Upscale any video of any resolution to 4K with AI. (Get started for free)
How Rolling Shutter Effects Create Motion Artifacts in AI Video Upscaling
How Rolling Shutter Effects Create Motion Artifacts in AI Video Upscaling - Camera Sensor Technology Behind Rolling Shutter Motion Capture
The way cameras capture motion is fundamentally tied to the technology used in their sensors. Many modern cameras, particularly those using CMOS sensors, employ a method called rolling shutter. In this approach, the sensor reads out the image row by row, not all at once. This means each row is exposed to light at slightly different moments in time. The duration of this exposure per row is often called "line time," and its variation is key to understanding the rolling shutter effect.
This sequential capturing process inherently creates geometric distortions when objects in the scene are in motion or the camera itself is moving. These distortions manifest as warping, skewing, and other artifacts that can distort the perceived shape and movement of objects. These artifacts can be particularly problematic in fields like robotics or autonomous driving, where accurate motion capture is crucial.
However, the benefits of rolling shutter, such as faster frame rates, have led to its wide adoption. The ability to quickly capture a sequence of images is advantageous for various applications, including video recording. Although researchers are exploring methods to compensate for the artifacts, rolling shutter's inherent limitations continue to pose challenges for image processing and video analysis.
Rolling shutter sensors operate by capturing image data line by line, each line exposed at a slightly different time. This sequential capture introduces a temporal discrepancy, a kind of time-staggered snapshot, which becomes more noticeable in dynamic scenes.
The time it takes to read out a single line, the "line time," influences the severity of the artifacts. While advancements in sensor technology have shortened these times, typically to milliseconds, the inherent nature of the rolling shutter process always presents the possibility of skew or warping, particularly when subjects or the camera are moving quickly.
Interestingly, different sensor types have distinct approaches to image capture. CMOS sensors, prevalent in many modern devices, often employ rolling shutters. In contrast, CCD sensors more commonly utilize a global shutter, which captures the entire image at once, effectively eliminating this specific distortion.
The implications of this staggered capture become more pronounced when it comes to complex vision systems. Algorithms reliant on accurate motion tracking or precise object recognition can be negatively impacted by rolling shutter artifacts. For applications like robotics and autonomous driving, these distortions can be problematic, leading researchers to explore solutions for minimizing or compensating for them.
However, the advantages of CMOS sensors, like faster frame rates and overall performance, have led manufacturers to favor them despite this inherent limitation. It seems that faster capture outweighs the complexities in many applications. In situations where absolute accuracy of captured motion is paramount, though, a global shutter might remain the preferred choice.
Ultimately, the visibility of rolling shutter effects depends on a range of variables. The camera model, the scene's motion, the frame rate being used, and even subtle differences in lighting conditions can all play a role in how these artifacts manifest. In some high-end cameras with fast frame rates, rolling shutter effects can be remarkably subtle, while in others, they can be quite noticeable. Understanding these nuances becomes crucial for researchers and engineers striving for accurate and artifact-free motion capture.
How Rolling Shutter Effects Create Motion Artifacts in AI Video Upscaling - Why Propellers and Fast Moving Objects Create Wave Like Distortions
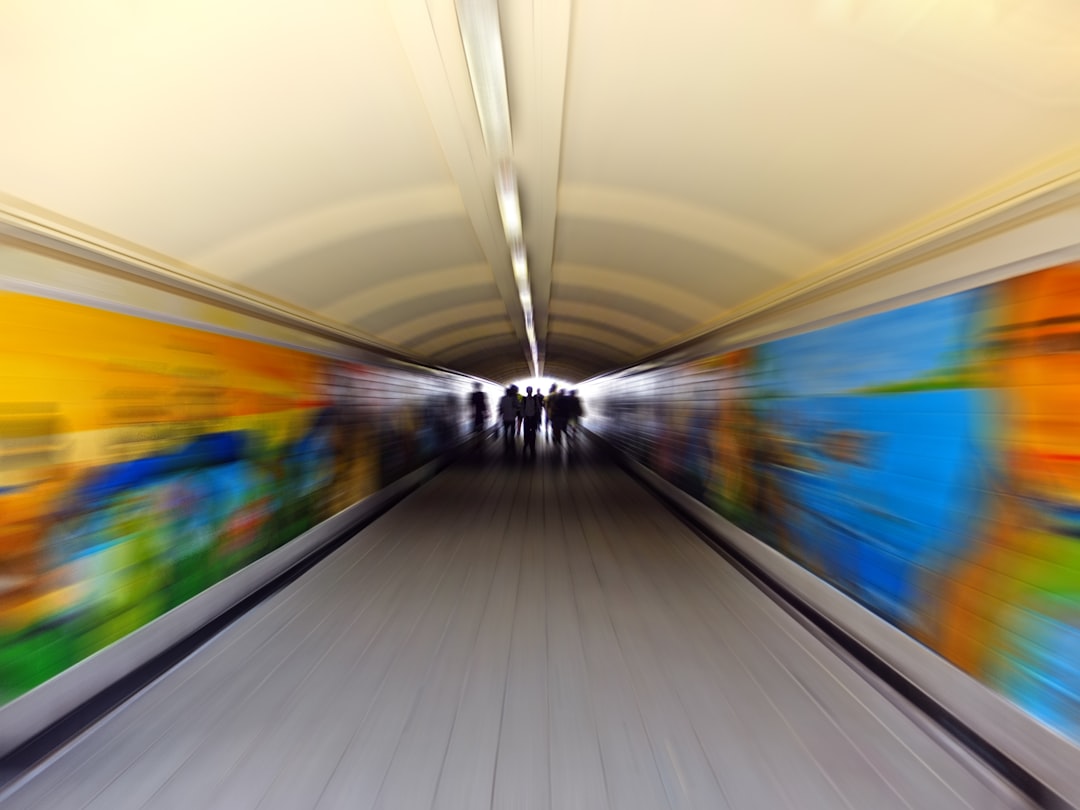
When propellers or objects move quickly across the frame of a camera using a rolling shutter, a wave-like distortion often appears in the resulting image or video. This distortion stems from the way many modern cameras capture images—line by line—using CMOS sensors. Each line is exposed to light at a slightly different moment in time.
As a fast-moving object travels across the sensor, the timing mismatch between these exposed lines creates artifacts that visually warp the object's shape. Straight lines might become curved, and the overall motion may appear distorted or wobbly. This is particularly noticeable in scenarios like capturing spinning propellers or recording with handheld cameras.
The resulting distortions, sometimes called the "jello effect," highlight the inherent limitations of rolling shutter technology when dealing with rapid movements. While rolling shutter offers advantages in terms of speed and frame rate, its sequential nature sacrifices the ability to capture motion with absolute precision. Recognizing these artifacts is important when aiming for accurate motion portrayal or seeking to minimize unwanted distortions in videos and photos.
The way propellers and fast-moving objects distort the surrounding medium, like air or water, is intrinsically linked to their speed and the forces they generate. These objects, by their very movement, displace the medium, creating pressure waves akin to those we see in sound. Think of a boat cutting through water – the waves it creates are a simple analogy. As the speed of an object increases, particularly as it nears the speed of sound (Mach 1), these waves become more complex, transitioning into shock waves and, in the case of exceeding Mach 1, the phenomenon of a sonic boom. These sharp changes in pressure dramatically impact how we visualize motion, especially with the rolling shutter effect at play.
The shape and velocity of objects also play a critical role, as exemplified by a spinning propeller. Bernoulli's principle explains that faster-moving objects generate lower pressure above and higher pressure below, causing the surrounding air or water to flow in a wave-like fashion. This pressure differential is clearly visualized in the swirling patterns of propeller blade tip vortices, a classic example of this dynamic interaction. The frequency of these distortions directly relates to the object's rotational speed and the medium it travels through. Faster spinning translates into higher frequency distortions.
Furthermore, these wave-like distortions interact with external structures, generating reflections and echoes that add another layer of complexity to the already convoluted capture and interpretation of motion by a camera. When you consider a scenario with a rapidly spinning propeller near a wall, the interaction of these waves with the wall can create a very complex pattern of pressure variations, leading to challenges in video capture and particularly challenging the efficacy of AI upscaling in its attempts to clean up motion.
Subsonic and supersonic speeds lead to very different wave patterns. Subsonic movements lead to gradual wave fronts, while supersonic objects generate nearly instantaneous shock waves. The sequential frame-by-frame capture inherent to rolling shutter can struggle to fully capture this contrast in wave behavior, potentially leading to a skewed interpretation of the object's movement and creating a kind of motion illusion. The classic Doppler effect, where the frequency of sound waves changes relative to the observer due to the object's motion, compounds these issues in visual perception and motion analysis.
In water, propellers and similar underwater propulsion devices generate cavitation, the formation and collapse of vapor bubbles. This leads to shock waves and associated noise, adding a further layer of visual complexity. The temporal resolution of a camera sensor becomes critically important in the capturing of such dynamic motion. The rapid fluctuations in wave behavior, as an object changes speed or direction, can exceed the temporal capabilities of many rolling shutter sensors. The resulting artifacts can dramatically misrepresent the actual motion and dynamics. These are some of the primary reasons why these effects can create motion artifacts. Understanding this interaction is crucial for any effort to minimize distortions in motion capture.
How Rolling Shutter Effects Create Motion Artifacts in AI Video Upscaling - Rolling Shutter Motion Artifacts During AI Frame Interpolation
Rolling shutter artifacts create a particular set of problems when AI is used to interpolate frames in video. Fast movements, due to the way CMOS sensors capture images row-by-row, often lead to distortions that are challenging to fix. This staggered exposure, where each row of the image is captured at a slightly different moment in time, causes visual distortions that can manifest as a "jello" effect. This makes the job of video enhancement algorithms much harder, as they strive to create clearer, higher-quality versions of the footage.
While traditional frame interpolation methods have relied on multiple frames to address motion artifacts, they sometimes struggle to maintain visual fidelity, especially when things are moving quickly. More modern approaches, including methods that focus on single-frame correction and those that leverage how frames are connected in time, are showing some promise. Examples include approaches like RSDiffusion or the RSSR pipeline. However, the fundamental issue remains: rolling shutter's nature can introduce a level of distortion that obscures the real motion within a scene. This makes the challenge of completely eliminating the artifacts a complex and ongoing pursuit for researchers and developers. New and innovative techniques will likely be required to fully resolve this issue.
Rolling shutter (RS) artifacts stem from the line-by-line exposure characteristic of CMOS sensors, leading to distortions during fast camera movements or when objects within the scene are in motion. These distortions, which can appear as warping, blur, or other anomalies, make it harder for AI algorithms to accurately reconstruct sharp, high-frame-rate images—the goal of many video upscaling techniques.
Traditional RS correction often relied on analyzing multiple frames to gather temporal information for artifact reduction. More recently, techniques like RSDiffusion, based on diffusion models, focus on correcting RS distortions within a single frame. The idea is that by reducing noise and artifacts in a frame-by-frame manner, overall image quality improves.
Another approach combines deblurring with RS correction, aiming to tackle both blur and distortion simultaneously for better frame interpolation. Interestingly, it's been shown that these motion-related distortions, be it from rolling shutter or even global shutter blur, can contain temporal clues that help AI systems better estimate intermediate frames during video frame interpolation (VFI).
While event-based vision sensors (EVS) offer potential improvements in motion capture compared to CMOS, they're not without their own complexities. Handling things like pixel latency remains a challenge if EVS is to fully deliver on its promise of higher-quality motion capture. One approach, called Rolling Shutter temporal Super-Resolution (RSSR), tries to simultaneously correct for RS effects and interpolate frames by examining temporal relationships between frames.
Some AI models simplify the correction process by assuming the camera moves at a constant rate and that the scene is mostly static. This streamlines the process but may limit accuracy in more complex scenarios. Ultimately, a more comprehensive strategy, one that integrates deblurring, RS correction, and interpolation, is likely needed to tackle the challenges of high-motion video capture. This kind of integrated approach offers the best path toward better video quality, especially when capturing fast-moving subjects under difficult lighting conditions.
However, it's important to recognize that the success of any correction method hinges on the nature of the scene itself. The complexity of the scene, with elements like multiple moving objects, variable lighting, or a blend of fast and slow motion, can heavily impact the ability of any algorithm to correct for rolling shutter artifacts. While some models assume static scenes or constant camera motion, this simplification may not always hold true, hindering accuracy. The viewer's perception of these artifacts is also subjective, with those accustomed to high-quality video being more sensitive to these kinds of distortions than the average viewer.
While rolling shutter technology remains prevalent in a wide range of imaging devices, global shutter technology, which eliminates temporal discrepancies, presents a more accurate path toward truly artifact-free motion capture. However, global shutter technology typically necessitates more complex and expensive sensor designs. The choice between rolling shutter and global shutter often involves a trade-off between cost, performance, and motion accuracy. Even older technologies, like mechanical shutters, have limitations when it comes to handling fast movements. These issues highlight that effectively capturing and correcting rolling shutter artifacts, especially in AI-powered video upscaling, remains an area with active research and development.
How Rolling Shutter Effects Create Motion Artifacts in AI Video Upscaling - Motion Vector Analysis Methods for Rolling Shutter Compensation
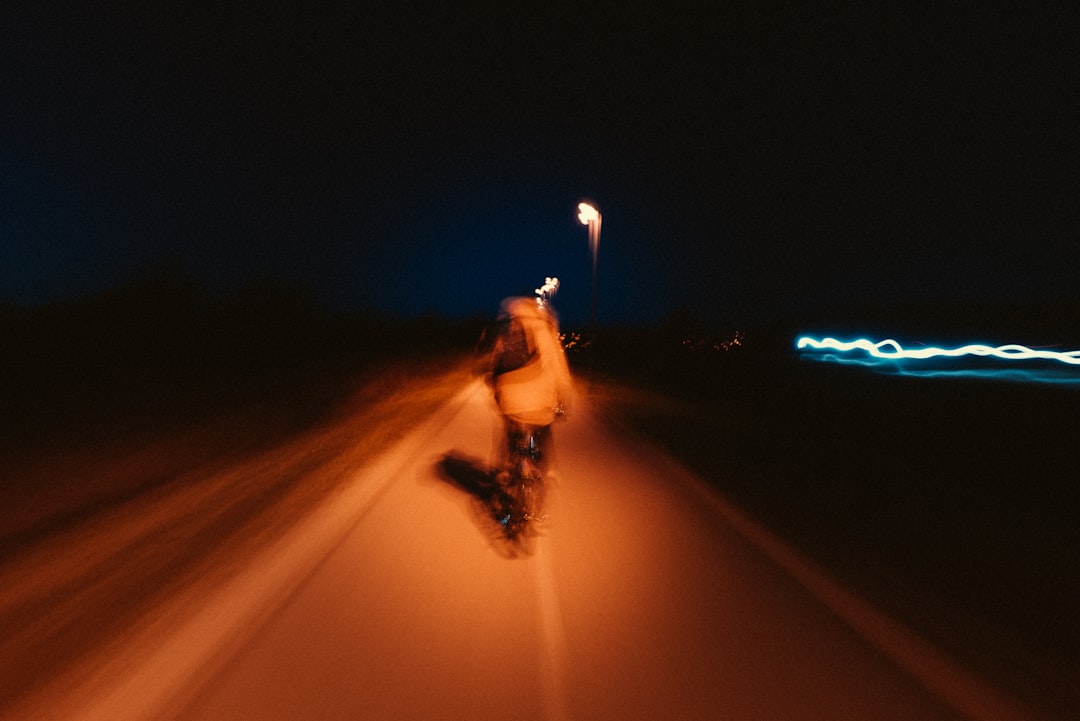
Rolling shutter compensation has become increasingly important as video quality expectations rise. The sequential line-by-line scanning of CMOS sensors, while offering speed, creates distortions when cameras or objects in the scene are moving quickly. Motion vector analysis methods offer a path to fix these issues by identifying local motion within each frame. This information is used to correct for the geometric distortions that result from the rolling shutter effect.
Newer approaches to motion estimation try to simplify the process, avoiding the complicated 3D matching techniques that were once standard. Probabilistic methods, which rely on predicting the likelihood of certain motion patterns, offer one way to simplify the problem. Additionally, researchers are exploring how to analyze the distortions within and between frames in more straightforward ways. These efforts aim to make rolling shutter compensation faster and more efficient.
Another promising area of research involves integrating the way objects look and how they move. This combined approach, known as joint appearance and motion learning, seeks to extract more complete information about the scene than older methods that separate motion analysis and object recognition into distinct stages. The hope is that these integrated approaches may produce better rolling shutter compensation results in real-world applications.
Overall, rolling shutter distortion remains a significant challenge across various applications. These emerging techniques, focusing on motion vector analysis and related methods, represent a vital area of research in video processing and AI-based upscaling. The need to refine and improve video quality will continue to drive further innovation in this area.
1. **Sequential Capture and Time Delays:** The rolling shutter effect stems from the sequential way CMOS sensors capture images, row by row. This sequential capture inherently introduces time delays, leading to distortions that can manifest as warping or skewing, particularly when objects move quickly within the frame.
2. **Motion Path Accuracy:** The line-by-line capture creates a latency that impacts motion path accuracy, especially when precise motion reconstruction is crucial. This latency can create a noticeable difference between the perceived motion in the video and the actual motion that occurred, which is problematic for many applications.
3. **Sensor Temporal Resolution's Role:** The effectiveness of motion vector analysis for rolling shutter correction hinges heavily on the sensor's temporal resolution. If the sensor has a low temporal resolution, it might not be able to capture rapid changes in motion, potentially making the artifacts even more pronounced.
4. **Challenges with Fast Objects:** Rapidly moving objects, such as a spinning propeller, pose a particular challenge for rolling shutter systems. The sensor's sequential readout can cause the tips of the propeller to appear bent or distorted due to the timing mismatch between the rows of the sensor during capture.
5. **Speed and Pressure Waves:** Different speeds of motion generate different pressure wave patterns. Rolling shutter effects can misrepresent these wave patterns, especially for objects traveling near the speed of sound. This can be problematic for any motion analysis algorithm that tries to reconstruct or compensate for this distortion.
6. **Error Accumulation Over Time:** As motion vectors are tracked across frames, any errors from the rolling shutter effect can compound, potentially leading to a significant divergence from the true object trajectory, particularly during high-speed movement.
7. **Leveraging Distortions as Clues:** Interestingly, some newer approaches exploit the distortion patterns caused by rolling shutter effects as temporal clues. While promising, the effectiveness of these methods depends on the scene's complexity and the nature of the motion being captured.
8. **Sensor Technologies and Their Tradeoffs:** The sensor technology plays a major role in motion analysis and rolling shutter effects. CMOS sensors, despite their speed and efficiency, are prone to these artifacts. Global shutter systems offer a more robust solution, but they often come at a higher cost.
9. **The Subjectivity of Artifact Perception:** How much someone cares about rolling shutter artifacts seems to be a matter of taste. Someone used to high-quality video may notice these artifacts more than the average viewer, making standardization of correction methods challenging.
10. **Future Directions and Emerging Technologies:** New technologies, such as event-based vision sensors (EVS), hold potential for improving motion capture by overcoming some of the limitations of traditional sensors. However, EVS is still developing, and issues like pixel latency need further refinement before they can be a true improvement for applications requiring very accurate motion reconstruction.
How Rolling Shutter Effects Create Motion Artifacts in AI Video Upscaling - Temporal Inconsistencies in Neural Network Based Video Processing
Neural networks applied to video processing can introduce issues related to how time is represented, which is problematic for upscaling videos. When each frame is processed independently, the resulting video can suffer from a lack of smooth, consistent motion. This can manifest as unwanted visual distortions, especially when objects move quickly, including warping and skewing. Addressing this issue has led researchers to explore novel training methods that focus on pairs of videos – an original and a processed version – rather than relying on huge datasets. This paired approach helps to establish a better sense of temporal consistency.
Despite these improvements, developing a single solution that handles a wide variety of motion scenarios remains elusive. The complexity of a scene, including the presence of multiple moving objects or changes in lighting conditions, can challenge the consistency of the resulting video. There's a growing need for a more flexible framework for video processing techniques that can be readily applied to different scenarios and reliably produce temporally consistent output. This is crucial for improving the quality of AI-based video enhancements, particularly when dealing with challenging motion scenarios.
1. **Temporal Differences in Image Capture:** The way rolling shutter cameras capture images, line by line, introduces subtle time differences between each row's exposure. While usually imperceptible, these small time lags can create noticeable motion artifacts, especially when dealing with scenes containing quick movements. This can lead to objects appearing distorted or warped.
2. **Challenges for Motion Tracking:** Algorithms designed to track motion rely on consistent and accurate data about how things are moving. The temporal inconsistencies from the rolling shutter effect can throw off these algorithms, making it harder to accurately track motion, which is crucial for things like robotics or self-driving systems.
3. **Line Time's Influence on Artifact Severity:** The duration it takes for the sensor to read each line, called "line time," plays a big part in how severe the artifacts become. Cameras with longer line times tend to produce more pronounced distortion effects. It's something to consider when choosing a camera for applications that need precise motion tracking.
4. **Speed's Impact on Artifacts:** The faster things move, the more noticeable the artifacts tend to be. As motion speeds increase, the time discrepancies between lines become more significant, leading to a greater degree of distortion. This is particularly noticeable with cameras that have slower line times or operate at lower frame rates.
5. **Difficulties for AI Video Enhancements:** AI algorithms trying to enhance video captured with a rolling shutter face an uphill battle because of the inherent distortions. These distortions complicate the process of cleaning up the video and creating a more pleasing visual experience. Algorithms trying to smooth out motion and improve overall quality can struggle to overcome the inconsistencies inherent to rolling shutter.
6. **Accumulating Errors in Interpolated Frames:** As AI algorithms try to create new frames between existing ones, the rolling shutter distortions can lead to an accumulation of errors. This can lead to interpolated motion appearing noticeably off, with the overall motion becoming less smooth and more inaccurate. The faster the motion being interpolated, the more these errors compound and the more apparent they become.
7. **Using Distortions as Clues:** Some researchers are finding ways to use the distortions themselves as a source of information about motion. If these distortions can be analyzed and understood, they may offer clues to better estimate how things are moving. While promising, these techniques are still being developed and tested.
8. **Global Shutter as an Alternative:** Cameras that use a global shutter capture the whole image at once, avoiding the temporal inconsistencies of rolling shutter. While global shutter cameras can be more complex and costly, they offer a simple solution to the distortion problem and are a strong option when truly accurate motion capture is a must.
9. **Subjective Nature of Artifact Visibility:** Not everyone notices rolling shutter artifacts to the same degree. Viewers who are used to high-quality video might be more sensitive to these issues, while others might not even notice them. This difference in perception makes it difficult to determine when a particular level of distortion becomes problematic.
10. **Promising New Sensor Technologies:** There are newer types of image sensors, like event-based cameras, which offer the potential to overcome some of the limitations of traditional rolling shutter CMOS sensors. However, challenges still exist with these new technologies, such as pixel latency, which need to be solved before they can be widely adopted for applications needing high-quality motion capture.
How Rolling Shutter Effects Create Motion Artifacts in AI Video Upscaling - Technical Limitations of Current Rolling Shutter Correction Algorithms
Current algorithms designed to correct for rolling shutter effects face limitations that hinder their ability to fully eliminate motion artifacts in video. These algorithms frequently struggle with scenes featuring both camera and object movement, resulting in a complex interplay of motion blur and rolling shutter distortions. Many established methods were primarily developed for correcting distortions in static scenes and often don't translate effectively to dynamic, real-world conditions. Furthermore, traditional approaches that rely on multi-frame analysis for motion correction can fall short when dealing with rapid movements, potentially exacerbating the artifacts they aim to reduce. Although newer approaches, such as diffusion models, have shown promise in addressing these issues by offering single-frame correction possibilities, developing universally effective and robust rolling shutter correction algorithms remains an active area of research and a key hurdle in achieving artifact-free motion capture.
1. **Temporal Differences in Sensor Readout:** The core of the rolling shutter issue is the slight time difference between when each sensor row captures light. While often tiny, these time offsets can add up, creating noticeable image distortions when motion is present. It's a fundamental limitation of the technology that's hard to completely eliminate.
2. **Frame Rate Limitations:** The relationship between frame rate and rolling shutter correction is complex. While higher frame rates can sometimes lessen some distortions, they can also worsen other issues if the sensor's line reading speed isn't optimized. This makes it tricky to get a perfectly synchronized motion capture.
3. **Increased Distortions with Speed:** The faster things move, the more severe the rolling shutter effects become. Blur caused by motion can mix with the "jello" effect, making it difficult for algorithms to tell the difference between genuine motion and the artifact. It's a particularly challenging problem for capturing fast moving objects.
4. **Relying on Motion Vector Accuracy:** Algorithms that try to fix rolling shutter often rely on motion vectors to track movement. But the unpredictable nature of the artifacts means that if the motion vectors are inaccurate, the fixes might make things worse. Accurate motion estimations are crucial to effective rolling shutter correction.
5. **Varied Line Times Across Sensor:** When the sensor's line reading times aren't consistent across the whole sensor, it can further complicate rolling shutter correction. These inconsistencies make artifacts worse, especially when fast-moving objects travel across the frame. It highlights the need for uniform and finely tuned sensor designs.
6. **Challenges Integrating AI Models:** While machine learning-based methods offer promise for rolling shutter correction, they require training on vast datasets representing various motion scenarios. The trouble is, it's difficult to capture every conceivable motion, leading to algorithms that struggle when presented with new and unexpected situations.
7. **Comparing Event-Based and Rolling Shutter:** Event-based cameras show promise over traditional rolling shutter cameras because they capture motion events with very little delay. However, these newer sensors also have their own complexities. Processing their data and integrating them into current systems are both hurdles that must be addressed.
8. **Implications for 3D Reconstruction:** Rolling shutter can wreak havoc on 3D reconstruction efforts because the slight time differences between rows make it tough for algorithms to accurately estimate the position of moving objects. This impacts applications that rely on precise depth perception like computer vision and robotics.
9. **Error Build-up in Correction:** When trying to fix rolling shutter artifacts, any errors can accumulate from frame to frame. This cascading effect can make corrections less effective, particularly in scenes with a lot of fast motion. Finding a way to minimize error accumulation is key to successful correction.
10. **Trade-off Between Cost and Performance:** The choice between using a CMOS sensor with its rolling shutter or a more expensive global shutter system reflects a common challenge in the field. Global shutters offer uniform motion capture without distortions, but their higher cost and complexity limit their use in more affordable devices. It's a balancing act that influences what technologies are used in different applications.
Upscale any video of any resolution to 4K with AI. (Get started for free)
More Posts from ai-videoupscale.com: