Extracting High-Quality Images from Video A 2024 Guide to Frame-by-Frame Conversion
Extracting High-Quality Images from Video A 2024 Guide to Frame-by-Frame Conversion - Leveraging AI-Powered Frame Analysis for Optimal Image Selection
The ability to extract the most impactful images from video is being transformed by AI-powered frame analysis. Tools that automate frame selection eliminate the tedious task of manually reviewing every frame, leading to significant time savings. Beyond simply extracting frames, these AI techniques refine the selection process. Methods like self-supervised learning and algorithms that consider motion between frames, enable a more intelligent and accurate identification of visually compelling frames. The increasing volume of video content, coupled with the expanding field of video analytics, creates a growing need for reliable and efficient ways to pinpoint key frames. This move towards automated and AI-driven frame selection marks a clear step forward in reliably extracting the most visually striking and informative images from videos. While still nascent, these techniques are beginning to redefine how we approach video content and extract meaningful still images from it. There's still room for improvement; we need robust methods that can handle diverse video types and complex scenes. However, the promise of achieving better results with less manual effort is undeniable.
The traditional approach to picking the best frames from a video often relies on a person's subjective judgment, but AI-powered frame analysis brings a new level of objectivity. It employs algorithms to assess and rank images based on specific factors, which leads to a more precise and accurate selection process. AI can quickly process thousands of frames, spotting subtle shifts in lighting and composition that might evade human observation. This capability helps to pinpoint optimal images that improve the overall visual quality.
These systems leverage deep learning to spot patterns within the footage. This lets them prioritize images with qualities like sharpness, clarity, and balanced composition, which are critical for achieving high-quality results after upscaling. The automation offered by AI-driven frame selection drastically cuts down the time spent in post-production. It automatically eliminates poor-quality frames, freeing up engineers and content creators to focus on the creative aspects of their work.
Furthermore, AI models can be taught to identify not only technical quality but also the message or emotional impact of a frame. This means they can select frames that better convey the intended story or feeling, which can enhance the overall narrative effectiveness. By incorporating edge detection algorithms, AI can precisely extract visually interesting features within a frame, helping to ensure that the final selection is aesthetically pleasing.
These tools can also adapt to individual user preferences, learning from past choices to refine future recommendations. This creates a personalized workflow that develops along with the user's specific style and requirements. Advanced frame analysis techniques can assess the movement within a video to favor frames where subjects are captured in dynamic or engaging postures. This leads to improved action shots or visually engaging scenes in narrative-driven content.
AI can also significantly streamline workflows through batch processing. Rather than working on one frame at a time, AI can analyze entire sequences of footage in parallel, greatly increasing efficiency. It's crucial to note that while AI streamlines frame selection, it's essential to keep a discerning eye. Relying solely on automated systems could miss out on unique human insights and intuition that are often key to truly memorable visuals.
Extracting High-Quality Images from Video A 2024 Guide to Frame-by-Frame Conversion - Exploring Advanced Interpolation Techniques in 2024
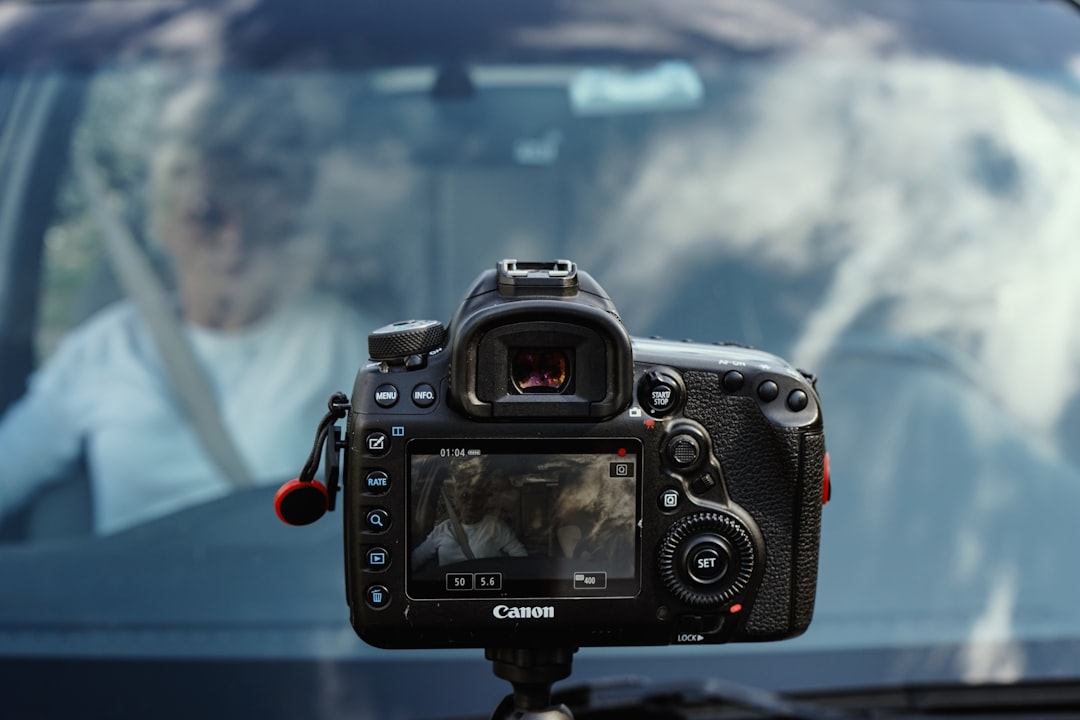
The field of video enhancement is seeing increased interest in advanced interpolation techniques, particularly in the realm of video frame interpolation (VFI), in 2024. These techniques, which aim to create new frames between existing ones, are being developed to improve video quality and create smoother motion. The pursuit of higher quality video is driving research into innovations like the FILM framework, which has shown promising results in generating slow-motion footage from nearly-identical images, and the use of texture consistency loss to address ambiguities in motion. The growing reliance on neural networks in VFI emphasizes the importance of better feature representation for creating seamless and natural-looking interpolated frames. Despite these advancements, there are still challenges. Existing evaluation methods are not considered robust enough, indicating a need for standardized benchmarks to more accurately compare and assess the performance of different VFI algorithms. This points towards an ongoing need for development and refinement in this area, as the desire for smoother, higher quality video continues to push researchers forward.
The field of video frame interpolation (VFI) is experiencing a surge of interest, with researchers actively developing new frameworks and methods to improve both accuracy and performance. One notable approach, VFIMamba, utilizes a State Space Model (SSM) adapted for VFI, employing a MixedSSM Block (MSB) to efficiently model the relationship between frames. However, current evaluation techniques for these methods are still lacking, highlighting the need for improved benchmarking standards.
There's an increasing push to address the challenge of motion ambiguity in VFI. Texture consistency loss (TCL) is a new supervision approach that leverages cross-scale features to enhance alignment accuracy during the interpolation process. This need to refine alignment accuracy underlines a growing awareness that better handling of motion ambiguity is crucial for achieving higher-quality results.
Frame interpolation has demonstrated impressive capabilities, particularly in the realm of slow-motion generation. FILM (Frame Interpolation for Large Motion) stands out for its ability to transform nearly identical photos into smooth slow-motion footage, resembling a video camera's output, which showcases significant progress in high-quality frame interpolation. These advancements show a trend toward utilizing complex neural networks to improve quality and model temporally consistent frames.
Furthermore, researchers are investigating interframe attention mechanisms to more efficiently extract both motion and appearance information from videos. By refining feature representation within the interpolation process, they hope to achieve more accurate and nuanced results. Essentially, VFI combines enhancement and reconstruction techniques to produce smoother visual outputs that aim for a higher level of perceptual quality.
While impressive, many advanced methods still face challenges in complex scenarios. Rapid motion or occlusions can present hurdles that current techniques struggle to overcome. Moreover, the integration of 3D estimations into some interpolation methods is a promising area of research. It seeks to provide a deeper understanding of video scenes, ultimately leading to more realistic output, especially when handling dynamic imagery. Keeping these limitations in mind, ongoing research needs to focus on improving the robustness of these techniques across diverse video contexts to truly unlock the full potential of frame interpolation for image extraction.
Extracting High-Quality Images from Video A 2024 Guide to Frame-by-Frame Conversion - Balancing Resolution and File Size in Extracted Images
When extracting images from video, finding the right balance between image resolution and file size is critical. While higher resolution delivers better image quality, it often comes with significantly larger files. This can cause problems with storage and can make sharing online more difficult. To manage this, you can use methods like starting with lossless image formats for initial extraction and then using compression tools to reduce size when converting to a format like JPEG. Carefully controlling the level of compression and selecting the right output format lets you maintain a good image quality without creating files that are too large. By carefully tweaking these settings, you can extract images that look good and are a reasonable size to work with.
When extracting images from video, we often face the challenge of balancing the resolution and the resulting file size. There's a complex relationship between them—increasing resolution, which means more pixels, can significantly increase the file size, often exponentially. This trade-off becomes especially apparent when dealing with higher-resolution videos, such as 4K or 8K, where a subtle shift in resolution can quickly impact storage needs.
The choice of compression method also plays a key role. Lossy methods, like JPEG, significantly reduce file size but introduce artifacts or blurriness, particularly noticeable in areas with fine detail. Lossless compression methods, such as PNG, preserve the image's original data but usually lead to larger files. The type of compression we choose should depend on how we intend to use the extracted images; a JPEG may be suitable for web use, but a PNG might be better for further image processing or printing.
The number of bits used to represent each color component (bit depth) also has a notable effect on both file size and visual fidelity. Deeper bit depths, while capturing a wider range of colors and tones, lead to larger file sizes. For high-quality image extractions, we might find ourselves making compromises depending on the specific requirements of our projects.
Furthermore, aspects of human perception, which is how we perceive the visual world, can be used to optimize storage. Psycho-visual compression exploits the fact that our eyes are less sensitive to certain frequency ranges, enabling the compression algorithms to more aggressively discard less perceptible details. This allows for a smaller file size without a noticeable loss in perceived quality.
The DPI setting, which impacts print quality, needs to be factored in for specific applications. Higher DPI leads to sharper output when printed, but it also creates larger files. We need to balance quality based on the final intended purpose, be it digital displays or print media. Similarly, the color space chosen (like sRGB or Adobe RGB) will influence the file size because the latter uses more information per pixel. This means, if we choose higher-quality color space, we must account for the storage space requirement.
Moreover, many advanced methods can improve efficiency through data redundancy elimination. In videos, frames can often be highly similar, particularly if they're part of a steady or slowly changing scene. We can leverage these patterns, essentially removing the duplicated or redundant information, resulting in much smaller files. We call this temporal redundancy.
Finally, as resolution standards continue to increase, we should anticipate a shift towards higher resolution displays becoming ubiquitous. Choosing a lower resolution during extraction might lead to undesirable results in the future if these images are used on new, higher resolution displays. We must consider long-term compatibility when we make choices concerning resolution and storage during the extraction process. As a result, our selection of settings for frame extraction, a seemingly simple task, can have far-reaching implications for the overall outcome of our project. The interplay between resolution, compression techniques, and color settings demands careful consideration, especially as we continue to deal with ever-increasing resolution video content.
Extracting High-Quality Images from Video A 2024 Guide to Frame-by-Frame Conversion - Automating the Frame Extraction Process with Custom Scripts
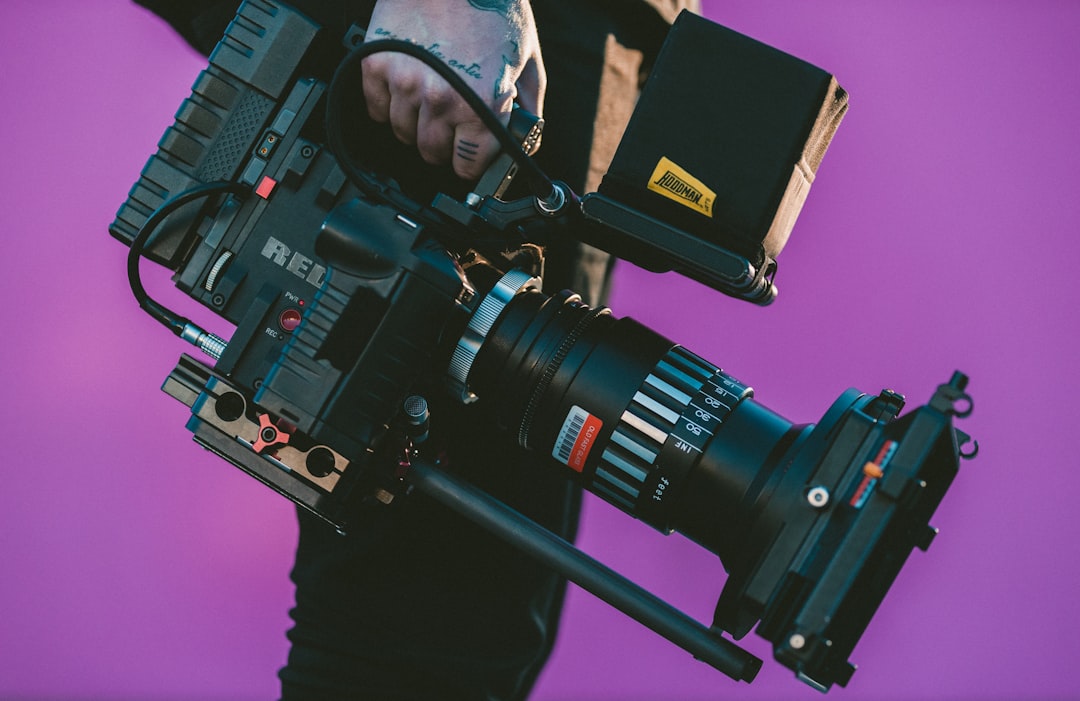
The ability to automate the frame extraction process through custom scripts has seen significant progress in 2024, making it easier to transform video content into a sequence of high-quality still images. Python offers a powerful way to customize the extraction process, enabling control over aspects like the frame rate and the resolution of the output images. Libraries such as OpenCV, readily available and popular for image and video processing, greatly simplify this task. Furthermore, online repositories like those found on GitHub provide ready-made tools for various extraction purposes, including optimized approaches. For instance, parallel processing can significantly speed up frame extraction from large video files, while frame sampling techniques help reduce redundancy in situations where there are many very similar consecutive frames.
Interestingly, there are also scripts that can help with intelligent frame selection. Advanced algorithms, like histogram analysis, can analyze the pixel distribution within each frame to identify scenes with significant changes, thus automatically selecting "key frames" that capture crucial moments within the video. While automation brings increased efficiency, it's important to be mindful of its limitations. Simply relying on automated tools to extract the “best” frames can sometimes overlook nuances that human judgment can easily spot. It is still wise to review the automated selection to ensure that the output matches the desired goals of the frame extraction. Overall, custom scripts offer a powerful means to take control of the process of extracting high-quality images from videos, but a balance between the speed of automation and human insight remains necessary.
1. Automating frame extraction with custom scripts can drastically increase processing speed, potentially reaching hundreds of frames per second. This is a major advantage over manually reviewing each frame, especially when dealing with high-resolution or lengthy videos. Leveraging parallel processing within the scripts is key to achieving this level of efficiency.
2. Beyond basic frame extraction, custom scripts can be designed to respond intelligently to changes within a video. This could mean prioritizing frames where the scene changes significantly or where there's a shift in emotional content, providing a more nuanced understanding of the video's narrative. It goes beyond just extracting images based on technical quality.
3. One benefit of using custom scripts is their ability to handle various video formats and codecs. By utilizing libraries that can effectively decode raw video data, engineers can extract frames from a wide range of videos without facing compatibility issues or sacrificing image quality. This allows for greater flexibility in working with different kinds of source material.
4. Implementing techniques like Otsu's thresholding in scripts allows for a more refined selection process. By intelligently identifying which pixel information is essential, we can minimize distractions caused by unnecessary background noise or unimportant details, resulting in cleaner and sharper extracted images.
5. Efficient memory management is a key aspect of writing effective extraction scripts. By carefully managing the allocation of memory, only necessary data is loaded during the extraction process. This optimization reduces the risk of crashes that can occur when working with very large video files, leading to more stable and reliable frame extraction.
6. Custom scripts offer the potential for integrating objective visual quality metrics into the frame selection process. Algorithms like SSIM and PSNR can be incorporated into the script to provide a numerical assessment of the quality of the extracted frames. This ensures that only frames that meet predefined quality standards are selected, enabling a consistent level of output.
7. Another intriguing feature of custom scripts is the ability to extract frames at multiple resolutions simultaneously. This flexibility allows users to tailor extracted images to different purposes. The same source video can yield frames optimized for web use as well as high-resolution prints, enhancing adaptability and convenience.
8. For certain projects where a smooth narrative flow is crucial, custom scripts can be designed to assess the temporal consistency between successive frames. This can ensure that extracted frames form a coherent sequence, important for applications that require a focus on narrative or chronological information.
9. The automation potential of custom scripts extends to batch processing. Entire sets of video footage can be systematically analyzed and frames extracted based on a predefined set of criteria. This saves tremendous post-production time by eliminating manual intervention and helps to standardize the quality of frame extractions across multiple projects.
10. A well-structured script allows for user-defined parameters. This means that content creators can specify factors like desired frame rate, output resolution, and quality thresholds. This level of customization ensures that frame extraction aligns precisely with individual project needs, granting greater control over the final outcome.
While still a relatively new field, the potential benefits of using custom scripts to automate frame extraction are clear. This approach provides engineers with the flexibility to optimize extraction based on the specific needs of the project. However, it's worth noting that relying on automated systems should not eliminate the importance of human judgment in ensuring that the extracted frames align with the creative intent of the project. Ongoing research and development will likely refine these methods further, improving the reliability and precision of frame extraction for various video content types.
Extracting High-Quality Images from Video A 2024 Guide to Frame-by-Frame Conversion - Preserving Metadata and Color Information During Conversion
When converting video frames into still images, preserving the original metadata and color information is crucial for maintaining high quality. Formats like TIFF are particularly useful because they can store multiple image layers, support a variety of color spaces, and hold detailed metadata, all important for preserving image integrity. However, changing file formats during the conversion process can lead to the loss of some data or cause changes in the appearance of the extracted image.
Therefore, choosing the right format and carefully managing the conversion process is important. For example, embedding wide-gamut metadata during the conversion process can greatly enhance color accuracy and ensure that extracted images retain their original vibrancy. Paying close attention to metadata and color space management can have a big impact on the quality and longevity of extracted images, which is especially important when the images are destined for specific applications such as digital archives or art preservation projects. A thorough approach to both metadata and color handling is essential to extract high-quality images from video that retain their original character.
When extracting frames from videos, it's crucial to think about preserving the original information embedded within the video, like the settings used to record it and how colors are represented. This includes things like resolution, frame rate, and the color profile used (like Rec. 709 for high-definition video). If we don't pay attention to keeping this metadata, we risk losing valuable context that's important for maintaining high-quality image results.
Color plays a huge part in how our eyes perceive an image extracted from a video. Maintaining the original color space is essential, especially in professional settings where accuracy matters. Otherwise, the extracted images might show colors differently than how they appear in the original video footage.
Using compression methods to reduce file sizes can be helpful, but we have to understand that it can sometimes affect both the image quality and the accuracy of the colors. It's a tricky balance between managing storage and preserving a high level of quality. Engineers need to be mindful of this trade-off during the extraction process.
The number of bits we use to store color information, called color depth, also affects both file size and visual quality. While deeper bit depths capture more color nuances, they lead to larger files, presenting a challenge for optimization.
Software tools like ffmpeg can greatly simplify the frame extraction process, but we need to be careful how we use them. If not configured correctly, we could end up losing important data.
Lighting conditions can significantly impact the color reproduction in extracted images, making it tricky to get accurate colors across different parts of a scene. Stabilizing lighting conditions is often a necessary step to maintain consistent color grading in extracted frames.
The way video codecs work can affect how color information is compressed. Some codecs process color differently, potentially leading to variations in color between extracted frames and the source video. This can be a problem for people who need color accuracy.
It's a good idea to use lossless formats for the initial frame extraction process, as this helps maintain the color fidelity and the original video metadata. This is one of the best ways to ensure the highest quality in the resulting images.
When we're adjusting colors after extracting frames, we need to be very careful. Color correction done incorrectly can distort the original color profile and make the images look different from the original video, compromising their usefulness for professional applications.
There's ongoing research into better color profiling software that lets us create custom profiles for specific types of videos. This leads to better color accuracy during frame extraction, showing us how far we've come in understanding and preserving image integrity during conversions.
Extracting High-Quality Images from Video A 2024 Guide to Frame-by-Frame Conversion - Integrating Frame Extraction into Professional Video Workflows
Integrating frame extraction into professional video workflows is increasingly vital for content creators aiming to improve image quality and streamline their processes. The ability to automate frame extraction, using tools like Python scripting and libraries like OpenCV, alongside advanced frame selection algorithms, significantly reduces the manual effort traditionally required. These improvements allow for efficient extraction of stills optimized for various purposes, such as web or print media. Furthermore, they support diverse video formats and ensure the integrity of important metadata and color information. However, while automation brings significant efficiency gains, maintaining a critical human role in the process is crucial to verify the artistic vision and ensure the selected frames are aligned with the project's objectives. In the evolving landscape of frame extraction, the most promising results will likely stem from a blend of automation and careful human oversight.
1. The effectiveness of frame extraction from video is heavily influenced by the characteristics of the original video codec. Certain codecs employ aggressive compression techniques that can lead to the loss of important visual details. Consequently, choosing codecs that prioritize data preservation during the extraction process is crucial for maintaining high image quality.
2. The video's frame rate also plays a role in the extraction process. While standard extraction usually operates at a set rate, extracting more frames from high frame-rate videos can dramatically improve the quality of slow-motion sequences. This can result in clearer, more detailed images for analysis, revealing finer aspects of the motion.
3. Color science is fundamental for accurate frame extraction. Utilizing various color spaces, like Rec. 709 or Rec. 2020, can significantly impact the representation of color detail and saturation in extracted images. These choices affect not only the visual fidelity but also how images are perceived across different displays, potentially leading to varying interpretations of the captured scene.
4. Human visual perception has limitations that can influence frame selection. For example, we're less sensitive to changes in brightness when objects are moving compared to when they are stationary. Recognizing these limitations can guide engineers to make better choices about which frames to extract, favoring scenes with distinct visual differences, even if those differences are subtle enough to go unnoticed in casual viewing.
5. Maintaining metadata isn't just about recording settings; it's also about the viewing environment. Details about the display's illumination and color calibration can be crucial for achieving consistent image appearance across different viewing conditions. Integrating this type of information into extracted images can enhance their value by helping to maintain accuracy when the images are viewed in diverse settings.
6. Researchers are actively developing sophisticated machine learning techniques to predict which parts of a video will be most engaging for viewers based on behavioral analysis. This predictive power can inform frame extraction, highlighting segments that may resonate better with the target audience, leading to more effective visual storytelling.
7. Maintaining temporal continuity is particularly important in narrative-driven video content. Extracting frames that follow a clear chronological order helps preserve the flow of the story. This is crucial in cinematic or complex storytelling where the order of events plays a pivotal role in creating a meaningful experience for viewers.
8. It's vital to understand that compression algorithms can introduce visual artifacts, like color banding or blocky textures. These artifacts might not be present in the original video but can emerge during the frame extraction process. Recognizing the potential for such imperfections can enable engineers to make smarter choices during extraction and format conversions.
9. The relationship between resolution and bit depth significantly impacts the perceived quality of extracted images. Higher bit depth captures finer tonal variations, which enhances visual fidelity. However, when coupled with high resolutions, it significantly increases the storage and processing demands, potentially creating challenges for managing extracted data.
10. Frame extraction methods are often tailored to the intended application of the extracted images. For instance, images destined for online use might prioritize speed and file size optimization. In contrast, images intended for high-quality printing will prioritize high resolution and accurate color representation, underscoring the adaptability of extraction workflows to diverse needs.
More Posts from ai-videoupscale.com: