AI-Driven Photo-to-Video Conversion Analyzing Stable Video Diffusion's Impact on Content Creation
AI-Driven Photo-to-Video Conversion Analyzing Stable Video Diffusion's Impact on Content Creation - Stable Video Diffusion's Framework for Image-to-Video Conversion
Stable Video Diffusion introduces a new approach to image-to-video conversion by building upon the foundation of latent diffusion models. Instead of being confined to 2D images, it incorporates time-based aspects, effectively creating a framework for video generation. This involves adding temporal elements and refining the model with specific video datasets. With this, it becomes possible to animate still images, producing short video clips. Though the model can create videos with 14 frames at 576x1024 resolution, the quality of the generated animations isn't always consistent.
The model cleverly utilizes existing parts from Stable Diffusion 2.1, but introduces a special decoder that handles the unique demands of generating video, specifically dealing with flicker reduction. This innovation potentially impacts many areas such as content creation, marketing, and teaching. The decision to make the model open-source is significant. It offers a platform for researchers and developers to explore and further refine the technology, while simultaneously creating a space for the wider community to interact and provide feedback. This open approach may ultimately drive innovation and improve the technology in unexpected ways. Whether the creative community can leverage it in compelling ways remains to be seen, as some may find its capabilities limited or its output not entirely satisfactory.
Stable Video Diffusion builds upon the foundation of latent diffusion models initially developed for still images, but extends their capabilities to the temporal domain of video. It achieves this by incorporating temporal layers and fine-tuning on specialized video datasets, albeit relatively small. Essentially, it's been adapted to understand and generate videos instead of just single images.
The process of animating still images is possible with Stable Video Diffusion, however, the quality of results can fluctuate. Currently, it can produce videos of 14 frames at a resolution of 576x1024 based on an input image of the same size. This is achieved through the use of the Stable Diffusion 2.1's image encoder paired with a custom-built decoder that's aware of temporal dependencies, helping to reduce unwanted flickering effects that are common in early video generation efforts.
The wider goal of Stable Video Diffusion is to provide a toolset that can be applied across a spectrum of domains, including media production, entertainment, teaching, and promotional materials. Recognizing its potential, Stability AI has made it available to the research community under an open-weight preview license. This strategy encourages a collaborative environment for development and testing. Anyone interested in exploring its capabilities can interact with a demo through Google Colab, which lets people test how it turns images into video snippets.
By being open-source, it reflects a vision of making AI accessible for creative endeavors. However, users planning to utilize Stable Video Diffusion commercially need to review the associated guidelines on GitHub. It remains to be seen how this balance between open access and licensing controls will play out in the longer term.
AI-Driven Photo-to-Video Conversion Analyzing Stable Video Diffusion's Impact on Content Creation - Real-Time Video Generation from Still Images
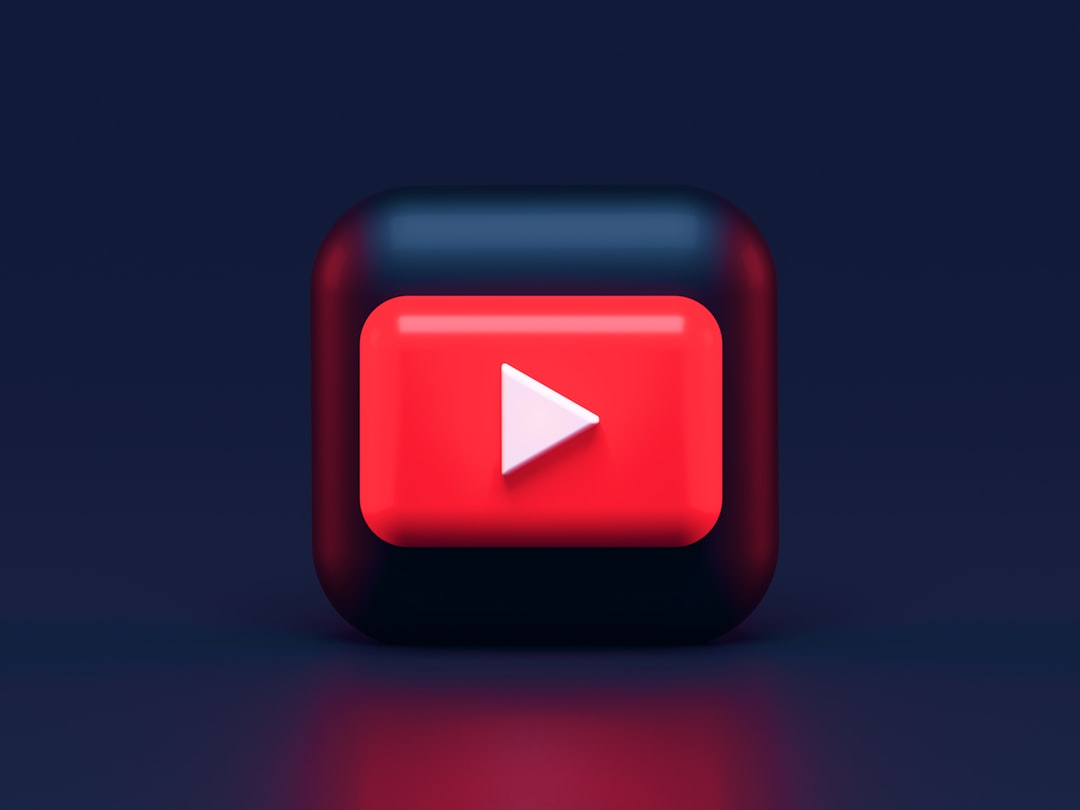
The ability to generate video content in real-time directly from still images represents a notable advancement in AI-powered content creation. These systems utilize sophisticated machine learning algorithms to analyze the relationships between still images and their corresponding video sequences, essentially learning how to bridge the gap between static and dynamic visuals. This allows for the creation of short video clips from single images, offering a new avenue for enhancing storytelling and engagement in various content formats.
However, the technology is not without its drawbacks. The quality of generated videos can vary, and maintaining visual coherence and authenticity can be a challenge. There are also questions that arise about the impact this technology has on the creative process and industry itself, particularly in terms of originality and attribution of creative work. The evolving capabilities of real-time video generation present a fascinating opportunity, but they also warrant careful consideration of their potential limitations and implications for the future of digital content creation. As the technology matures, it's likely that we'll see a greater understanding of its capabilities and also potentially a broader debate about its impact on artistic expression and originality.
Real-time video generation from still images utilizes AI algorithms that go beyond simply processing individual frames. These models now integrate a sense of time, allowing them to understand and predict movement across sequences of frames. This temporal awareness is key to creating smooth transitions and animations from static pictures. However, the current implementations, like Stable Video Diffusion, still have limitations. They typically produce videos with a maximum of 14 frames, which can be a constraint for those wishing to build longer, more elaborate narratives.
One of the clever aspects of Stable Video Diffusion is the specialized decoder designed to minimize flickering, a frequent issue in early video generation efforts. It's a significant step forward in creating visually stable output. But the quality of the output is also impacted by the data used to train these models. They need specialized datasets to learn about the temporal elements of video, and the quality and variety of this data ultimately limits the model's ability to generalize across diverse video styles.
The open-source nature of tools like Stable Video Diffusion is interesting. It allows the community to experiment through platforms like Google Colab. This creates opportunities to enhance the models but also shifts responsibility for quality control. While referred to as "real-time," performance can depend on both image complexity and available computing resources, leading to delays that may limit certain use cases.
Furthermore, some detail inevitably gets lost when transforming a static image into motion. This means the resulting videos might lack the sharpness of the original image, a potential concern for those seeking high fidelity output. The creative potential of being able to animate still imagery is clear, but it also raises questions about artistic ownership and originality when someone creates a moving piece based on a static one.
It's worth noting that the results of these models can vary greatly. Some outputs achieve a quality that's close to professionally produced video, while others can be quite basic. This variability makes it difficult to gauge the technology's practical impact across diverse situations. Finally, the successful integration of AI-driven video generation within existing professional workflows can be tricky. Many creators need consistent and rapid production, and incorporating these AI tools in those systems remains an ongoing challenge. It's an area ripe for research and development as we explore how AI can reshape the creation of video content.
AI-Driven Photo-to-Video Conversion Analyzing Stable Video Diffusion's Impact on Content Creation - Impact on Content Creation Workflows
AI-powered tools that convert photos into videos, such as Stable Video Diffusion, are transforming how content is created. These tools make it easier and faster to produce video content by simply animating a still image. This can be beneficial in streamlining production, potentially leading to faster content delivery. Yet, relying on AI to do this raises concerns about the quality of the final video. Inconsistencies and a lack of visual coherence can be issues. Furthermore, as more content creation tasks are automated, it becomes crucial to consider the potential impact on the originality of the work itself. The potential for AI-generated content to impact creative freedom needs careful consideration. While the potential for these technologies is undeniable, it's important to weigh the positive changes against the possible drawbacks to both the creative process and the integration of these tools within existing workflows.
The integration of AI into video production, particularly with features like photo-to-video conversion, is reshaping workflows by potentially reducing the time traditionally spent on editing. This can lead to faster project turnarounds and enable creators to focus more on the narrative and strategic aspects of their content.
However, one notable consequence of this technological shift is the uneven quality of the resulting videos. This can force a reassessment of established quality standards within the content creation industry, making it necessary to develop clearer guidelines for acceptable output, especially as AI-driven videos become more prevalent.
Furthermore, the automation inherent in these AI-powered tools presents a possible dilution of personal expression. Creators might become overly reliant on AI-generated results, potentially neglecting the development and use of traditional creative methods that truly convey their unique artistic vision.
Another point to consider is the crucial role of the data used to train these AI video generation models. The quality and diversity of these datasets have a profound impact on the reliability and overall consistency of the generated output. If datasets are limited or poorly curated, the model's ability to generate high-quality and varied results suffers, underscoring a key area needing further development.
It's also possible that tools like Stable Video Diffusion could democratize video creation. Individuals who may lack extensive technical skills could potentially generate visually engaging narratives. However, it's likely that the outputs from these tools would require refinement or enhancements from professional editors before they would be suitable for commercial use.
Real-time video generation could fundamentally change content discovery. Online platforms could leverage this to provide more personalized and engaging content tailored to individual users' preferences and behaviors, influencing marketing strategies across various industries.
The introduction of temporal awareness in AI models reflects a substantial shift in content creation workflows. Traditional processes, largely centered around static media, are being challenged to adapt to the changing dynamics of video storytelling.
The integration of AI tools into creative workflows creates a double-edged sword. While offering increased productivity, it raises concerns about the potential erosion of conventional artistic skills that have traditionally defined the creative process.
The open-source nature of tools like Stable Video Diffusion allows for community contributions which can be beneficial. However, it also brings into question quality control as contributions might introduce inconsistencies, impacting the reputation of content creators using these tools.
As AI-driven video generation progresses, questions surrounding originality and ownership of content will become more pressing. Determining the lines between creative inspiration and replication will become increasingly challenging in this new environment.
AI-Driven Photo-to-Video Conversion Analyzing Stable Video Diffusion's Impact on Content Creation - Comparing Stable Video Diffusion to Traditional Video Editing Methods
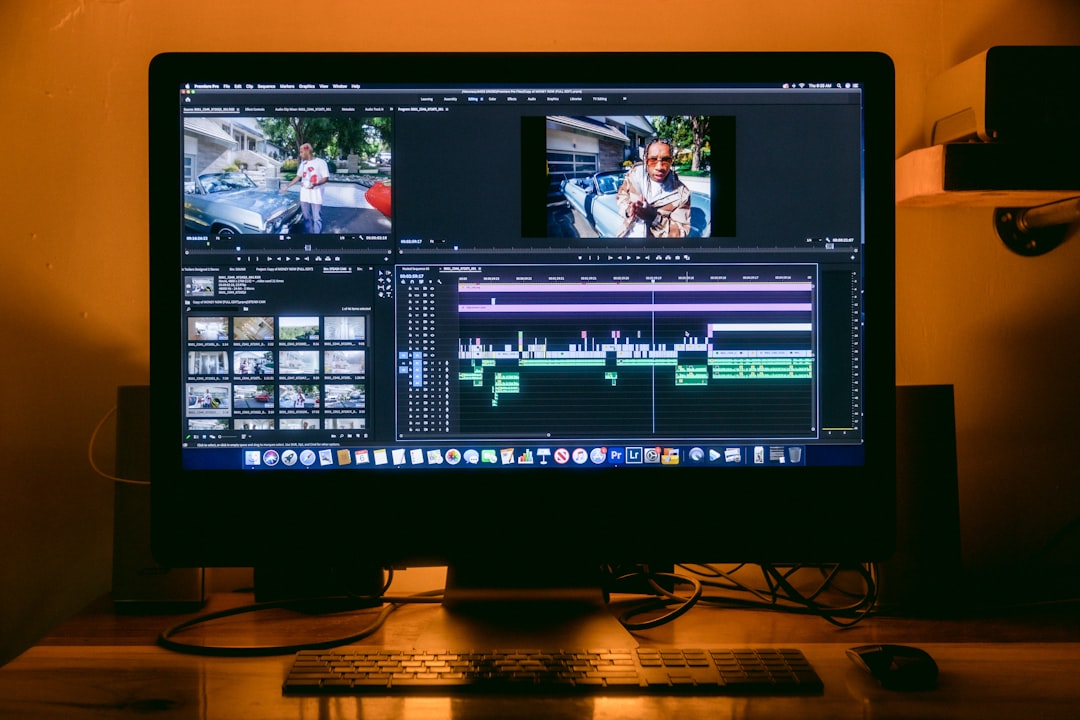
When comparing Stable Video Diffusion to conventional video editing, it's apparent that this AI-powered approach represents a notable shift in video creation. Traditional methods often face difficulties in maintaining visual consistency across frames, a challenge that Stable Video Diffusion tackles by incorporating temporal dependencies. This allows for a more seamless and coherent manipulation of video elements over time. Furthermore, Stable Video Diffusion streamlines the workflow by offering increased speed and flexibility, minimizing the need for extensive manual intervention compared to standard editing techniques. Despite the allure of quicker content production, the variation in video quality can be a concern, raising questions about the reliability of AI-generated content when juxtaposed with the polished visuals typically seen in professional video productions. The adoption of these AI-driven tools presents content creators with the interesting dilemma of integrating their capabilities while preserving the nuanced artistic elements integral to conventional editing methods.
Stable Video Diffusion presents a different approach to video creation compared to traditional methods, which often rely on specialized software like Adobe Premiere and require a significant level of technical expertise. With Stable Video Diffusion, anyone can potentially transform still images into short video clips, making video production more accessible to a broader audience. This ease of use comes with a trade-off, though. Traditional video editing often involves a considerable time investment, with skilled editors needing hours to achieve intricate edits. Stable Video Diffusion, while faster, currently generates limited frames, requiring us to reassess our expectations for project timelines.
Traditional video editing offers unparalleled control and precision over every frame and detail, unlike Stable Video Diffusion. Its automated nature, however, can lead to unpredictable results. The model's learning process, which depends heavily on training data, can inadvertently introduce visual imperfections that may diminish the quality of the final output, hindering the user's ability to produce truly polished work. Stable Video Diffusion has a degree of temporal awareness, meaning it can better understand the flow of a video compared to methods that focus on individual frame manipulation. While this approach can be efficient, traditional editing offers more fine-grained control over each frame, enabling more nuanced and artistic results, but this level of precision increases the time required for each project.
The rise of AI-powered video generation prompts questions regarding the very notion of originality. Traditional video editing practices empower the creator to inject their unique artistic style and vision into the process. AI, on the other hand, can blur the lines between creation and replication, raising concerns about the generation of derivative, rather than entirely novel, content. Traditional video creation, while requiring expensive software and hardware, tends to be a costly endeavor. Stable Video Diffusion, in contrast, leverages open-source frameworks, enabling a greater number of individuals to explore and engage with AI-driven video generation without needing to make a large upfront investment.
When crafting a complex narrative, traditional editing methods afford a much higher level of flexibility in controlling the pacing and arrangement of the story. This ability to finely tune the viewing experience isn't available in Stable Video Diffusion, which, at least for now, is constrained by a maximum of 14 frames per generated video. The increasing role of AI in video production might reshape established professional roles within the creative industries. While traditional workflows often require specialized personnel, such as editors and animators, AI tools like Stable Video Diffusion might streamline certain tasks and potentially lead to a reduction in the need for some of these positions.
While promising, the quality of videos generated by Stable Video Diffusion remains inconsistent. The output is not always reliable in maintaining a consistent quality, which contrasts sharply with the predictable minimum standard achieved by skilled editors using traditional methods. This highlights the ongoing need for improvements in the stability and reliability of AI-powered video generation. The potential impact of Stable Video Diffusion could also change the nature of video creation. It could shift the primary focus from honing technical editing skills to a more concept-driven approach. Creators might emphasize story and concept over painstaking manual edits, thus influencing what is valued in terms of content creation in the evolving digital landscape.
AI-Driven Photo-to-Video Conversion Analyzing Stable Video Diffusion's Impact on Content Creation - Ethical Considerations and Future Development of AI-Driven Video Tools
The rise of AI-driven video tools brings into sharp focus the ethical implications of their development and use. As these technologies become more sophisticated, it's essential that their design incorporates a strong ethical foundation. This involves thoughtfully addressing potential issues like safeguarding user privacy, respecting copyright protections, and preventing the spread of misleading or manipulated content. While AI can undoubtedly accelerate content creation and improve video quality, it's critical to acknowledge the inherent risks of algorithmic bias. The potential for AI-generated videos to perpetuate or exacerbate societal biases in representation necessitates careful scrutiny. Moreover, the ability of these tools to create realistic deepfakes raises troubling questions about trust and authenticity in digital content. It's becoming clear that a collaborative effort is needed, involving developers, users, and policymakers, to establish robust ethical guidelines that ensure the responsible use of AI in video production. The future trajectory of AI-driven video tools will depend on this ongoing conversation about technology, ethics, and governance. This intersection of innovation and responsibility will determine how these powerful tools are utilized and ultimately shape the future landscape of video content.
The rise of AI-driven video tools presents a fascinating yet complex landscape of ethical considerations. The ability of these systems to generate content resembling existing works raises concerns about copyright and originality. Determining the boundaries of intellectual property becomes murkier when AI-powered tools can create derivative content, potentially challenging traditional notions of authorship.
Furthermore, the training process for AI models like Stable Video Diffusion requires vast datasets, creating the risk of bias within the generated outputs. If training data isn't representative, the videos produced might reinforce societal biases or limit the diversity of artistic expression, ultimately impacting the inclusivity of the generated content.
The animation of static images also introduces questions about the nature of artistic authenticity. While these tools can mimic motion convincingly, some argue they lack the creative spark and genuine emotional depth found in human-produced art. This raises the broader question of art's value in an era of sophisticated AI-generated visuals.
Despite the promise of faster video creation, current limitations like the 14-frame cap for some systems restrict their applicability to more elaborate storytelling. This necessitates a reevaluation of narrative structure and pacing in video, highlighting the need for continued advancements in AI capabilities.
The open-source nature of tools like Stable Video Diffusion empowers user experimentation, but also introduces a potential for inconsistencies in output quality. Users with varying levels of skill and understanding might produce uneven results, presenting a challenge for the standardization and regulation of AI-generated content.
There's also the potential that the reliance on AI for video creation could lead to a decline in traditional editing skills within the creative community. The speed and efficiency of AI-driven tools may push creators to rely more heavily on automation, potentially leading to a decline in the use of nuanced storytelling techniques and artistic craftsmanship.
Another ethical concern revolves around the potential displacement of jobs in the creative sector. As AI streamlines production processes, some traditionally skilled roles might become less relevant, sparking conversations about workforce transition and the future of employment within creative industries.
Furthermore, AI-generated videos could shift audience expectations toward an even higher level of visual polish. This pressure for pristine aesthetic quality might impact creators' perspectives on their work, potentially emphasizing technical proficiency over conceptual development and impacting their creative processes.
The models underlying AI video generation could inadvertently perpetuate existing visual trends and conventions. It's essential that developers consider the ethical implications of their algorithms and their potential long-term effects on the visual landscape, preventing the reinforcement of established tropes and fostering diverse creative possibilities.
Finally, as these technologies advance, discussions around accountability become increasingly important. Determining liability if an AI-generated video infringes copyright or relies heavily on existing content is a complex legal and ethical issue, with the roles of the user, developer, and AI itself yet to be clearly defined. The ethical landscape of AI-driven video generation is undoubtedly complex and requires ongoing scrutiny and dialogue as the technology evolves.
More Posts from ai-videoupscale.com: