How AI Upscaling Improves Public Domain Films Analysis of Internet Archive's HD Movie Collection
How AI Upscaling Improves Public Domain Films Analysis of Internet Archive's HD Movie Collection - How AI Processing Doubled Resolution of 1920s Silent Films at Internet Archive
The Internet Archive has employed AI to significantly improve the visual fidelity of silent films from the 1920s, effectively doubling their resolution. This innovative approach not only enhances the clarity and quality of these historical films but also expands their accessibility to contemporary audiences who might find the original formats less engaging. By applying AI techniques, the archive has been able to bring these films into a sharper focus, making the viewing experience richer and more enjoyable. One example of this is "A Day in 1920’s Paris," which has undergone a remarkable transformation through AI, now showcasing a higher frame rate and enhanced resolution. This initiative demonstrates how AI can be used in a positive manner to preserve film history and extend the reach of these cultural artifacts. The increased viewership of these AI-enhanced films on platforms like YouTube is a strong indication of their greater appeal to modern viewers, highlighting the potential of AI for revitalizing cinematic history. While some worry about AI's potential misuse, this project serves as a compelling demonstration of AI's capacity to restore and cherish our cinematic past.
The Internet Archive has successfully employed AI to double the resolution of a selection of silent films from the 1920s. These films, originally limited to 480p or lower quality, have been upscaled to 1080p, leading to a noticeable improvement in visual detail and sharpness. The process essentially involves AI algorithms analyzing the existing frames and using deep learning techniques to infer and fill in missing pixel data, based on the textures and patterns within the source material. This is akin to how our own eyes interpret and piece together visual information.
However, the quality of the upscaling is highly dependent on the original film's condition. Films that were well-preserved initially benefit from this treatment more than those that are already damaged or degraded. Aside from resolution, the AI algorithms also focus on mitigating artifacts like noise and blur, further refining the viewing experience for these older films.
These AI models are trained on enormous datasets of high-quality video. This allows them to learn the relationships between different levels of resolution and generate realistic, high-resolution outputs from lower-resolution inputs. It's fascinating that the earliest efforts at AI-driven film enhancement sometimes generated results some critics found artificial. This sparked debates about finding a balance between restoration accuracy and enhancing the viewing experience.
The upscaling process raises concerns about the authenticity of film restoration. Some believe AI manipulation can compromise the film's historical integrity, while others argue that this is a necessary step to make classic films accessible to modern viewers. One approach frequently used involves temporal interpolation, where the AI predicts intermediate frames. This results in smoother motion in the final output, potentially making these silent films more engaging.
In some instances, upscaling has unveiled hidden details previously unnoticed, such as fine text displayed onscreen or characters' expressions. These newly-revealed details can provide deeper insight into the original filmmaker's artistic intentions. As computational resources and AI algorithms continue to evolve, we can expect further enhancements to these techniques, leading to even more remarkable visual improvements. This could completely transform how we appreciate and understand films from the silent era.
How AI Upscaling Improves Public Domain Films Analysis of Internet Archive's HD Movie Collection - Frame Rate Interpolation Techniques Used on Public Domain Charlie Chaplin Movies
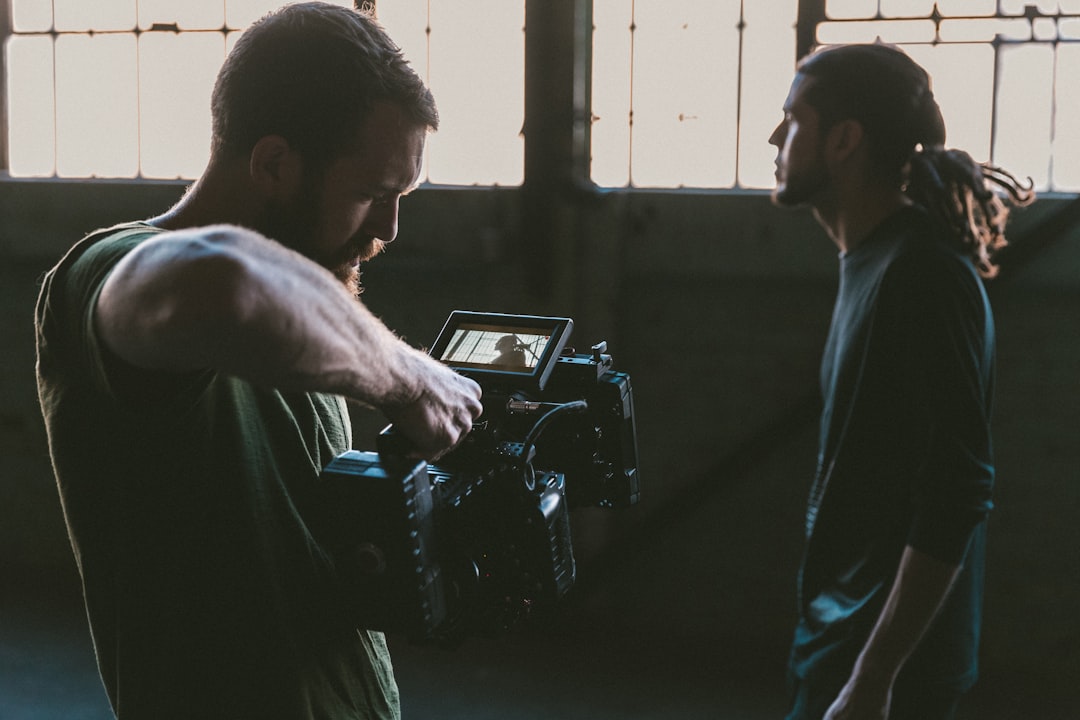
Charlie Chaplin's silent films, a cornerstone of the public domain, were often captured at a low frame rate, a limitation of the early filmmaking technology. This can lead to a jerky, less fluid viewing experience for modern audiences accustomed to smoother motion. Frame rate interpolation addresses this issue by utilizing algorithms to generate artificial frames between the original frames. This process effectively increases the film's frame rate, resulting in more natural-looking motion. This has the potential to revitalize these silent film classics and make them more accessible to a wider audience.
Interpolation techniques, specifically those focused on motion analysis, allow for smoother transitions and movements within the film, making the overall viewing experience more enjoyable. While some might question whether altering a film's original frame rate compromises its authenticity, this process can be viewed as a means of preservation, making it easier for contemporary viewers to engage with the historical and artistic value of these cinematic gems. The combination of frame interpolation and AI upscaling can truly enhance the viewing experience of these classic works, bringing them closer to the visual standards of modern film production while also respecting the spirit of their origins. As the field of AI video processing develops, we can likely expect even more sophisticated frame rate interpolation techniques that further enhance the accessibility and appeal of Chaplin's films for generations to come.
Charlie Chaplin's films, a cornerstone of the public domain, are prime candidates for modern enhancement techniques, including frame rate interpolation. These early silent films, often captured at 16 to 20 frames per second due to the limitations of the time, can benefit greatly from these techniques. Frame rate interpolation essentially adds new frames between the original frames, creating a smoother, more fluid appearance of motion. This can significantly improve the viewing experience, especially for films accustomed to lower frame rates.
While simple techniques initially relied on basic interpolation, modern approaches leverage complex algorithms, including deep learning, to generate these new frames. These algorithms analyze the changes between frames, effectively predicting the motion and creating intermediate frames. The goal is often to achieve a final frame rate exceeding 60 frames per second, a substantial leap from the original. The increased fluidity can be especially important for silent films, where much of the storytelling relies on physical comedy and subtle nuances of movement.
However, this process isn't without its drawbacks. Critics have pointed out the so-called "soap opera effect," a perceived unnatural smoothness that some argue can disrupt the authentic aesthetic and pacing of older films. The challenge lies in achieving a balance between modern standards of motion and preserving the original cinematic intent.
On the other hand, research in motion perception suggests that smoother motion can lead to increased viewer engagement and potentially help retain the attention of contemporary audiences who may be less accustomed to the pacing of classic films. Unfortunately, the effectiveness of interpolation also depends on the original film's quality. Damaged or fragmented footage can introduce artifacts that counteract the intended improvements.
Interestingly, in some cases, frame rate interpolation has uncovered hidden comedic timing in Chaplin's work. The enhanced fluidity showcases his physical comedy and visual gags more effectively. However, this also raises questions about the integrity of the original film. The filmmaker's artistic intent, reflected in the original pace and style, could be obscured by the smoothing effects of the technology. It's a balancing act: some viewers prioritize increased visual fidelity, while others champion the preservation of the historical film's original pacing.
With ongoing advancements in computational power, future interpolation techniques may offer real-time adjustments. Viewers could potentially choose the frame rate they prefer, adding an interactive layer to film viewing. This could revolutionize how we experience and understand films from the silent era, particularly the works of innovative filmmakers like Charlie Chaplin, who helped shape cinema's evolution.
How AI Upscaling Improves Public Domain Films Analysis of Internet Archive's HD Movie Collection - Machine Learning Models Behind Internet Archive's HD Film Enhancement Project
The Internet Archive's HD Film Enhancement Project utilizes machine learning to improve the quality of public domain films. This initiative uses advanced AI techniques, including deep learning, to upscale the resolution of these older movies. These models essentially "fill in the gaps" in the original footage, creating a higher-resolution image based on patterns and textures. This process, often referred to as super-resolution, helps to sharpen and clarify blurry areas that are common in older film prints.
Furthermore, the models can adjust the frame rate, creating smoother motion that is more appealing to modern viewers accustomed to higher frame rates. This frame rate interpolation, while improving the viewing experience, can also be a point of contention, with some expressing concern about altering the original film's aesthetic. The process aims to strike a balance between improving the quality of the films for modern audiences and preserving the original intent and history of these cinematic works. As AI technology continues to evolve, we can anticipate even more sophisticated methods for enhancing the visual quality of older films, potentially changing how we engage with and understand film history.
The Internet Archive's HD Film Enhancement Project relies on machine learning models that are typically trained on a wide variety of film footage, spanning both modern high-definition content and historical films. This training allows the AI to learn subtle differences in texture and detail, which is essential for accurately restoring older film footage. The AI learns to discern intricate details and apply them in a way that complements the original source.
A technique called temporal interpolation, used in this project, involves algorithms that estimate motion and predict intermediate frames. This process not only boosts the perceived frame rate but also smooths out movement, potentially creating opportunities for a more engaging viewing experience in films where physical expression is key, like silent films.
The success of AI-powered upscaling hinges heavily on the original film's condition. Films that are damaged or poorly preserved often present a greater challenge, as they might introduce visual artifacts that make the upscaling less effective. It highlights the complexities involved in restoring films that have deteriorated over time.
Upscaling algorithms often employ convolutional neural networks (CNNs), which aim to replicate human visual perception. This approach helps the AI intelligently fill in gaps in pixel information by recognizing patterns and textures, resulting in a more authentic restoration. The algorithms mimic how we visually perceive and process visual cues from our environment.
Some early attempts at AI-driven upscaling were criticized for generating unnaturally enhanced results. This led engineers to refine the algorithms significantly, illustrating both the initial challenges and the rapid advancements made in machine learning within the field of film restoration. It speaks to the evolution of this technology and the constant refinement needed to achieve desired outcomes.
One notable aspect of the enhancement process is the ability to reveal hidden details—subtle expressions or visual jokes—that might have been obscured in the original versions. This increased clarity helps viewers better understand the filmmaker's intentions and the historical context in which the film was made. It sheds light on how we can learn more about how films were created in different eras.
Frame rate interpolation can occasionally inadvertently alter the pacing of a film's comedic timing, especially in the work of masters of silent film like Chaplin. This brings up interesting questions about the trade-off between using modern technology and preserving the historical integrity of the film. Where do we draw the line between enhancing the experience and potentially compromising authenticity?
The AI models used in this project are frequently enhanced through reinforcement learning, which allows them to continuously improve as they process more film footage. The models become better at image enhancement as they gain exposure to a wider array of film styles and types. It's fascinating how these models adapt and refine their output as they encounter more data.
Counterintuitively, some argue that AI-enhanced films may create a sort of perceptual gap between generations. Viewers raised on modern filmmaking might expect a certain level of visual perfection and be less attuned to the unique aesthetic qualities of older films. The advancement of technology can create differing expectations in viewers and perhaps disconnect them from older forms of media.
The potential for future enhancements in this automated upscaling process is vast, possibly including the ability to adjust frame rates and enhancement levels in real-time. This could lead to a customizable film viewing experience where each viewer can tailor the way they watch films, a revolutionary concept previously not possible. We may see a future where we have even more personalized methods of watching movies and experiencing older films.
How AI Upscaling Improves Public Domain Films Analysis of Internet Archive's HD Movie Collection - Hardware Requirements and Processing Time for Large Scale Film Upscaling
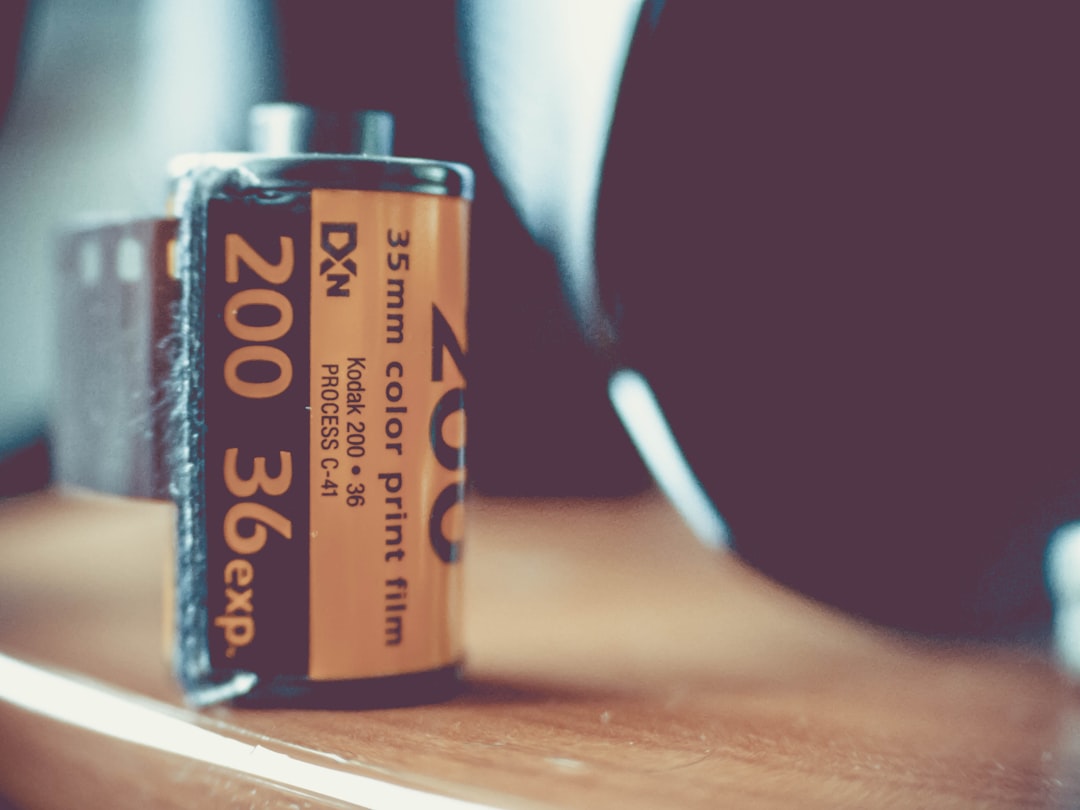
When examining the practical aspects of AI-driven film upscaling on a large scale, it becomes clear that the hardware and processing demands are substantial. AI upscaling relies on complex algorithms that require significant processing power. While basic hardware might be sufficient for small projects, upscaling large volumes of film, especially at higher resolutions and frame rates, can overwhelm less capable systems. This often results in long processing times, hindering efficiency and making the task less practical.
Conversely, systems equipped with advanced hardware, particularly those incorporating powerful graphics processing units (GPUs), are well-suited for these tasks. These specialized processors can drastically shorten processing time and even enable real-time upscaling, allowing for smoother adjustments and easier integration into workflow. However, the cost of maintaining high-performance hardware can be a significant obstacle for many individuals and organizations seeking to engage in film restoration projects. This raises the question of accessibility and equity within the realm of AI-driven film preservation.
While AI has undoubtedly advanced our ability to restore and enhance films, the hardware needs and processing times remain a crucial aspect to consider when undertaking large-scale projects. This highlights the continuous tension between the innovative capabilities of the technology and the practical constraints of implementation.
AI-powered film upscaling, while promising, presents significant hardware challenges, especially when dealing with large-scale projects. For instance, efficiently handling the complex algorithms often necessitates GPUs equipped with thousands of CUDA cores—a level of processing power typically found in high-end gaming computers. The sheer computational load can make processing times quite lengthy, potentially requiring several hours for a single feature film. Upscaling a full-length film involves intricate deep learning computations, significantly impacting overall processing time.
The need for high-speed data transfer during upscaling has led to the incorporation of high-bandwidth memory (HBM) in some systems. This type of memory can significantly speed up the processing of large video files, which are common with high-definition film projects. However, even with powerful hardware, upscaling isn't always a magic bullet. If the source film is significantly damaged or contains lots of visual imperfections, the AI's ability to enhance the quality can be limited, sometimes resulting in outputs that are worse than the original.
Beyond processing power, storage also becomes a major concern. Upscaling a typical 1080p film from a lower resolution could easily require ten times the original disk space. This increased storage demand adds another layer of complexity to these projects, especially when dealing with large film archives.
When considering real-time upscaling, the hardware demands rise even further. To maintain a smooth, fluid viewing experience, processing speeds need to consistently exceed 60 frames per second—a feat that's currently impractical for most consumer-grade hardware.
Interestingly, AI upscaling methods often incorporate techniques like batch processing to improve efficiency. Instead of processing frames one by one, they process multiple frames simultaneously, which can significantly reduce the overall time required for a project.
While basic consumer-grade graphics cards can handle upscaling tasks, high-performance, research-grade GPUs offer a significant speed advantage. In some cases, these research-focused systems can complete film projects five to ten times faster than their entry-level counterparts. This difference in speed highlights the need for specialized hardware when tackling large-scale upscaling endeavors.
Distributed computing, where multiple machines work together, is another approach that can significantly reduce processing time. This technique allows for quicker completion of shorter film projects, potentially reducing processing from hours or days to mere minutes.
Looking towards the future, the development of quantum computing holds the potential to revolutionize film upscaling. Theoretically, quantum computing could significantly reduce processing times, enabling real-time analysis of resolution enhancement in a way that's currently impossible. This area represents a frontier in AI-based film restoration and promises to reshape our ability to interact with and enhance these historical artifacts.
How AI Upscaling Improves Public Domain Films Analysis of Internet Archive's HD Movie Collection - Quality Comparison Between AI Enhanced and Original Public Domain Films
Examining the differences between AI-enhanced and the original versions of public domain films reveals a complex relationship between technological advancements and the preservation of artistic integrity. AI upscaling can dramatically improve the visual quality, frequently increasing the resolution of older films by a factor of four, making hidden details more apparent. Yet, some argue that these enhancements, while creating a more captivating viewing experience, can inadvertently diminish the film's original artistic expression and character. This prompts a persistent discussion on how to best balance the advantages of AI-driven restoration with the importance of respecting the authenticity of historical films. As AI continues to develop, it presents thought-provoking questions on how we appreciate and engage with cinema's history in our current digital age, raising questions about the trade-offs between modernization and preserving a film's original spirit.
When evaluating the quality differences between AI-enhanced and original public domain films, several key factors emerge. The success of AI upscaling is strongly tied to the original film's condition. Pristine films respond well, revealing sharper details, while those with existing damage or degradation might not see as dramatic an improvement. Sometimes, the upscaling process can unearth hidden details, like subtle expressions or text, which might subtly change how we interpret the film's story and the filmmaker's intent.
Beyond resolution, AI algorithms also work to reduce noise and blur that are common in older films. This leads to a cleaner and often more enjoyable viewing experience, though it does bring up questions about altering the original film's character. Critics frequently debate whether AI-enhanced films lose some of their original feel, while others see this type of restoration as necessary for making these classics more accessible to modern audiences.
It's also interesting that even when applied to the same film, different machine learning models can yield distinct upscaled results. The variation comes from the training data used and the specific choices made by the engineers working on the model. This means that we can potentially get quite different interpretations of the same film simply by altering the AI's training or the underlying algorithms it uses.
One logistical hurdle is that upscaling can significantly increase a film's file size, sometimes up to ten times the original size. This can be a challenge for organizations managing large collections of films, as they need more storage capacity.
When we look at real-time processing, upscaling films at a high frame rate while also maintaining a smooth viewing experience remains a significant technical challenge. Current consumer-grade computing hardware isn't always up to the task. And while the technology is fascinating, it potentially creates a generational gap in how viewers perceive older movies. People accustomed to modern film quality might not readily connect with the stylistic elements of films from a different era.
However, AI engineers have discovered that using batch processing, which works on many frames simultaneously, can substantially speed up upscaling compared to processing them one at a time. This makes AI upscaling more efficient for large projects.
The field is constantly evolving. In the future, it's conceivable that quantum computing could dramatically accelerate upscaling processes. If this were to become a reality, we could see near real-time improvements and enhancements that are impossible with our current computer technology. This would change the way we interact with and explore our cinematic history, allowing for even more profound insights into these historical treasures.
How AI Upscaling Improves Public Domain Films Analysis of Internet Archive's HD Movie Collection - File Format Changes and Storage Impact of HD Versions in Internet Archive
The Internet Archive is continually refining its approach to digital media, particularly with regards to its growing collection of high-definition films. This involves adjustments to file formats and storage practices to enhance user experience and accessibility. For example, the archive commonly converts audio files from FLAC to MP3 and video files from large MKV containers to more easily streamable MP4 formats. This ongoing shift in how files are handled aims to expand access to its vast collection, particularly for online users.
Beyond simple conversions, the archive implements significant changes to movie files through deinterlacing and upscaling, often using AI to improve resolution to 1920x1080. While beneficial to viewers, these HD enhancements can considerably increase storage requirements. In some cases, upscaled files may consume ten times the storage space of the original version, significantly impacting the archive's overall storage needs. Further, specialized file formats like DCP and MXF are employed for certain movie content to ensure optimal preservation and compatibility with various platforms.
This drive towards enhanced digital media experiences for users creates a balancing act. The Internet Archive must navigate the growing need for higher-quality, accessible content against the practical challenges of managing exponentially increasing storage demands. These shifts in file formats and storage demonstrate the evolving nature of digital preservation and the complexities that arise as new technologies and expectations reshape access to historical film collections.
The Internet Archive's conversion of older films to newer formats, especially the shift towards HD versions, often leads to a significant increase in file size, sometimes as much as ten times the original. This presents a noticeable challenge for storage and archival management, demanding more robust data management systems and increased computational resources to handle the sheer volume of data.
Upscaling to HD resolution (1920x1080), while enhancing the viewing experience, can impose a substantial strain on storage infrastructure. To accommodate these larger file sizes, archival organizations are carefully reconsidering their storage architectures. This may involve transitioning from older hard drive systems to high-capacity array systems designed for the increased data demands.
To mitigate the storage impact, advanced video compression techniques have become vital. Utilizing newer codecs, like H.265, in place of older ones like H.264, can maintain a comparable level of visual quality while using a lower bit rate, thus helping to address the storage issues associated with HD upscaling.
However, the transition to HD doesn't just involve the video data. Enhanced HD versions usually require comprehensive metadata to maintain quality and facilitate smoother transitions across different formats and playback devices. This comprehensive metadata, while aiding in content retrieval, also increases storage demands and introduces complexities for database management systems.
Furthermore, the change in file formats might introduce incompatibility issues with older systems, which may require emulators or converters to maintain access to both original and upgraded versions of films. This creates an extra layer of complexity for users and archivists who need access to the original and modified files.
During the conversion process, there's always the risk of quality degradation if the process isn't precise. This leads to questions about the accuracy of archival processes and whether the core aesthetic elements of the original film are adequately preserved.
The availability of HD versions also necessitates a wider understanding of how films perform on different platforms, from mobile devices to high-definition displays. Each platform may process upscaled content differently, potentially impacting the viewing experience and even discouraging audience engagement.
The time needed for the upscaling process can increase substantially with the higher resolution and larger datasets. While more data improves the AI's ability to upscale, it also means longer processing times, potentially hindering the efficiency of archival workflows.
In the long run, the constantly evolving nature of film formats and viewing technologies highlights the importance of future-proofing digital archives. Organizations are increasingly motivated to adopt adaptable storage solutions that can accommodate future advancements in file formats and storage technologies.
As we move forward, the challenges associated with managing these growing HD archives emphasize the constant balancing act between enhancing content for modern viewers and preserving the integrity of these historical records. This involves ongoing refinement of storage infrastructure, data management practices, and a critical understanding of the evolving digital landscape for film preservation.
More Posts from ai-videoupscale.com: