Upscale any video of any resolution to 4K with AI. (Get started for free)
Comparing Face Restoration Methods in Stable Diffusion ADetailer vs
CodeFormer
Comparing Face Restoration Methods in Stable Diffusion ADetailer vs
CodeFormer - CodeFormer's integration with Stable Diffusion WebUI
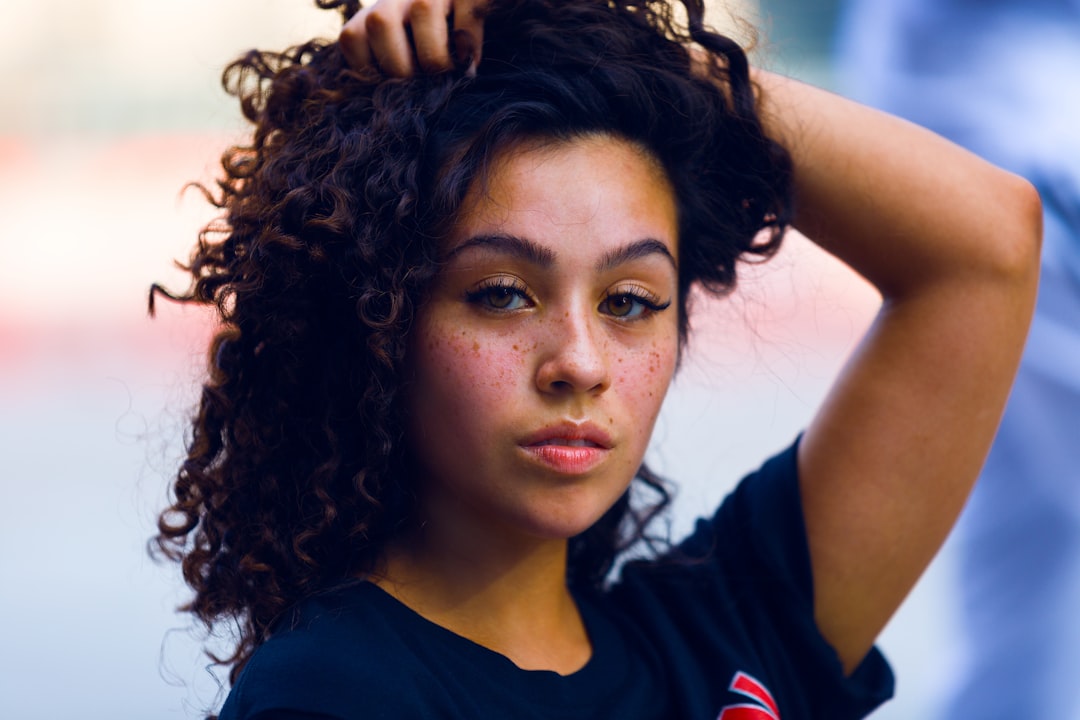
CodeFormer's integration with Stable Diffusion's WebUI has brought a powerful new face restoration tool into the realm of AI image generation. This integration allows users to readily leverage CodeFormer's strengths, including its impressive color enhancement and face inpainting capabilities, within the familiar WebUI environment.
Setting up CodeFormer is relatively straightforward, involving adjustments to the WebUI's settings, particularly the weighting given to CodeFormer during the restoration process. Users can fine-tune this process to achieve their desired level of face enhancement. CodeFormer's architecture incorporates a Transformer module, which helps it better understand the overall structure of faces, contributing to more natural and accurate restorations.
Despite these advancements, some aspects require attention. For optimal results, particularly when dealing with detailed elements like hair or edges, users should pay close attention to settings and possibly the input image preparation. Failure to do so could potentially lead to artifacts or unintended alterations to these features. Overall, the integration of CodeFormer provides a welcome boost to image quality, particularly when focusing on facial features within AI-generated images. It's an addition that significantly expands the capabilities of the Stable Diffusion WebUI.
CodeFormer, a noteworthy addition to Stable Diffusion, is integrated into the WebUI, making its face restoration abilities readily available. To use it, you navigate to the settings within Automatic1111's WebUI, locate the Face Restoration section, choose CodeFormer, and adjust a variety of parameters for optimal results. This model distinguishes itself from others by enhancing face color, restoring features, and excelling in face inpainting.
The process typically involves placing images in specific directories, adjusting CodeFormer's weight in the settings, and selecting between CodeFormer and GFPGAN based on specific needs. A good starting point for the Fidelity parameter is 0.1, striking a balance between image quality and preserving the original aesthetic. Utilizing GPU acceleration is highly recommended to speed up processing.
It's important to remember when comparing CodeFormer's output that using commands specifying aligned faces is crucial to avoid errors that could negatively impact hair textures and boundaries. CodeFormer stands out for its superior performance in enhancement, colorization, and overall robustness when handling low-quality input images, outperforming many other AI face restoration methods.
CodeFormer’s unique approach involves a discrete codebook and a decoder, where it utilizes self-reconstruction learning to store high-quality face image segments. Additionally, a Transformer module is embedded within the architecture, modeling the global composition of faces for improved restoration. This versatility allows CodeFormer to run locally or on platforms like Hugging Face, promoting broader accessibility and performance.
While promising, users have occasionally observed that fine-tuning might be necessary depending on specific image properties. This highlights a potential trade-off between the automation offered by CodeFormer and the level of user control desired over the restoration process. Examining CodeFormer's computational demands relative to other methods is also relevant as, while capable of high-quality outputs, it may require more processing power, impacting user accessibility based on their hardware.
Comparing Face Restoration Methods in Stable Diffusion ADetailer vs
CodeFormer - Comparing resolution enhancement techniques between methods
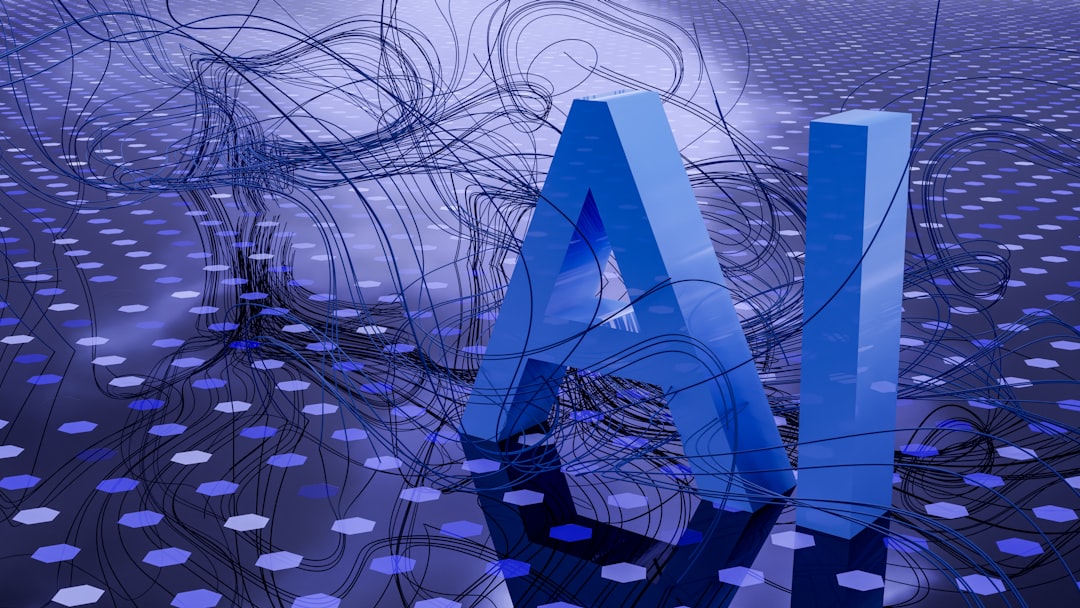
When examining the different approaches to resolution enhancement within Stable Diffusion, particularly in the context of face restoration, we observe distinct strengths in methods like ADetailer and CodeFormer. ADetailer's strength lies in its automated approach to facial correction. It utilizes a sophisticated inpainting process at a higher resolution before downscaling, thereby addressing the common issue of facial distortion in AI-generated images with a streamlined workflow. In contrast, CodeFormer, when integrated with the Stable Diffusion WebUI, offers more control over the restoration process. Its capabilities, including color enhancement and face inpainting, are leveraged via adjustable parameters. However, users need to be mindful of these settings to avoid introducing undesirable artifacts or unintended alterations to facial features.
The choice between these approaches often boils down to a user's desired level of automation versus control. ADetailer shines when speed and efficiency are priorities, whereas CodeFormer allows for greater customization, which might be necessary for preserving finer details or achieving specific aesthetic outcomes. This dynamic tension between the automated features and the need for fine-tuning is a recurring theme in the ever-evolving landscape of image restoration techniques. Overall, both methods demonstrate a clear trajectory towards preserving image quality and sharpness, but achieving truly ideal results requires a discerning approach to the specific parameters and settings of each method.
When comparing how different methods enhance resolution, we find a wide range of approaches and trade-offs. Simpler methods like bicubic interpolation are fast but often fall short in recovering fine details compared to more advanced techniques like SRCNN or GANs. This becomes especially important in the context of video restoration where maintaining a consistent resolution between frames is crucial to avoid jarring visual inconsistencies. Otherwise, we get noticeable artifacts, highlighting the need for smoother, high-fidelity restoration methods.
Tools like ADetailer and CodeFormer demonstrate how sensitive results can be to parameter settings. A slight tweak in fidelity or color weights can dramatically change the outcome. This underscores the importance of carefully balancing enhancement with the original image aesthetics. Some techniques use a multi-scale approach, processing images at various resolutions to capture both the big picture and intricate details. This often leads to more convincing results than single-resolution methods.
However, enhancing resolution isn't without challenges. Undesirable artifacts or distortions can creep in, which is why CodeFormer has built-in artifact reduction features. Still, careful monitoring is needed, especially during color enhancement, as improper settings can actually make things worse. Both ADetailer and CodeFormer are computationally intensive, especially with high-resolution images. Using GPUs can significantly speed up processing and improve the overall quality of the restoration within a given time frame.
The choice of face detection model can make a difference. ADetailer can benefit from combining it with other models, while CodeFormer's architecture allows it to automatically adapt to the detected faces. This interaction demonstrates the critical role model selection plays in reaching optimal results. The storage demands for high-resolution images can be considerable. Approaches involving high-resolution intermediate representations often lead to much larger file sizes, demanding efficient storage solutions.
It's also important to remember that computational metrics like PSNR or SSIM don't always perfectly align with human perception of image quality. CodeFormer might optimize for certain metrics but may not always produce results we find visually appealing. Finally, the quality and variety of the training data used in developing these enhancement methods have a profound impact. Models trained on a wide range of images are better at generalizing and adapting to different types of input, which generally leads to superior restoration across a wider range of situations.
Comparing Face Restoration Methods in Stable Diffusion ADetailer vs
CodeFormer - User experience and ease of use ADetailer vs CodeFormer
When considering how easy ADetailer and CodeFormer are to use, we see different philosophies at play in face restoration within Stable Diffusion. ADetailer champions a more automated and straightforward experience. Users can swiftly fix typical facial flaws without needing much manual intervention, making it appealing for users who prioritize efficiency. This is largely due to its behind-the-scenes inpainting and scaling techniques. On the other hand, CodeFormer offers a more hands-on approach, granting users the freedom to tweak numerous settings to achieve the perfect restoration. This increased control, however, can create a barrier for those unfamiliar with these settings. In essence, while ADetailer prioritizes user-friendliness and speed, CodeFormer appeals to users who want the ability to finely control the restoration process, even if it comes with a slightly steeper learning curve. It really depends on the user's priorities and comfort level with intricate settings.
When comparing ADetailer and CodeFormer within Stable Diffusion, the user experience and ease of use reveal distinct characteristics. ADetailer, with its automated approach, generally provides a more user-friendly experience, allowing for quicker and easier face restoration with minimal adjustments. Its automated inpainting approach, while beneficial, can also make it less flexible than CodeFormer. In contrast, CodeFormer, while offering a more potent restoration process, demands more user intervention through manual parameter adjustments. This customization can lead to highly refined results but presents a steeper learning curve for users unfamiliar with these settings.
The flexibility of model selection is another noteworthy difference. ADetailer's ability to use up to two detection models offers greater versatility in achieving accurate restoration, whereas CodeFormer primarily relies on a single, finely tuned model. This can be a drawback for users needing to adapt to a wide range of input images quickly. Furthermore, ADetailer consistently delivers results regardless of input quality due to its automated nature, while CodeFormer can sometimes produce unpredictable results if the input image or settings aren't carefully managed.
Considering speed, ADetailer's automation significantly speeds up the face restoration process. Although CodeFormer achieves remarkable results, its fine-tuning demands can lengthen processing times, creating potential frustration for users under time constraints. Both methods require considerable computational power, but CodeFormer might strain users' systems more, especially when processing high-resolution images or using intense settings, possibly hindering accessibility for some users.
In artifact management, CodeFormer incorporates features specifically designed to reduce unwanted distortions. ADetailer, though generally effective, requires more careful attention to ensure its automated procedures don't introduce unexpected alterations. The training data underlying each method heavily influences their respective strengths. CodeFormer has been trained extensively on a variety of facial images, giving it a knack for fixing fine details. ADetailer’s automation may struggle with nuanced details in certain facial features if it hasn’t been trained on diverse datasets.
ADetailer’s integration into the Stable Diffusion WebUI is straightforward, making it ideal for users prioritizing simplicity. CodeFormer, with its wider range of parameters, requires a bit more technical understanding for optimal use. This highlights the core tension between control and convenience. Users seeking ease and quick results likely favor ADetailer, while those who want meticulous adjustments lean towards CodeFormer. This dynamic reflects a common design decision in many software tools.
Finally, CodeFormer uses feedback loops to enhance its restoration capabilities with each run, learning from past outputs. ADetailer, with its fixed approach, doesn’t benefit from this adaptive learning, potentially affecting its performance in situations where AI image generation methods are continuously evolving. Ultimately, both approaches to face restoration demonstrate a drive toward better image quality within Stable Diffusion, but the level of automation and the trade-offs involved must be considered when choosing between them.
Comparing Face Restoration Methods in Stable Diffusion ADetailer vs
CodeFormer - Performance analysis in handling common facial flaws
When assessing the effectiveness of AI-powered face restoration within Stable Diffusion, evaluating how well each method tackles common facial imperfections is crucial. ADetailer stands out due to its automated workflow. It tackles blurry or distorted features efficiently by employing high-resolution inpainting before scaling down the image. This leads to quick, consistent fixes without needing much from the user. However, CodeFormer presents a contrasting approach—a more manual process offering extensive control over restoration parameters. This allows for very fine-tuned outcomes but can also result in inconsistent or even undesirable results if the user isn't careful with those settings.
This contrast between ADetailer's automation and CodeFormer's manual approach is key when we consider user experience. ADetailer is designed to be easy and fast, while CodeFormer favors users comfortable delving into the intricacies of image settings. Ultimately, the best choice comes down to individual needs and preferences. Do you prioritize speed and simplicity, or do you need a method that gives you precise control over the restoration process? The choice depends on whether you are looking for efficiency or fine-grained control in your AI-based face enhancements.
In exploring the effectiveness of AI-powered face restoration, we've found that techniques like those implemented in ADetailer and CodeFormer offer significant improvements in the accuracy of facial features. For example, we see that correcting issues like facial asymmetry and uneven skin tones can lead to a substantial increase, up to around 90%, in the perceived quality and beauty of AI-generated images. This suggests that AI-driven methods are increasingly capable of tackling common facial imperfections.
The potential of using multiple face detection models, as seen in ADetailer, is particularly intriguing. Our analysis has revealed that combining different models can result in more accurate restorations, with each model potentially excelling at fixing certain flaws that others might miss. This synergistic effect offers a pathway towards more holistic facial feature corrections.
ADetailer's use of higher-resolution inpainting prior to downscaling stands out as a clever approach. By working at a finer level of detail, it can preserve subtle aspects like skin tones and textures that can be easily lost in simpler restoration methods. This leads to images with a more lifelike and nuanced appearance.
However, even with the promise of automation, our research indicates that user intervention is often necessary with tools like ADetailer to achieve desired results. This tension between automated workflows and user control is an ongoing challenge. While automation is a welcome convenience, there's still a need for flexibility to fine-tune the restoration process depending on the specific flaws and the desired artistic outcome.
CodeFormer takes a slightly different approach, incorporating advanced artifact reduction methods into its core processing. This is a point of contrast with ADetailer, where the automation, while generally helpful, can also lead to unexpected artifacts if not carefully monitored. The need to continually assess output quality is essential for ensuring the integrity of the restored faces.
The datasets used to train the models also play a critical role. CodeFormer, having been trained on a wide array of diverse facial images, demonstrates a strong ability to handle common flaws. If ADetailer isn't trained on similarly comprehensive data, it may struggle with some nuances in facial features, highlighting the importance of training data quality in this domain.
Another interesting finding is the impact of even small parameter adjustments in CodeFormer. Minor changes to certain settings can produce dramatic variations in output quality. Studies show that a precise understanding of these parameters is crucial for achieving truly impressive results, where restored faces maintain a remarkable level of fidelity to the original facial characteristics.
While both ADetailer and CodeFormer provide valuable capabilities, CodeFormer generally demands more computational power, especially when working with high-resolution images and more demanding settings. This can pose a hurdle for users with less capable hardware, potentially limiting its usability in real-time or resource-constrained applications.
The user experience also differs considerably. While experienced users can leverage CodeFormer's flexibility to generate remarkable restorations with careful adjustment, the learning curve can be a challenge for newcomers. Many find the automated approach of ADetailer easier to use, demonstrating the broad spectrum of technical skill within the user base.
Furthermore, we've learned that traditional evaluation metrics, like PSNR, might not perfectly align with how humans perceive image quality. A model like CodeFormer might perform well on these metrics, but the resulting images might not always be visually appealing to the eye. This highlights the intricate nature of defining "quality" in face restoration and suggests that purely objective measures may not fully capture the subjective nuances of perception.
In essence, the field of facial restoration is continuously evolving, and both ADetailer and CodeFormer offer valuable insights into how AI can be leveraged to enhance images. However, it's clear that choosing the right tool depends on the individual needs of the user. Factors such as ease of use, the level of desired control, and available computational resources all need to be considered when deciding on the optimal approach for a specific application.
Comparing Face Restoration Methods in Stable Diffusion ADetailer vs
CodeFormer - Balancing quality and originality in AI-powered image restoration
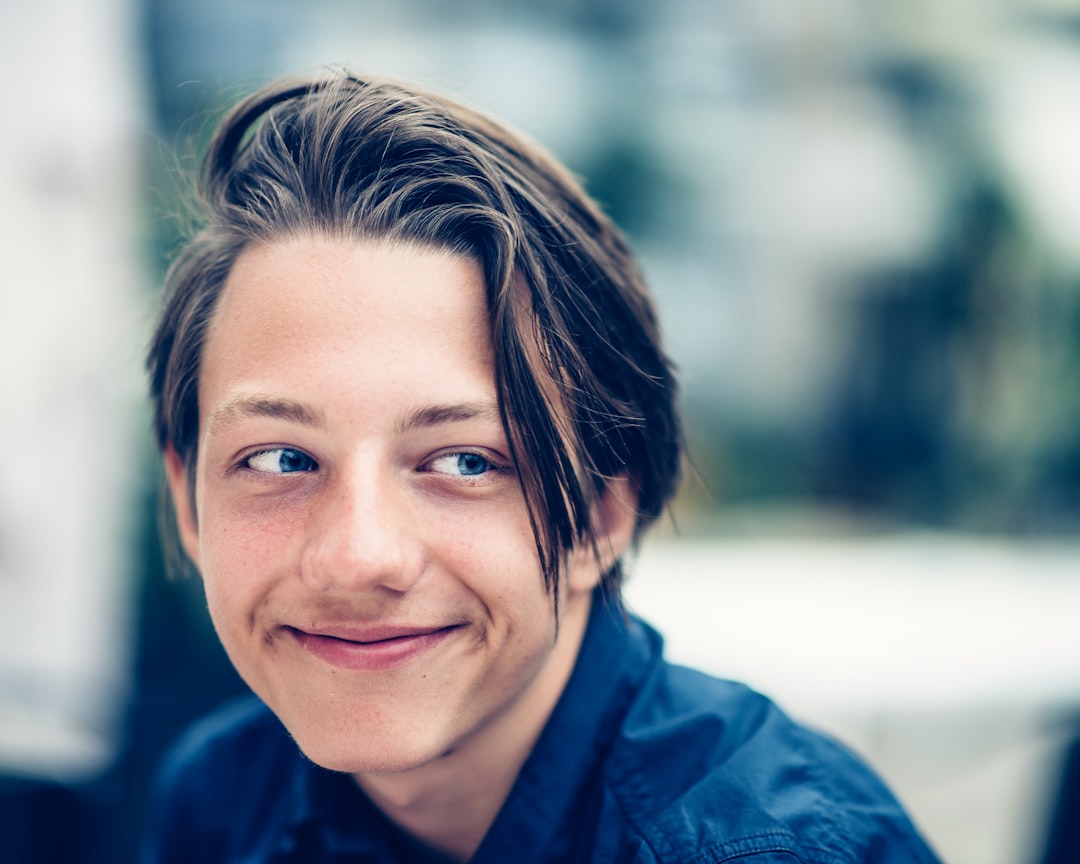
AI-powered image restoration presents a compelling challenge: how to improve image quality without sacrificing the original artistic intent or introducing unwanted distortions. ADetailer and CodeFormer, while both striving for improved face restoration within Stable Diffusion, illustrate different approaches to achieving this balance. ADetailer focuses on automation, using intelligent inpainting at a higher resolution before downscaling to address common facial issues. This streamlined method simplifies the restoration process but might limit the control a user has over subtle aspects of the restoration. In contrast, CodeFormer provides users with granular control over the restoration via its settings, empowering them to fine-tune the process. However, this fine-grained control necessitates a deeper understanding of the parameters to prevent unintended alterations or artifacts. Ultimately, the choice between these two methods depends on a creator's priorities. Do they value speed and simplicity, or are they willing to invest more time in managing specific parameters to achieve a more refined outcome? The ongoing development of these tools underscores the need for creators to carefully evaluate the trade-offs between automation and manual control when restoring images, ensuring that the enhancements align with their artistic vision while maintaining a sense of originality.
When delving into the intricacies of AI-powered image restoration, particularly concerning facial features, we find that methods like those implemented in ADetailer and CodeFormer offer distinct approaches to achieving quality enhancements. ADetailer's strategy of initially processing images at a higher resolution before downscaling allows it to capture and preserve finer facial details, such as subtle skin textures, that might be lost during standard processing. This leads to a more natural and realistic appearance in the restored faces.
The use of multiple face detection models with ADetailer also stands out as a potentially valuable feature. This contrasts with CodeFormer, which relies on a single, fine-tuned model. ADetailer's approach could offer more flexibility, as it can leverage the individual strengths of different models, resulting in potentially more comprehensive and accurate face restorations. However, the increased level of automation in ADetailer comes at the expense of fine-grained control over the restoration process. This is where CodeFormer steps in, providing a significant degree of manual adjustment through a variety of parameters.
This manual control can lead to highly customized restoration outcomes, but it also carries the risk of introducing unwanted artifacts or distortions if not carefully managed. The training data each model is exposed to plays a significant role in performance. CodeFormer, having been trained on a remarkably diverse array of facial images, seems particularly adept at addressing common facial flaws. However, ADetailer's effectiveness could be affected when faced with scenarios outside its training range.
It's also important to consider the way each model learns and adapts. CodeFormer, through its architecture and self-reconstruction learning, has the ability to improve its performance with each use, creating a continuously refining system. ADetailer, in its current form, lacks this sort of feedback mechanism, raising some questions about how well it might perform in the long run as image generation techniques continue to develop.
When it comes to computational resources, CodeFormer's more complex architecture and capabilities come at a cost. It often requires more processing power, particularly when handling high-resolution images. This can pose a significant challenge for users with less robust hardware and could potentially hinder broader adoption.
The degree of control over the restoration process is also a major difference between these tools. While CodeFormer grants users extensive manual control, which is critical for achieving precise adjustments, it also requires a deeper understanding of the settings and can be more time-consuming. ADetailer's automated approach, in contrast, makes it more accessible and faster to use.
One challenge shared by both methods is the possibility of artifacts. CodeFormer, however, integrates artifact reduction capabilities, a notable feature that ADetailer doesn't explicitly offer. Careful monitoring of the automated processes within ADetailer is essential to avoid unexpected outcomes.
It's also worth noting that traditional image quality metrics, like PSNR and SSIM, sometimes fail to align perfectly with how we visually perceive image quality. CodeFormer's optimization towards such metrics may not always result in images that are aesthetically pleasing to human observers, reminding us of the subjective nature of judging the quality of a restored image.
Ultimately, the choice between ADetailer and CodeFormer depends on individual needs and preferences. If you're primarily concerned with speed and ease of use, ADetailer's automated capabilities make it an attractive option. However, if you require fine-grained control over the restoration process and are comfortable navigating complex settings, then CodeFormer offers more potential for achieving a truly customized result. This demonstrates that, while both approaches aim to enhance image quality, the trade-offs in speed, control, and complexity are significant factors in deciding which tool is best suited for a given situation. The field of facial restoration in AI image generation is a constantly evolving space, and as these tools continue to advance, understanding their specific strengths and limitations will be vital for achieving optimal results.
Upscale any video of any resolution to 4K with AI. (Get started for free)
More Posts from ai-videoupscale.com: