How Match Cuts Transform 4K AI Upscaled Videos A Technical Analysis
How Match Cuts Transform 4K AI Upscaled Videos A Technical Analysis - Match Cut Basics Using Light Direction and Object Shape
When examining match cuts beyond mere visual similarity, we find that the interplay of light and object shapes significantly impacts their effectiveness. A well-crafted match cut can exploit the directional flow of light across scenes, drawing the viewer's eye across the transition and maintaining a sense of visual continuity. Similarly, using objects of similar form or silhouette, like a round window and a full moon, creates a striking visual link, often hinting at deeper thematic connections.
This approach extends beyond mere aesthetics, impacting the emotional and narrative fabric of the piece. The way light and shapes are mirrored across a match cut can subtly influence how we interpret a transition. A shift from a dimly lit scene to a brightly lit one, mirrored by a change in an object's shape, might signify a character's emotional shift or a metaphorical transformation within the story. This manipulation of our perception can effectively guide the emotional journey of the viewer, enriching the overall storytelling.
Ultimately, skillful use of light direction and object shape within a match cut becomes a powerful tool for a filmmaker. It can forge stronger narrative connections between seemingly disparate moments, helping create a compelling viewing experience that effortlessly flows while subtly manipulating viewers' subconscious interpretations.
Utilizing light direction in a match cut can establish a visual bridge between two distinct scenes. By emphasizing similar lighting patterns, we can guide the viewer's eye and build a stronger thematic thread, suggesting a deeper connection.
The concept of object shape in a match cut revolves around our innate ability to recognize silhouettes. When similar shapes are juxtaposed across cuts, our minds naturally establish links, improving the flow of the story and enhancing its emotional impact.
However, lighting can be tricky. If a match cut involves shifting from a brightly lit object to one in shadow, it can be jarring unless handled expertly. It's crucial to carefully craft the transition, ensuring focus shifts smoothly without disrupting the overall sense of continuity.
Crafting a compelling match cut requires a collaborative effort throughout the entire filmmaking process. From the initial vision of the director to the technical expertise of the cinematographer, every aspect must be aligned to perfectly complement the use of light and shape for a seamless visual connection.
The background itself can alter the impact of a match cut. Moving from a complex, cluttered background to a simple one, or vice versa, can change the viewer's emotional response. Clutter can feel more complex, whereas simplicity offers a feeling of clarity.
A related idea, the 30-degree rule, suggests that abrupt camera angle shifts between cuts can harm the effectiveness of a match cut. Keeping consistent camera angles throughout creates better spatial coherence and improves viewer engagement.
Sound design is also vital. When a sound effect is carefully timed to coincide with the match cut, it can further solidify the link between the two images. In contrast, a lack of corresponding sound can weaken the match cut's effect.
Match cuts are not limited to scenes involving movement or action. They can also be applied to contrast static objects, where shape similarity holds particular emotional or narrative importance. This highlights how stillness can be integrated dynamically into the narrative using skilled editing.
The power of match cuts likely arises from the brain's innate ability to recognize patterns. When a viewer encounters a match cut, they subconsciously analyze the relationship between objects and lighting, thereby enhancing their overall understanding and engagement with the story being told.
The evolution of video editing technology, particularly the ability of AI to upscale video, has brought about a new level of precision in crafting match cuts. This heightened control enables creators to push the boundaries of visual storytelling by manipulating light and shape with incredible accuracy, unlocking new levels of creative expression in filmmaking.
How Match Cuts Transform 4K AI Upscaled Videos A Technical Analysis - Frame Matching With Neural Networks For Better Movement Flow
"Frame Matching With Neural Networks For Better Movement Flow" introduces a novel approach to enhancing the smoothness of video transitions. It's no longer just about matching static elements like light and object shapes; now, the focus is on leveraging movement itself. Neural networks analyze frames and identify points of visual continuity based on motion, leading to more natural transitions called "motion match cuts". These cuts seamlessly blend scenes by recognizing shared dynamic elements like camera pans or the movements of subjects within a scene.
The effectiveness of these techniques relies on advancements in algorithms that can accurately predict the flow of motion across frames. Pixel-by-pixel matching and sophisticated multiframe analysis are used to build a more robust understanding of how movement evolves over time. This allows for more precise and rapid identification of matching frames, resulting in smoother and less jarring transitions. This area of research holds promise for revolutionizing how we perceive and experience video narratives, possibly allowing for a more fluid and intuitive experience for the viewer. However, it remains to be seen whether the technology is capable of producing results that are perceptually superior to traditional techniques, which are still often applied for aesthetic and stylistic reasons.
Neural networks are increasingly being used for frame matching, allowing for a more refined approach to creating smooth transitions in video editing. These networks can analyze hundreds or thousands of frames a second, identifying subtle visual cues that might be missed by a human editor. For instance, convolutional neural networks (CNNs) can delve into the texture and movement patterns within each frame, aiding in the creation of more seamless cuts.
Using methods like optical flow, these networks can gauge the motion between frames, helping editors choose match cuts that keep the flow of movement consistent, crucial for action scenes. Furthermore, these algorithms can learn from experience through feedback loops, improving their accuracy in identifying effective transitions based on pre-defined criteria or successful edits from past projects.
This differs from conventional editing, which often relies on manual selection of cuts. AI-based methods consider a wider variety of visual factors simultaneously, which helps to minimize errors and speed up the editing process. And the ability of AI to understand scene context goes beyond just visual similarity, allowing the algorithm to consider the thematic relevance of a cut, leading to transitions that more accurately match the narrative's overall direction.
The availability of high-quality editing tools is democratized through the use of these frame matching techniques. Independent filmmakers who lack extensive editing resources can achieve polished results more readily. The impact on editing workflows can be significant, as editors can spend less time on the more technical aspects of editing, allowing them to focus more on the creative parts.
Looking towards the future, we can expect these neural networks to become more proficient at understanding motion and how it might influence viewers. Potentially, algorithms could even anticipate viewer responses based on how frames transition, ensuring optimal emotional impact.
However, using neural networks in this way brings up important ethical concerns. The ability to manipulate viewer reactions through precisely engineered transitions raises questions about transparency and the creator's responsibility in how they use such technologies. It becomes crucial to balance the use of these tools with the need for genuine storytelling.
How Match Cuts Transform 4K AI Upscaled Videos A Technical Analysis - Color And Contrast Challenges During 4K AI Scale Up
Upscaling videos to 4K using AI offers a compelling path to enhance resolution, but it introduces notable challenges in preserving color and contrast. Although AI can significantly simplify the editing process, achieving accurate color reproduction and maintaining a balanced contrast range isn't always straightforward. The AI may introduce inconsistencies, leading to overly vibrant colors or a loss of the nuanced tonal variations that characterize high-quality visuals. This is especially problematic when aiming for the smooth transitions of match cuts, as sudden, jarring shifts in color and contrast between scenes can disrupt the flow and undermine the intended emotional impact. Therefore, achieving a well-calibrated color and contrast profile during the upscaling process becomes crucial to ensuring the narrative remains coherent and the viewer experience remains engaging. While the potential of AI in upscaling is evident, maintaining a delicate balance of these fundamental visual elements remains vital for a seamless and effective outcome.
In the pursuit of enhancing video quality through 4K AI upscaling, several color and contrast related challenges emerge, particularly when dealing with the nuances of human visual perception. One recurring issue is maintaining **color fidelity** across different scenes. Neural networks, while powerful, sometimes struggle to accurately replicate colors, especially when there are subtle shifts in lighting conditions. These inconsistencies can lead to a jarring visual experience, detracting from the overall impact of the video.
Furthermore, our eyes are not equally sensitive to contrast in all lighting situations. 4K AI upscaling methods attempt to address this by selectively enhancing contrast, ensuring vital details remain visible. However, this process needs to be carefully managed to avoid creating artificial-looking results or compromising the original visual style.
Another complication arises from **chroma subsampling**, a common compression technique that can lead to loss of color information. AI upscaling needs to intelligently compensate for this loss during the upscaling process. The goal is to reconstruct finer color details without introducing unwanted artifacts, which can be a difficult balancing act for the algorithms involved.
Video editors often rely on **histograms** to evaluate color distribution within scenes. One notable hurdle with AI-upscaled content is ensuring that color histograms remain consistent across different cuts or transitions. Significant color variance can create a sense of disorientation for the viewer, pulling them out of the narrative. This emphasizes the need for careful calibration and control throughout the upscaling process.
The process of upscaling itself can lead to **color banding**, particularly noticeable in smooth gradients. AI upscaling tools need to counteract this by employing dithering or smoothing techniques. This ensures a more natural appearance and prevents distracting visual artifacts, particularly in scenes with subtle color variations.
Similarly, the perception of **saturation** is influenced by surrounding colors and contrast. When AI upscaling algorithms automatically adjust saturation for vibrancy, there's a risk of creating an over-saturated look that doesn't match the original material. This is especially problematic if the original visual style leaned towards more subdued or muted colors.
Maintaining consistency in how **light reflections** are rendered during transitions, particularly in match cuts, presents another challenge. The color temperature and intensity of light reflections play a vital role in establishing a coherent emotional tone for a scene. Inconsistent transitions can muddle this, leading to unintentional alterations in the narrative impact.
When considering **dynamic range** adjustments, it becomes crucial to maintain detail in both bright and dark areas. Excessive enhancement of bright areas can lead to clipped highlights, while overly brightening shadows can cause the loss of detail. Both scenarios can compromise the intended storytelling aspects of the video.
Moreover, adhering to established **color calibration standards**, such as Rec. 709 or DCI-P3, is crucial for ensuring that colors are represented accurately across different display types. The AI upscaling algorithms must be sophisticated enough to perform precise calibration that aligns with the required standards.
Lastly, managing **temporal color adjustments** presents a unique challenge. This involves analyzing the color changes that happen over time, especially during scene transitions, to ensure a consistent flow. It's critical for maintaining an unbroken visual experience that supports the narrative's flow and avoids jarring shifts in color perception.
While 4K AI upscaling is undeniably a powerful tool for improving video quality, it's crucial to acknowledge and address these color and contrast related challenges. Only then can we fully unlock the potential of this technology for crafting compelling and visually engaging video experiences.
How Match Cuts Transform 4K AI Upscaled Videos A Technical Analysis - Motion Vector Analysis In High Resolution Video Transforms
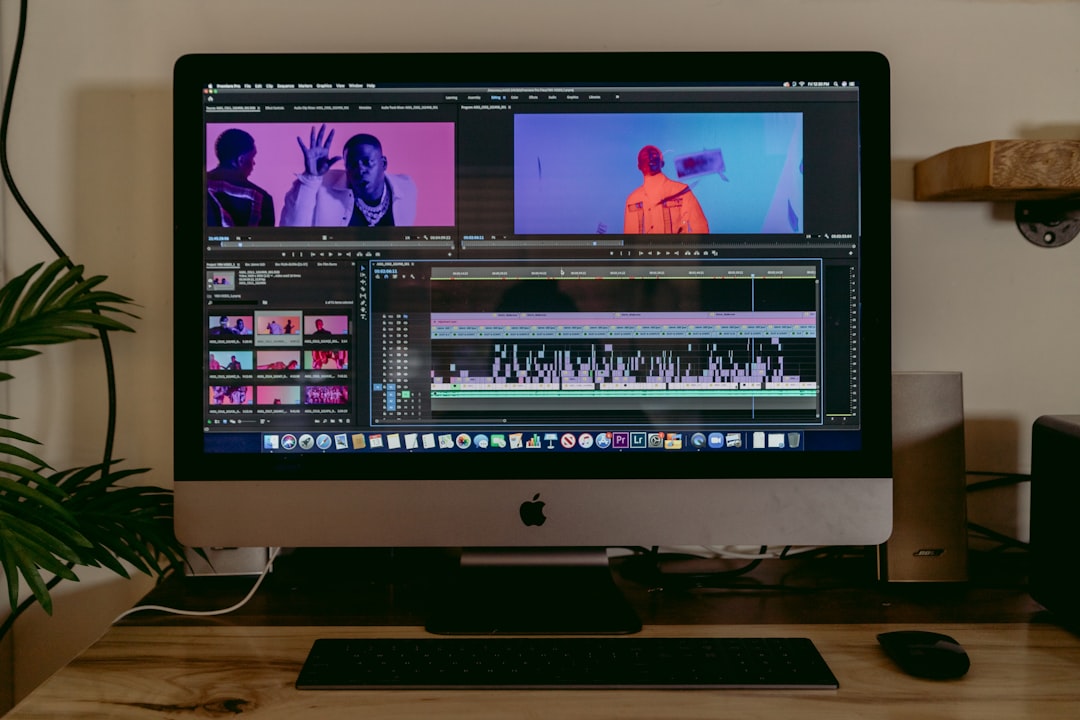
Motion vector analysis is crucial for high-resolution video processing, especially in how videos are compressed and reconstructed. These vectors describe the movement within frames, which helps video decoders understand how to reconstruct the video using motion compensation. The information related to motion, the so-called residual data, is key to compressing the video effectively, allowing for efficient storage and transmission. Modern approaches, like Video Motion Customization (VMC), utilize clever techniques to refine motion vectors for improved keyframe generation, leading to better video quality. Furthermore, encoding methods within video codecs are constantly being improved, incorporating innovations like Motion Vector Coding and Block Merging. These advanced techniques are designed to optimize video compression without compromising visual quality. While these technical advancements offer exciting possibilities for high-resolution video, it's important to ensure these tools are applied in a manner that aligns with the desired artistic or narrative direction of the video. The challenge remains to use these tools in a way that complements creative vision rather than hindering it.
Motion vector analysis plays a crucial role in high-resolution video, especially when dealing with intricate movements and transitions like match cuts. It essentially provides a detailed map of how objects and the camera move within a video sequence, which is fundamental for achieving seamless transitions. This level of precision allows filmmakers to fine-tune match cuts, ensuring the movement flows naturally and viewers don't experience jarring shifts in their perception.
The technology behind motion vector analysis operates at the pixel level, generating a wealth of data about each frame's movement. This granular approach allows for a nuanced understanding of how motion dynamics evolve over time, contributing to more seamless and compelling transitions. By analyzing motion vectors across frames, editors can create match cuts that align with the inherent movement patterns, leading to smoother and more coherent transitions.
Furthermore, motion vectors enable data-driven decision making in editing. Rather than relying solely on intuition, editors can base their decisions on the actual motion data within each frame. This makes it possible to craft more compelling match cuts by aligning dynamic elements based on their past motion. Ultimately, this leads to improved temporal coherence within the edited sequence, where movements transition seamlessly across cuts.
This ability to map and understand motion also plays a significant role in enhancing the storytelling aspect of video. The connections between different scenes, particularly in match cuts, can be made more meaningful by considering the interplay of motion vectors. Filmmakers can create thematic continuity beyond simply matching visual elements, allowing them to leverage the power of motion to enhance the emotional impact of transitions.
Advanced algorithms have been developed to optimize these motion vector analysis processes. This translates to faster frame assessment and improved overall editing efficiency, particularly in large-scale productions where time constraints are significant. Additionally, some motion vector analysis techniques can even operate in real-time during the editing process, enabling immediate feedback, which is critical for dynamically changing scenes, such as action sequences.
While powerful, the accuracy of motion vector analysis has its limitations. Obstacles like object occlusion or variable lighting can introduce errors into the calculations, resulting in potentially flawed motion interpretations. These inaccuracies can negatively impact the efficacy of match cuts if not addressed properly. Nevertheless, studies suggest that well-crafted match cuts, informed by accurate motion vectors, lead to higher levels of viewer engagement and a stronger emotional response.
The development of advanced motion vector analysis draws heavily upon earlier methods like optical flow, which primarily focused on estimating motion based on pixel displacement. However, today's techniques build upon this foundation, incorporating machine learning to significantly refine and enhance the process of motion tracking. This advancement is propelling innovation in video editing and the creation of increasingly dynamic visual narratives.
In essence, the use of motion vector analysis offers a pathway to a new level of precision in video editing, specifically for match cuts. While not without its challenges, it represents a significant stride in how we understand and manipulate motion for storytelling purposes. The future potential for this area of research appears promising, as it continuously refines and redefines the possibilities of creating compelling video experiences.
How Match Cuts Transform 4K AI Upscaled Videos A Technical Analysis - Scene Detection Algorithms For Automated Match Cut Selection
Scene detection algorithms are essential for automating the selection of match cuts, revolutionizing the way videos are edited. These algorithms divide a video into distinct scenes, making it easier to locate appropriate moments for smooth transitions. This involves identifying cuts where the framing, action, or overall composition are similar, creating a sense of visual continuity. Tools like OpenCV and AI-powered software, including CutMagic, have made the process of scene detection automated, leading to faster and more intuitive workflows.
Algorithms like AdaptiveDetector provide a more nuanced approach to scene change identification by using a continuous assessment of frame differences, offering greater accuracy than basic threshold methods. As scene detection becomes more advanced, it allows filmmakers to refine their editing process, giving them more time to focus on the creative aspects of their work, rather than getting bogged down in tedious manual tasks. The trend towards greater automation in video editing is arguably making the creation process more accessible, at least at the technical level. While still evolving, these tools are allowing creators to refine the narrative arc of their films through carefully chosen transitions.
Scene detection algorithms are crucial for automating the selection of match cuts. They leverage sophisticated image processing techniques to pinpoint key visual and temporal cues, enabling software to identify potential match points across diverse frames with impressive accuracy. These algorithms frequently rely on machine learning models trained on large datasets of labeled footage, allowing them to grasp not only individual frames, but also the broader context of movement and scene dynamics—essential elements in crafting effective transitions.
In the context of match cuts, temporal coherence is specifically analyzed through scene boundaries. Many algorithms can identify shifts in visual content and structure within mere milliseconds, leading to faster and more streamlined editing workflows. By dissecting patterns in motion vectors, scene detection algorithms can select cuts that retain the natural flow of action, creating a seamless viewing experience that feels instinctive and connected, even when diverse scenes are juxtaposed.
Algorithms adept at clustering similar scenes can aid in discovering potential match cuts by grouping sequences with comparable motion and visual aspects. This can serve as a stimulating starting point for filmmakers' creative exploration. Some contemporary scene detection techniques integrate multimodal data, merging visual, audio, and textual (like subtitles) information to enrich the contextual understanding of scenes, directly influencing the quality and selection of match cuts.
Despite their impressive capabilities, the efficacy of these algorithms can be hindered by occlusions—when objects obscure others during a scene—making the development of exceptionally precise scene detection a challenging endeavor for engineers. Color histograms play a significant role in scene analysis. Algorithms can compare the color distribution across frames to optimize cuts, ensuring that visual tonalities are aligned for smoother transitions and preventing abrupt color shifts.
One unexpected limitation of scene detection algorithms is their difficulty in interpreting emotional content. Identifying whether a scene is tense or humorous necessitates a profound contextual understanding that goes beyond mere visual recognition, highlighting a gap in present-day AI capabilities. Progress in scene detection is closely linked to advancements in video compression techniques, as efficient encoding methods need to integrate accurate scene identification to optimize the storage and streaming of high-resolution video, illustrating a unique convergence of editing and technology.
More Posts from ai-videoupscale.com: