Upscale any video of any resolution to 4K with AI. (Get started for free)
AI Upscaling Advancements What to Expect by January 5, 2024
AI Upscaling Advancements What to Expect by January 5, 2024 - Real-time AI upscaling for live video streaming
The realm of live video streaming is witnessing a significant shift with the integration of real-time AI upscaling. This technology leverages artificial intelligence to enhance the resolution and clarity of video content in real-time, promising a more immersive viewing experience for audiences. While the initial implementations have focused on improving standard definition to higher resolutions, the future holds the potential for even more remarkable advancements.
The ability to upscale video in real-time presents a significant benefit for content creators and viewers alike. It allows for the delivery of higher-quality content without the need for pre-processing or significant delays. This is particularly impactful in scenarios where viewers expect instant gratification, like live gaming streams or breaking news events.
However, the technical challenges are substantial. Ensuring the upscaling process maintains a smooth and consistent viewing experience without introducing noticeable artifacts or lag is a complex problem that requires powerful processing capabilities. Despite these challenges, the progress is noteworthy. The future, as we approach January 5, 2024, hints at even more sophisticated algorithms and processing methods, potentially revolutionizing the way live streaming is experienced. It's plausible that we could see a seamless transition between resolutions or a more nuanced understanding of image content during upscaling, leading to genuinely stunning video results in real-time.
Real-time AI upscaling in live video streaming leverages convolutional neural networks (CNNs) to analyze the spatial and temporal relationships within video frames, enabling a more intricate reconstruction of textures compared to conventional upscaling methods. This can be particularly useful for reducing the demand for high-bandwidth connections, as lower-resolution streams can be transmitted with less data while still providing a satisfying visual experience. Certain implementations achieve impressive upscaling rates, potentially reaching up to 240 frames per second, resulting in remarkably fast processing without introducing noticeable delays during live broadcasts.
Intriguingly, real-time upscaling can also elevate the quality of content that might not inherently benefit from AI enhancements, such as footage captured with older cameras, lending a more modern, high-definition look. This suggests that the technology can potentially breathe new life into existing content archives. These systems are also surprisingly adaptable, employing different algorithms for varying content types. They can optimize the rendering quality for fast-paced action sequences differently from slower, more static footage, adjusting in real time to specific visual characteristics.
Evidence suggests a strong correlation between AI-enhanced video quality and improved viewer retention. This makes sense, as visually appealing content tends to keep viewers engaged, a significant metric for streaming platforms trying to keep their subscriber base. Some advanced algorithms go beyond simple resolution enhancements by predicting motion and stabilizing frames, which helps to minimize the blurriness and artifacts commonly seen in low-quality live video streams.
The use of real-time AI processing necessitates significant computing resources, typically requiring high-performance GPUs or specialized hardware. This creates a compelling incentive for innovation in processing technologies specifically for digital media. Despite its promising potential, many viewers are still unfamiliar with the intricacies of AI upscaling. This can lead to doubts about the authenticity of "live" streams, prompting questions regarding the boundary between genuine and enhanced content.
Looking towards the future, the implementation of real-time AI upscaling may spark innovations in live broadcasting techniques. We might see substantial changes in the way we experience events like sports, concerts, and other live formats, as high-quality streaming becomes accessible to wider, remote audiences. This potential transformation highlights how advancements in AI and video processing can redefine the future of live content.
AI Upscaling Advancements What to Expect by January 5, 2024 - Integration of AI upscaling in consumer-grade devices
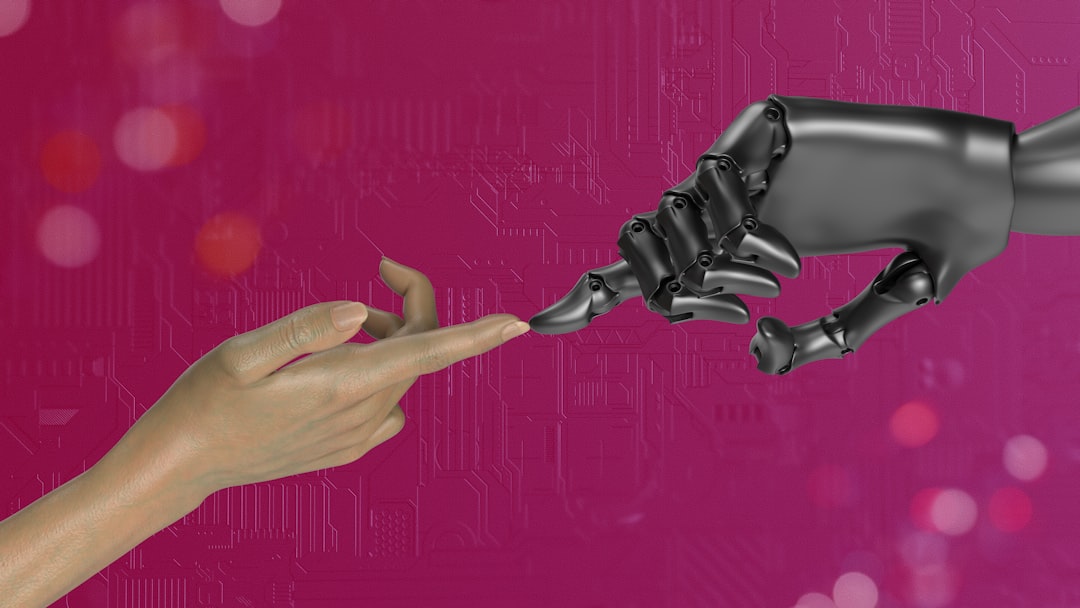
The integration of AI upscaling into consumer-grade devices is gaining momentum, particularly within the realm of gaming and streaming. Companies like NVIDIA and AMD are leading the charge, embedding AI capabilities into their latest graphics cards to enhance video quality. This trend signifies a shift towards sharper, more detailed images, especially when dealing with lower-resolution content. Streaming experiences could be significantly improved, with content appearing clearer and more vibrant.
Samsung has taken a different approach, introducing AI upscaling in their monitors. Marketed as beneficial for both gaming and productivity, these monitors aim to upscale lower-resolution streams to 4K quality. This move indicates a growing awareness of the potential for AI upscaling to become a standard feature for devices across various usage scenarios.
Despite the exciting possibilities, there's some hesitation. The practical implementation of these AI upscaling solutions in everyday settings remains a question mark. How efficiently do they perform? Are there any reliability issues or noticeable artifacts? Will users be able to readily adapt to and appreciate the enhanced visual experience? The answers to these questions will determine the true success of AI upscaling's adoption across a broader range of consumer devices.
The integration of AI upscaling into consumer-grade devices is an exciting development, although not without its challenges. We're seeing powerful graphics cards from NVIDIA and AMD that heavily emphasize AI capabilities, leading to noticeable improvements in video quality, especially for streaming. NVIDIA's RTX cards are pushing boundaries with AI-powered upscaling and HDR conversion, making content appear clearer and more vibrant. AMD is also in the game, with their FidelityFX Super Resolution (FSR) technology expected to receive a significant AI-driven boost soon.
Beyond dedicated graphics cards, other companies are jumping in. Adobe's VideoGigaGAN demonstrates the potential for significant resolution increases, even up to 8x, greatly impacting how we handle older, blurry videos. Samsung's foray into monitors with integrated AI upscaling positions them as flexible devices for both gaming and productivity, aiming to transform lower-resolution streams into 4K-like quality.
NVIDIA's SHIELD devices, along with new streaming TV boxes from other manufacturers, offer a glimpse into the future of video consumption. They allow users to directly compare basic and AI-enhanced upscaling, highlighting the technology's potential. Samsung's approach utilizes machine learning to analyze vast amounts of video, generating optimized filters for superior image quality.
While promising, the integration of AI upscaling in consumer devices isn't without its quirks. Devices frequently need robust processing power akin to high-end gaming systems, leading to increased heat and energy consumption, potentially impacting the device's lifespan. Interestingly, these algorithms are not one-size-fits-all. They adapt to the specific genre of video, applying different processing techniques depending on if it's fast-paced action or slower, dialogue-heavy content. This showcases AI's adaptability and potential.
Perhaps surprisingly, AI upscaling can bring new life to older footage. Think of classic films or even personal home videos. By applying AI enhancement, we can significantly improve the visual experience without the need for full-scale remastering, which is quite appealing.
However, there are hurdles. One challenge is achieving real-time processing with minimal latency. While many devices manage it well, keeping the delay under 100 milliseconds—particularly in live situations like gaming streams—remains important. Sometimes, upscaling can introduce unwanted artifacts, especially with low-quality streams, so there's ongoing effort to improve algorithms that focus on reducing them.
Surprisingly, even slight AI enhancements can noticeably improve viewer perception. People tend to associate sharper images with better content, thus, these enhancements can impact viewer engagement and satisfaction. We're also witnessing how this technology is becoming more accessible. It's not just limited to high-end gear, gradually moving into mid-range devices, making high-quality video processing more affordable.
This technology is also becoming more sophisticated. Some systems dynamically adjust processing based on content complexity, using more processing power when needed and less when possible, thus optimizing performance. The quality of AI upscaling can be impacted by network speeds; faster internet allows for higher-resolution streams, but this technology also allows for a reasonable viewing experience even at lower bandwidths.
It's an exciting time, but also raises concerns. The ability to drastically improve video quality naturally raises questions about the authenticity of media. When we see AI-enhanced content in our everyday devices, it will be important to think about the distinction between genuine and enhanced imagery, raising ethical questions surrounding the integrity of content creation. We're at a juncture where technological advancements are challenging our understanding of what's "real" in our digitally enhanced world.
AI Upscaling Advancements What to Expect by January 5, 2024 - Advancements in preserving fine details during upscaling
AI upscaling is increasingly effective at retaining fine details during the enhancement process. Newer AI techniques are better at reconstructing the missing elements in lower-resolution images and videos, resulting in outputs that are sharper and more detailed while retaining textures and edges. Some newer systems are capable of significantly increasing resolution, in some cases up to eight times the original, all while maintaining a natural look and feel. Unfortunately, conventional upscaling techniques still struggle with detail loss, emphasizing the importance of AI upscaling for truly high-quality results. By early 2024, we expect even more progress in this area, with greater emphasis on ensuring fine detail is retained during the upscaling process. There's a growing demand for upscaling solutions that can produce remarkably improved images without sacrificing the subtle details that give them realism and richness.
The field of AI upscaling is making significant strides in preserving fine details during the upscaling process, going beyond simply increasing resolution. We're witnessing a fascinating shift towards more nuanced approaches that leverage the power of AI to understand and reconstruct intricate features within images.
One notable trend is the emergence of sub-pixel rendering techniques. These methods allow algorithms to consider fractional pixel information, resulting in sharper edges and enhanced clarity of fine details, something traditional methods often struggle with. There's a growing focus on content-aware scaling, where algorithms intelligently analyze images frame-by-frame and adjust the upscaling method based on specific features. This ability to adapt to content specifics allows for better preservation of textures and object contours compared to a 'one-size-fits-all' approach.
Interestingly, some researchers are exploring Fourier Transform methods. By analyzing frequency patterns in image data, these techniques aim to reconstruct intricate details while maintaining a natural look and feel, particularly valuable for images with subtle textures or delicate features like human faces. The use of sophisticated deep learning architectures, such as GANs, is another significant advancement. These models don't just upscale but also learn to generate new pixel data based on what they've seen in high-resolution images, leading to more impressive results in detail representation.
Multi-frame techniques have shown promise in recovering details lost during fast motion. By incorporating information from multiple consecutive frames, these algorithms can predict and reconstruct details that would otherwise be blurred, significantly improving the quality of dynamic scenes. Adaptive filters are also becoming increasingly common. These filters change based on the upscaled content, allowing for selective detail enhancement in specific areas while preventing unwanted artifacts in others.
The ability of these algorithms to dynamically adjust their processing intensity in real-time is another significant development. They can allocate more resources to intricate sections of an image while scaling back processing in simpler regions, leading to a more balanced visual output. Additionally, the field has seen significant improvements in preserving the size and shape of small elements within images. This 'size and shape invariance' during upscaling points to remarkable advancements in maintaining the integrity of fine subject matter.
There's also a fascinating trend towards incorporating virtual camera effects into upscaling technologies. This includes simulating depth of field and motion blur even in low-resolution footage, resulting in a more sophisticated representation of depth and clarity in images that previously appeared flat. Research is even pushing the boundaries with cross-domain techniques, where AI models trained on one type of imagery, like paintings or cartoons, can improve the upscaling of different types of images, such as photographs. This hints at a surprising adaptability and the potential to achieve detail fidelity across a wide range of image types.
While the field is progressing quickly, it's essential to remain mindful of the potential downsides. Questions about the authenticity of media in a world where upscaling becomes ubiquitous will inevitably arise. As we continue to explore these advancements, it will be important to consider the ethical implications of artificially enhancing imagery, especially in cases where it may blur the line between what is real and what is digitally enhanced.
AI Upscaling Advancements What to Expect by January 5, 2024 - Reduction in computational requirements for AI upscaling
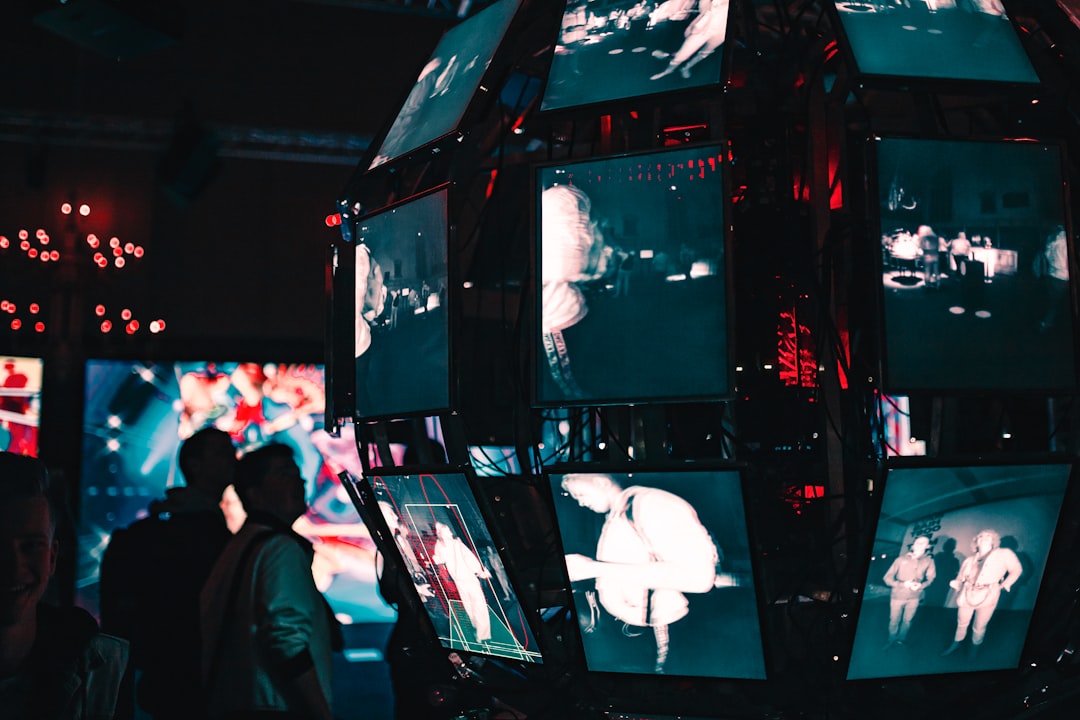
AI upscaling is becoming more efficient, requiring less computing power to achieve high-quality results. This is a big change because it means AI upscaling can potentially be used in a wider range of devices, from smartphones to laptops, without needing powerful, specialized hardware. This shift towards lower computational needs could make real-time AI upscaling more common, offering better video and image quality without noticeable lag or delays.
However, it's crucial to examine if this efficiency comes at the cost of output quality. We need to carefully analyze how the new, more efficient algorithms handle upscaling and if they can consistently produce high-quality results without creating unwanted artifacts or losing important details in images or videos. As these advancements continue, the key will be striking a good balance between the speed and efficiency of AI upscaling and the quality of the resulting enhanced content, ensuring the technology delivers a positive experience for users in diverse settings.
The field of AI upscaling is witnessing exciting developments in reducing the computational burden associated with high-quality output. Researchers are exploring methods to make AI upscaling more accessible and responsive, even on less powerful hardware. This is crucial for enabling real-time applications on everyday devices like phones and computers.
One approach involves using smaller, more compact AI models that can still generate impressive upscaling results. This shift towards streamlined models aims to reduce the overall processing demands, making them feasible for devices with limited computing capabilities. Techniques like sparse convolutions are becoming increasingly popular. These techniques allow the neural network to focus on only the most relevant parts of an image, effectively skipping unnecessary computations and significantly speeding up processing.
Another intriguing approach involves the use of pre-trained models. These models have been trained on other tasks, and their learned knowledge can be transferred to the upscaling task. This 'transfer learning' significantly reduces the need for extensive training on new datasets, leading to quicker deployment and implementation of upscaling systems. Furthermore, mixed precision training is gaining traction. This technique allows AI models to use less precise calculations during training and inference without sacrificing quality. This can dramatically increase the speed and efficiency of processing, significantly lowering computational demands.
Techniques that analyze temporal frames, that is, multiple sequential frames within a video, have also shown promising results. By incorporating information from previous frames, the need for repetitive calculations is minimized, leading to quicker upscaling. This is particularly beneficial for dynamic scenes where maintaining clarity and detail across rapidly changing visuals is essential. Likewise, multi-resolution processing methods are gaining ground. Here, an image is initially upscaled to a lower resolution before being further enhanced using higher-resolution techniques. This 'divide and conquer' strategy effectively splits up the workload, reducing peak computational requirements.
We are also seeing the development of algorithms with adaptive kernel sizes. These algorithms intelligently adjust their focus based on image complexity, allocating more processing power to areas with intricate detail while requiring less for simpler regions. This dynamic approach leads to more efficient resource utilization and faster processing speeds. Surprisingly, it's been found that, in some situations, models with lower memory demands can outperform traditional methods. This counters the long-held assumption that more powerful processing always leads to superior results.
Research into neural architecture search (NAS) offers another promising avenue. NAS techniques automatically optimize the design of neural networks for specific tasks. This is leading to the development of upscaling models that are both smaller and faster, crucial for facilitating real-time processing with impressive visual quality. The ultimate goal of these efforts is to enable the smooth operation of AI upscaling solutions on a wider range of devices, including those with limited processing power. This could greatly expand access to high-quality video enhancement across broader consumer markets.
While progress is exciting, questions remain. As with many AI advancements, there's a need to ensure that the efficiency gains don't come at the cost of output quality. It's also important to carefully consider the ethical ramifications as these AI upscaling tools become more accessible. As the line between enhanced and genuine imagery becomes increasingly blurred, there are important conversations to be had about media authenticity in our digitally enriched world.
AI Upscaling Advancements What to Expect by January 5, 2024 - Improved handling of motion artifacts in video upscaling
AI upscaling is steadily improving its ability to manage motion artifacts, a persistent challenge in enhancing video resolution. Previously, upscaling methods often resulted in noticeable blurriness or distortion when dealing with fast-moving content. This was particularly noticeable in action-packed scenes or videos with rapid changes in camera angles. However, the newest AI upscaling techniques are specifically addressing this issue. They aim to predict and compensate for movement during the upscaling process, smoothing out the transitions between frames and mitigating the occurrence of artifacts. The algorithms now employ more sophisticated neural networks to analyze frames within a sequence and predict how motion should look in the higher-resolution output. This more nuanced approach can significantly minimize the blurriness or jittery effect often seen when upscaling dynamic content. Looking ahead to early 2024, we expect to see an even greater reduction in motion artifacts, leading to a more natural and fluid viewing experience, especially when enjoying high-action or sports content. The future of AI upscaling seems poised to deliver a more refined visual experience, potentially preserving fine details and resulting in crisper and more realistic high-resolution video output. While still under development, it's reasonable to be optimistic about this particular area of upscaling technology.
The field of AI video upscaling has seen significant progress in managing motion artifacts, a persistent challenge in enhancing lower-resolution videos. Researchers have developed more sophisticated algorithms that are better at predicting motion across video frames. These algorithms analyze motion vectors, anticipating the movement of objects within a scene. This prediction capability is a crucial step in minimizing blur and sharpening fast-paced footage, leading to more visually impactful results.
Maintaining a consistent appearance of objects throughout the video sequence is another critical advancement. AI upscaling systems now place more emphasis on temporal consistency, ensuring that objects don't appear disjointed or abruptly change between frames. This is especially important for dynamic scenes, where inconsistencies can create an unnatural or unsettling viewing experience.
Interestingly, some new upscaling methods are even capable of adjusting the frame rate on the fly. This dynamic frame rate adaptation adjusts the processing based on the speed and intensity of motion within a video. This dynamic approach allows for smoother transitions between different levels of motion, ultimately improving visual fidelity while avoiding unnecessarily high processing demands.
Multi-frame integration has proven highly effective in enhancing detail preservation. Instead of working on just a single frame at a time, newer techniques simultaneously synthesize information from multiple, consecutive frames. This technique can capture a more comprehensive picture of motion and consequently retain significantly more visual detail compared to traditional, single-frame approaches.
Newer AI architectures, like those incorporating spatial-temporal convolutional networks, have shown tremendous potential. These networks are specifically designed to identify patterns and dependencies across both space and time in video frames. This combined approach allows for better management of artifacts, especially those generated by rapid and intricate movements.
Advanced algorithms have also focused on pixel-level refinement during upscaling. These algorithms meticulously examine minute pixel changes across frames to pinpoint specific areas for enhancement. This refined approach ensures that small, subtle details that might otherwise be overlooked aren't lost, leading to remarkably clear and precise results.
The ability of AI upscaling to adapt to the specific content of a video is another notable development. Algorithms can now intelligently analyze and adjust their approach based on the content being upscaled. For instance, action-packed scenes might be processed differently from dialogue-heavy scenes, optimizing the overall visual quality by prioritizing critical elements within specific video genres.
Furthermore, researchers have focused on the development of innovative artifact reduction techniques. These techniques are designed to actively identify and correct common artifacts introduced by fast movements like ghosting or trailing. This approach effectively reduces undesirable visual elements, leading to a more polished and natural viewing experience.
Upscaling low-bitrate streams, which often exhibit more pronounced motion artifacts due to compression limitations, has also seen improvements. Recent innovations in this area allow for enhanced quality of low-bitrate streams without significantly increasing the computational load, leading to an improved viewing experience, even when bandwidth limitations are present.
The achievement of real-time upscaling with minimal latency has surprised many researchers. This is a major leap, allowing upscaling to be integrated into a wide variety of live video applications, including live sporting events and game streaming. It showcases the rapidly evolving capabilities of AI upscaling technology.
While progress has been phenomenal, researchers continue to study and refine algorithms. Ensuring that these systems maintain a high level of visual fidelity while using minimal computational resources is an ongoing area of research. Moreover, as upscaling becomes more ubiquitous and seamless, questions surrounding the authenticity and integrity of video content will continue to be discussed and addressed. The potential for these powerful AI technologies to change how we consume video is fascinating, and understanding its implications will become increasingly crucial in the future.
AI Upscaling Advancements What to Expect by January 5, 2024 - Cross-platform compatibility for AI upscaling tools
By early 2024, a key trend emerging for AI upscaling tools is a growing focus on cross-platform compatibility. This means that developers are striving to create tools that function smoothly across a range of operating systems, like Windows, macOS, and Linux. Tools are increasingly being designed with open-source principles in mind, making it easier for users to leverage AI-enhanced image quality regardless of their specific device or platform preference. The goal is to make these powerful tools accessible to a wider audience. Furthermore, we're likely to see a standardization of support for AI-generated images, ensuring that upscaling tools can effectively enhance visuals created with neural networks and algorithms, maintaining visual clarity. Despite this positive trend, some concerns remain. It's crucial for users to consider potential inconsistencies in quality and overall performance across diverse platforms. This aspect will be crucial in determining whether these tools deliver reliable and consistent results across varying environments and usage scenarios.
The realm of AI upscaling is seeing a growing emphasis on cross-platform compatibility, making these tools increasingly accessible across a wider range of devices. It's fascinating how developers are striving for hardware versatility, aiming to provide high-quality image and video enhancements even on less powerful devices like smartphones and tablets. This reduces the need for users to have specialized or expensive graphics cards, making the technology more inclusive.
Some upscaling algorithms show impressive adaptability by adjusting their processing load based on the device's capabilities. It's intriguing that the same tool can offer different quality levels depending on the hardware it's running on. This means that the performance of AI upscaling can vary significantly across platforms.
Additionally, developers are exploring ways to optimize their tools for specific platforms. For example, leveraging features like GPU acceleration available on certain operating systems can lead to substantial performance increases, highlighting the significance of platform-specific optimization.
A critical aspect of good AI upscaling is cross-device consistency. Users appreciate the ability to experience a similar visual enhancement across devices, whether it's a laptop, desktop, or gaming console. This consistency is a key factor in maintaining user satisfaction.
The growing adoption of open-source frameworks for AI upscaling is fostering collaboration across developers. This collaborative spirit can help accelerate the pace of improvements in algorithm performance and ensures compatibility across a broader range of systems.
We also observe developers working on seamlessly integrating upscaling tools with a variety of video codecs. This integration is important for maintaining quality when users upscale content from multiple sources and different streaming services.
However, despite the advancements, there's a notable variability in processing latency across different platforms. This inconsistency can be traced back to the differences in how hardware handles real-time processing, influencing user perception of the upscaling's quality.
With the increase in mobile video consumption, it's no surprise that several tools are beginning to prioritize mobile-first optimization. This presents a challenge, as developers need innovative approaches to maintain performance while dealing with mobile devices' limitations on processing power and heat.
The field is also starting to grapple with the need for standardization. Researchers and developers are working on creating standard testing protocols for evaluating cross-platform compatibility in upscaling tools. This standardization is crucial for ensuring quality control across various devices and operating systems.
As AI upscaling tools become more commonplace across platforms, it's vital to examine the associated ethical implications. Users might struggle to discern the authenticity of video content, leading to discussions about transparency and user trust in digital media. It's a crucial point of discussion as the line between genuine and enhanced imagery continues to blur.
Upscale any video of any resolution to 4K with AI. (Get started for free)
More Posts from ai-videoupscale.com: