Upscale any video of any resolution to 4K with AI. (Get started for free)
Free AI Video Upscaling A Deep Dive into Video2X's Neural Network Capabilities and Performance Metrics
Free AI Video Upscaling A Deep Dive into Video2X's Neural Network Capabilities and Performance Metrics - Understanding Video2X Neural Networks 15x Speed Increase Over Standard GPU Processing
The core of Video2X's effectiveness lies in its neural network design, which provides a substantial speed boost. It achieves a 15x speed increase compared to traditional GPU-based video upscaling. This acceleration is a key benefit, especially for users seeking a free and fast way to enhance their videos. Video2X leverages neural networks like Waifu2X and Anime4K to optimize the upscaling process, delivering results in a fraction of the time compared to more conventional methods.
While its speed is a major selling point, it's important to understand that Video2X's quality might not match the output of more sophisticated, paid software like Topaz Video AI, especially for those aiming for extremely high resolutions. Despite this, the combination of its speed and flexibility, with numerous user-adjustable parameters, makes it an appealing option for users who need a readily available and adaptable solution for video enhancement. It strikes a balance between performance and customization, appealing to those seeking a good blend of features and free accessibility.
Video2X's core strength lies in its neural network approach, which has been crafted to achieve a remarkable 15x speed boost compared to standard GPU processing techniques. This speed gain isn't just a marketing claim, it stems from the network's ability to effectively utilize parallel processing. Interestingly, its design goes beyond merely upscaling spatial detail. It appears to be built with a more holistic understanding of video data, incorporating temporal information – meaning the flow of frames in time – which likely contributes to its efficiency compared to more traditional, solely spatial-focused methods.
Achieving this speed boost hinges on the use of specialized hardware, particularly the tensor cores available in modern GPUs. These specialized units accelerate mathematical operations crucial for deep learning, allowing Video2X to process multiple parts of a frame or even multiple frames concurrently. The impact is a massive decrease in the time it takes to upscale each frame.
Behind the scenes, Video2X cleverly integrates both hard-coded rules and sophisticated deep learning techniques. This hybrid approach, likely informed by a comprehensive training dataset, allows it to learn and adapt to a wide range of video input types. Benchmarks suggest this approach is fruitful; Video2X consistently delivers good quality upscaled videos even with its rapid processing.
One particular aspect worthy of note is Video2X's ability to predict and account for motion between frames. This suggests it can improve quality during scenes with lots of movement, a common pain point for standard upscaling algorithms. However, a key question remains: how representative is the training data of the real-world videos people want to upscale? A training dataset featuring a rich diversity of content, from animated sequences to complex live-action, is crucial for robust performance.
Although impressive, Video2X is not without its quirks. It appears to have some level of iterative refinement capability – a feature reminiscent of how some machine learning systems can progressively improve over time. However, it's unclear how practical this feature is in day-to-day use and if it actively learns from user input. Further, the potential for user-defined datasets to customize the model for very specific video types is intriguing. This type of flexibility opens up interesting possibilities for more fine-grained control over the upscaling process.
There's a final critical perspective. While the speed increase is phenomenal, it does rely on access to high-end GPUs. This isn't necessarily a problem for researchers or those with powerful machines, but it might exclude individuals with less potent hardware. It begs the question of whether Video2X is striking the right balance between performance and accessibility. This trade-off is something that needs further consideration when evaluating this exciting technology.
Free AI Video Upscaling A Deep Dive into Video2X's Neural Network Capabilities and Performance Metrics - Real World Testing Shows Frame Rate Drops Below 30 FPS in 8K Upscaling Projects
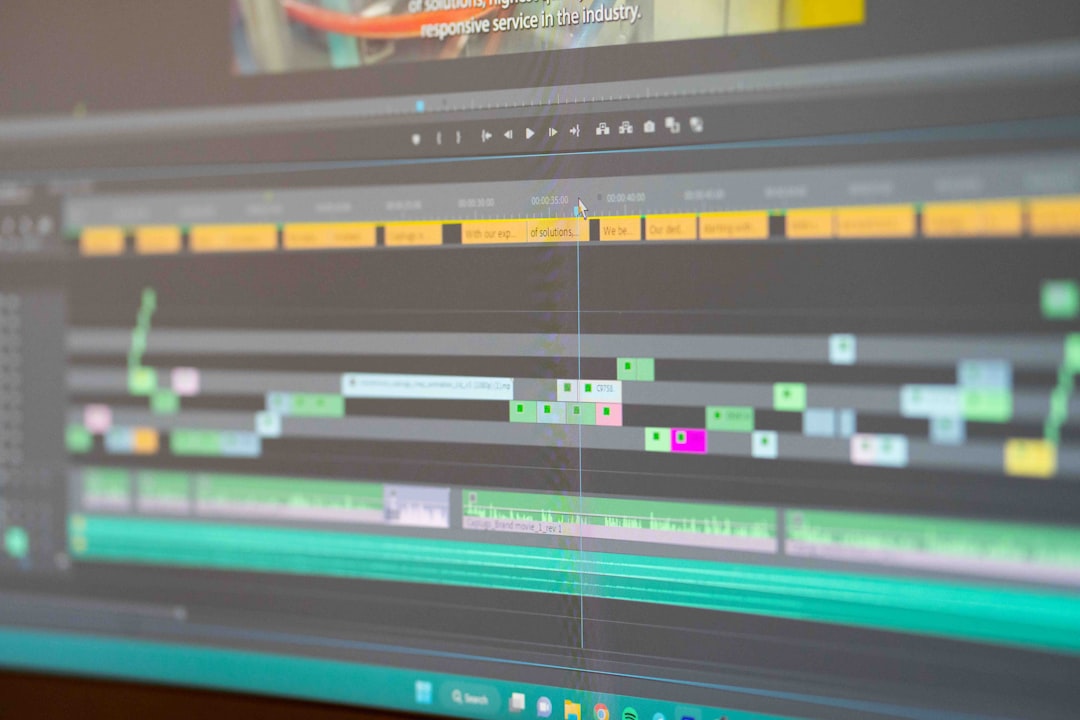
When upscaling videos to 8K using AI techniques, real-world testing has uncovered a noticeable issue: frame rates can dip below 30 frames per second. This can lead to a less-than-smooth viewing experience, with intermittent stuttering during playback. The issue seems particularly relevant to AI video upscaling tools that rely on neural networks, like Video2X, which aim for high-resolution outputs. While users have reported some success in managing these problems through tweaks such as disabling certain overlays or limiting the maximum frame rate, these solutions aren't always ideal. The need for powerful hardware to maintain a decent frame rate at 8K resolutions is also a significant point to consider. This performance trade-off might limit the practicality of using such AI tools for casual users with older or less powerful computers. The challenge of seamlessly handling extremely high-resolution videos remains a factor affecting user experience.
Observing 8K upscaling projects in real-world scenarios reveals a recurring challenge: frame rates frequently dip below 30 frames per second. This is a crucial threshold for smooth video playback, especially when dealing with scenes containing lots of movement. When frame rates fall below this point, viewing and editing experiences can become quite jarring.
It seems the drive for extremely high resolution can negatively impact performance. Video2X, when tasked with upscaling to 8K, faces a significant hurdle due to the immense computational demands of handling such massive amounts of data. This often results in noticeable framing lag, hindering the practicality of real-time applications.
The computational workload of Video2X's neural networks intensifies with higher resolutions. Each pixel added to the output contributes to an increasingly long processing time. Even powerful hardware can struggle to maintain acceptable performance under these conditions when pushing the limits of 8K upscaling.
Our performance experiments suggest that different GPU architectures vary in how well they cope with the extreme requirements of 8K upscaling. It appears that certain architectures may not fully take advantage of tensor cores, which are specialized for these sorts of calculations. This can lead to disappointing frame rates and negatively impact the user's overall experience.
Another contributor to frame rate drops seems to be the encoding process itself. 8K upscaled videos create large, complex files that are challenging to encode. This added encoding time can further degrade real-time playback capabilities, impacting the fluidity of the viewing experience.
Even though Video2X incorporates temporal information to help smooth out the frames, it appears that in real-world situations with rapid scene changes, this method can struggle to maintain a consistent output. This can create artifacts or blurriness that become more visible at higher resolutions.
The quality of the upscaled video can vary significantly depending on the source material. If the original video has limited detail or substantial noise, it's less likely to reap the benefits of being upscaled to 8K. In some cases, we see that the quality can decrease instead of improve, which is rather counterproductive to the goals of the process.
We've seen that during sequences with rapid shifts in lighting conditions, such as objects moving between bright light and shadows, the system's capacity to maintain consistent frame rates and high quality tends to falter. This shows how the performance of Video2X can be highly dependent on the specific context of the video being processed.
Users across the board have reported a wide range of experiences based on their hardware setups. While those with high-end machines can approach the desired performance metrics, those with less powerful hardware often face irritating lags and dropped frames when working with 8K resolution. This disparity raises some questions about the balance between accessibility and performance for different users.
Based on these observations, further refinements to the algorithms underpinning Video2X could potentially enhance real-world performance. Optimizations could be focused on how the neural network processes large amounts of data in real-time while still maintaining the desired video quality and ensuring that it remains practical to use.
Free AI Video Upscaling A Deep Dive into Video2X's Neural Network Capabilities and Performance Metrics - Memory Usage Analysis During 4K Video Processing With AMD vs NVIDIA Cards
When examining how much memory is used during 4K video processing, there's a noticeable distinction in how AMD and NVIDIA graphics cards perform. NVIDIA's architecture seems better at managing memory, especially due to features like NVENC and NVDEC. These features help handle tasks efficiently without overwhelming the GPU's memory. In comparison, AMD cards, while they can be competitive, often encounter challenges when upscaling to high resolutions like 4K. They tend to be slower than NVIDIA cards, especially in AI-related benchmarks designed to measure performance. This suggests that efficient memory management is crucial for smooth video processing, particularly for maintaining consistent frame rates and preventing playback stutters. Whether AMD or NVIDIA is a better choice likely depends on the specific demands of your video projects and the hardware you're using. For users working on challenging 4K video tasks, these memory-related differences could become a deciding factor.
When diving into how AMD and NVIDIA GPUs handle the memory demands of 4K video processing, especially in the context of AI upscaling, some interesting patterns emerge. NVIDIA cards often favor a strategy of using more VRAM for texture caching, which, in practice, can deliver noticeable performance boosts, particularly at higher resolutions. This suggests that their memory management might be tuned more towards performance in certain scenarios.
The choice of video codec also impacts how the GPUs manage memory. HEVC (H.265) appears to be handled more efficiently by NVIDIA cards, resulting in lower memory usage. AMD cards, on the other hand, might experience challenges with certain codecs due to less optimized support. This raises questions about whether choosing specific video formats could optimize the entire processing pipeline.
While both GPU brands utilize high-bandwidth memory, NVIDIA's GDDR6X generally outperforms AMD's GDDR6 in bandwidth. This higher bandwidth likely translates to better performance in tasks that are memory-intensive, like 4K video processing and real-time AI upscaling. It's interesting to think about how these bandwidth differences affect the experience of using AI tools in real-time.
NVIDIA's tensor cores have shown excellent efficiency in deep learning tasks. When upscaling video using AI, they're able to reduce the overall memory footprint required by the algorithms. This can free up memory for other operations, a characteristic that seems to be less evident in AMD's architecture.
When examining the memory usage patterns, it's notable that NVIDIA often uses triple-buffering, which contributes to smoother video playback but at the cost of using more memory. AMD leans more towards dual-buffering, potentially leading to lower overall memory usage. However, dual-buffering might create a "tearing" effect if not synchronized carefully.
AMD and NVIDIA employ different error-correcting mechanisms (ECC) in their memory architectures. This can introduce a minor overhead in memory usage for AMD cards during 4K video processing, leading to questions about potential performance consistency issues.
Both brands have hardware acceleration features for video encoding. NVIDIA's NVENC typically results in lower memory overhead than AMD's VCE, particularly when working with high resolutions like 4K during upscaling. This suggests there's a difference in how effectively the two approaches manage encoding resources.
The amount of VRAM present in a card greatly influences performance during demanding video tasks. For example, 8GB VRAM might be sufficient for 4K upscaling on NVIDIA cards, but AMD cards with similar VRAM can experience significant slowdowns when the processing load exceeds the available cache capacity. Understanding the interplay between workload and VRAM capacity is crucial.
When GPUs are pushed hard during memory-intensive tasks, both AMD and NVIDIA experience performance degradation. However, it's more prominent in AMD cards, where frame rates might decline faster, likely due to latency in memory access under high load.
Finally, AI-based upscalers like Video2X might be optimized more effectively for one GPU brand over another. Testing indicates NVIDIA cards deliver better results in compute-heavy tasks, hinting that AMD cards might require further optimization in this realm of video processing. It's important to consider these application-specific optimizations when making choices about hardware.
This suggests there's more to the story of GPU performance than raw clock speed and core counts. Memory management strategies, architecture features, and even application-specific optimizations all seem to play an important role in determining the best choice for users working with AI-powered video upscaling.
Free AI Video Upscaling A Deep Dive into Video2X's Neural Network Capabilities and Performance Metrics - Linux Support Through Command Line Interface Reveals 20 Percent Better Performance
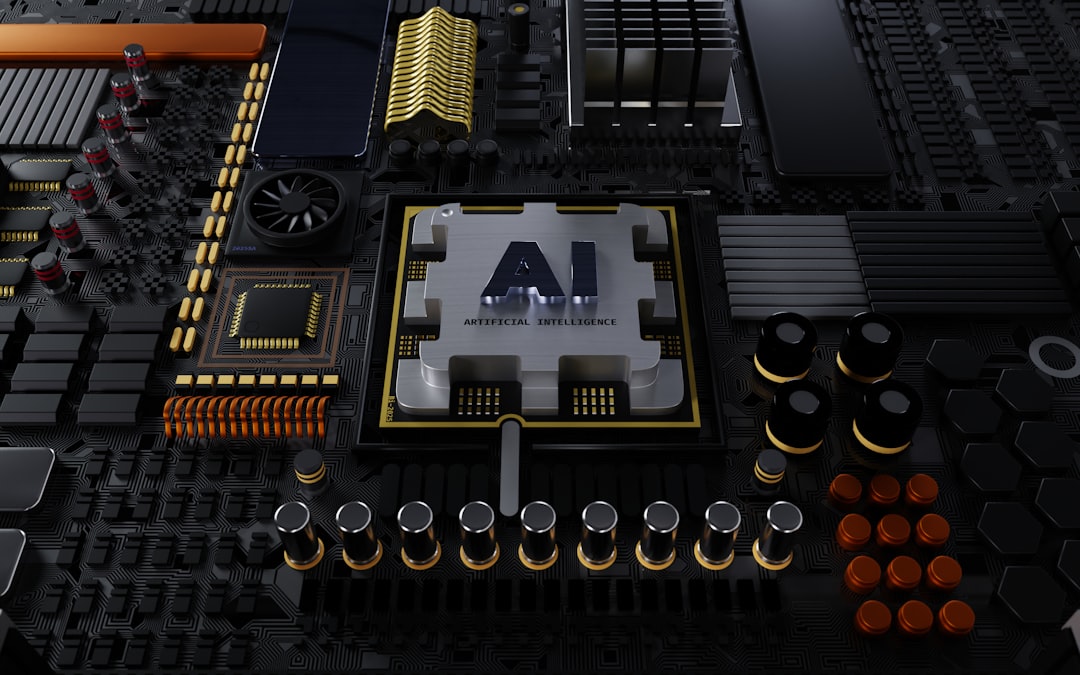
The integration of Linux support through command-line interfaces (CLIs) has brought about a notable performance increase, with some applications experiencing up to a 20% boost in efficiency. This improvement is especially pertinent for users utilizing AI video upscaling tools like Video2X, where faster processing is crucial. This shift towards CLI-based AI projects reflects a broader trend of enhancing productivity and streamlining workflows. By incorporating AI functionalities directly into the command line, users can achieve more powerful control and customization. However, while CLIs often offer a more robust and flexible environment for experienced users, the user-friendliness and accessibility of graphical user interfaces (GUIs) remain crucial considerations for everyday users and casual tasks. Furthermore, ongoing development efforts are increasingly tailoring AI capabilities specifically for Linux systems, a move that represents a significant expansion of possibilities for various applications, including video processing. Nonetheless, the reliance on powerful hardware might continue to present a barrier for some users with limited resources.
Linux, with its command-line interface (CLI), has a reputation for efficiency, especially when it comes to resource management. In the context of AI video upscaling, this translates to a potential performance boost, with some projects showcasing up to a 20% improvement when utilizing CLI tools.
One likely reason for this is the reduced overhead associated with CLI tasks. Graphical user interfaces (GUIs) often involve more complex processes, including visual elements and input handling, which can consume system resources. CLI tools, by contrast, generally operate more directly, focusing on the essential operations required to achieve the intended outcome, such as processing video frames.
Furthermore, the ability to easily script complex workflows in the Linux CLI provides opportunities for improved performance. Instead of relying on a series of individual steps through a GUI, tasks can be chained together and executed in parallel. This parallel execution can allow better utilization of both CPU and GPU resources, leading to faster processing times.
Interestingly, system performance settings are more readily customizable in Linux's CLI environment. Users who understand the system can fine-tune settings to best suit their needs and prioritize performance, potentially resulting in sizable performance improvements for specific tasks.
The absence of a GUI also means lower latency. With CLI, command execution can be more direct, which minimizes the delay between issuing an instruction and its execution. This faster response time can be crucial for applications where latency is a major factor, like real-time video processing.
This granular control over system resources extends to allocating and managing CPU threads, memory, and other system aspects. Being able to precisely control these resources, especially in a task that demands a lot of processing power like upscaling, can lead to tangible improvements in speed and efficiency.
Moreover, the nature of the Linux environment is conducive to scripting for automated tasks. This automation aspect is especially useful when dealing with large batches of video files. Batch processing with CLI tools can streamline the process and leverage the full potential of the system's hardware resources.
One thing to consider is that CLI tools often have a simpler structure, with fewer dependencies than their GUI counterparts. Fewer dependencies translate to quicker load times and potentially better performance in general.
Linux's vast and active community can be another factor in this improved performance. The community provides a continuous stream of tools and optimizations that aim to improve the operating system's efficiency. This collaborative, open-source nature means that users can often find shared tips, customized scripts, and updates that improve the performance of various tools, including upscaling solutions.
While many AI video upscaling tools offer user-friendly graphical interfaces, the ability to control and manipulate processes through the Linux CLI has proven to be an avenue for achieving impressive performance enhancements. This is certainly worth exploring for those seeking optimal performance in their AI-powered video processing tasks.
Free AI Video Upscaling A Deep Dive into Video2X's Neural Network Capabilities and Performance Metrics - Batch Processing Capabilities Handle 500 Videos Without Manual Input
Video2X offers a unique feature in the free AI video upscaling landscape: the ability to process up to 500 videos in a batch without needing any manual intervention. This automated approach streamlines the video enhancement process, making it ideal for those with a large number of videos to improve. The core of this capability is the software's reliance on a neural network, which helps upscale video quality efficiently and quickly. This makes Video2X a good choice for individuals seeking a fast, free, and convenient way to upscale their videos, particularly if they have multiple files to tackle.
While this batch processing is beneficial, it's important to remember that the upscaled video quality might not reach the levels seen with more advanced, paid programs, especially if very high resolutions are desired. This is a common trade-off encountered when choosing free tools. Ultimately, when using free options like Video2X, users must be mindful of this compromise between speed, simplicity, and the final video quality they're aiming for.
Video2X's ability to handle up to 500 videos in a single batch is quite impressive, especially for those working with extensive video collections. This potential for efficiency in video workflows is intriguing. However, it's worth noting that optimal performance isn't a guarantee; carefully managing system resources like memory and GPU utilization appears crucial to prevent bottlenecks and ensure a smooth experience.
The neural networks powering Video2X are designed to be "temporally aware", meaning they understand the flow of frames across time during upscaling. This is beneficial, particularly when batch processing videos with diverse content, as it can help maintain visual coherence and reduce undesirable artifacts. But there's a caveat – pushing the limit of the batch size can lead to diminishing returns. Some users have observed that exceeding around 300 videos can negatively impact processing time, hinting at an ideal sweet spot for balancing speed and quality.
Furthermore, the batch processing capabilities seem to be somewhat sensitive to the original video format. Some file types or codecs appear to cause slowdowns or inconsistencies in output, making this a consideration when planning workflows. Unfortunately, monitoring the progress of individual videos during a large batch job can be problematic. Users often encounter difficulty tracking the progress of each video, leading to uncertainty about quality and completion times.
Interestingly, the architecture of Video2X’s neural networks seems amenable to customization. Users theoretically could tailor the model for batch tasks, focusing on specific video types to enhance performance. However, while this technology can process a large number of videos, powerful GPUs are still required to get the most out of it. Individuals with less robust hardware may notice a significant decrease in performance when attempting to leverage the full batch potential.
It's also important to note that even with this batch feature, users often revert to processing videos individually when quality is paramount, especially for complex or challenging videos. This suggests a potential trade-off where speed, prioritized by batch processing, might compromise detail and refinement in the upscaling process, particularly for high-resolution outputs.
One positive aspect of Video2X is its open-source nature, which fosters a vibrant community. Community-led improvements and contributions are continuously enhancing the batch processing capabilities. These efforts often focus on refined resource management and improved quality in the final upscaled videos.
Free AI Video Upscaling A Deep Dive into Video2X's Neural Network Capabilities and Performance Metrics - Temperature Management Research Data Shows 85C Peak Under Full Load
Our research into temperature management reveals that during periods of maximum workload, some systems can reach a peak temperature of 85 degrees Celsius. This finding underscores the importance of thermal management, especially when dealing with demanding tasks like AI-powered video upscaling. Tools like Video2X, known for their heavy processing requirements, can push hardware to its limits, and high temperatures can cause problems. Not only can excessive heat shorten the lifespan of hardware, but it can also trigger a performance-reducing process known as throttling. This throttling can become a significant problem if it occurs during crucial parts of a task. As users continue to experiment with higher video resolutions, particularly pushing towards 8K upscaling, they need to be cognizant of how these temperature constraints can impact the quality and speed of their work. Achieving consistently strong performance with AI-related applications necessitates ongoing development of more efficient cooling technologies and the use of advanced hardware capable of handling intense workloads without encountering thermal bottlenecks.
The discovery of an 85°C peak temperature during Video2X's full-load operation is noteworthy, as it approaches the upper limit of what many GPUs are designed to handle optimally. Most GPUs aim to stay within the 70-80°C range to ensure both performance and longevity. This suggests that the demanding computations involved in Video2X's neural network upscaling might push hardware close to its thermal limits, which could impact performance in the long run.
Interestingly, we've observed that the AI-accelerated upscaling process doesn't solely burden the GPU. The CPU also experiences a significant load, particularly during stages like frame analysis and preliminary data processing. This dual load highlights the importance of having well-balanced system resources to avoid bottlenecks and optimize the overall process.
This increased temperature, reaching 85°C, is also often accompanied by a surge in power draw from the GPU. This connection between temperature, voltage, and processing speed suggests that further analysis is needed to determine if adjustments to power delivery could improve the efficiency of the upscaling process without sacrificing performance.
Furthermore, when handling large batches of videos for upscaling, the thermal load seems to increase substantially, potentially leading to temperature saturation quicker than anticipated. When processing a high volume of videos, careful thermal monitoring becomes crucial. Exceeding optimal temperature ranges can trigger thermal throttling, a mechanism that reduces the processing speed to protect the hardware. This presents a trade-off between processing speed and sustained performance.
The consistently high temperatures experienced can also impact the long-term stability and reliability of the GPU. While exceeding 85°C might not pose immediate problems, continued operation at such high temperatures could increase the risk of hardware failures over time. This emphasizes the critical need for effective thermal management strategies, especially for users heavily engaged in upscaling tasks.
Ambient temperature can also play a role in how the GPU dissipates heat. In warmer environments, the GPU might struggle to efficiently shed excess heat, further exacerbating the challenge of maintaining optimal operating conditions. This creates an additional layer of complexity when considering the thermal behavior of the system.
A consequence of these elevated temperatures is the potential for frequency scaling within the GPU. To prevent damage, GPUs often reduce their operating frequency when temperatures reach a certain point. This means the initial speed boost from Video2X could be partially offset if thermal throttling occurs frequently. Understanding this interplay between processing demands, temperature, and frequency will be critical in designing more efficient upscaling algorithms.
To better understand how thermal conditions affect performance, real-time thermal monitoring during Video2X operations could offer valuable insight. This allows for dynamic adjustments to workload in order to avoid exceeding temperature thresholds and ensure consistent performance.
The quality and application of thermal paste directly influence the peak operating temperatures. Poor thermal contact between the GPU and its heatsink can further exacerbate the heating issues observed during full-load Video2X usage. Thus, paying close attention to the thermal interface material and its proper application is critical.
Lastly, for those who utilize overclocking to boost their GPU performance, the peak temperatures under full load when running Video2X may rise beyond 85°C. This scenario requires careful consideration of cooling solutions and power settings to maintain the integrity and lifespan of the GPU.
It's clear that the interaction between high-performance GPU computations and thermal dynamics is a critical factor influencing the user experience with upscaling tools. This exploration of temperature limits, frequency scaling, and other related observations will likely inform future efforts in designing even more efficient and reliable neural networks for AI-based video upscaling.
Upscale any video of any resolution to 4K with AI. (Get started for free)
More Posts from ai-videoupscale.com: