Upscale any video of any resolution to 4K with AI. (Get started for free)
AI-Enhanced Upscaling Techniques for Improving Football Video Quality in 2024
AI-Enhanced Upscaling Techniques for Improving Football Video Quality in 2024 - Machine Learning Algorithms for Resolution Enhancement
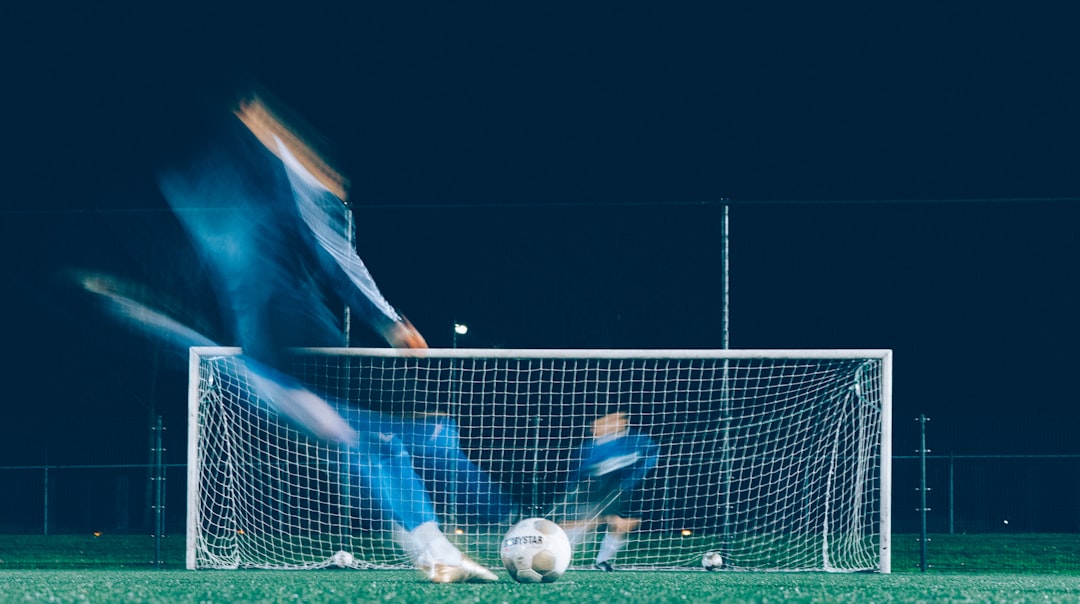
Machine learning has emerged as a powerful tool for improving video resolution, particularly in domains like football broadcasting where high-quality visuals are paramount. Methods such as Generative Adversarial Networks (GANs) stand out for their ability to significantly enhance image and video quality. However, using these methods to enhance resolution presents some complexities, especially when it comes to retaining fine details within images, such as the intricate textures of a football field or the rapid movement of players.
The field of deep learning is yielding innovative techniques to tackle these challenges, including newer recursive learning models that strive to refine the output quality of video enhancement processes. The growing desire for crystal-clear video content is driving the incorporation of these machine learning approaches, signaling a shift in how we achieve superior video quality. It's essential, though, to carefully consider the potential trade-offs between enhancing resolution and any subsequent decline in overall image or video quality. The ongoing research into applying machine learning algorithms to upscaling video holds the key to future advancements in improving visual quality across various applications.
Machine learning algorithms, especially those based on convolutional neural networks (CNNs), are rapidly changing the landscape of video resolution enhancement. While the potential for improvements is substantial, with some algorithms capable of increasing resolution by impressive factors, the quality and nature of the training data play a pivotal role in success. Algorithms trained on pristine, high-resolution data can struggle when confronted with lower-quality video, emphasizing the need for consistent and diverse datasets.
Generative adversarial networks (GANs) stand out as a particularly potent approach to super-resolution. Their ability to synthesize missing pixel information based on context opens up the possibility of remarkably accurate predictions even from low-resolution input. However, a significant challenge for all these approaches remains the preservation of natural motion in the output video. Since our eyes are so good at noticing even subtle inconsistencies, maintaining a smooth and coherent appearance in the enhanced video is crucial for successful upscaling. Techniques that leverage multi-frame analysis show promise, offering more context to make sharper, more faithful image predictions.
The computational cost of applying machine learning to video upscaling has dramatically reduced in recent years. The use of modern GPUs has enabled real-time enhancement of video streams, something that would have been nearly impossible just a short while ago. This improvement has wide-ranging implications for the usability and practical application of these techniques. Beyond the speed increases, researchers are also exploring the use of transfer learning for broadening the applicability of these algorithms. Adapting models trained on one specific type of sports footage to other sports or video domains holds considerable potential.
However, it is important to acknowledge the limitations of the current approaches. The presence of artifacts like blurring or ringing is a common outcome in enhanced videos, requiring ongoing development of sophisticated algorithms aimed at reducing these distortions. Additionally, the biases that can be introduced through machine learning algorithms are becoming a concern. If training data is not diverse enough, the upscaled footage could inadvertently skew the representation of players or teams, underscoring the need for careful dataset selection and evaluation.
Finally, the exploration of unsupervised learning techniques in video upscaling is exciting. These methods hold the possibility of significantly reducing the reliance on labelled data for effective training. Achieving this would be a major step towards developing more versatile and robust super-resolution algorithms. While still in its early stages, it shows a possible route to resolve certain challenges in creating effective upscaling models for the future.
AI-Enhanced Upscaling Techniques for Improving Football Video Quality in 2024 - Real-time AI Processing for Live Football Broadcasts
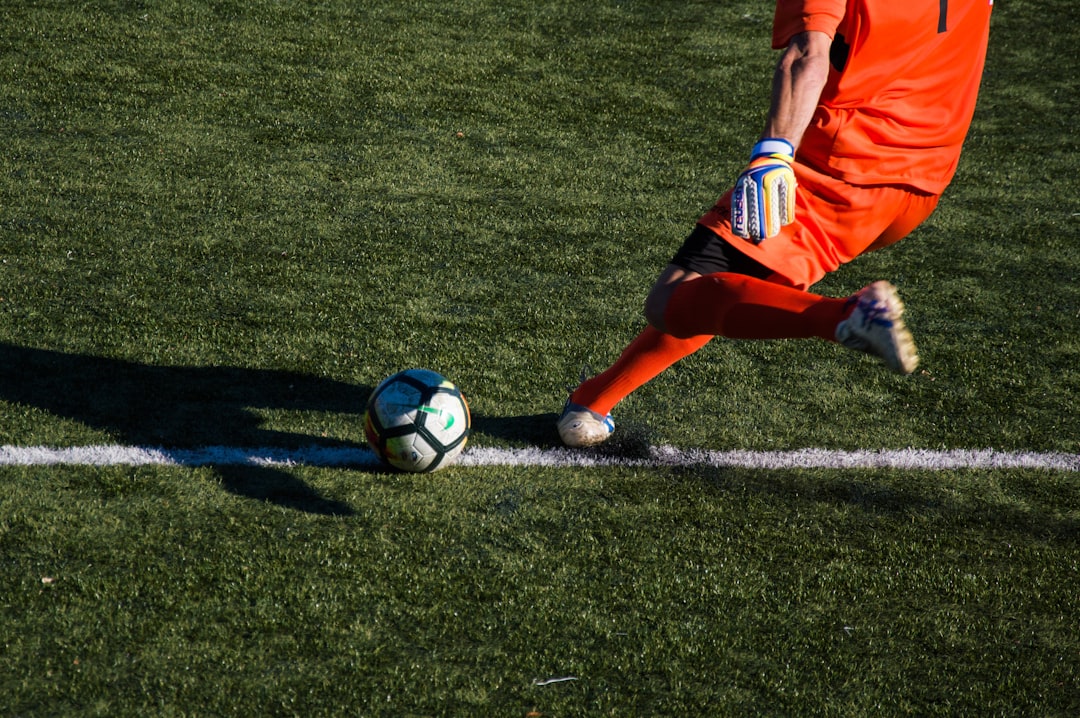
The integration of real-time AI processing is reshaping how live football broadcasts are produced and experienced. AI's role extends beyond simple image enhancement, offering a range of capabilities that elevate the overall viewing experience. For example, AI can intelligently identify and remove distractions in the camera feed, like unwanted objects or people intruding on the shot. Furthermore, AI can dynamically adjust the lighting, color, and audio elements in the broadcast to counteract fluctuating conditions, thus ensuring a more polished and consistent aesthetic.
These capabilities are underpinned by advanced algorithms that analyze the video in real-time, producing immediate insights that benefit both broadcasters and viewers. This constant data processing informs decisions that can enhance the coverage, from automated adjustments to instant replays and slow-motion analysis of crucial plays. Essentially, AI acts as a behind-the-scenes collaborator, improving the quality and impact of the broadcast in a multitude of ways.
While undeniably promising, it's important to acknowledge that the application of AI for real-time processing in live sports is still in its early stages. While aspects like eliminating camera distractions are being explored, more complex and sophisticated integration is needed to fully realize the potential of AI to revolutionize the live football broadcast experience. There's room for substantial refinement and expansion of techniques, with a future where AI plays an even more central role in capturing and sharing the excitement of football matches.
AI's integration into live football broadcasts is steadily expanding, enabling a range of novel capabilities. For instance, AI algorithms can now process player movements at impressive speeds, such as 200 frames per second, providing immediate feedback and data that enriches the viewer experience. We're seeing AI systems becoming adept at recognizing and categorizing plays as they occur, identifying key tactical maneuvers, and generating real-time analytics useful for commentary and viewer insights. It's fascinating to see how AI can simultaneously segment individual players and their actions, allowing the creation of personalized content, such as focused highlight reels of specific players during a game.
Beyond player actions, AI is also being utilized to capture and emphasize moments of heightened audience emotion within the stadium, giving a richer narrative context to the broadcast. Some systems even automatically adapt camera angles and focus during matches based on player positioning and the unfolding game, offering a more dynamic viewing experience. There's intriguing potential for AI to forecast game outcomes using past game data, creating probabilistic narratives that can further enhance critical game moments.
Social media sentiment and audience reaction during a match can now be monitored and analyzed in real-time by AI, providing broadcasters with information that might inform shifts in content and commentary to better match the audience's immediate interests. The development of voice recognition powered by AI allows for instantaneous translation of player interactions, broadening the appeal of broadcasts to a more diverse audience. Combining real-time AI processing with augmented reality techniques lets viewers see tactical formations and player stats directly on screen, adding another dimension to the analytical side of watching a game.
However, this rapid advancement also prompts some caution. There's a risk of over-reliance on AI algorithms leading to misinterpretations of game situations or player intentions. It's important to remember that a balanced approach, integrating human expertise alongside automated systems, remains crucial to ensuring the accuracy and integrity of broadcasts. The continued research in this space presents opportunities for refinement of these systems to enhance viewer experience and understanding of the beautiful game while navigating the inherent challenges of relying on automated decision-making in such a complex and dynamic environment.
AI-Enhanced Upscaling Techniques for Improving Football Video Quality in 2024 - Noise Reduction and Artifact Removal in Fast-paced Gameplay
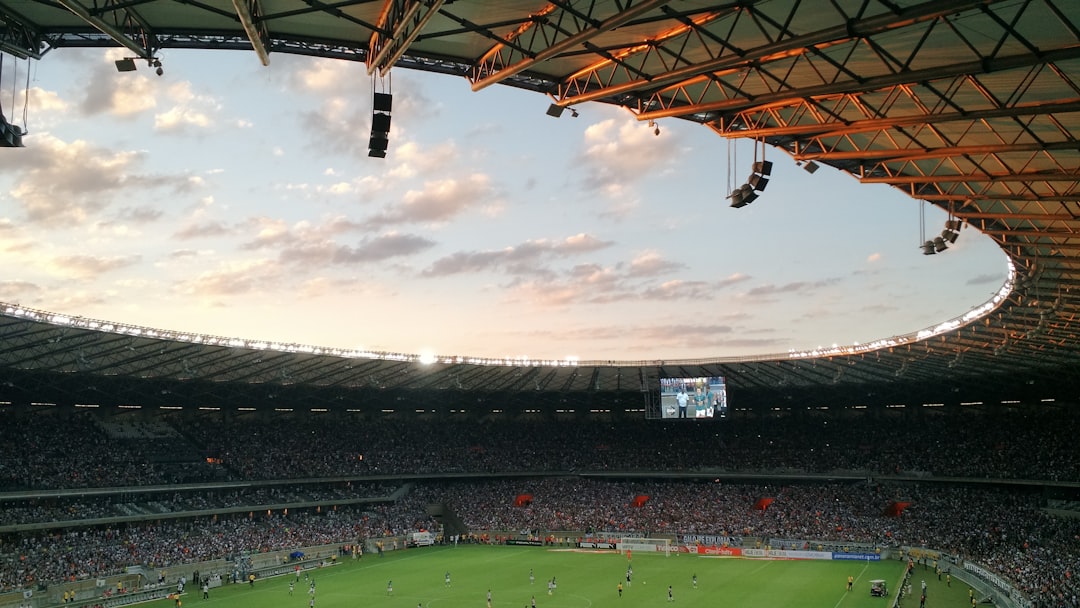
Fast-paced football action necessitates clear video quality, making noise reduction and artifact removal essential for a compelling viewing experience. AI-powered techniques are increasingly being used to combat common issues like blurring and excessive grain, which can detract from the energy of the matches. These methods cleverly differentiate between crucial visual details and distracting noise, leading to real-time enhancements that seamlessly integrate into fast-moving game sequences. While these AI approaches demonstrate promise in improving clarity and stability, challenges remain in preserving natural movement without inadvertently creating new visual imperfections. This underscores the ongoing need for sophisticated algorithms that can refine the upscaling process. The path forward involves carefully balancing enhancements with authenticity to fully capture the spirit of the sport without compromising the integrity of the action.
In the realm of enhancing football video quality, achieving smooth and clear visuals during the fast-paced action is crucial. Noise reduction and artifact removal become particularly important in this context, as they can significantly impact the viewer's perception of the game.
Techniques that leverage multiple frames, referred to as temporal noise reduction, have proven effective in smoothing out inconsistent pixel data. This approach not only reduces noise but also helps to maintain the fluidity of motion, a vital aspect of conveying the excitement of the game. We're also seeing a surge in advanced spatial filtering methods, like bilateral filtering, which intelligently differentiate between noise and actual movement. This selectivity allows for targeted enhancement or blurring, producing sharper visuals without compromising the integrity of the on-field action.
Researchers have also begun to explore motion vector analysis to further refine noise reduction. By pinpointing areas with significant player movement, algorithms can strategically prioritize noise reduction in these key sections of the video, ensuring crucial actions remain clear while minimizing disturbances in less critical frames. The development of artifact identification algorithms offers another promising direction. These algorithms automatically detect and rectify common visual artifacts like ghosting and banding, improving overall quality without the need for manual correction. This automation is particularly important for real-time broadcasting, where efficiency is paramount.
Maintaining the sharpness of edges is vital to retaining the dynamism of football. Newly developed edge preservation techniques are designed to preserve the high-contrast areas surrounding players and the ball, ensuring that the action is consistently discernible against noise. We also see that frequency domain processing, leveraging techniques like Fourier transforms, has the potential to effectively distinguish between noise and important motion frequencies. By isolating these elements, we can selectively filter noise while preserving the fast-paced nature of the game and preventing distracting distortions.
Adaptive noise reduction approaches are gaining popularity as they dynamically adjust their parameters based on the activity level within a given scene. This adaptation is particularly helpful for preserving a balance between smoothness and clarity, especially during quick transitions characteristic of football matches. By capitalizing on the inherent redundancy found in consecutive video frames, advanced algorithms can reconstruct high-quality visuals even from lower-resolution input. This ability to minimize compression artifacts is essential for ensuring that fast-paced moments remain clear and detailed.
However, the practical application of these noise reduction methods is often limited by the need for real-time processing. Many techniques are optimized for speed, sometimes at the cost of in-depth analysis, which can compromise video quality during intense periods of play. Interestingly, the design of effective noise reduction strategies increasingly involves considering the inherent limitations of the human visual system. By tailoring algorithms to capitalize on aspects like motion perception and contrast sensitivity, we can maximize viewer satisfaction while simultaneously minimizing the distractions introduced by noise. It's an intriguing area of exploration where the complexities of signal processing meet the nuances of human perception.
In conclusion, noise reduction and artifact removal are becoming increasingly sophisticated tools for enhancing football video quality. The ongoing research into these areas promises to further refine these techniques, leading to a more compelling and engaging viewing experience. However, the inherent challenges of real-time processing and balancing speed with quality continue to push researchers towards more innovative and effective solutions for the future.
AI-Enhanced Upscaling Techniques for Improving Football Video Quality in 2024 - Smart Frame Interpolation for Smoother Motion
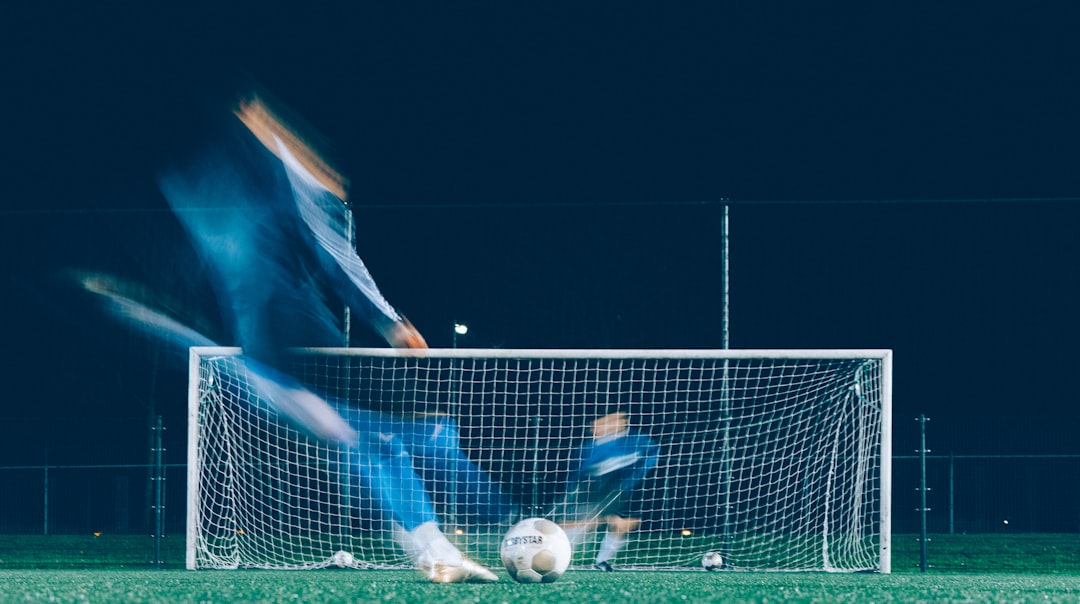
**Smart Frame Interpolation for Smoother Motion**
Smart frame interpolation is a technique that creates new frames between existing ones in a video sequence, which makes the motion appear smoother. This is especially useful for sports like football, where rapid movement can lead to blurring. By essentially increasing the frame rate, interpolation can make motion look more fluid and allows for better slow-motion effects. This results in a higher-quality video with enhanced visual fidelity.
Despite the clear benefits, there are still challenges related to the quality of the interpolated frames. AI models can sometimes struggle to generate frames that blend seamlessly into the original video, particularly when there's significant object motion or objects blocking each other. Maintaining a sense of natural movement is crucial to avoid a distracting or jarring experience. The quality of frame interpolation and its ability to stay faithful to the original footage is a critical factor, so it's an area that requires ongoing development. Given the increasing demand for higher quality video, it will be crucial to keep refining these methods in the future.
Smart frame interpolation, a technique that aims to create smoother motion in videos by generating new frames, faces some intriguing technical challenges. One major hurdle is ensuring consistent motion, especially during rapid movements like players' sprints. If the algorithms don't properly account for non-linear motion, it can result in a jarring effect called ghosting, which disrupts the smoothness we aim for.
Deep learning models offer promising avenues for predicting intermediate frames, using surrounding frames as context. However, these models are computationally intensive and require a massive amount of high-quality training data to minimize errors in capturing motion. The challenge of generating these intermediate frames in real-time, especially during live broadcasts, presents a significant hurdle. The speed of computation and rendering new frames can quickly become a bottleneck, particularly during the most exciting parts of the match when there's the most data to process.
Techniques involving analysis of multiple frames, sometimes called temporal analysis, have the potential to refine frame interpolation, but they can also add delays to processing. Finding that sweet spot between processing speed and accurate motion representation is a constant research area. Another issue arises from the inconsistent frame rates used by different football leagues. Algorithms trained on one frame rate may not perform as well on footage with a different rate, leading to inconsistencies in output quality.
There's a risk that if deep learning models are trained too extensively on one specific type of football, they might become overly specialized or "overfit" to that data. This can cause problems when encountering varied gameplay situations, like changes in weather, player interactions, and field conditions. Maintaining the illusion of natural, uninterrupted motion – temporal coherence – is key to a positive viewing experience. If the transitions between frames aren't smooth, it can create an unnatural, choppy effect. Thus, robust algorithms that prioritize this smoothness are crucial.
Frame interpolation sometimes introduces unintended blurring, especially during rapid sequences. Algorithms struggle to distinguish between actual motion blur and the artifacts from interpolation, leading to potentially misleading visuals. The impact of the resulting image quality isn't just about the technology, it's also about the viewer's perception. Even highly advanced interpolation techniques can fail to meet viewer expectations for naturalism and smoothness, suggesting that simply improving the technical aspects may not be enough.
There is exciting potential in combining frame interpolation with other AI video enhancement tools like noise reduction and artifact removal. However, making sure these methods work together seamlessly can be difficult. Synchronizing and coordinating these various steps adds another level of complexity to achieve optimal output quality. In conclusion, while promising, frame interpolation technologies still require careful development to overcome several limitations and ensure they enhance, rather than detract from, the experience of watching the sport.
AI-Enhanced Upscaling Techniques for Improving Football Video Quality in 2024 - AI-driven Color Grading and HDR Conversion
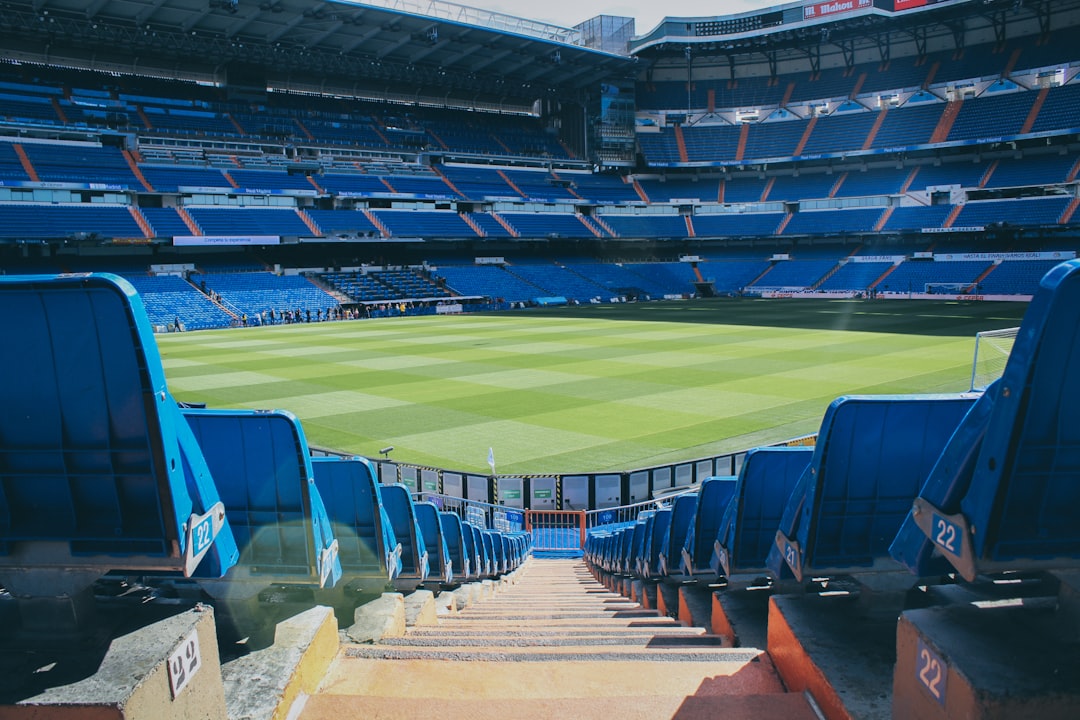
AI-powered color grading and HDR conversion are increasingly important for enhancing the quality of football videos. In 2024, AI algorithms are allowing for much more precise control over colors, creating specific atmospheres and enhancing the visual impact of broadcasts. Software like DaVinci Resolve and Filmora offer AI-driven tools that not only correct colors but also convert footage to HDR, making videos appear more vibrant and immersive. However, these techniques can sometimes lead to difficulties like keeping colors natural and consistent. This is an ongoing challenge as AI evolves to improve video quality, it is important to be discerning about the changes to ensure they actually improve the experience without altering the original content in a negative way.
The realm of AI is steadily transforming how we perceive and experience football video, and color grading alongside HDR conversion are no exception. AI is capable of dynamically adjusting a video's dynamic range, enabling a greater span between highlights and shadows. This is especially useful for football footage since lighting conditions can change dramatically during a match, making sure key plays remain visible despite shifts in exposure.
Furthermore, AI is being used to automate color grading processes. Algorithms analyze entire video sequences, learning optimal color palettes to enhance sports footage. This eliminates the need for a video editor to meticulously tweak each frame, leading to more consistent and appealing results. AI can even intelligently detect changes in a scene, like a shift from a defensive to an offensive play, and automatically adjust the color grading accordingly. This creates a more dynamic and immersive viewing experience, mirroring the ups and downs of the game.
However, the conversion to HDR, while improving image quality and color vibrancy, has limitations when the original source footage is of low quality or not captured in HDR format. In these situations, AI struggles to achieve the best outcomes, often resulting in unnatural color transitions or banding.
Another significant challenge in fast-paced sports is maintaining consistent color grading throughout the video. Inconsistent colors between frames can be incredibly distracting to the viewer, so ensuring uniformity in these AI-driven approaches is crucial. Fortunately, advancements in real-time processing allow AI algorithms to adapt to shifting lighting conditions in the stadium, dynamically changing the color grading during live broadcasts, enhancing the quality and viewer experience.
Many AI systems used for HDR and color grading incorporate adaptive learning, which means they improve over time by analyzing and learning from a diverse range of footage. This flexibility is critical in ensuring high-quality results across diverse football broadcasts that often have vastly different conditions in terms of lighting, camera angles, and the field itself.
Interestingly, human perception plays a vital role in how AI color grading is evaluated. Our visual systems are remarkably sensitive to color contrasts, so well-designed AI can capitalize on this aspect to create a more satisfying experience. However, a fine line exists between enhanced colors and preserving the authenticity of the footage, ensuring the viewer does not feel that the image is distorted.
Color grading during HDR conversion can unfortunately create visual artifacts, like color fringes or halos, particularly at high-contrast areas. Thankfully, AI systems that analyze pixel data in real-time can effectively identify and mitigate these unwanted visual effects, resulting in higher overall image quality.
Looking ahead, there's potential for these AI-driven color grading systems to connect with existing game analytics. This integration could lead to automatic color adjustments linked to critical events on the field, making highlights even more impactful and adding a deeper layer of narrative to the broadcast.
These insights paint a compelling picture of how AI is reshaping the world of color grading and HDR conversion in football video production. While the field has made notable strides, challenges remain in balancing quality, authenticity, and the complexities of processing large amounts of information in real-time.
AI-Enhanced Upscaling Techniques for Improving Football Video Quality in 2024 - Automated Camera Angle Optimization using Computer Vision
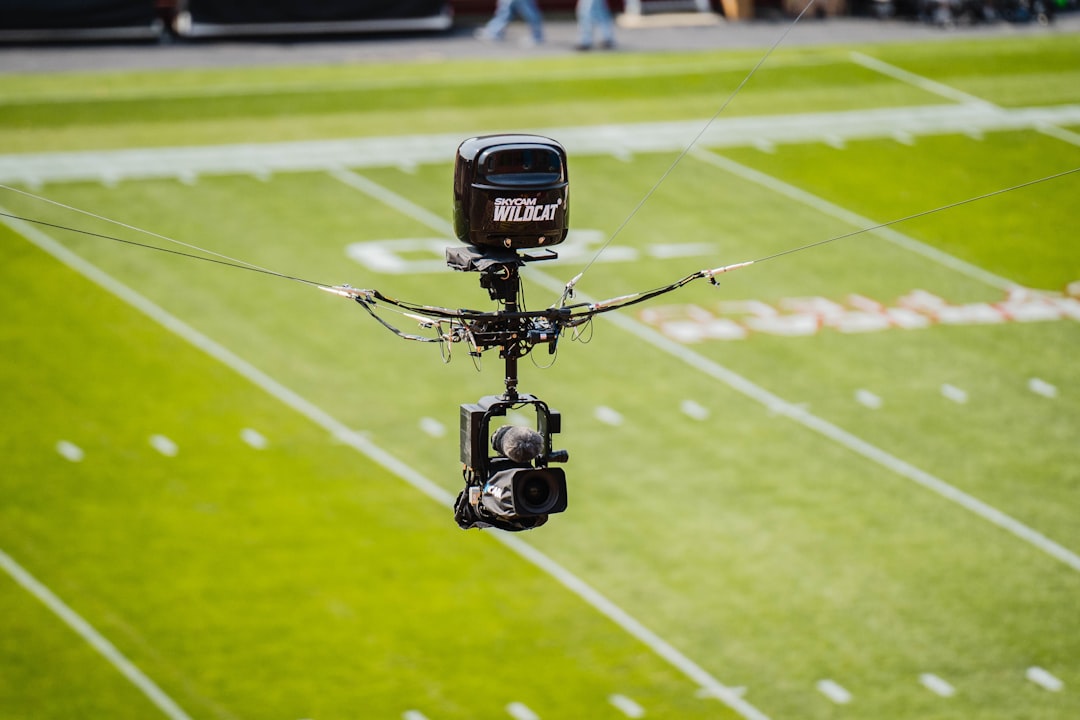
Computer vision is increasingly being used to automatically optimize camera angles during sports broadcasts, especially in football. AI algorithms can analyze live video and figure out the best camera positions and shot choices, ensuring key moments are shown clearly and accurately. This leads to a better viewing experience and makes production more efficient since it reduces the need for human camera operators. As this tech improves, it'll be vital that it can keep up with fast-paced plays and unexpected events on the field, leading to a more immersive viewing experience. However, it also brings up concerns about relying too much on automation versus the intuitive decision-making that human camera operators bring to live sports. There's a delicate balance to be found in this evolving field.
The concept of intelligent cinematography is finding a foothold in sports broadcasting, particularly football, by leveraging AI and computer vision to optimize camera angles. AI is being explored as a way to enhance the quality of video content by automating tasks previously done by human camera operators, improving efficiency and potentially offering more dynamic coverage. We've seen how AI can refine image and video quality in other ways, like increasing resolution and removing artifacts, but applying it to cinematography introduces a whole new set of possibilities for improving the viewing experience.
One interesting area is the ability to dynamically adapt the camera angle in real-time based on player movements and positions. This isn't just about zooming in on a key player, but actively predicting where the action is likely to go and shifting the focus. It’s similar to how a skilled human operator would react, but with the speed and consistency of an AI. Using past game data, we can build predictive models that help the camera system anticipate significant moments or plays. Sophisticated computer vision systems can track the trajectory of players, enabling the cameras to follow their likely path, thus keeping the focus on critical actions in a fast-paced game.
The challenge becomes coordinating multiple cameras to provide the best angles. These systems are being developed to switch seamlessly between different camera feeds, ensuring the viewer is never missing the most critical action. There are a lot of hurdles though, as algorithms still struggle with unexpected scenarios like sudden player movements or crowd interference. It highlights that there is a lot of room for improvement in these computer vision models, and the real-world environments of football matches can be chaotic and difficult to predict perfectly.
Interestingly, some automated camera systems can even analyze audience reactions on social media in real-time to understand viewer preferences. By monitoring these sentiment trends, the AI can adjust camera angles and focus to improve engagement, creating a more personalized viewing experience. These systems also incorporate collision detection algorithms to make transitions smoother, so there’s not a sudden jarring switch that disorients the viewer. By reducing the reliance on human operators, automated camera angle optimization can free up resources for other critical tasks during high-stakes matches.
Using AI in this way could also introduce new, more unique angles during a game. These can offer viewers fresh perspectives on tactical plays and maneuvers that are otherwise hard to see from traditional positions. When you combine AI-driven camera angles with augmented reality tools, the viewer gets a much richer experience. Imagine seeing real-time player performance stats or tactical formations overlaid directly on the screen during a game. It’s the kind of thing that can bring fans deeper into the strategy and analysis of the sport.
While this approach offers promise, there’s a long way to go. AI models still need significant refinement, especially to handle the unpredictability of live events. The integration of these tools with existing infrastructure and workflows needs careful consideration. It's a fascinating area that could change how football is broadcast and experienced, but it also underscores the need to approach these technologies with a balanced perspective, carefully weighing their benefits and drawbacks to ensure that the enhancements improve, not detract from, the core excitement and beauty of the game.
Upscale any video of any resolution to 4K with AI. (Get started for free)
More Posts from ai-videoupscale.com: