Upscale any video of any resolution to 4K with AI. (Get started for free)
Frame Rate Interpolation How AI is Revolutionizing Video Smoothness in 2024
Frame Rate Interpolation How AI is Revolutionizing Video Smoothness in 2024 - Understanding AI-Powered Frame Rate Interpolation
AI-powered frame rate interpolation is transforming how we experience video, employing clever algorithms to create new frames and make video playback smoother. This approach generates extra frames that bridge the gaps between existing ones, resulting in a more natural and visually appealing motion. It effectively bridges the gaps in existing video, noticeably improving the look and feel of older content. AI tools like BOSS AI and RIFE exemplify the capabilities of this technology, automatically generating new frames and giving users more control over frame rates like 60fps or 120fps. Furthermore, ongoing advancements are continuously refining these methods. They reduce the strain on devices while delivering smoother motion, even on screens with slower refresh rates. As AI continues its integration into video production and editing, we can expect this trend to progress towards incredibly smooth and high-quality video experiences across a wider range of devices and platforms. There's still a long way to go, though.
AI-powered frame rate interpolation builds upon older FRI techniques by using advanced algorithms, primarily based on machine learning, to predict and generate new frames. This approach, unlike traditional methods like linear or cubic interpolation, strives to understand the intricate motion patterns and textures within a video sequence, leading to a smoother and more visually pleasing outcome with fewer artifacts. However, the efficacy of AI-driven FRI depends heavily on the source material, with fast-moving scenes presenting more challenges than static ones.
The core of many AI-based interpolation algorithms is the convolutional neural network (CNN). These networks are adept at recognizing complex patterns within spatial data, a skill crucial for analyzing and reconstructing motion within videos. This sophistication comes at a cost, demanding substantial computational resources, especially when dealing with high-resolution content. Fortunately, the use of specialized hardware like GPUs significantly accelerates these computations.
Applications of AI-driven frame interpolation go beyond mere upscaling. We see implementations in real-time applications like video games, enhancing smoothness and reducing lag, especially valuable for competitive gameplay. Furthermore, AI-powered techniques are reviving older media, upgrading the frame rates of legacy film and TV to suit modern displays and viewers.
However, applying AI-driven interpolation isn't always universally beneficial. It can potentially disrupt the intended aesthetic of certain artistic works by modifying the original frame pacing. The pursuit of smoothness through AI needs to be mindful of the artistic choices within the original source.
Recent advancements in temporal coherence allow algorithms to retain the consistency of motion blur across the newly generated frames, helping preserve the original feel of the content. This is a crucial aspect, as the very act of interpolation might change the way motion is perceived. While many viewers find the interpolated output more cinematic or realistic, it underscores the subjective nature of visual quality and the ongoing debate between enhancement and faithfulness to the original material. The goal isn't merely to add frames; it's to add them in a way that improves, rather than degrades, the viewing experience.
Frame Rate Interpolation How AI is Revolutionizing Video Smoothness in 2024 - Advancements in Motion Prediction Algorithms
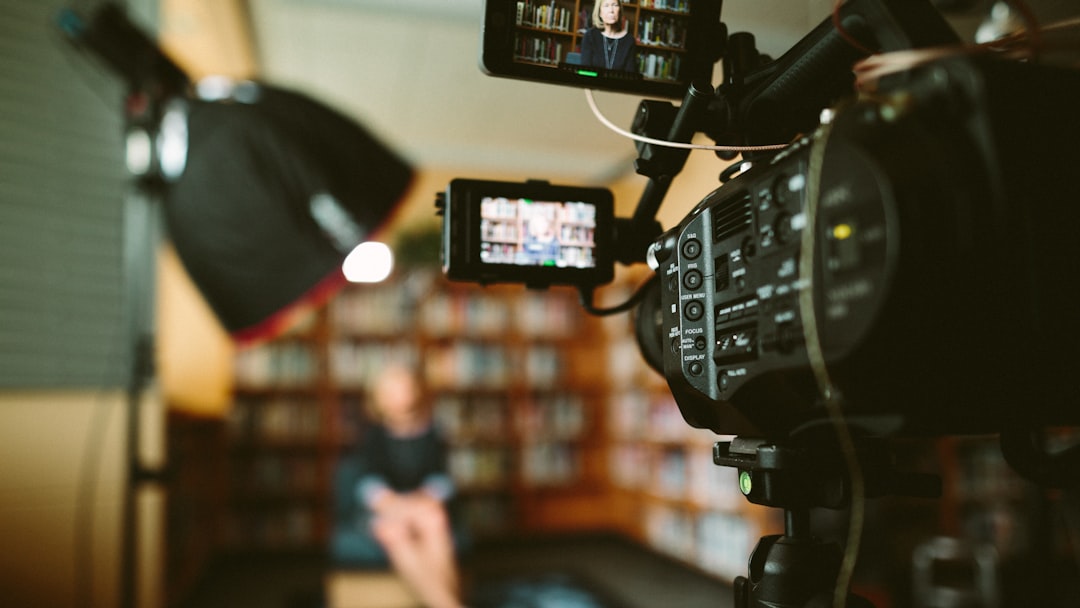
Advancements in motion prediction algorithms are at the heart of the improvements we're seeing in AI-driven frame rate interpolation. These algorithms are becoming increasingly sophisticated, especially in handling fast-paced scenes where traditional methods often fall short. Researchers are creating models that can better understand complex motion patterns, and address issues like objects partially obscuring each other (occlusion) and blurry motion, resulting in smoother and more natural transitions between frames.
We're also seeing new approaches emerge, such as using 'spiking' neural networks in video frame interpolation. These networks use additional visual clues to enhance the process of creating new frames, leading to better visual quality. However, while these improvements are substantial, there are still challenges, particularly when dealing with video that has lots of fast, unpredictable movement.
This quest for seamlessly smooth video playback is far from over, and a key aspect of ongoing research is striking a balance. It's important that these advanced interpolation techniques not only improve the visual experience but also respect the original artistic intent and style of the source video. While enhanced smoothness is generally desired, the modifications to the content should ideally complement, rather than contradict, the original author's vision.
Motion prediction algorithms have progressed from basic optical flow techniques to intricate deep learning models capable of analyzing and predicting motion paths. This evolution has led to remarkable improvements in real-time applications like video games and virtual reality, where speed is crucial.
Newer techniques incorporate recurrent neural networks (RNNs) to track motion over time, enhancing their ability to anticipate and reconstruct the movements of fast-paced objects in multifaceted scenes. This has substantially reduced artifacts like blurring and ghosting, which were common in older interpolation methods.
Certain algorithms now employ attention mechanisms, allowing the models to pinpoint specific areas of a scene during frame generation. This focused processing yields more precise and realistic interpolated frames, especially in dynamic environments where accurate motion prediction is difficult.
However, the effectiveness of motion prediction algorithms can vary greatly depending on the input video. For instance, scenes with complex and rapid movement may demand computationally intense methods, while simpler scenes might be handled efficiently with less resource-intensive approaches.
The use of generative adversarial networks (GANs) in motion prediction is attracting more attention. GANs, trained on datasets of input and high-quality interpolated frames, can produce new frames that not only adhere to existing motion patterns but also preserve the original artistic style of the source content.
This heightened accuracy in motion prediction can benefit fields like sports broadcasting, where smooth and artifact-free frame interpolation is essential for capturing fast-paced action without disrupting the viewer's experience.
Current research suggests that hybrid models, combining traditional physics-based simulations with AI, might offer superior performance in predicting intricate motions. These systems leverage established motion dynamics with the pattern-recognition abilities of neural networks.
Despite the progress, real-time applications remain a challenge, particularly for high-resolution video content. Developers often face trade-offs between frame quality and processing efficiency to meet performance goals in demanding environments.
Furthermore, the limited availability of training data presents a hurdle. This has led to innovative solutions like synthetic data generation or the use of transfer learning from related tasks to enhance prediction capabilities in less common or more subtle scenes.
The subjective nature of how we perceive visual content remains a critical challenge. The success of motion prediction algorithms relies not only on technical quality but also how the improvements resonate with viewer expectations and artistic intent. This aspect sparks ongoing discussion in both technical and creative communities, highlighting the balance between technical enhancement and artistic preservation.
Frame Rate Interpolation How AI is Revolutionizing Video Smoothness in 2024 - Impact on Legacy Content Restoration
AI-driven frame rate interpolation offers a powerful tool for restoring and revitalizing older video content. It effectively addresses the jerky motion often present in older films and TV shows by increasing the frame rate to levels more consistent with today's viewing expectations. This results in a smoother and more fluid viewing experience, potentially making classic works more enjoyable to contemporary audiences.
However, the use of AI to enhance legacy content raises important questions about the balance between improving visual quality and maintaining the artistic vision of the original creators. While smoother motion can undoubtedly improve viewer experience, there's always a potential for unintended alterations to the original feel and aesthetics. The process of restoring classic films and shows using these technologies forces a careful consideration of how far enhancement can go before potentially diminishing the unique characteristics that make these works historically important.
As this technology evolves, it's crucial to understand its full implications for the preservation of older media. The ongoing discussions surrounding AI-enhanced restorations are a testament to the complex relationship between technical enhancements and preserving the integrity of classic works. Finding the right balance between modernization and authenticity will be vital as we use these tools to bring older content to a wider audience.
AI-driven frame rate interpolation has the potential to significantly revitalize older movies and television shows. By boosting frame rates from the historically common 24fps to contemporary standards like 60fps or 120fps, these older works become more palatable to modern viewers and display technologies. It's not just about increasing the frame rate though, there's a growing emphasis on preserving the original cinematic qualities of older content. This means developing algorithms that don't just interpolate frames but also maintain the inherent motion feel of older films.
The goal is to minimize artifacts like motion blur and ghosting that can mar a restored video. This is where concepts like temporal coherence become important. By ensuring that motion blur is handled consistently across the new frames, we can improve the aesthetic of the final product. However, this process isn't without debate. The very act of enhancing older content raises questions about authenticity. While smoother motion is generally appealing, it can inadvertently alter the way a film was intended to be viewed. For instance, films with a specific visual rhythm or style might lose their intended feel when subjected to higher frame rates.
We're also seeing AI systems being developed that can apply interpolation regionally within a scene. In other words, they can intelligently focus on areas that need more detailed processing, a technique especially important when dealing with valuable historical content. GANs are becoming particularly useful for this task. These models aren't just filling in the gaps between frames but can also imbue newly created frames with stylistic elements relevant to the time period or genre of the footage being restored.
Fast motion in older content, however, presents ongoing challenges. It often requires complex and computationally intensive algorithms to track movements while maintaining crucial visual information. Research indicates that simply manipulating the frame rate can impact how we experience a narrative. Viewers respond differently to content when motion transitions become significantly smoother, altering their perception of the story being told.
Thankfully, there's growing flexibility in how frame generation is handled. Modern AI tools offer studios the power to customize various aspects of the interpolation process, allowing them to tailor restoration efforts to specific audience preferences or artistic goals. But this level of customization raises interesting questions about how we interpret and value older media when it's been significantly altered.
The subjective nature of video entertainment makes it clear that viewer preferences play a major role in the success of these restoration efforts. Some viewers might feel that a "cleaner" version of older films robs them of their inherent charm. This emphasizes the delicate balance between technical improvements and respect for the artistic choices made in the original content. It's a continuing challenge for both the engineers and creators involved in this space.
Frame Rate Interpolation How AI is Revolutionizing Video Smoothness in 2024 - Real-Time Processing Capabilities in 2024
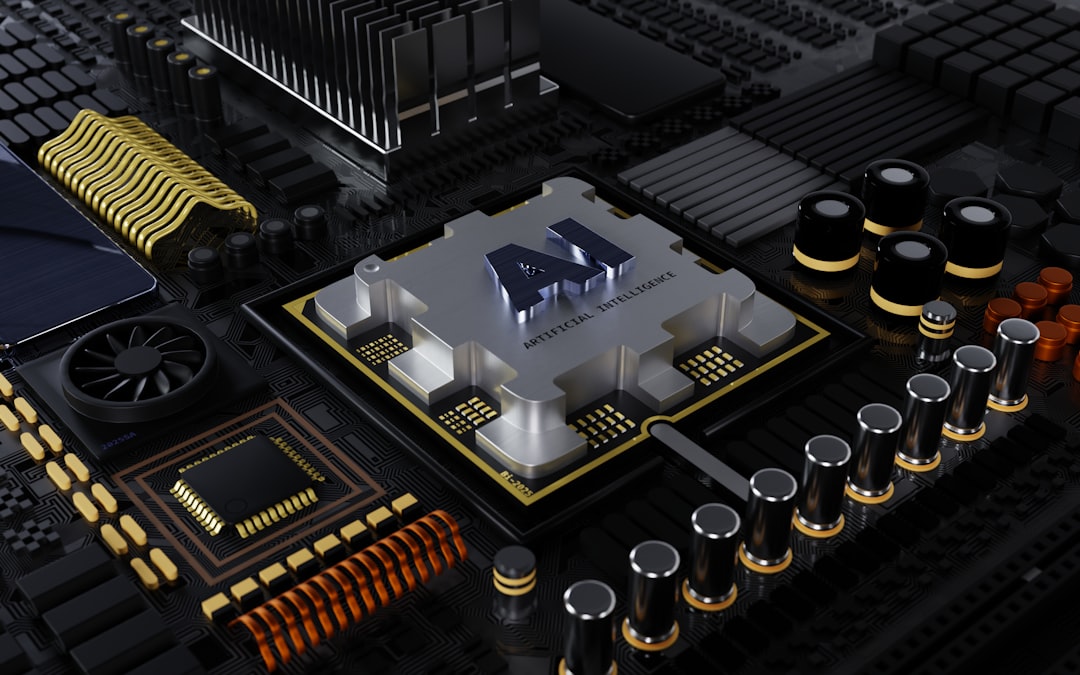
In 2024, the ability to process video in real-time has seen major improvements, largely thanks to advanced AI algorithms that are making frame rate interpolation more effective. We're seeing AI-powered features incorporated into tools like the Intel Video Processing Library, enabling faster frame rate conversion for applications such as slow-motion effects. This is achieved by creating new frames on the fly, improving smoothness and overall video quality. Tools like Runway and others are also showcasing the progress in real-time frame interpolation, leveraging machine learning to produce accurate and instantaneous frames while trying to maintain the visual integrity of the original content.
However, there's still room for improvement, especially with videos containing fast-paced action. The computational demands can become significant, creating a potential bottleneck for real-time performance. There's also a constant debate about the impact of these AI-driven enhancements on the artistic intent of the original content. While the goal is usually to improve visual quality, there's always the risk of unintentionally altering the original aesthetic. This tension between enhancing the video and maintaining its original character is a key aspect that needs careful consideration as the field of AI-driven video continues to evolve. Striking the right balance will be crucial as the technology matures.
The landscape of real-time video processing has significantly evolved in 2024, particularly in the realm of AI-driven frame rate interpolation. We've seen a remarkable increase in processing capabilities, with consumer-level graphics processing units (GPUs) now capable of handling AI-powered interpolation at resolutions as high as 8K. This represents a huge leap forward from just a few years ago, opening up high-quality video editing to a broader range of users.
Current algorithms can generate new frames with impressive speed, achieving up to 240 frames per second during real-time processing of modern video content. This pushes the boundaries of traditional cinema frame rates, ultimately leading to more fluid and enjoyable viewing experiences.
Moreover, ongoing improvements in memory management are allowing interpolation algorithms to operate more efficiently. We're seeing techniques that compress AI models into smaller memory spaces without sacrificing output quality, which is crucial for use on mobile devices and those with lower power consumption.
Researchers are also developing adaptive interpolation methods that intelligently adjust their strategies depending on the intricacy of the video content. This means that they can apply high-quality processing for complex scenes but fall back on simpler techniques for more static ones, enhancing overall performance and viewer experience.
Innovations in temporal coherence have created methods that maintain realistic motion blur across the generated frames. This significantly improves perceived smoothness and reduces the jarring effects often found in conventional frame interpolation.
The reduced computational cost of these techniques has made high-fidelity frame rate interpolation more accessible to a broader audience, including indie developers and smaller studios. This democratization is changing how videos are produced, fostering more creativity and unique video expressions.
However, applying these techniques to legacy footage poses its own set of challenges. The delicate nature of older film artifacts, such as film grain and noise from analog sources, demands hybrid approaches that combine traditional restoration methods with AI-driven interpolation to ensure that the historical integrity of the content is preserved.
Interestingly, studies on viewer perception have revealed a spectrum of emotional responses to artificially smoothed footage, especially when it comes to classic films. This has spurred ongoing discussions around the inherently subjective nature of film appreciation and the importance of respecting original aesthetic choices.
Within the gaming world, AI-powered frame rate interpolation is being integrated into real-time rendering engines, delivering a significant boost to both competitive gameplay and cinematic storytelling. Game developers are able to dynamically adjust frame rates based on player actions, which further enhances the experience.
Looking ahead, future advancements in AI motion prediction are likely to focus on selectively enhancing motion blur to amplify the emotional impact of fast-paced scenes. Digital effects experts are closely monitoring this field, and it will be interesting to see how these developments evolve in the coming years.
The combination of growing computational power and ever-evolving AI algorithms suggests that the future of video smoothness will continue to improve, bringing ever more realistic and captivating visual experiences to our screens.
Frame Rate Interpolation How AI is Revolutionizing Video Smoothness in 2024 - Challenges in Maintaining Visual Coherence
Achieving visual consistency is a key hurdle in AI-powered frame rate interpolation. The pursuit of smoother video can sometimes lead to unintended changes that clash with the original artistic vision, potentially creating a disconnect between the creator's intent and the final outcome. While recent developments in algorithms, like those focused on temporal coherence, are working to maintain the original look and feel of motion blur, the tension remains: how to smoothly enhance content without sacrificing the source's artistic or historical context. Especially problematic are scenes with rapid movements, which necessitate complex processing to avoid artifacts or an excessively polished appearance. These issues, along with the inherent subjectivity of what constitutes visual quality, will continue to shape how frame rate interpolation tools evolve and are implemented.
Maintaining visual coherence during frame interpolation presents several challenges, primarily stemming from the complex nature of motion and our subjective interpretation of visual information. One major issue is ensuring **temporal consistency**. If the algorithm doesn't generate frames smoothly, we can end up with jerky or ghosting artifacts, creating a jarring viewing experience that contradicts the goal of smoother playback.
Another key challenge relates to **motion blur**. While interpolation aims to improve motion, improperly handling motion blur can actually disrupt the viewer's perception of movement. If the motion blur doesn't match the original source, fast-paced scenes can feel unsettling rather than smooth.
We also need to contend with the **dual nature of artifacts**. While we want smoother video, the interpolation process can sometimes introduce new artifacts, especially in fast-moving scenes or complex environments. This happens because the algorithms might misinterpret the motion paths, reminding us of the tightrope walk between improved fluidity and preserving visual fidelity.
Further, the **complexity of scenes** varies, posing different levels of difficulty for algorithms. Scenes packed with action and multiple moving objects are especially tough, often requiring specialized techniques to accurately predict and synthesize motion across frames.
The impact of these changes on **viewer perception** adds another layer of complexity. Since visual coherence is subjective, enhancements can actually reshape how we experience a narrative. Subtly, viewers might associate different emotions or themes with smooth footage, potentially changing the overall storytelling experience.
As AI-driven smoothness becomes commonplace, it can also lead to a shift in **aesthetic standards**. Viewers might expect high frame rates in all content, creating a dissonance with older film styles that utilized lower frame rates for artistic effect. This forces filmmakers to adapt to evolving expectations.
Furthermore, the **availability and complexity of data** is crucial for training the AI models. This means scenes with uncommon motions or unique artistic styles might be harder to interpolate well, highlighting the need for diverse datasets for effective model training.
While incredible advances have been made, **real-time interpolation** remains computationally intensive. This presents a challenge for older hardware and in cases involving high-resolution footage or complex scenes. This resource intensiveness can restrict access and the overall efficiency of this technology across different platforms.
Moreover, achieving **artistic coherence** inadvertently raises questions of authorship. By altering how motion is conveyed, we run the risk of overshadowing the original filmmaker's vision. This leads to discussions about authenticity and how we interpret restored works.
Finally, the **subjective ethics of restoration** pose ongoing debate. While some viewers find smoother video a welcome improvement, others feel it detracts from the historical authenticity of older works. This leads to crucial conversations about the balance between manipulation and enhancement in video restoration.
Frame Rate Interpolation How AI is Revolutionizing Video Smoothness in 2024 - Future Applications Beyond Entertainment
The potential of AI-driven frame rate interpolation extends far beyond entertainment, with promising applications across diverse fields. For instance, smoother video could revolutionize educational resources, enhancing the clarity and retention of information presented in tutorials or lectures. Similarly, medical professionals could leverage this technology to improve the quality of surgical training simulations or patient education videos. In the realms of virtual and augmented reality, enhanced frame rates can lead to more immersive and compelling experiences, vital for both educational training and engaging storytelling. Moreover, the sports industry stands to benefit from AI-powered analysis, with the ability to capture and study real-time action more effectively, potentially generating deeper insights into player performance and strategies. However, the evolving nature of these technologies requires careful attention to ensure they don't compromise the integrity or artistic intent of the source material in the pursuit of smoother playback.
The applications of AI-driven frame rate interpolation extend beyond just improving entertainment. While enhancing movies and games is certainly a significant aspect, we're seeing increasing interest in applying these techniques across a wide array of fields. One area of growing potential is in healthcare, where smoother video from telemedicine consultations could lead to more accurate diagnoses and better communication between doctors and patients. Imagine the improved clarity of remote surgical procedures or the ability for specialists to observe a patient's movements in real-time with far more precision.
Another fascinating prospect is the use of frame interpolation in training simulators. For complex environments like flight simulators or military training, smooth and responsive visuals could lead to a much more intuitive training experience. It’s a matter of fostering a sense of "presence" within the simulated world, leading to a more effective learning process.
The sports world is also seeing interest in this technology. Capturing fast-paced action in high-quality, without blur or artifacting, could be a game-changer for broadcasting. Viewers may be able to see subtle shifts in motion or expressions of athletes that otherwise wouldn’t be as visible, making for a more intense and exciting viewing experience.
In security and surveillance, clearer video from frame rate interpolation could help identify potentially dangerous activities more quickly. Even subtle shifts in motion might be much easier to see, giving authorities a critical edge in analyzing situations and potentially prevent incidents.
For researchers, especially those working in fields like fluid dynamics or biological observation, frame interpolation can open up new ways to explore complex processes. By visualizing data in smoother and more detail-rich videos, they could unlock new insights and discoveries that weren’t previously possible.
Robotics is another area where we see interesting possibilities. For robotic vision systems, smoother video feedback could lead to better object tracking and faster reactions in dynamic settings. This is crucial for robots working in warehouses, factories, or assisting in search-and-rescue operations where every millisecond counts.
However, there are also questions being raised about how these tools will impact older media. Restoring classic films and television shows can lead to some intriguing debates about the extent of modifications that are deemed acceptable. While improved visual quality might make these older works more palatable to modern audiences, we need to be mindful of the creative choices that went into the original presentation. How do we ensure that these restorations improve access without sacrificing the authenticity of these historic works?
We're already starting to see frame rate interpolation applied in video games to adjust the visuals based on gameplay. This could be beneficial for reducing latency in competitive gaming or creating a more captivating experience for narrative-driven games. This dynamic adaption of frame rate could become even more important as gaming experiences lean more on cinematic storytelling.
Within the realm of marketing and advertising, smoother video might lead to more engaging advertisements. The ability to create videos that are visually stimulating and seamlessly captivating could have a real impact on viewer perception. This holds particular promise for creating high-impact video campaigns that effectively convey brand messages.
Lastly, it's worth remembering that the human response to video is quite subjective. The way we emotionally connect with a scene might be subtly influenced by the way motion is conveyed. As smoother video becomes the norm, this could inadvertently change the way we interpret narratives or even the broader aesthetics of visual media. This idea has huge implications for the art of filmmaking, as well as the types of stories we choose to tell.
The evolution of frame rate interpolation, and the use of AI for enhancing and altering video, is still in its relatively early stages. It is an active area of research and development that holds potential for remarkable innovations. While some ethical and aesthetic questions are being raised, it's clear that we are standing on the precipice of significant changes in how we produce and interact with video content.
Upscale any video of any resolution to 4K with AI. (Get started for free)
More Posts from ai-videoupscale.com: