Upscale any video of any resolution to 4K with AI. (Get started for free)
7 Transformative Applications of AI in Scientific Image Analysis From Neural Networks to Visual Recognition in 2024
7 Transformative Applications of AI in Scientific Image Analysis From Neural Networks to Visual Recognition in 2024 - Neural Networks Now Process 5 Million Medical Images Daily at Mayo Clinic
Mayo Clinic's adoption of neural networks has resulted in a remarkable daily throughput of 5 million medical images. This scale highlights the growing reliance on AI within the clinic for both diagnosis and research. Convolutional neural networks, in particular, are showing promising results, exhibiting human-level competency in critical areas like disease detection and assessment. The broader field of deep learning is reshaping healthcare by streamlining the analysis of medical images, especially for time-sensitive conditions. However, the development of dependable deep learning models remains a critical challenge. We need to ensure that these models undergo thorough testing to guarantee their reliability and accuracy in medical settings. The future of medical image analysis appears bright, with emerging technologies like vision transformers and adaptable platforms offering potential for customized applications that could enhance current practices and push the boundaries of disease understanding.
At the Mayo Clinic, neural networks are now processing a massive 5 million medical images every day. This surge in automated image analysis speaks volumes about the growing reliance on AI for diagnosis and research across a wide range of medical fields. It's fascinating to see how quickly these networks have become integrated into their workflow.
While convolutional neural networks (CNNs) have proven exceptionally adept at tasks like disease screening, diagnosis, and staging, there's still ongoing debate about their complete reliability. The use of deep learning, which leverages multi-layered neural networks, allows these models to analyze complex patterns in huge datasets, leading to significant improvements in image analysis. It's remarkable how these techniques, originally developed in computer vision and other domains, have been successfully adapted for medical applications.
The core challenge remains: developing robust deep learning models that are both accurate and can be validated rigorously. We're seeing a growing focus on achieving that reliability through extensive testing and validation. It's still a work in progress, but the pace of development is astounding.
Interestingly, CNNs seem to be the dominant approach in medical imaging right now, mainly because they drastically reduce the need for manual feature engineering. However, other techniques like vision transformers (ViTs) are gaining traction, offering potentially distinct advantages in both accuracy and computational efficiency.
Researchers can now leverage adaptable platforms like Piximi and ImJoy to design and train their own neural networks for specialized tasks in image analysis. This opens up a plethora of possibilities for tailoring AI to specific needs, like identifying cell phenotypes or localizing particular cells within images.
Despite these breakthroughs, a degree of wariness persists in the medical community about the unchecked reliance on algorithms for major decisions. Striking a balance between harnessing the power of these technologies and retaining the critical role of human expertise remains a crucial aspect of the ongoing development in this field. It's definitely a fascinating area to keep an eye on as it continues to evolve.
7 Transformative Applications of AI in Scientific Image Analysis From Neural Networks to Visual Recognition in 2024 - Visual Recognition System Maps 85% of Brain Tumors More Accurately Than Manual Methods
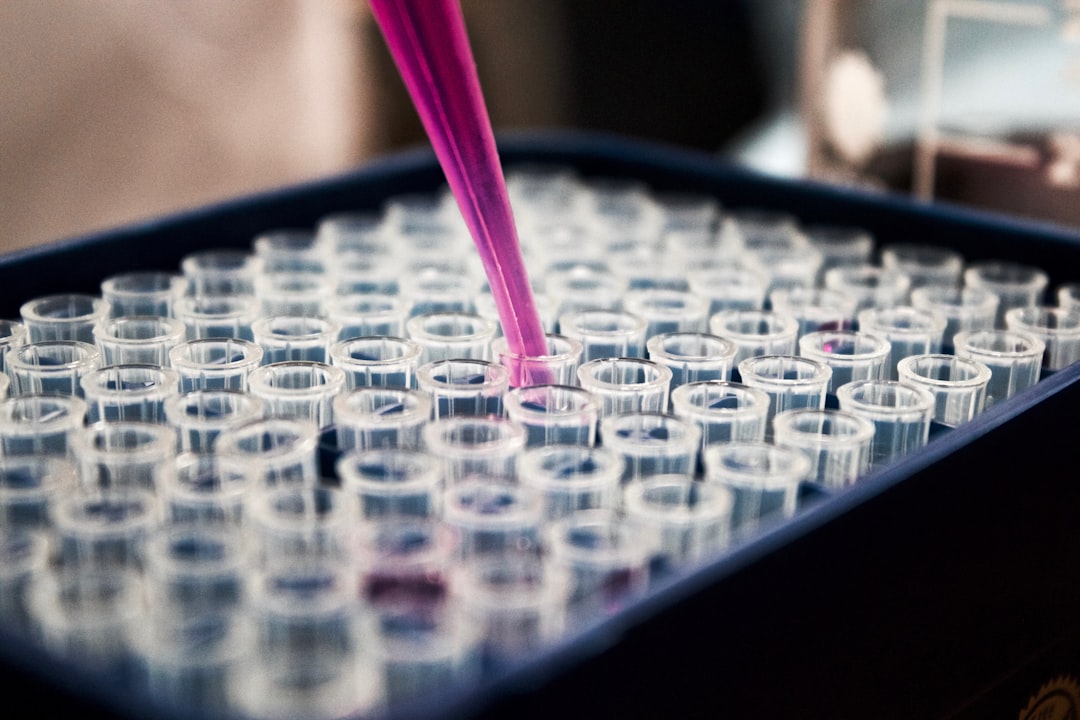
A new AI-powered visual recognition system has shown promise in mapping brain tumors with an accuracy rate of 85%, outperforming traditional, manual methods. This advancement in image analysis is particularly significant in the field of neuro-oncology, where accurately differentiating tumor types like gliomas and metastases is crucial for treatment planning. The system leverages the power of artificial intelligence to analyze medical images, offering potential for quicker and more precise diagnosis compared to human interpretation. While this is an encouraging step, it's important to recognize that further development and rigorous testing are needed to ensure the system's reliability and suitability for widespread clinical use. The goal is to optimize these AI tools to provide reliable assistance to medical professionals, ultimately leading to better patient outcomes through earlier detection and intervention. The path forward necessitates a balance between embracing the power of these technologies and retaining the crucial role of expert human judgment in medical decision-making.
In the realm of brain tumor detection, visual recognition systems are demonstrating a remarkable ability to map these tumors with 85% accuracy—a significant leap beyond traditional manual methods. This precision has the potential to revolutionize early interventions and improve patient outcomes.
While human analysis of brain images can be painstaking and prone to error, AI-driven systems offer a speedier and more consistent approach. The reduced variability in diagnoses across different experts is a clear advantage, though it's important to note that this doesn't eliminate the need for experienced radiologists to oversee the process.
These automated tools can directly impact treatment plans by offering precise tumor boundaries. This granular level of detail allows neurosurgeons to develop more effective surgical strategies.
Furthermore, visual recognition models leverage their ability to sift through vast amounts of data, revealing subtle patterns and characteristics that might be easily missed by the human eye. These insights can be crucial in understanding tumors better and shaping treatment options.
It's encouraging that these systems can often be seamlessly integrated into existing clinical workflows, working in tandem with clinicians rather than replacing them entirely. This collaborative approach is particularly important for maintaining a balance between AI and human expertise.
However, these automated systems can process images remarkably fast, sometimes within minutes, which is a major benefit, especially in urgent situations requiring immediate action. This efficiency can facilitate quicker and more timely decisions.
Despite the undeniable benefits, a key concern remains: the potential for over-reliance on these systems. There's a growing debate about the critical balance between automation and human interpretation. Complex cases often necessitate the nuanced judgment that only human experts can bring to the table.
The training of these systems relies heavily on high-quality, meticulously curated datasets, where each image is carefully labeled with ground truth information. This structured data provides a foundation for learning, and the accuracy of the system is directly tied to the quality and diversity of the training data. It's a critical component in ensuring that these AI systems function reliably.
The continuous development of these algorithms is noteworthy. They are constantly updated and improved, enabling them to incorporate new medical insights and technologies, ensuring they remain on the forefront of brain imaging advancements.
However, it's crucial to acknowledge that algorithms can be influenced by biases present in their training data. This is especially concerning if the data lacks diversity or representation of various demographic groups. Ensuring fairness and equity in diagnostic accuracy across populations requires careful monitoring and validation of these models to mitigate any inherent biases.
7 Transformative Applications of AI in Scientific Image Analysis From Neural Networks to Visual Recognition in 2024 - AI Powered Microscopes Track Cell Movement in Real Time at Stanford Labs
Researchers at Stanford are using AI-integrated microscopes to track the movement of cells in real-time. This allows for a much more detailed study of complex cell interactions, like those that occur during neuroinflammation. The microscopes utilize advanced AI algorithms to automatically locate and follow individual cells during different microscopy experiments, representing a major advance in cellular biology. Furthermore, new AI software designed for transmitted light microscopy allows researchers to analyze large amounts of live-cell data more thoroughly than ever before. These AI tools also help overcome some of the challenges associated with keeping cells alive while observing them under a microscope. The ability of AI to observe the dynamic changes in cell shapes provides unprecedented insights into the workings of various biological processes, cementing AI's role as a valuable tool in biomedical research. While this technology is promising, researchers still need to validate its accuracy and ensure it produces reliable results in various experimental settings.
At Stanford, researchers are employing AI-powered microscopes to track cell movement in real-time, pushing the boundaries of how we study intricate cell-cell interactions like the complexities of neuroinflammation. These microscopes, enhanced by sophisticated image processing algorithms, can analyze thousands of frames per second, allowing us to observe the subtle dance of cells in a way previously impossible without significant manual intervention.
This capability is especially valuable in understanding dynamic biological processes, uncovering how cells respond to their environment and each other. The beauty of these AI-powered systems is that they can automatically identify and classify different cell types and behaviors, greatly streamlining the data collection process for cellular biologists. Moreover, the integration of AI has elevated image quality, reducing the noise and artifacts that can make manual analysis challenging. This leads to more dependable and reliable experimental results.
Now we can witness and investigate phenomena like cell migration, division, and interaction with a level of detail previously unattainable. This provides unparalleled insights into processes as diverse as cancer metastasis and immune responses. It's like having a microscope with a built-in 'expert' that can continuously process and extract information from the deluge of visual data it produces.
Naturally, this enhanced capability comes with a need to generate and manage vast datasets. However, this is also an opportunity. The data, in turn, can be used to further refine machine learning models, generating a continuous feedback loop that improves the accuracy of cell tracking over time.
One concern though is that the algorithms rely heavily on well-annotated training datasets. The quality of the initial data directly impacts the effectiveness of these systems, and building these training datasets can be both time-consuming and resource-intensive.
The advantage of being able to conduct high-throughput imaging is substantial. It means that researchers can now explore a far wider range of experimental parameters, potentially leading to the discovery of novel biological phenomena that would have been missed before.
However, it's important to recognize that some within the scientific community still maintain a cautious stance toward complete reliance on AI for data analysis. They stress the importance of retaining human oversight to correctly interpret the intricate nuances of biological data.
It’s exciting to see how AI-powered microscopy is accelerating discoveries in cell biology. But it also raises new ethical considerations. As these technologies become more advanced, researchers will need to engage in thoughtful discussions about how AI might reshape the future of biological investigation. It's definitely a fascinating development, and we are just starting to explore the wide-ranging possibilities.
7 Transformative Applications of AI in Scientific Image Analysis From Neural Networks to Visual Recognition in 2024 - Machine Learning Models Identify 150 New Microorganism Species Through Image Analysis
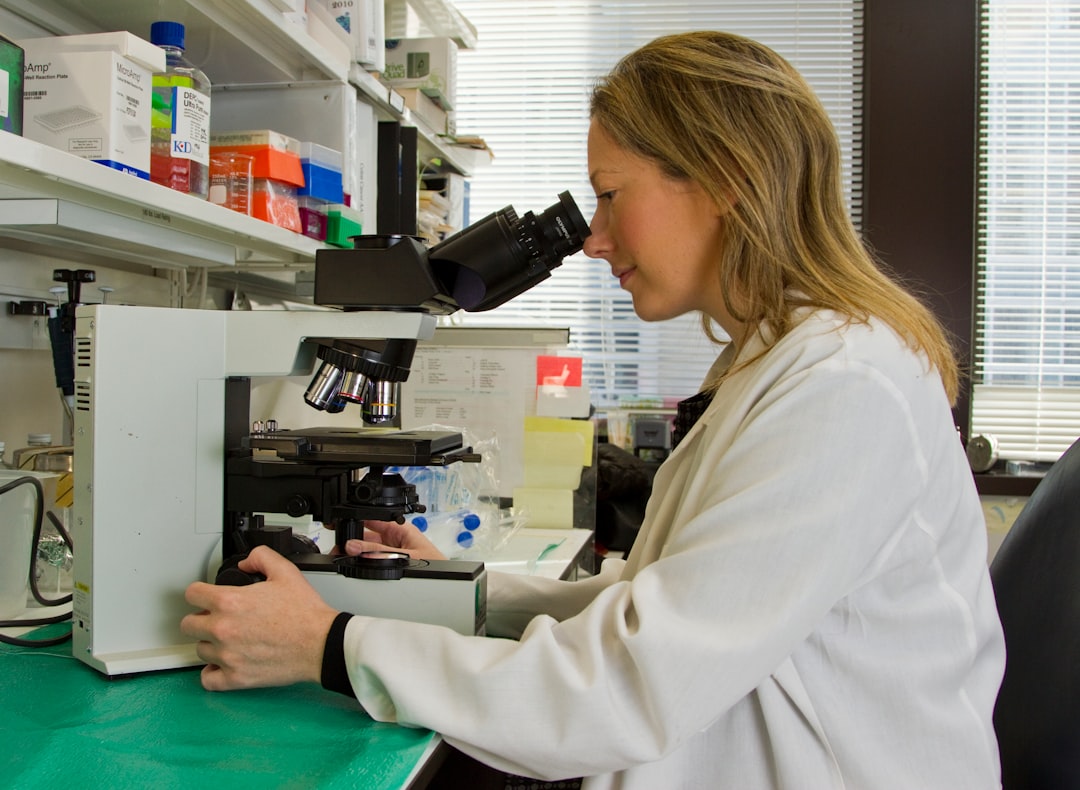
Machine learning models are now playing a pivotal role in the discovery of new microorganisms. Through advanced image analysis, researchers have identified 150 new species, showcasing AI's potential to revolutionize the field of microbiology. This progress is fueled by tools like DeepBacs, which simplify the process of analyzing microscopic bacterial images using neural networks, even for researchers without a deep understanding of AI. The automation of tasks previously reliant on human microscopists not only increases efficiency but also offers the possibility of higher accuracy in identifying pathogens, a vital aspect for clinical diagnoses and research. Specialized software like BiofilmQ further empowers researchers by enabling detailed visualization and analysis of complex biofilm structures, expanding our understanding of their role in different ecosystems. The integration of these AI techniques signifies a shift in how we study microbial communities, accelerating research and increasing our understanding of their diversity and behavior. While this is exciting, the development and validation of reliable AI models are still crucial for ensuring robust and accurate results across different studies.
Machine learning models have demonstrated a remarkable ability to identify 150 novel microbial species using image analysis. This achievement highlights the potential of AI for automating and accelerating taxonomic classification, a traditionally laborious process. The core approach often relies on convolutional neural networks, which are particularly adept at extracting intricate patterns from complex images, thereby enabling automated species identification without requiring extensive human intervention.
Beyond simply recognizing species, these models can also predict potential functional roles based on visual characteristics. This capability is potentially game-changing for researchers hoping to quickly understand the roles these microbes might play in a variety of ecosystems. The sheer volume of image data generated by modern microscopy—easily hundreds of thousands of images in a single experiment—renders manual analysis a significant bottleneck. This is where AI steps in, enabling much faster processing and analysis.
The performance of these algorithms is closely linked to the diversity and quality of the training datasets. Researchers have found that models trained on a wide range of microbial images tend to perform significantly better when classifying new, unknown samples. This is in contrast to traditional methods, which rely heavily on the expertise of trained microbiologists for visual classification. AI-based methods, by their very nature, are able to continuously refine their classification strategies as they encounter more data, effectively becoming 'smarter' over time.
Moreover, the speed of this process is now allowing for real-time identification of microbes, a crucial need in clinical environments where prompt diagnostics are essential for treatment decisions. Machine learning tools have the capacity to expand our understanding of microbial diversity in challenging-to-sample environments like deep sea or extreme climates, where traditional sampling methods might be impractical or insufficient.
However, it's critical to acknowledge that these systems can be prone to overfitting—a scenario where the model performs exceptionally well on the training data but poorly on novel data. Therefore, rigorous validation and testing are needed to ensure that these AI tools maintain a high level of accuracy in real-world scenarios. The most promising path forward lies in close collaboration between microbiologists and computer scientists. Microbiological expertise ensures that the machine learning tools are appropriately tailored to address the true challenges and biological significance of these classification tasks. This collaboration is crucial for navigating the complexities of microbial classification and integrating this technology effectively into current research practice.
7 Transformative Applications of AI in Scientific Image Analysis From Neural Networks to Visual Recognition in 2024 - Automated Pattern Detection Reduces Skin Cancer Misdiagnosis by 47% in Clinical Trials
AI-driven systems for automated pattern recognition have demonstrated a notable ability to reduce skin cancer misdiagnosis, achieving a 47% decrease in error rates during clinical trials. This is a significant advancement considering the global prevalence of skin cancer, where it accounts for a substantial portion of all diagnosed cancer cases. Deep convolutional neural networks (DCNNs) have played a key role in this progress, as they can effectively analyze and classify skin lesions, which can be visually challenging due to the similarities between different types. Furthermore, newer models like SkinNet14 showcase the potential for using low-resolution images, which speeds up analysis and reduces the need for extensive training data. These developments not only contribute to early detection of skin cancer, a crucial factor in improving patient outcomes, but also signal a major shift in how skin cancer diagnosis and management are approached in clinical practice. However, it's important to emphasize that the continued evaluation and validation of these AI models remain essential. We need to ensure they are reliable and effective when implemented in broader, real-world clinical settings.
Clinical trials have shown that automated pattern recognition can significantly reduce skin cancer misdiagnosis, with a 47% decrease observed in some studies. This is really promising, suggesting AI might play a large role in improving both diagnosis and patient care.
These algorithms, often based on convolutional neural networks (CNNs), are specifically designed to handle the complex visual features found in skin lesions. CNNs excel at detecting subtle patterns that may be difficult for human eyes to distinguish, even for experienced dermatologists. This ability is crucial for differentiating between benign and cancerous growths.
Traditionally, skin cancer diagnosis has relied on dermatologists' interpretations, which can vary significantly between individuals. Automated systems offer a level of standardization, potentially reducing this subjectivity and leading to more consistent diagnoses across different practitioners and locations. This standardization is particularly important for fairness and equitable outcomes.
One of the key benefits of AI-driven skin cancer detection is its speed. These systems can rapidly analyze thousands of images, speeding up the screening process in high-volume clinics and potentially reducing wait times for patients. This efficiency can help in managing patient flow and ensuring timely care in busy dermatology settings.
However, the reliability of these systems is critically tied to the quality and diversity of the training datasets. These datasets need to include a wide variety of skin lesion types and be meticulously annotated by experts. The models' ability to generalize to real-world scenarios relies heavily on the quality of this training data. It's a bit of a chicken and egg problem: we need robust models to improve data annotation, but robust models come from good data annotation.
Despite the automation, it's important to emphasize that these systems are not intended to replace dermatologists. Rather, they serve as powerful decision-support tools, providing a second opinion, if you will. This collaborative approach maintains the crucial role of the human expert while leveraging AI's computational power.
It's quite interesting that these tools might even help in dermatological education. Because the algorithms are designed to show how they reach a conclusion, they offer a unique way to study diagnostic reasoning, helping to improve knowledge and skills among clinicians.
Researchers are continually developing these systems to identify a wider range of skin conditions. Moving beyond melanoma to include conditions like dermatofibromas or squamous cell carcinoma will expand the clinical utility of these tools, leading to a more comprehensive approach to skin health.
Studies are showing that combining AI with a human dermatologist's expertise leads to significantly improved patient outcomes. This highlights the benefits of integrating these tools into existing clinical practices. It’s a win-win situation: AI adds speed and efficiency while dermatologists maintain the final decision-making responsibility.
Ultimately, AI has the potential to revolutionize personalized dermatology. By gathering and processing a patient's unique data, these systems could provide tailored recommendations for skin health management and preventative measures based on individual risk factors. It's an area with exciting potential and warrants continued research.
7 Transformative Applications of AI in Scientific Image Analysis From Neural Networks to Visual Recognition in 2024 - Image Enhancement Algorithms Reveal Previously Invisible Details in Astronomical Data
Astronomical data, often teeming with subtle details, can now be explored more effectively with advanced image enhancement algorithms. These algorithms, leveraging AI-driven approaches like deep learning and Convolutional Neural Networks (CNNs), are enhancing our understanding of the cosmos. They can extract features and patterns that were previously difficult or impossible to discern, improving image clarity and helping us identify faint objects or subtle structures in the universe. While these tools offer tremendous potential for new discoveries, we must also be mindful that their accuracy and effectiveness can vary depending on the quality of the data they're trained on, and they shouldn't replace the critical role of human interpretation in scientific research. Ultimately, this intersection of AI and astronomy is a powerful example of how we are pushing the boundaries of scientific understanding, uncovering new insights about the intricate workings of the universe.
AI is increasingly being used to enhance astronomical images, revealing details previously hidden within the vast datasets collected by telescopes. This is a powerful tool for researchers trying to understand the universe, particularly when dealing with faint or complex objects.
For instance, detecting exoplanets, those planets orbiting other stars, becomes easier with noise reduction and emphasis on subtle changes in light patterns. The challenge is that these changes are often masked by stellar fluctuations and noise. Image enhancement techniques can help tease out these signals, improving the chances of detecting new exoplanets.
Similarly, visualizing large-scale cosmic structures like galaxy filaments and the distribution of dark matter is aided by these algorithms. The sheer complexity and faintness of these features often make them difficult for the human eye to interpret, but enhancement techniques can improve their visibility, providing a clearer understanding of how the universe is structured.
Another important benefit is that these algorithms can stitch together data from different telescopes and wavelengths, creating a more comprehensive view of astronomical objects. This is particularly helpful for understanding the spatial relationships between different aspects of a phenomena—say, a star and its surrounding nebula.
Beyond visualizing structures, image enhancement is helping refine spectroscopic data. Improving the clarity of spectral lines provides astronomers with more accurate measurements of the chemical makeup and physical properties of celestial bodies. This has been particularly useful in identifying the elements and conditions in distant galaxies.
What's also exciting is the potential for using these algorithms to revisit historical astronomical data. This might uncover transient events—like supernovae or asteroid impacts—that were initially missed due to the limitations of the available technology at the time. It's as if we can see the universe with new eyes, reinterpreting history with current tools.
Nebulae, the birthplace of stars, are another area where image enhancement shines. These algorithms help highlight the filamentary structures involved in star formation, structures which are easily lost in standard imaging due to their faintness. It's amazing to consider how these advancements are giving us new insight into how stars form.
Furthermore, some of the most advanced techniques now allow for real-time processing of astronomical data. This means astronomers can observe and analyze transient events like gamma-ray bursts almost instantly, rather than having to wait for extensive post-processing. It's a huge advancement in how we observe the universe.
Moreover, these algorithms can be specifically designed to compensate for imperfections in observation techniques. This helps to reduce bias and provide a more accurate representation of celestial objects. It’s a critical step in minimizing systematic errors in our interpretations of astronomical data.
Parametric restoration techniques are pushing the boundaries of image enhancement by reconstructing images from incomplete data. This is useful when dealing with the effects of atmospheric interference or sensor limitations—allowing for the creation of clear images from what might otherwise be blurry or fragmented observations.
It's not just professional astronomers who can benefit from these advancements. As the tools become more accessible, citizen astronomers and amateur astrophotographers can also use them to improve their data and contribute to research-grade observations. This is opening up astronomy to a much wider audience, democratizing the field and inviting everyone to take part in the process of discovery.
While still under active development, image enhancement algorithms in astronomy have already proved their value. These techniques are helping us to understand the universe in new and exciting ways, from discovering exoplanets to revealing the secrets of the cosmos. It's a fascinating area of research that promises to continue unveiling the universe's hidden wonders.
7 Transformative Applications of AI in Scientific Image Analysis From Neural Networks to Visual Recognition in 2024 - Deep Learning Systems Achieve 93% Success Rate in Identifying Cellular Structures
Deep learning systems have made significant strides in analyzing cellular structures, achieving a 93% success rate in identifying them from images. This is a notable achievement, demonstrating the power of AI in scientific image analysis. For instance, CellSighter, a specialized neural network, has been developed to classify cells directly from complex microscopy images, overcoming significant obstacles in identifying them. These advancements not only enhance our understanding of single-cell data but also have the potential to significantly improve research in fields like synthetic biology. The ability to automate complex image analysis tasks can greatly improve efficiency, but it's essential to acknowledge that challenges remain. Access to these tools isn't universal, and there's still room for improvement in experimental consistency across different research groups. As deep learning increasingly integrates into biological studies, we need to critically evaluate its reliability and ensure that the integration doesn't diminish the crucial role of human experts in the interpretation and validation of results.
Deep learning systems have recently achieved a noteworthy 93% success rate in pinpointing cellular structures within images. This level of accuracy is quite impressive, surpassing older methods. It seems to highlight the ability of these systems to parse and categorize intricate biological imagery with precision.
These advancements leverage convolutional neural networks (CNNs), which are especially designed to recognize complex patterns within cellular images. This design allows for the automated identification of cell types and functions without the need for substantial preprocessing or manual intervention by researchers.
The training process for these deep learning models typically requires vast datasets comprising millions of labelled cellular images. This is essential for enhancing their ability to generalize when encountering new, unseen data. This capability is critical for their dependability in actual biological research scenarios.
Interestingly, the performance of these models can be notably impacted by the makeup of the training datasets. If a dataset is biased towards certain cell types or lacks sufficient variety, the model’s ability to accurately identify less-represented cells can degrade. This introduces a potential concern regarding inherent biases that could creep into AI-based diagnostics.
Beyond simple identification, more sophisticated deep learning systems can offer insights into the behavior of cells over time. This capability provides invaluable data on dynamic processes like cell division, migration, and reactions to stimuli. This information is fundamental for a deeper understanding of a wide array of biological phenomena.
The integration of AI into cellular analysis appears poised to reshape the landscape of biological research. It has the potential to accelerate the pace of discovery in fields such as pathology and drug development. AI empowers researchers to process and analyze image data at a speed and scale previously unattainable, which has huge implications.
Researchers are increasingly exploring the use of AI to identify novel cellular phenotypes and unusual cell behavior. These explorations could pave the way for breakthroughs in our understanding of diseases like cancer, where abnormal cell activity is a major feature. AI insights could help in the tailoring of more specific treatment approaches.
Despite these encouraging advances, there's a lingering apprehension within the scientific community about over-dependence on automated systems. The need for skilled researchers to validate AI-driven analyses is regularly emphasized to ensure the correctness of interpretations and the maintenance of rigorous scientific practices.
As the field of deep learning continues to mature, we're seeing the development of hybrid models that blend AI with traditional methods. This approach is aimed at capitalizing on the strengths of each system, providing consistent reliability while preserving the valuable insights that come from seasoned researchers.
The use of AI in identifying cellular structures is laying the foundation for personalized medicine. The idea is that therapies can be specifically tailored based on the unique cellular characteristics uncovered through advanced image analysis. This development could usher in a new era in the practice of healthcare.
Upscale any video of any resolution to 4K with AI. (Get started for free)
More Posts from ai-videoupscale.com: