Upscale any video of any resolution to 4K with AI. (Get started for free)
Comparative Analysis Free AI Portrait Enhancers vs
Video Upscaling Techniques in 2024
Comparative Analysis Free AI Portrait Enhancers vs
Video Upscaling Techniques in 2024 - AI Portrait Enhancers Pixel Perfection Technologies
The field of AI portrait enhancement has seen remarkable advancements in 2024, delivering what could be called "pixel perfection technologies." These technologies, readily available through tools like Upscale Media and BeFunky, promise seamless improvements to portrait photos. Features like skin texture refinement, eye brightening, and expression enhancement are now commonplace, allowing users to achieve professional-looking results with minimal effort. The convenience of these tools, with their single-click solutions, is undeniable.
However, the ease of use sometimes comes with drawbacks. While offering various customizations like background adjustments and facial retouching, the potential for over-processing and a loss of image authenticity exists. The choices become complex as the variety of tools and their capabilities grow, demanding that users understand the trade-offs between effortless editing and retaining a natural-looking image. Although these AI tools provide quick and noticeable improvements, their effectiveness can depend heavily on the initial photo's quality and complexity. Understanding these limitations is crucial for achieving the desired results without compromising the image's integrity.
AI portrait enhancers, often leveraging Generative Adversarial Networks (GANs), are increasingly sophisticated in their ability to refine and enhance facial features. These networks learn from vast datasets of human faces, pushing the boundaries of realism. Interestingly, some advanced models are even starting to grasp the subtle nuances of facial expressions, aiming to enhance not just aesthetics, but also capture the underlying emotional intent.
This pursuit of perfection, however, necessitates meticulous data balancing. Training these models on massive image libraries, which can exhibit significant variation in skin tones, raises the need for addressing potential biases. The development process has seen major strides in accuracy and speed; AI portrait enhancers can now achieve remarkably high accuracy in facial recognition, with some surpassing 99%. This has implications beyond photo editing, opening doors in security and identification technologies. Processing times have also undergone a remarkable transformation, with real-time enhancement becoming feasible— a remarkable feat given the complexity of the tasks involved.
Moreover, recent advancements in deep learning empower these tools to address challenges like shadows or reflections that might obscure features in a photo, producing a clearer output without sacrificing the natural depth and context of the image. Beyond basic enhancements, some tools now integrate neural style transfer, which allows users to experiment with applying various artistic styles to their photos in real-time, offering a unique blending of technology and creative expression.
Despite these advances, it's noteworthy that different portrait enhancement algorithms vary widely in their capabilities. The number of dimensions processed is a key differentiator— some algorithms work with a limited range, around 75, whereas others utilize over a thousand dimensions for a richer, more nuanced output. Furthermore, AI portrait enhancers are trained on diverse facial datasets, meaning they can tailor their enhancements based on cultural and ethnic characteristics. While this demonstrates the potential for personalization, it also raises essential questions about representation and the ethical implications of such technologies.
Many of these tools also utilize facial landmark detection to selectively apply enhancements. This technique permits localized adjustments to specific features, like the eyes, nose, or mouth, striving for a natural look while optimizing the overall quality of the portrait.
Comparative Analysis Free AI Portrait Enhancers vs
Video Upscaling Techniques in 2024 - Comparative Analysis Methodology Benchmarking Criteria
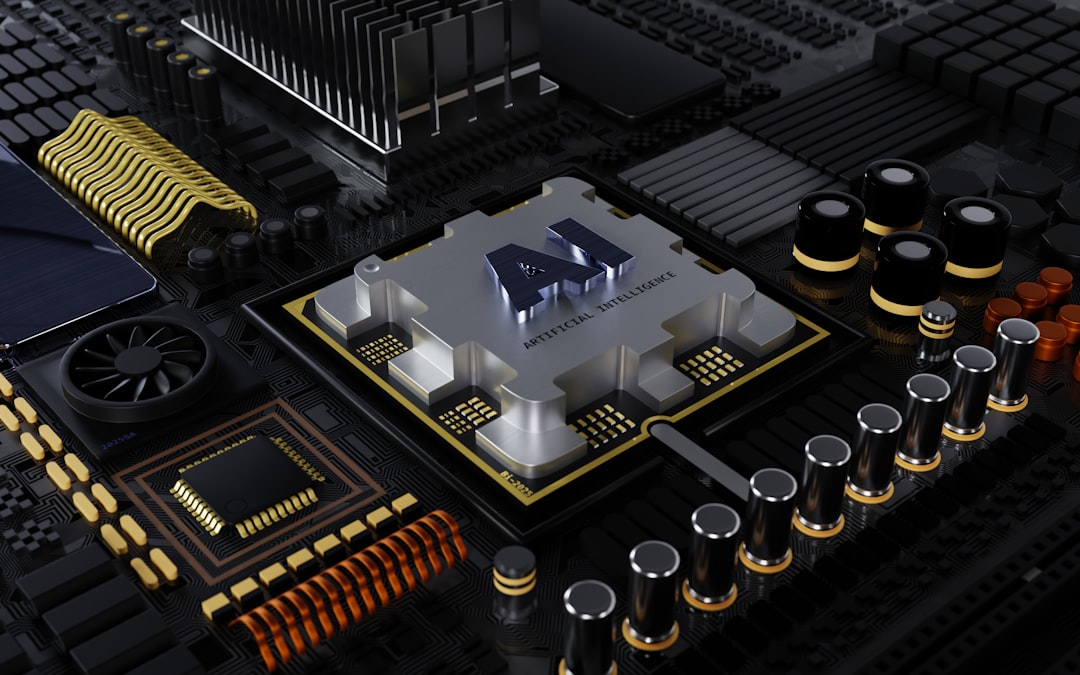
When comparing AI portrait enhancers and video upscaling techniques, a structured approach is crucial. Comparative analysis methodology involves a systematic process of evaluating and contrasting these technologies, focusing on identifying similarities and differences in their performance and outcomes. To effectively understand and analyze these technologies, a set of specific benchmarking criteria is defined. This includes identifying key performance indicators (KPIs) that measure crucial aspects, such as image quality, processing speed, and the ability to maintain natural-looking results. The chosen KPIs are then used to objectively assess the strengths and weaknesses of each tool, offering a clearer understanding of their capabilities and limitations.
Additionally, the process of comparative analysis emphasizes the importance of selecting suitable tools and techniques to visualize the collected data. Utilizing methods like charts and graphs helps to organize the findings, making complex data more readily accessible and comprehensible. Visualizations can highlight areas where one technology excels over another or reveals limitations that might need further development.
Given the rapid evolution of AI in 2024, these comparative analysis techniques are becoming increasingly valuable. They help differentiate between the various AI-powered tools available and assess how well each fulfills the needs and expectations of users. The insights gleaned from a robust methodology help guide development decisions and ensure that future technologies prioritize the optimization of user experience and address emerging challenges within the field.
1. **The Challenge of Benchmarking Criteria Selection**: When comparing different AI-powered image and video enhancement tools, selecting the right criteria for benchmarking can be tricky. The specific metrics chosen can significantly influence the outcome of the comparison, potentially leading to biased or misleading results if they don't align well with the research goals.
2. **Addressing Algorithmic Bias in Benchmarks**: A key concern with AI tools is the potential for hidden biases in their algorithms. It's difficult for standard benchmarks to always effectively identify these biases, highlighting the need for more nuanced frameworks that evaluate not just technical performance but also fairness and inclusivity.
3. **The Importance of Dimensionality in Video Upscaling**: When analyzing video upscaling methods, the number of dimensions considered during processing plays a crucial role. Using a larger number of dimensions can capture richer details, but it also demands greater computational resources, making the comparison between performance and efficiency a complex matter.
4. **Temporal Consistency in Video Analysis**: Evaluating video upscaling techniques requires a different approach than simply analyzing still images. We need to consider how well a method preserves visual coherence across a sequence of frames. Ensuring consistent quality over time can be a deciding factor in determining the effectiveness of a particular technique.
5. **Resolution Fidelity: A Neglected Factor**: Surprisingly, many comparisons of AI image and video enhancement tools don't pay enough attention to how well they preserve or improve resolution. It's easy to focus on subjective visual improvements, but quantifying actual resolution gains is critical for understanding how practical these tools are.
6. **The Danger of Error Propagation**: In video upscaling, a minor error in one frame can cascade through subsequent frames, creating a series of distortions that degrade the overall quality. This 'error propagation' issue emphasizes the need for benchmark criteria that focus on robustness and error handling.
7. **Domain-Specific Benchmarks**: The metrics that are useful for evaluating AI portrait enhancement may not always translate directly to video upscaling. These two areas have different workflows and intended uses, highlighting the necessity of having specific benchmarks that are tailored to each domain.
8. **User Experience in Comparative Analysis**: While quantitative data is important, we shouldn't neglect the subjective user experience. Feedback on how easy and intuitive a tool is to use can provide valuable insights into real-world applicability, but these subjective aspects are often overlooked in formal benchmarking methods.
9. **The Speed-Quality Trade-Off**: It's crucial to acknowledge that AI enhancement tools often involve a trade-off between speed and image quality. Some benchmarks might prioritize speed over detail, which might not accurately reflect how these tools perform in real-world situations where users have specific quality expectations.
10. **Integrating Ethics into Benchmarks**: Although not always included, incorporating ethical considerations into benchmark criteria is becoming increasingly vital. As these AI-powered technologies become more prevalent, it's important to guide their development through benchmarks that consider potential social impacts and promote responsible design and deployment.
Comparative Analysis Free AI Portrait Enhancers vs
Video Upscaling Techniques in 2024 - Free AI Tools Market Landscape Open-Source Alternatives
The realm of free AI tools in October 2024 presents a diverse landscape, with both proprietary and open-source options emerging. This variety provides choices for those seeking assistance with tasks such as market research, data analysis, or content generation. Open-source offerings like TensorFlow Lite Microcontrollers enable users to deploy AI models on a wide range of devices, even those with limited resources, and thereby promote more localized data analysis. Tools like Jamovi simplify statistical analysis, making advanced techniques more accessible to a wider user base. Similarly, platforms like H2O.ai strive to democratize access to AI development through easier model construction.
However, while these open options provide a valuable counterpoint, it's notable that some prominent AI tools remain proprietary. Advanced language models, for example, like Falcon 180B, and popular interfaces like ChatGPT often come with limitations and potential concerns about accessibility and equitable access to the power of AI. Furthermore, the training data used in these models often raise concerns about inherent biases and the potential for uneven representation.
The growing awareness of these potential biases underscores the importance of ethical considerations in the development and application of AI tools. As the market evolves, it becomes critical for users and developers alike to be mindful of these potential drawbacks and seek solutions that prioritize transparency and fairness for all. Ultimately, users must carefully assess the strengths and limitations of each tool in order to make informed choices as AI technology continues its rapid advancement and plays an increasingly important role across various fields.
The world of free AI tools in 2024 is a dynamic landscape shaped by both proprietary and open-source solutions. The increased availability of free alternatives, like ChatGPT and TensorFlow Lite Microcontrollers, is creating a competitive environment that's pushing innovation. Tools previously found only in premium software, such as real-time processing and complex feature extraction, are now more widely available. This competitiveness is fueled by the open-source community, where developers worldwide contribute, leading to faster updates and a broader range of perspectives. This collaborative approach is also making people more mindful about how data is used in AI, with developers aiming for transparency about training datasets and potential biases.
Interestingly, certain niche applications can see some open-source tools, like Falcon 180B, outperform their commercial counterparts. They are sometimes optimized for specialized uses or resolutions that mainstream platforms haven't prioritized yet. Furthermore, many free AI tools have been designed to be efficient, running smoothly on average hardware. This is a significant advantage for users with limited computing resources. We are also seeing more integration of these tools with existing software ecosystems, such as photo and video editors. This expands their capabilities and creates new possibilities for users to be more productive in creative tasks.
The ability to customize these tools is often higher compared to many closed-source alternatives. Open-source tools allow users to adjust algorithms or parameters, which can be particularly helpful when a more specific outcome is desired. The open-source model has also helped fast-prototyping tools become more readily available. This allows for rapid experimentation and development cycles, making the tools even more versatile. While beneficial, the open-source nature of these tools introduces security considerations since the code is publicly accessible. This has resulted in a growing focus on secure coding practices and consistent updates to reduce potential vulnerabilities.
As the free AI tools landscape continues to grow, we are seeing applications in diverse fields, like gaming and education. The evolution of these tools expands beyond basic tasks like enhancing portrait photos and video, suggesting a potential for unforeseen applications. This adaptability hints at a promising future, where free and collaborative solutions contribute to technological advancements across numerous disciplines. There's still a need for ongoing dialogue around ethical concerns and responsible AI development. However, the free AI tools market presents a strong case for democratizing access to advanced technologies that can empower a wider range of individuals and researchers.
Comparative Analysis Free AI Portrait Enhancers vs
Video Upscaling Techniques in 2024 - Hardware Requirements GPU vs Cloud Processing Debate
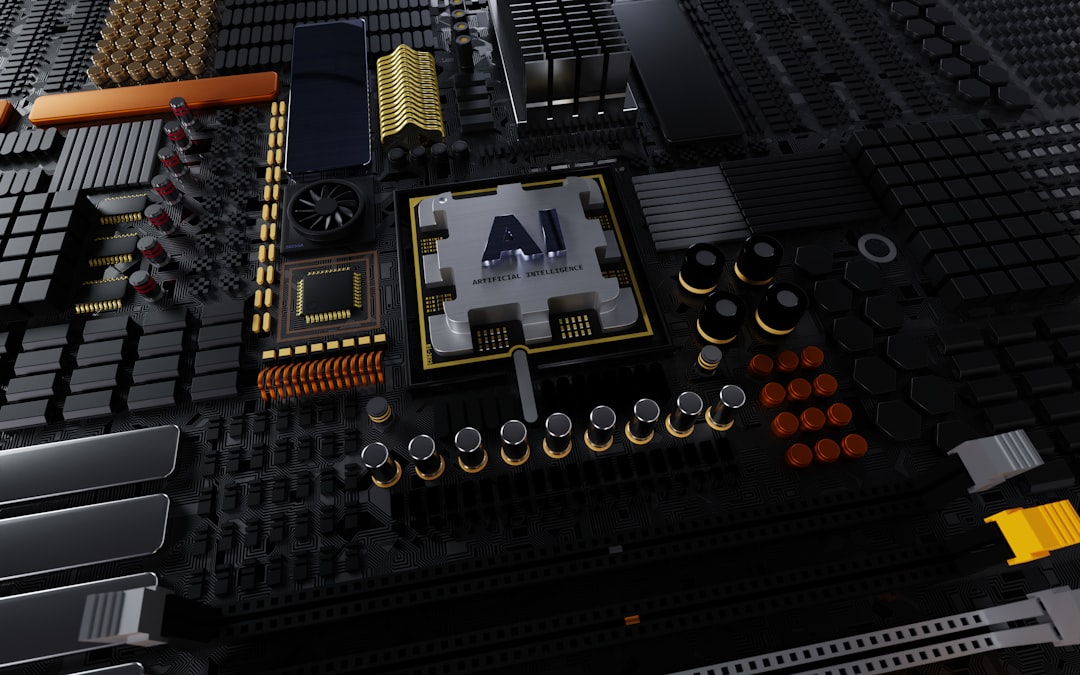
The choice between using a dedicated GPU or relying on cloud processing for AI tasks is a persistent topic of discussion, particularly in the context of computationally intensive applications. GPUs, with their specialized architecture and parallel processing strengths, remain a popular option for handling many AI workloads. This is especially true for demanding tasks that benefit from their raw processing power. However, cloud-based solutions are gaining traction, driven by their flexibility and the ability to scale resources up or down as needed. This makes them a more attractive option for AI initiatives with fluctuating demands or limited local hardware capacity.
Further adding to the complexity of this decision, specialized processing units like NPUs and TPUs are becoming increasingly relevant. These hardware designs are tailored for AI acceleration and offer substantial performance and energy efficiency improvements compared to more general-purpose CPUs and GPUs. This raises the stakes in the hardware decision, forcing developers to consider a wider range of options and assess their individual needs more thoroughly.
In 2024, the decision boils down to balancing multiple considerations: raw processing power, computational efficiency, budgetary limitations, and the need for readily scalable solutions. Whether choosing a powerful GPU or tapping into cloud processing resources, developers must navigate a complex interplay of these factors to optimize performance and achieve their AI project goals.
When considering AI workloads, the choice between using a dedicated GPU and relying on cloud processing services presents a complex set of trade-offs. One key aspect is the inherent delay or latency often seen with cloud-based options. While they offer powerful computing, the need to transmit data over networks introduces delays that can be critical for real-time applications, unlike local GPU processing.
Local GPUs typically operate at a more efficient level, achieving utilization rates of around 60-90% depending on the task. However, cloud services can operate at lower utilization due to shared resource environments, potentially making them less cost-effective as users might not fully use the computational power they're paying for.
Cloud processing excels at scaling up to handle massive workloads. Nevertheless, expanding capacity frequently leads to cost increases and can cause delays in resource allocation. Locally, GPUs allow for more immediate adjustments to processing capabilities, particularly for anticipated workloads.
Energy consumption is another aspect to consider. On-premise GPUs tend to have a more predictable power usage based on their specifications. Cloud datacenters have fluctuating power consumption driven by workload and efficiency optimizations, introducing potential unpredictability in operational expenses.
From a financial perspective, the cloud usually has lower initial costs. However, over time, these expenses can add up quickly, especially for consistently intensive processing tasks. In contrast, buying a local GPU might be a more significant upfront investment, but it can result in lower long-term costs with continuous usage.
Security and privacy become concerns when relying on the cloud, as data is stored and processed offsite, and users may lose direct control. Using a local GPU configuration provides more direct oversight of sensitive information.
Cloud platforms often have standardized setups and limits on the software environment, while local GPUs provide much more flexibility for software configurations and customizations. These customized environments can be crucial for certain specialized applications that necessitate specific optimization techniques.
Local GPUs are typically optimized for a focused set of tasks related to graphics and parallel computing. Cloud providers offer diverse specialized instances tailored for a wider range of tasks including machine learning and database operations, which can broaden the appeal depending on the specific application.
Although cloud services heavily invest in reliable operation, unexpected disruptions can still occur due to maintenance or outages. Local GPUs within a personal or dedicated system can offer increased stability for users who want to manage their own computing without relying on external factors.
A developing trend is the use of hybrid solutions which combine local GPUs and cloud processing to get the best of both. This method strives to strike a balance between the responsiveness and control of local hardware with the flexibility and scale of the cloud, paving the way for innovative computing strategies.
Comparative Analysis Free AI Portrait Enhancers vs
Video Upscaling Techniques in 2024 - Future Trends Quantum Computing Integration Possibilities
The prospect of integrating quantum computing into various fields, particularly in conjunction with artificial intelligence, is gaining significant momentum in 2024. A key development is the transition towards more stable and dependable quantum computations through error-corrected logical qubits. This represents a crucial step forward in the reliability of quantum computing. The fusion of quantum computing and AI, referred to as Quantum AI (QAI), opens up new avenues for complex data analysis and decision-making. These combined capabilities are poised to tackle problems that traditional computers find exceptionally challenging.
The potential of quantum computing has attracted considerable financial backing, with substantial investments from both venture capitalists and governments globally. This strong interest suggests a shared belief in the transformative power of quantum computing for future technologies and industries. As quantum computers become more sophisticated, the interplay with AI is likely to lead to new applications and unexplored fields of study. Additionally, with the increasing reliance on quantum systems, the need for understanding and managing the associated cybersecurity risks is becoming increasingly urgent. Developing robust strategies for risk assessment and vulnerability scanning within the context of quantum computing is critical for both IT procurement and overall security practices. These developments signal the emergence of a new phase in computational power and its implications for various sectors.
The emergence of error-corrected logical qubits in 2024 represents a pivotal step forward in quantum computing, hinting at more stable and reliable computations. This progress, coupled with the field of Quantum AI (QAI), suggests a future where quantum computing's capabilities are harnessed alongside artificial intelligence to unlock advanced data analysis and decision-making beyond the limitations of traditional computing. While overall tech investments experienced a downturn, quantum computing still garnered substantial funding in 2023, with $12 billion in venture capital, underscoring investor optimism in its long-term potential. This optimism is echoed by governments globally, as they're investing heavily in quantum computing initiatives, recognizing its significance in future technological advancements.
The integration of quantum and AI is anticipated to reshape society and the economy, possibly fueling the next phase of innovative technologies. Quantum computing's ability to perform many calculations concurrently, in parallel, offers exciting possibilities when combined with AI, potentially boosting the efficiency and capabilities of data processing tasks. As quantum computers evolve, the continuous improvement of AI could unlock new avenues for utilizing quantum systems, perhaps leading to novel applications and industries. Quantum memory, leveraging superposition to store extensive datasets, provides potential for handling more intricate information management.
Emerging practices for quantum cyber vulnerability scanning and risk assessment are gaining importance for IT departments and cybersecurity strategies. This is especially important given how quantum computing could impact existing encryption methods. The merging of quantum computing and AI is widely viewed as transformative for Industry 4.0, presenting significant challenges for effectively managing change and successfully integrating these new technologies into existing operations. Developing methods for smoothly introducing quantum technologies into established workflows will be crucial.
The field is still in its infancy, facing numerous technical challenges. Quantum systems are very sensitive to environmental conditions, such as temperature and electromagnetic fields. Maintaining the fragile quantum states required for computation is a very complex problem, and the development of better quantum error correction is a crucial area of research. However, quantum computing, despite these challenges, shows remarkable potential for a broad range of problems in a variety of fields. We are entering an era where its implications will likely be profound.
Upscale any video of any resolution to 4K with AI. (Get started for free)
More Posts from ai-videoupscale.com: