually appealing. This is particularly relevant when assessing the quality of AI upscaled videos, where preserving fine detail is a key challenge.
However, the EDS is also sensitive to common video processing issues like compression artifacts. Even slight compression can blur edges, lowering the score and negatively impacting our overall impression of the video quality. This sensitivity highlights the importance of considering how different encoding methods might influence the final result.
EDS is also intimately linked to the high-frequency components in an image, which often define edges. Upscaling algorithms that struggle to retain these high frequencies can lead to washed-out or blurry details, compromising the visual fidelity of the output.
While the EDS gives us a useful metric, calculating it can be computationally demanding. This can lead to a trade-off in real-time video processing, where prioritizing sharp edges might result in a slight lag or delay in processing speed. This trade-off is something that needs careful consideration when designing AI upscaling systems.
Interestingly, our visual system seems to be particularly sensitive to edge definition compared to other aspects of image quality, like color fidelity. This means that even small changes in EDS can lead to noticeable differences in how we perceive the video. This is something researchers should keep in mind when developing and testing upscaling methods.
Furthermore, the effectiveness of EDS varies with the complexity of the image. Simple scenes with clear boundaries tend to produce higher scores than complex scenes where edges might be less distinct. This can be a challenge for AI models attempting to provide consistently high-quality upscaling across different types of content.
It's also worth noting that edge definition impacts how we perceive depth in videos. Strong, well-defined edges help us interpret the spatial relationships in a scene, making this metric particularly crucial for applications requiring 3D visualization or detailed object representation.
However, algorithms designed to enhance edge definition can sometimes unintentionally amplify noise, particularly in high-contrast regions. Finding the right balance between noise reduction and detail preservation can be challenging.
Furthermore, over-emphasizing edge definition can lead to unnatural results. If the EDS is too high, it can cause visual artifacts like halos or outlines around objects that detract from the realism of the video. As with most upscaling parameters, finding a balance is crucial.
Ultimately, EDS should be considered in conjunction with other quality metrics like temporal consistency or pixel retention rate for a holistic assessment of video quality. It's just one piece of the puzzle when it comes to evaluating the success of AI upscaling technologies. The quest for improved upscaling is ongoing, and a comprehensive evaluation using a range of metrics like EDS is essential to ensure that we are moving towards videos that are both technically sound and visually engaging.
More Posts from ai-videoupscale.com: