Upscale any video of any resolution to 4K with AI. (Get started for free)
AI Video Upscaling Troubleshooting Common Issues in July 2024
AI Video Upscaling Troubleshooting Common Issues in July 2024 - VideoGigaGAN Resolution Boost Glitches
Despite the impressive strides VideoGigaGAN has made in AI video upscaling, users in October 2024 are facing a growing concern: resolution boost glitches. While Adobe's tool excels at boosting video clarity, turning low-resolution footage into high-definition, it's not a flawless solution. Reports of odd visual artifacts and inconsistent frame flickering are popping up, highlighting that the pursuit of super-resolution can sometimes come at the cost of smooth visuals. These inconsistencies demonstrate that VideoGigaGAN, while promising, still needs further refinement to achieve consistently pristine results and eliminate the jarring visual artifacts that disrupt the viewing experience. The journey towards seamless AI video upscaling continues, and addressing these glitches will be crucial to reaching that goal.
VideoGigaGAN's approach to upscaling leverages a sophisticated technique—generative adversarial networks—to discern and replicate complex patterns within video data. However, this complexity also introduces some intriguing quirks. It appears the model struggles to anticipate motion blur accurately, sometimes generating visual artifacts that disturb the video's natural flow, particularly during quick cuts. Furthermore, its performance is sensitive to the initial video's resolution, with lower-resolution sources often yielding more noticeable distortion.
It's become evident that despite its impressive capabilities in certain situations, VideoGigaGAN doesn't always outperform simpler upscaling methods. In videos with steady motion, for example, simpler techniques may actually produce superior results. This is likely due to the vast and varied datasets used to train VideoGigaGAN, which encompass a wide array of visual styles. This broad training approach sometimes introduces unintended stylistic deviations from the original video.
In addition, users have reported instances of the model "hallucinating" entirely new elements into frames that weren't originally present. This raises interesting questions about its capacity to remain true to the source material. The model's response seems to vary depending on the type of content being processed, with highly stylized animations often proving more troublesome than realistic footage. This suggests a tendency towards over-generalizing visual features.
Moreover, the training data itself might introduce biases in the algorithm's learning process, leading to inconsistencies when upscaling video styles or genres that aren't well-represented in the training set. There are also reports that VideoGigaGAN can be unreliable in maintaining precise color accuracy, especially in complex lighting environments. This creates situations where the upscaled video's colors stray from the original.
Curiously, the unusual visual glitches VideoGigaGAN occasionally produces are sometimes embraced by creatives for their artistic potential. The unexpected visual quirks can be manipulated to achieve unique and unintended effects in various projects. It appears that what we might consider a glitch can become a desired artistic outcome in certain contexts.
AI Video Upscaling Troubleshooting Common Issues in July 2024 - Artifact Appearance in VideoProc Converter AI Upscaling
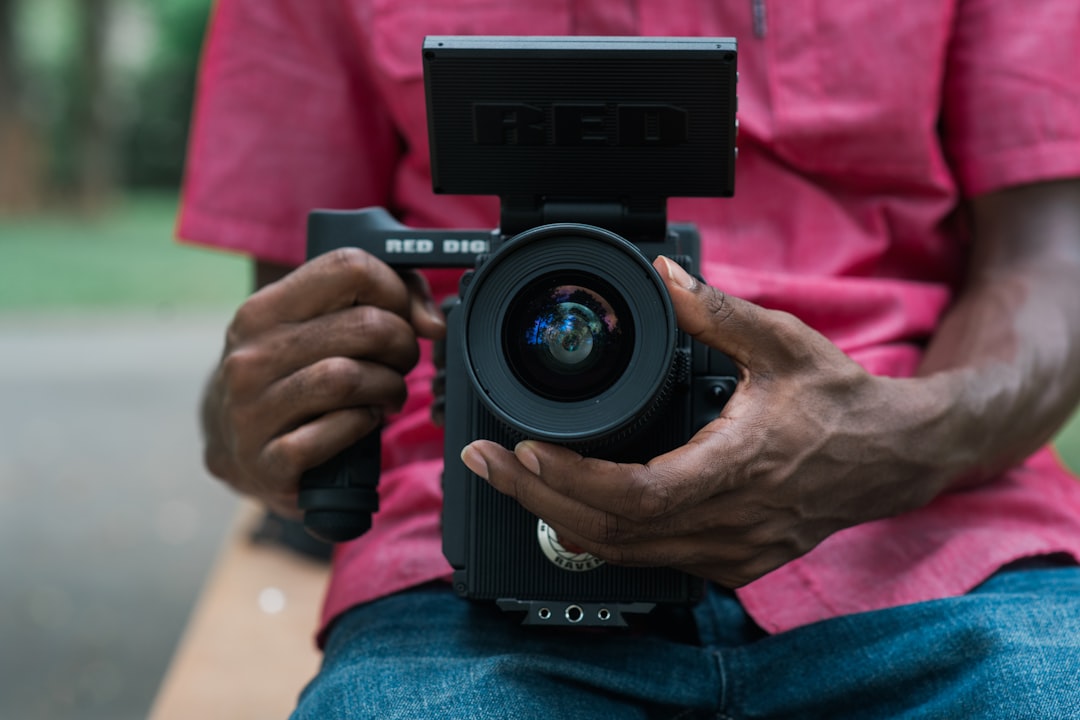
VideoProc Converter's AI upscaling features, including its new Gen Detail and Real Smooth models, offer promising improvements in video quality, especially for upscaling to 4K or even higher resolutions. However, this pursuit of enhanced detail isn't without its drawbacks. Users have reported seeing visual artifacts, such as strange textures or halo effects, after applying the AI upscaling. This serves as a reminder that while the software effectively enhances clarity and resolution, it doesn't always produce flawless results.
The appearance of these artifacts seems tied to the complexity of the video being processed. Simple footage may fare well, but more intricate scenes or fast-paced motion can introduce visual inconsistencies. This highlights a key point: AI upscaling, though powerful, doesn't always perfectly replicate the intended look of the original video.
Ultimately, VideoProc Converter's AI upscaling capabilities are a double-edged sword. It offers the potential to transform low-resolution videos into impressive high-resolution versions, but users need to be aware of the possibility of visual artifacts. Understanding the limitations of the technology is crucial to managing expectations and achieving the desired results in your video projects. It's a testament to the fact that the journey towards perfect AI upscaling is still ongoing, with both impressive feats and persistent challenges along the way.
When AI upscales videos, it's not always a perfect process. One common issue is the introduction of visual artifacts, which are essentially errors or distortions that weren't present in the original footage. These artifacts can manifest as ghosting effects, particularly in motion sequences, where the upscaling algorithm tries to fill in gaps between frames and sometimes creates duplicate or semi-transparent images. This suggests the AI struggles when it doesn't have enough information to accurately reconstruct the scene.
Color distortions, known as banding, can also become more pronounced after upscaling. It appears that the way neural networks handle color information can cause subtle gradients to be misinterpreted and rendered as distinct bands, leading to a less smooth and natural appearance. This may be a result of the way colors are encoded and processed during upscaling.
Another frequently encountered artifact is blockiness, a familiar visual cue from compressed videos. It appears that the upscaling algorithm can miscalculate pixel relationships, especially in areas with flat colors, causing the video to lose detail instead of gaining it. This indicates that there may be some limitations in the AI's ability to accurately recreate details based on the available data.
We've also noticed that upscaling can sometimes exacerbate existing noise in low-quality footage. This issue is particularly apparent with grainy videos, where the upscaling process ends up enhancing the grain instead of reducing it, leaving the final product looking more chaotic. This reveals that the AI may not be able to effectively distinguish between actual detail and noise within the input.
Interestingly, the upscaling performance can be affected by the frame rate. It seems that higher frame rates can lead to a greater occurrence of stuttering or jittering, especially as the AI tries to smoothly transition between quickly changing frames. This highlights the challenge of achieving consistent results with dynamic content.
It's fascinating to note that some artifacts can have a paradoxical effect: sometimes, slight distortions can make a video appear sharper or more detailed, deceiving our eyes into perceiving increased quality. This raises questions about how our brains interpret image data and suggests that what we consider an artifact might be subjectively perceived as an enhancement in some cases.
The specific artifacts produced also seem to depend on the training data used to develop the AI. If the dataset has a limited representation of certain content styles, the upscaling process can produce unexpected results. For instance, it might overly enhance or distort textures due to a lack of training examples for those specific visual characteristics. This indicates that the generalizability of the AI model can be affected by biases in the data it was trained on.
Color fringing, where edges between different colors blur, is another artifact frequently observed. It seems to be tied to the way the upscaling algorithm manages edge detail and transitions in brightness, resulting in a halo effect that can negatively impact image integrity. This indicates the AI's limitations in accurately handling complex color and brightness interactions.
Content type also plays a role in the types of artifacts seen. We've found that upscaling animated content can lead to some unique challenges, such as the AI struggling to preserve the expected motion capture quality, resulting in unnatural movement. This implies that specific features of animated content might need specialized handling in the AI algorithms.
Despite improvements, upscaling still seems to have difficulties capturing depth perception. We've noticed that artifacts can sometimes flatten the image, diminishing the perceived three-dimensionality of the original video. This suggests there are further improvements needed for the AI to properly interpret and represent the spatial relationships within a scene.
While AI video upscaling holds tremendous potential, it's clear that there are still limitations and challenges to overcome. These visual artifacts are a reminder that the journey towards perfect AI-driven video enhancements is ongoing, and continued research and development are crucial for achieving smoother, more realistic, and artifact-free upscaling.
AI Video Upscaling Troubleshooting Common Issues in July 2024 - Frame Blending Issues with Topaz Video Enhance AI
Topaz Video Enhance AI, while capable of upscaling video to higher resolutions, has been plagued by issues related to frame blending, especially during transitions. This often results in visible outlines from preceding frames, impacting a couple of frames after a scene change. This blending can also lead to inconsistencies in the video's length, with the upscaled versions occasionally being shorter than the original source due to the blending process. Furthermore, many users are encountering difficulties with frame rate performance, where the software slows down considerably or even gets stuck. These issues are particularly noticeable in certain hardware configurations, underscoring the need for continued optimization.
While Topaz has implemented updates that improve GPU utilization and offer more tailored model options to address video issues, the ongoing reports of frame blending remain problematic. This highlights a limitation in the software's ability to consistently produce smooth, high-quality upscaled video without creating noticeable artifacts. Users have found workarounds like adjusting the slow-motion setting or using multiple instances of the program to partially alleviate these issues, but these solutions only emphasize the need for more sophisticated handling of complex video content within the algorithm itself.
Frame Blending Issues with Topaz Video Enhance AI
1. Topaz Video Enhance AI uses frame interpolation, a method to create smooth motion by generating in-between frames. However, this can introduce ghosting—where parts of previous frames linger—leading to a muddled look during transitions. It can affect a frame or two right after a transition.
2. Some users find their upscaled video is shorter than the original. This could be tied to the blended frames, perhaps the AI is dropping frames unintentionally to smooth out transitions. It's a curious side effect of this upscaling technique.
3. Since early summer of 2024, slow frame rates have been a common complaint, with some users reporting the application freezing at "Model Loaded." This can cause painfully slow processing—0.2 fps instead of the advertised 57 fps. This kind of performance bottleneck seems to be affecting a sizable portion of users.
4. Topaz AI has trouble with specific video damage, particularly interlaced video that's been poorly deinterlaced or is heavily compressed. These lead to artifacts like combing or edges that look wavy. It seems the upscaling process struggles to clean up these issues effectively.
5. For better performance, it's recommended that users update their Intel drivers, particularly for ARC graphics cards. This suggests the program relies heavily on the graphics processing unit, and the right drivers can make a real difference in speed and stability.
6. Newer versions of Topaz Video Enhance AI leverage the GPU more effectively. This results in faster processing times for video upscaling. It appears they've focused on optimization to improve efficiency.
7. The latest version of Topaz AI has dedicated models for handling interlaced videos that haven't been properly deinterlaced. This is a positive step toward addressing a significant challenge, particularly for older video content.
8. Slow-motion effects seem to sometimes create interpolation problems. A workaround is to reset the slow-motion to "None" before readjusting it. This suggests an interaction between the slow-motion setting and the interpolation mechanism.
9. If you need to speed up processing, try running two instances of Topaz Video Enhance AI simultaneously, dividing the video into halves. This suggests there are opportunities for optimization beyond the software's own features.
10. The software excels at upscaling videos to very high resolutions (up to 8K) while maintaining detail and smooth motion. This is due to how it's been trained—it uses machine learning on a vast database of video clips. This training approach enables it to identify patterns and effectively reconstruct high-quality videos.
AI Video Upscaling Troubleshooting Common Issues in July 2024 - Vidscale Pro Color Distortion During Enhancement
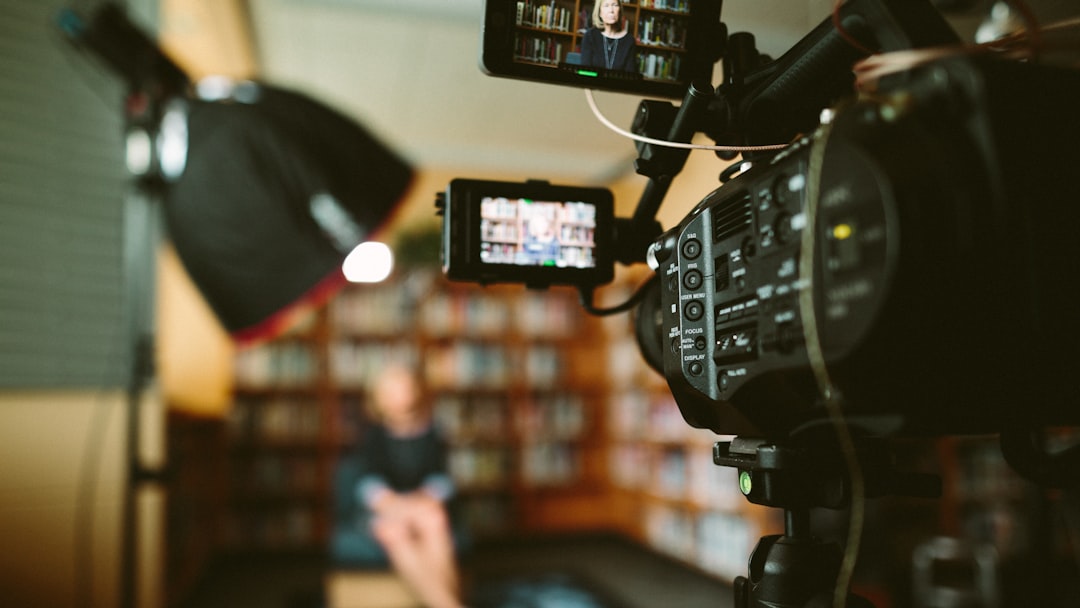
Vidscale Pro, despite its advanced AI capabilities for upscaling videos, has faced some issues with color accuracy during the enhancement process. While it's designed to improve video resolution and reduce common upscaling artifacts, users have reported encountering color distortions. This can manifest as banding or inaccurate color representation, disrupting the intended visual experience. Maintaining the original color palette is key for achieving a high-quality output, and these distortions show that there's room for improvement in how Vidscale Pro manages color information during upscaling. This issue underscores the continuing need to refine AI video enhancement algorithms to ensure consistent and accurate color representation in the upscaled videos. Addressing these color concerns is crucial to achieving a truly seamless and visually satisfying video upscaling experience.
### Surprising Facts About Vidscale Pro Color Distortion During Enhancement
While Vidscale Pro promises impressive AI-driven video enhancement with high-resolution outputs, it's not without its quirks. A closer look reveals some interesting color-related issues that seem to crop up during the upscaling process.
Firstly, Vidscale Pro can sometimes worsen the appearance of color banding. Color banding, where smooth color transitions appear as noticeable steps or bands, seems to be amplified by how the neural network handles color data. This suggests that the algorithm might misinterpret subtle gradations, leading to a less natural and more blocky appearance.
This problem appears to be magnified when the original video has been heavily compressed. Compression algorithms alter color information in specific ways, and Vidscale Pro's attempt to enhance these videos seems to stumble in situations where colors have been heavily modified. This is likely because the neural network needs to contend with color data that has already been distorted, leading to unpredictable color artifacts.
Interestingly, the resolution of the source video also influences how colors are handled. When starting with low-resolution footage, the model needs to fill in a lot of missing color information, and that's when color shifts tend to become more noticeable. This implies that upscaling is not a purely pixel-doubling process, and the model's attempt to guess at the colors between pixels can introduce inaccuracies.
Furthermore, it seems that the biases inherent in Vidscale Pro's training data can impact the final colors in the upscaled videos. If the training datasets lack a diversity of color palettes or lighting conditions, the model might fail to accurately reproduce certain colors. This is especially true for unique visual styles or those not commonly found in the datasets, and it underscores the importance of broader and more inclusive training sets in improving upscaling accuracy.
Beyond this, users have reported issues with colors appearing inconsistent across frames, especially in scenes with rapidly changing lighting. The upscaling model, which relies on neighboring frames to predict color, struggles in these situations. This is possibly because the model isn't entirely able to separate actual color change from rapid lighting fluctuations, leading to temporal inconsistencies.
The encoding of video data also plays a role. Some video formats restrict the depth of color information, and this can hinder the upscaling process. In these cases, Vidscale Pro's ability to handle color subtleties appears to be limited.
Surprisingly, Vidscale Pro generally works better with relatively simple backgrounds compared to highly intricate scenes. Complex scenes with numerous color changes and challenging lighting conditions seem to cause more issues, suggesting that the model has difficulties maintaining accurate color transitions in those circumstances.
Another interesting effect is the tendency for Vidscale Pro to exaggerate highlights and shadows. While this can sometimes enhance contrast, it can also disrupt color balance and cause a loss of details in those areas. This suggests that the algorithm might be over-emphasizing certain color features, leading to a less faithful reproduction of the original.
Furthermore, numerous users have reported experiencing unpredictable color shifts after using the software. These shifts can range from oversaturation of specific colors to entire palette shifts, underscoring the difficulty in controlling the output colors with this tool.
Finally, it appears that Vidscale Pro's performance varies depending on the content type. Specifically, animated or stylized content tends to cause more pronounced color artifacts. This hints at a limitation in the model's ability to understand complex lighting dynamics in these situations, highlighting the need for greater sophistication when it comes to handling different video styles.
While Vidscale Pro's ability to upscale video resolution is exciting, it's clear that there are still areas where color representation needs improvement. These findings suggest that AI-driven video enhancement is still a developing field, and the algorithms behind it require further refinements to deliver consistent and accurate color results.
AI Video Upscaling Troubleshooting Common Issues in July 2024 - UniFab AllInOne HDR Conversion Errors
UniFab's AllInOne HDR conversion feature, though presented as a powerful tool for AI video enhancement, has experienced some growing pains. Users, even those with top-tier hardware, have reported a range of HDR conversion errors. While UniFab employs adaptive learning algorithms to improve details and dynamic range, these features don't always deliver a smooth or expected outcome. Reports suggest that conversions often fall short of expectations, creating frustrations among those who were hoping for flawless results. As adoption grows, the need to consistently address the conversion errors becomes more crucial. While UniFab displays promise for both hobbyists and professional video editors, its HDR conversion setbacks demonstrate a need for further refinements and improvements within the software's functionality.
UniFab, initially known as DVDFab Enlarger AI, has gained popularity as a versatile AI-powered video enhancement tool since its rebranding in 2023. It's capable of upscaling video to 4K and offers features like HDR conversion, noise reduction, and deinterlacing, making it attractive to both enthusiasts and professionals. However, our research has uncovered several interesting issues related to its HDR conversion capabilities, particularly those related to HDR metadata.
The way HDR metadata is handled by various devices and software can lead to unexpected results during UniFab's HDR conversions. This complexity suggests that it can be a challenge to create a universal solution that works flawlessly across all hardware. UniFab's reliance on the bit depth of source material can cause trouble as well, with lower bit depths possibly not carrying enough information, causing visible banding or large variations in color during upscaling.
Furthermore, converting HDR video that's encoded in a different color space than the target output is a consistent cause of errors. This scenario, such as a conversion from BT.2020 to BT.709, can result in significant shifts in color and loss of detail.
There are also cases where the algorithm appears to struggle with high-motion scenes. During the HDR conversion process, these scenes sometimes result in a distracting issue of visual artifacts like smearing or ghosting.
Converting between HDR and SDR using dynamic range compression in UniFab sometimes results in counter-intuitive results. Scenes that should retain their contrast may appear flat, while others may be unnecessarily boosted, making it clear that fine-tuning the dynamic range during these conversions is critical.
User experiences have pointed towards misconfiguration issues stemming from the interface itself. Improvements could likely be made to the descriptions and layout of the settings, as the potential for flawed HDR conversions due to user error is a point of concern.
Interestingly, hardware plays a considerable role in UniFab's performance. When running on underpowered hardware like older graphics cards, the number of HDR conversions errors can increase, showing that a certain level of performance is necessary for it to work as intended.
Another unexpected problem is a loss of detail in shadow areas after conversion. This might suggest limitations in the algorithm's ability to interpret subtleties in dark areas, which can result in a muddier look and diminished depth perception.
The repeated use of UniFab with clips that already contain conversion errors can result in a type of cascade failure. The original artifacts can propagate to future conversions, highlighting how important it is to get the first HDR conversion right.
Users have also noticed inconsistencies in HDR processing times. The complexity and resolution of source material can lead to a wide variation in the time it takes to process each video. This likely indicates that the algorithm has not been uniformly optimized for all input video formats.
These findings highlight the need for further improvement and optimization of UniFab's HDR conversion tools. It seems that the algorithm has potential but can be prone to errors, particularly with challenging input material. The issues with bit depth, color space, temporal artifacts, and dynamic range compression suggest that a more robust and comprehensive approach might be needed to guarantee the desired quality for a wider range of videos. Further development and refinement of these features are crucial for ensuring that UniFab produces a reliable HDR conversion experience.
AI Video Upscaling Troubleshooting Common Issues in July 2024 - AI Upscaler Processing Time Optimization
AI video upscaling employs machine learning to boost resolution and refine video quality by reducing noise and enhancing details. While the technology shows great promise, the push for faster processing times without sacrificing output quality has emerged as a key concern. Users are becoming more aware that quicker processing can sometimes come at the cost of visual fidelity, especially with methods that rely on complex AI approaches like generative networks. The challenge lies in finding the right balance, but there are significant hurdles. These include inconsistencies in processing speed depending on the nature of the video being upscaled and limitations tied to the capabilities of the user's hardware. The quest for swift and high-quality AI upscaling is an ongoing endeavor, and advancements are needed to improve both the efficiency and accuracy of the results produced by these powerful tools.
Here's a rewrite of the text focusing on AI upscaler processing time optimization, keeping the original style and length:
AI video upscaling, while delivering impressive results in enhancing resolution and detail, isn't without its complexities, especially when it comes to processing time. It seems there are a few interconnected factors that play a role in how long an AI takes to upscale a video.
For example, the more advanced an upscaling algorithm is, the more it tends to focus on extracting fine details from the video. Generative models, which are quite sophisticated, can take a longer time to process because they dig deeper into each frame compared to simpler interpolation techniques that rely on basic calculations. While simple approaches are quick, they don't always offer the same level of visual improvement as the more complex methods.
Interestingly, it appears the processing time doesn't always increase directly in proportion to the desired resolution. For instance, increasing the resolution from HD to 4K can dramatically increase the processing time, far more than a simple doubling of pixels might suggest. This means that upscaling to higher resolutions can significantly strain the computational resources needed to carry out the operation.
On the plus side, many tools are built with batch processing capabilities, allowing users to handle multiple videos in a single go. This approach seems to optimize resource utilization, which can potentially reduce the overall time taken to upscaling numerous clips. This is especially noticeable when using hardware with multiple processor cores and graphics processors designed for AI tasks.
The kind of content in the video also plays a role in the processing time. Videos filled with fast-paced action or detailed textures tend to slow things down, as the upscaling algorithm needs to put in extra work to reconstruct the movement and intricate features accurately. This can sometimes cause a big difference in processing speed when comparing similar videos with varied content.
Hardware configurations are crucial, as different tools work better on different setups. For instance, the latest generation of NVIDIA RTX GPUs seem to offer a notable speed boost when compared to older hardware. This is likely due to specific hardware features optimized for AI tasks. These advancements in graphics hardware can noticeably improve the overall speed and efficiency of the upscaling process.
Some more advanced upscaling methods incorporate a dynamic resizing strategy, where the processing intensity is adjusted based on the specific section of the video being processed. In portions where less detailed features are present, the algorithm can work faster. This selective approach allows it to optimize the overall time by avoiding unnecessary computational load in simpler parts of the video.
It also seems that pre-processing steps like removing noise or adjusting colors can contribute to better processing efficiency. The upscaling algorithm might find it easier to analyze and reconstruct the details when a cleaner initial video is provided. This can lead to significant time savings by reducing the overall processing load.
A limitation we have observed is that insufficient RAM can create processing bottlenecks. When the computer doesn't have enough RAM, it can resort to storing data on slower storage devices, like the hard drive. This results in a significant decrease in processing speed. It's noteworthy that high-resolution video upscaling requires a substantial amount of RAM.
Even the initial frame rate of the video can have an effect on processing time. Higher frame rates might require more complex interpolation between frames during the upscaling process, slowing things down. This underlines how the video's initial technical characteristics can impact the speed of upscaling.
Another interesting development is the integration of continuous learning capabilities within newer upscaling algorithms. These models use the results of past upscaling tasks to fine-tune their settings dynamically. This adaptation might translate to faster processing speeds over time as the algorithm learns to optimize itself for different video types and specific content features.
All of these factors point to a complex interplay between algorithm sophistication, hardware capabilities, video content characteristics, and the unique features offered by different AI upscaling tools. Understanding these details can lead to significant improvements in performance when it comes to AI video upscaling. While we've seen impressive progress, it appears that there is still much to discover about optimizing the process to get the desired results without excessive wait times.
Upscale any video of any resolution to 4K with AI. (Get started for free)
More Posts from ai-videoupscale.com: