How AI Video Upscaling Enhances MP4 Downloads A 2024 Perspective
How AI Video Upscaling Enhances MP4 Downloads A 2024 Perspective - AI-powered resolution enhancement for MP4 files in 2024
The ability of AI to enhance the resolution of MP4 files has made substantial strides in 2024, offering users more ways to improve their video quality. This technology can transform standard-definition videos into high-definition or even 4K formats, effectively increasing the detail and clarity while reducing compression artifacts. A variety of tools leverage advanced AI algorithms to overcome challenges like noise reduction, blur removal, and stabilizing shaky footage. While the results are often impressive, achieving optimal speeds and consistently flawless upscaling across all types of videos remains a challenge. Some tools, especially when working with extremely low-quality source material, can produce unwanted distortions or artifacts. As the field progresses, users need to carefully consider the strengths and limitations of the available software to make informed choices for their needs.
In the realm of MP4 file enhancement, AI has made strides in 2024, pushing the boundaries of resolution upscaling. We're seeing algorithms capable of generating 8K output from lower resolution sources, a feat achieved through intricate convolutional neural networks that intelligently fill in missing details. A significant development is the emergence of temporal upscaling. By incorporating information across multiple frames, AI tackles a longstanding hurdle of traditional methods – achieving smoother motion, particularly during fast-paced scenes.
Furthermore, 2024's upscaling algorithms are better at analyzing the finer nuances of video, such as color and texture patterns, which leads to a substantial reduction in the visual artifacts that were more common in earlier iterations. This refined analysis also allows these models to distinguish between different types of scenes, enabling adaptive enhancement for things like landscapes or close-ups. This targeted approach often produces a more natural, less processed final product.
Perhaps most intriguingly, the efficiency of these AI methods has increased. Real-time upscaling is now a reality, making AI upscaling a feasible option for live events or streaming, where instant quality improvements are desirable. This improvement in speed is partly due to more efficient AI models, requiring less computational horsepower. This trend has the potential to democratize video editing and content creation, making sophisticated tools accessible to a wider range of users.
The ability to recognize specific elements within a video is also a noteworthy advance. Modern AI models can pinpoint focal points within a scene and concentrate enhancement there, allowing the preservation of key details while simultaneously minimizing noise in less important areas. This targeted approach helps maintain visual fidelity where it matters most.
However, the seamless integration of AI upscaling with established video editing suites is also a significant trend of 2024. This means that the process of applying resolution enhancement is becoming a standard part of the editing workflow, further streamlining content creation.
Despite these encouraging developments, challenges persist. The varying detail levels present in different segments of a video can lead to inconsistencies, potentially resulting in an uneven viewing experience if not carefully managed. As viewers demand more from video content, a trend toward more customization in upscaling settings is emerging. Future algorithms might incorporate more user-controlled settings, allowing a greater degree of finetuning by both engineers and content creators.
How AI Video Upscaling Enhances MP4 Downloads A 2024 Perspective - Neural networks revolutionize video quality improvement
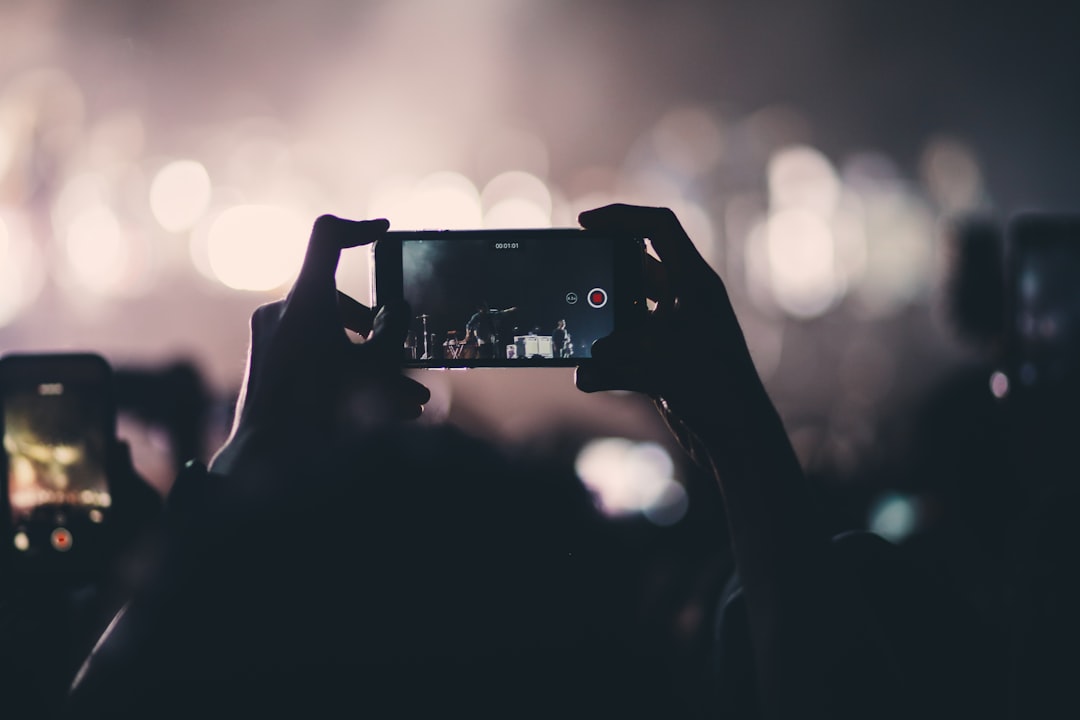
Neural networks are revolutionizing how we enhance video quality, particularly in 2024. These networks utilize sophisticated algorithms, including GANs, to intelligently analyze and improve video content. They can effectively increase resolution, decrease compression artifacts, and even tackle blurriness in a way that was previously impossible. A notable advance is temporal upscaling, where the algorithms analyze multiple frames to create smoother motion, especially in fast-moving scenes, a persistent challenge for older methods. While the potential for these advancements is significant, there are still limitations to be addressed. Certain types of low-quality source material can lead to unwanted artifacts during the upscaling process, and users should be aware of these potential issues. As these networks continue to evolve, their impact on video enhancement, particularly for formats like MP4, will likely be profound, setting new standards for visual fidelity.
The core of these advancements in video quality improvement lies in the application of neural networks. These networks, particularly in the context of "super-resolution," don't just stretch pixels to increase resolution. Instead, they predict the details that should exist in between those pixels, resulting in a more natural and detailed upscaled image. Interestingly, the design of some upscaling algorithms is loosely inspired by the human visual system, leading to outputs that appear more visually appealing and natural.
Research suggests that these neural network-based approaches can outperform traditional methods, offering a substantial improvement in image quality. Beyond simply enhancing still frames, some algorithms can even simulate motion blur based on object movements in a video sequence, making fast-paced scenes feel more authentic. One exciting development is the use of Generative Adversarial Networks (GANs). These networks essentially pit two neural networks against each other—one generates high-resolution frames, and the other critiques them for realism. This competition leads to remarkably believable outputs, almost as if they were captured in high detail from the start.
A key benefit of these modern neural networks is their ability to differentiate between background noise and important image features. This targeted approach means that upscaling can eliminate compression artifacts and pixelation more efficiently, leading to cleaner and more professional-looking videos. Furthermore, some cutting-edge tools can analyze video content in real-time, adapting their upscaling parameters dynamically depending on the scene. For example, adjustments are made to handle rapid background changes differently than static portraits.
The training process behind these sophisticated models is quite intensive, requiring massive datasets of high-quality image pairs. Through this process, the networks learn to recognize patterns and distinguish between low and high-resolution versions of images, continually refining their upscaling techniques. During the upscaling itself, they can recognize and remove certain compression artifacts by identifying them as deviations from learned patterns, contributing to a more polished final output.
Looking ahead, we're seeing a growing trend towards algorithms that can adapt to individual user preferences. This means the upscaling process could become more personalized, allowing engineers and content creators to fine-tune the results to their specific needs and styles over time. As these algorithms evolve, they may learn from our edits and choices, leading to more intuitive and tailored video enhancements in the future.
How AI Video Upscaling Enhances MP4 Downloads A 2024 Perspective - Addressing compression artifacts in downloaded MP4s
Downloaded MP4s often suffer from compression artifacts, especially when the source file or the download process involves significant data reduction. This can manifest as blurriness, pixelation, and other visual imperfections, which can be particularly noticeable on larger screens or when viewed in high resolutions. The increasing demand for higher video quality in 2024, fueled by advances in streaming and display technologies, has brought the issue of compression artifacts into sharper focus. Thankfully, artificial intelligence is offering promising solutions. AI algorithms, particularly those utilizing neural networks, can now analyze video content and intelligently identify and reduce these visual imperfections. These methods are not just about stretching pixels to increase resolution; they're capable of predicting missing details and improving clarity in a way that appears more natural. While significant progress has been made, it's important to note that applying these techniques to heavily compressed or low-quality source material can still produce undesirable results. Despite these potential limitations, the ongoing evolution of AI upscaling technologies highlights a growing desire for improved video fidelity, pushing the boundaries of what we can expect from digital media in the future.
Downloaded MP4s often suffer from compression artifacts, a consequence of lossy video codecs that discard data to minimize file size. These artifacts manifest as visible distortions, particularly noticeable in action sequences where motion blur can be misrepresented, negatively affecting the overall quality. Surprisingly, some compression techniques introduce blockiness, a visual artifact caused by inconsistent treatment of uniform color areas. This issue is more pronounced in videos compressed at lower bitrates, where the reduced data leads to more prominent blockiness.
AI-powered temporal upscaling methods, however, offer a potential solution. By analyzing the consistency of frames over time, they can help mitigate flickering or jumping artifacts that often appear due to uneven frame quality during compression. This is a fascinating aspect of AI video enhancement, addressing a common issue in downloaded videos. An interesting challenge arises because some compression artifacts can be mistaken by our eyes for natural film grain. This makes it difficult for upscaling algorithms to differentiate between unwanted imperfections and potentially beneficial artistic effects that could improve the viewing experience.
The phenomenon of "banding" – visible lines or stripes within gradients – is another consequence of compression. AI-based algorithms can counteract banding by using techniques like dithering to simulate smoother transitions in upscaled versions of these gradients. While promising, we must recognize that enhancement methods can, paradoxically, introduce new artifacts. Overshooting or haloing around edges are potential examples of unintended consequences. This emphasizes the importance of a balanced approach to enhance video quality without creating further distortions.
The effectiveness of AI in restoring video quality is heavily dependent on the quality of the input video. Research suggests that severely compressed videos respond less well to upscaling compared to those with minor artifacts. This implies a threshold effect, where extremely poor-quality source material limits the potential benefits of upscaling. Interestingly, some machine learning approaches to video enhancement use "inpainting" — a technique where the algorithm predicts and fills in damaged or artifact-ridden areas. This can be effective for restoring more complex videos with numerous compression-related issues.
The specific codec used for a video file can also influence the types of compression artifacts that appear. Certain codecs manage motion and static content differently, leading to codec-specific visual artifacts that can remain even after upscaling. This underscores the importance of considering codec properties during the enhancement process. Lastly, adaptive upscaling algorithms are increasingly prevalent. These tools can intelligently adjust enhancement levels based on the complexity of each scene, dynamically deciding when to emphasize detail in key subjects or suppress artifacts in less critical areas. This represents a significant potential for future progress in video quality enhancement, creating a more nuanced and tailored video experience.
How AI Video Upscaling Enhances MP4 Downloads A 2024 Perspective - Real-time upscaling capabilities for streaming platforms
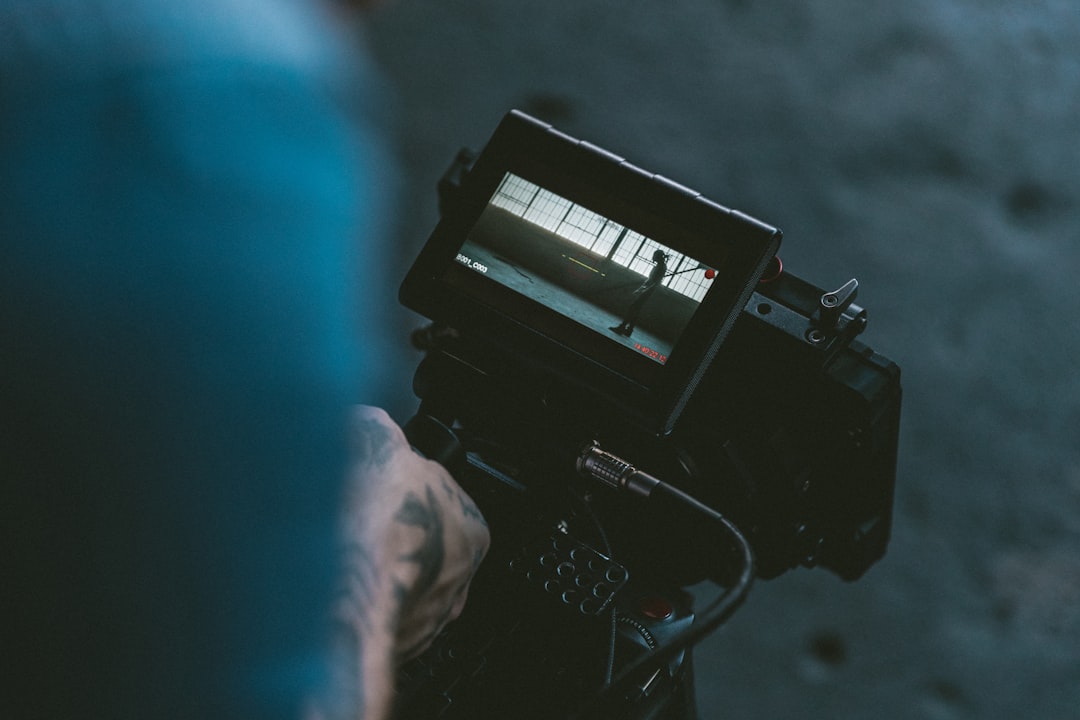
Real-time upscaling capabilities are transforming the streaming landscape in 2024, enabling immediate resolution enhancement during live events. AI algorithms and neural networks are powering this shift, allowing platforms to improve video quality on the fly without excessive processing power. Viewers benefit from smoother motion and enhanced clarity, particularly noticeable in fast-paced content like sports or gaming streams. While promising, maintaining consistent high quality across diverse video sources remains a challenge. Developers are striving to balance resolution improvement with a natural visual appearance, aiming to preserve the original aesthetic rather than creating an overly processed look. As this field matures, we can anticipate further advancements in real-time upscaling, potentially revolutionizing how we consume streamed video content.
The rise of high-resolution displays and the growing demand for high-quality streaming experiences have spurred the development of real-time upscaling capabilities for streaming platforms. We're seeing a shift towards on-the-fly resolution enhancement, where videos are upscaled in real-time as they're being streamed. This is a significant change from the traditional approach of pre-processing videos before they're uploaded.
One of the key drivers of this shift is the increasing efficiency of AI algorithms. Modern upscalers are now computationally lightweight enough to run on a variety of devices, including smartphones and laptops, which is a welcome development that widens access to high-quality video processing. No longer do we need high-end servers for such tasks.
Many modern upscaling methods employ dynamic adjustments based on the specific content being streamed. These adaptive approaches analyze each scene and selectively enhance critical elements, like details in a face or a complex landscape. This not only ensures a visually more engaging experience but also intelligently manages processing resources.
Traditionally, video upscaling often struggled with motion, leading to jittery and blurry sequences, particularly in fast-paced action scenes. However, some real-time upscalers are addressing this by utilizing multiple frames to better predict and synthesize smooth motion. This is a welcome change that significantly improves the overall viewer experience.
Another interesting development is the ability to identify and minimize compression artifacts in streaming video. These algorithms can recognize different types of distortions and effectively minimize their impact, resulting in cleaner and more refined videos.
The training processes behind these AI upscalers are becoming more sophisticated, utilizing massive datasets of video pairs to train these algorithms. This allows for improved accuracy and reduced latency in real-time applications, which is critical for a seamless streaming experience.
Many algorithms incorporate edge-preserving techniques to ensure that sharp lines and fine details are maintained throughout the upscaling process. This is important for ensuring that the overall quality and fidelity of the video are preserved during the enhancement.
We're also seeing the emergence of upscaling algorithms that incorporate real-time color and texture analysis, allowing for more nuanced and accurate enhancements. By analyzing color distributions and textures, the upscaler can fine-tune the process, leading to visually more appealing results.
Generative Adversarial Networks (GANs), initially known for creating incredibly realistic images, are increasingly finding applications in real-time video upscaling. These networks are trained on a blend of real and synthetic data to improve their ability to generate highly realistic and enhanced video, which pushes the boundaries of visual fidelity in streaming video.
Interestingly, some upscaling solutions are now starting to incorporate user-defined quality control. This is a significant step towards personalization in streaming, potentially allowing users to adjust the degree of enhancement based on their individual needs and content preferences. This can open up a future where users have a greater degree of control over how their content is presented, ultimately enhancing the video experience.
While the field is advancing quickly, there are still some open questions and limitations. The interplay of different compression techniques and upscaling methods, for example, is an area that warrants continued research. The potential introduction of new artifacts and the trade-offs between different enhancement methods are factors that need careful consideration as we continue to explore the possibilities of real-time AI-driven upscaling. Nonetheless, these developments suggest that we're moving towards an era where high-quality streaming experiences are readily available, personalized, and increasingly indistinguishable from native high-resolution content.
How AI Video Upscaling Enhances MP4 Downloads A 2024 Perspective - Ethical considerations of AI-enhanced video content
As AI-enhanced video content becomes more sophisticated, it's vital to consider the ethical implications. Transparency and accountability are paramount; audiences have a right to know when AI has been used to alter video, allowing them to make informed decisions about the content they view. The training process for these AI systems relies on massive datasets of existing videos, raising questions about the ethical sourcing of this data. Using content without explicit permission or creating misleading content, like deepfakes, poses ethical challenges that require careful consideration. The rapid development of these technologies demands a thoughtful approach to ensure responsible and ethical use, fostering trust and protecting the integrity of video content. Striking a balance between innovation and ethical safeguards is crucial to prevent potential harm and maintain the authenticity of the viewing experience.
The rise of AI-powered video enhancement, while offering impressive improvements in quality, also presents a complex tapestry of ethical considerations. One key concern revolves around content authenticity. As AI becomes increasingly adept at manipulating video, it becomes harder for viewers to differentiate between original and artificially enhanced content. This challenges the very notion of originality in media, blurring the lines between genuine creative work and digitally manipulated representations.
Closely related to this is the potential for AI to create and spread misinformation through deepfakes. The same tools that enhance video quality can also be used to fabricate convincing yet false content, posing a significant risk in areas like politics and social discourse. This raises concerns about the potential for harmful misrepresentation and the need for responsible development and deployment of these technologies.
Another critical issue is the potential for biases embedded within AI algorithms. Training data, often drawn from diverse sources like YouTube or open-source libraries, can reflect existing societal biases. If these biases are not carefully addressed, the resulting AI-enhanced videos may perpetuate harmful stereotypes or misrepresent certain groups or styles, necessitating a greater focus on building diverse and balanced datasets.
Furthermore, the use of AI in video creation introduces ambiguities around intellectual property. If an AI enhances a video, who owns the rights to the modified content? This uncertainty creates a complex legal landscape and challenges the traditional relationship between creators and their work.
The growing dependence on AI tools for video editing also raises concerns about the potential dilution of traditional filmmaking and editing skills. As engineers and content creators rely more on algorithms, we might see a decline in foundational knowledge and expertise in visual storytelling.
Moreover, the capabilities of real-time AI upscaling technologies raise anxieties about potential misuse for surveillance purposes. Enhanced clarity in live video feeds could exacerbate privacy concerns, potentially leading to more intrusive monitoring. The need for clear regulatory measures to safeguard individual privacy becomes ever more important.
Beyond the individual, the application of AI in video enhancement can lead to a loss of cultural context and ownership. Specific features of imagery that hold significance in certain cultures might be inadvertently altered or decontextualized through AI processing. This raises ethical concerns about the accurate and respectful representation of different cultures and identities within media.
The rise of AI-enhanced video also presents challenges for the video production workforce. As automation becomes more sophisticated, there's a growing worry about job displacement, particularly in fields like video editing and technical roles. This shift in the workforce necessitates adapting educational programs and retraining opportunities to prepare individuals for the changing landscape of media production.
Interestingly, the desire for ever-higher video quality can ironically result in over-enhancement, creating a hyper-realistic and overly polished final product. This aesthetic approach can feel artificial and might alienate viewers who prefer a more natural or authentic viewing experience.
Finally, the increasing sophistication of AI-driven video enhancement naturally raises questions about user control and agency. While AI algorithms become adept at adapting to the content, this can sometimes reduce the creative freedom of content creators. Striking a careful balance between AI assistance and the artist's ability to express their unique vision becomes vital for maintaining authentic storytelling and creative expression.
How AI Video Upscaling Enhances MP4 Downloads A 2024 Perspective - Future prospects AI video upscaling technology beyond 2024
Beyond 2024, AI video upscaling is likely to see even more dramatic improvements. We can expect more advanced algorithms, such as those built on generative adversarial networks (GANs), to create incredibly realistic video, potentially pushing the standard beyond 4K resolution. The ability to upscale video in real-time could become more common, transforming streaming services by enabling immediate quality adjustments during live events, ensuring viewers get the sharpest possible experience on demand.
However, even with this progress, concerns remain. Dealing with artifacts in a seamless way will continue to be an obstacle, as will ensuring that upscaled content appears consistently good across different video sources. The delicate balance between improving the image and keeping it looking natural, rather than overly processed, is a constant concern. As AI upscaling gets more sophisticated, questions of ethics and user control become more urgent. There needs to be a broader discussion about the role of AI in the future of video creation and consumption, particularly ensuring transparency and fostering trust in the technology.
The field of AI video upscaling has seen remarkable progress in 2024, and looking ahead, there's a sense that even more exciting developments are on the horizon. We're seeing a growing trend towards AI algorithms that can learn from user interactions. This suggests a future where upscaling becomes more personalized, tailoring enhancements to individual tastes and editing styles. It's fascinating to consider how AI might incorporate a wider range of data during training. For example, integrating audio cues and video metadata could lead to synchronized enhancements across different media types, a concept that could refine the quality and consistency of the upscaled output.
One of the most encouraging trends is the decrease in computational requirements for real-time upscaling. This means we could potentially see sophisticated video editing tools available not just on high-end desktops but also on smartphones and other more accessible devices. This wider accessibility could significantly democratize video editing and content creation, expanding the pool of individuals who can utilize these advanced technologies. Furthermore, we're seeing algorithms increasingly capable of handling nuanced visual challenges, like handling lighting discrepancies and shadow details more effectively. This suggests a future where upscaling won't just be about increasing resolution but also intelligently addressing diverse visual elements to create a more refined and engaging viewing experience.
I find it intriguing that AI upscaling algorithms might be designed to recognize and respect the original artistic intent of a video. This suggests a more subtle and sensitive approach to enhancement, allowing for better preservation of the aesthetic qualities of the source material. It's an interesting counterpoint to traditional methods that often have a tendency to introduce a uniform, almost overly processed look to videos. There's a clear opportunity to apply these algorithms to the world of archival film and documentaries, enabling a new wave of restoration efforts for older movies and documentaries, reviving classic content for new audiences.
However, this push towards incredibly high-quality and "perfect" video also poses some interesting questions. Will viewers who appreciate the inherent grain and imperfections of older films or documentaries feel a disconnect with AI-enhanced content that strives for unrealistic levels of sharpness? These kinds of questions about visual authenticity will likely become more prevalent in the future. We're also seeing more algorithms incorporate predictive frame analysis. This means AI upscalers could anticipate motion in scenes, potentially leading to incredibly smooth transitions, especially in demanding content like live sports broadcasts or gaming. This aspect is quite promising, with potentially revolutionary applications.
The legal and ethical landscape around AI video enhancements is also becoming more complex. As AI becomes more adept at modifying content, determining the ownership of the output can lead to disputes between original content creators, AI developers, and potentially other stakeholders. This is a vital area that will need further consideration. We might even see future algorithms incorporate engagement metrics. The idea that video platforms could analyze viewer reactions to a video and then adjust the enhancement level based on that feedback is both fascinating and a little bit mind-boggling. This could fundamentally change the relationship between viewers and video content and possibly create a very adaptive streaming experience.
Overall, the future of AI video upscaling appears quite exciting and rich with potential. While there are still many open questions and challenges to navigate, the field's continued advancements suggest that AI upscaling will continue to transform the way we consume and interact with video content, pushing the boundaries of visual fidelity and creativity in remarkable ways.
More Posts from ai-videoupscale.com: