Upscale any video of any resolution to 4K with AI. (Get started for free)
How to Upscale 1080p to 4K Using DaVinci Resolve 185 Free Step-by-Step Super Scale Guide
How to Upscale 1080p to 4K Using DaVinci Resolve 185 Free Step-by-Step Super Scale Guide - Setting Up Your Timeline and Import Settings for 4K in DaVinci Resolve
To effectively upscale 1080p footage to 4K within DaVinci Resolve, you need to set up your project correctly from the start. This involves establishing a 4K timeline resolution, which is essential for the upscaling process to work properly. Head to the Project Settings and choose Ultra HD as your timeline resolution. Doing this at the beginning ensures the software correctly scales your 1080p footage when you import it.
DaVinci Resolve's Super Scale tool is where the magic happens, leveraging AI to enhance the quality of lower resolution footage. While utilizing this feature, it's important to note how output settings can impact your final result. Be mindful of how you set your output scaling and codec options, as they can influence the clarity and integrity of your upscaled 4K video. The right codec choice can make a significant difference in the quality of your exported video. While these steps might seem minor, they play a crucial role in ensuring your upscaled footage retains its detail and sharpness.
1. When setting up a project specifically for upscaling, choosing the target resolution—like Ultra HD for 4K—right at the start in the project settings can be crucial for the Super Scale algorithm to function optimally during import and throughout the process. This early decision can help ensure that the scaling process itself is optimized from the initial stages.
2. While the underlying AI in Super Scale is quite powerful, the input format of the 1080p footage can impact the results. Certain video codecs and containers might interact differently with the upscaling process, so it’s worth exploring if some formats yield better outcomes than others for 1080p to 4K upscaling.
3. DaVinci Resolve’s Super Scale feature seems to leverage machine learning models, which can vary in their strengths. The internal logic of the AI itself is likely responsible for improving pixel quality and resolving details in a visually compelling way, but understanding the limitations and strengths of these techniques can help us interpret the results.
4. To use Super Scale for a particular clip, it's necessary to individually adjust clip attributes for each source file, applying the Super Scale settings as desired. This control allows for selectively applying Super Scale, a useful feature when only specific sections of your 1080p content require upscaling.
5. The export settings play a significant role in how well the upscaled content looks after the editing phase. Ensuring the 'Use Timeline Settings for Output Scaling' is activated means the settings for resolution you’ve applied to the timeline during editing will be carried over to the output, maintaining consistency.
6. When encountering different aspect ratios or resolution mismatches in the source footage, the 'Scale Entire Image to Fit' method can help create a more consistent image. However, there can be drawbacks to this method if the content features specific compositions that require more precise control, so it's worth considering on a case-by-case basis.
7. To simplify workflow and ensure the project is geared for the desired resolution, setting up a dedicated 4K project before importing any lower-resolution footage that will be upscaled is a good practice. This can prevent issues related to resolution mismatches or compatibility complications down the road.
8. The choice of codec during the export stage has a direct impact on the final quality, both in terms of visual fidelity and file size. Since compression plays a critical role, it’s vital to experiment with different codecs and assess their trade-offs, especially when comparing things like file size and quality retention.
9. VP9, in its current implementation, is commonly used in online platforms like YouTube for delivering 4K content. As technology evolves, newer codecs may emerge with further optimized compression, and these should be kept in mind when considering output formats in the future.
10. Upscaling effectively in Resolve 18 can seem daunting at first, but thankfully detailed resources and step-by-step guides are available to help guide users through the entire process. This ensures that individuals with varying levels of experience can get satisfactory results without having to rely on guesswork.
How to Upscale 1080p to 4K Using DaVinci Resolve 185 Free Step-by-Step Super Scale Guide - Getting Started With Super Scale Inside the Media Pool
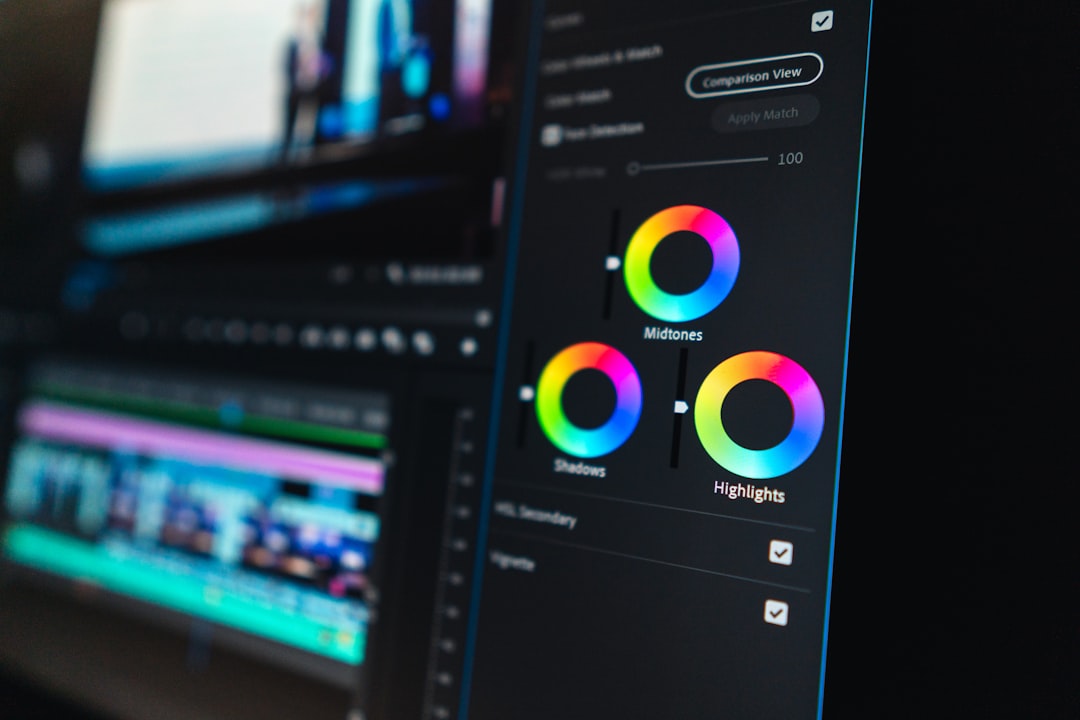
Within DaVinci Resolve's Media Pool, Super Scale offers a way to improve the resolution of your videos. To begin, import your 1080p footage into a timeline set up for 4K output. Then, right-click on the clip to find the "Clip Attributes" menu. In there, you'll locate the Super Scale option where you can choose the upscaling level – 2x for 4K, 3x for 6K, or 4x for 8K. However, keep in mind that this AI-powered upscaling tool is only available in the paid Studio version of the software. As a best practice, review your image scaling settings to avoid visual artifacts during the upscaling process. This feature can be especially useful if you're working with older footage or materials that originally didn't have the resolution you need for current projects. By employing advanced algorithms, Super Scale can significantly enhance the clarity and visual fidelity of your content, a boon for professionals striving for high-quality results. It's important to acknowledge, though, that the extent of this enhancement will depend on the source material itself.
Super Scale within DaVinci Resolve seems to be a hybrid of established upscaling methods and newer machine learning approaches. This begs the question: how much genuinely new detail can be synthesized from lower resolution sources, as opposed to just creating more pixels? The upscaling's effectiveness appears to depend heavily on the specific characteristics of the footage. For example, detailed scenes like expansive landscapes often yield much better outcomes compared to footage containing fast motion or low contrast.
The starting quality of the 1080p footage also significantly influences the final results. High-quality source material can surpass initial expectations, while lower-quality inputs might reveal compression artifacts that the algorithm struggles to fully mask. Interestingly, the resolution and pixel density of the display used to view the upscaled footage play a substantial role in its perceived quality. While even advanced algorithms cannot fabricate detail that wasn't originally present, they can improve the appearance of the upscaled footage on displays capable of presenting high resolutions.
DaVinci Resolve's Super Scale process can involve multiple passes of data, which can noticeably impact the render times. This trade-off between quality and time becomes crucial for large or complex projects. Some observations suggest that color accuracy can sometimes be subtly altered during the upscaling process. This hints that a final color grading step might be necessary after the upscaling to ensure the color fidelity is consistent with the original footage.
Upscaling clips with varying frame rates seems to introduce additional challenges. Maintaining a consistent frame rate across all clips before initiating the upscaling process might help in preventing potential playback issues after exporting the project. Effectively using Super Scale may require a deeper understanding of coding theory and visual perception, especially for experienced users. This points towards the need for a balanced combination of technical knowledge and artistic intuition during the post-production stage.
Users might find that system performance varies considerably when running Super Scale, especially across different hardware setups. Some systems could struggle under heavy workloads, while others handle the process with ease. This highlights the importance of carefully considering the hardware capabilities when setting up workflows for upscaling. Exploring how other editing software handles upscaling, and comparing their approaches with Super Scale in DaVinci Resolve, could provide a deeper understanding of best practices and workflow choices in video production. By doing so, we can potentially uncover further insights about the capabilities and limitations of the various tools available.
How to Upscale 1080p to 4K Using DaVinci Resolve 185 Free Step-by-Step Super Scale Guide - Applying Neural Engine AI Upscaling to Your 1080p Footage
DaVinci Resolve's Super Scale leverages its Neural Engine to boost the resolution of your 1080p footage, particularly when aiming for 4K output. This AI-powered upscaling tool, exclusive to the paid Studio version, creates new pixel data based on the existing content. You can scale up by multiples of 2, 3, or even 4, effectively achieving 4K, 6K, or 8K resolution. However, the success of this process hinges on the initial quality of your 1080p footage. While Super Scale can enhance the visuals considerably, it can't entirely eliminate artifacts present in poor-quality source material.
To optimize your results, you should consistently manage your settings and meticulously choose appropriate export configurations. Different codecs and formats react differently to upscaling, and making the right choices here can be pivotal in retaining a clear, visually appealing outcome. Understanding the limits and capabilities of the Neural Engine AI employed in Super Scale is key to harnessing its potential within your editing workflow. It's not a magical solution, but when used with care, it can elevate the visual appeal of older or lower-resolution video content.
DaVinci Resolve's Super Scale feature, powered by the Neural Engine, utilizes AI to enhance the resolution of 1080p footage to 4K and beyond. It operates by predicting pixel values based on surrounding pixels, essentially "guessing" what the missing detail might look like. The effectiveness of this prediction hinges heavily on the original footage quality.
The neural network models used are trained on diverse data, and some perform better on specific content than others. For instance, a model proficient with wide-open landscapes might struggle with scenes of rapid motion. This process of AI-driven upscaling is quite demanding on processing resources, leading to potentially extended render times. This is particularly noticeable for intricate or lengthy projects, a tradeoff between improved detail and waiting.
Interestingly, while Super Scale sharpens the image, it can also amplify any compression artifacts hidden within the original 1080p clip. So, the initial source's quality becomes critical for achieving a favorable upscaled outcome.
Furthermore, the resolution of the display used for viewing the upscaled footage greatly influences the perceived quality. Higher resolution displays can reveal the more subtle refinements from the algorithm, whereas lower resolution displays might not capture the same level of enhancement. The selected export codec can significantly impact the upscaling results, too. If a lossy codec is used, some of the benefits of AI upscaling can be lost through compression.
While AI can convincingly fabricate the impression of new details, it can't truly generate information that wasn't in the original. Hence, depending on the source quality, the upscaling effects can differ considerably. Clips with varying frame rates can pose problems. Maintaining consistent frame rates prior to the upscaling process becomes essential to prevent potential playback hiccups later. It's worth noting that color fidelity can sometimes be slightly altered during the upscaling process, so a subsequent color grading pass might be necessary to fine-tune the final output.
The efficiency of Super Scale depends significantly on the available hardware. Powerful GPUs and CPUs are better equipped to handle the resource-intensive nature of the upscaling process. This translates to faster render times and potentially higher-quality results. Examining how other video editing software handles upscaling, comparing them to DaVinci Resolve's Super Scale, could help refine our understanding of effective workflows for upscaling video. By comparing different approaches, we might gain insights into the capabilities and limitations of the diverse tools that exist.
How to Upscale 1080p to 4K Using DaVinci Resolve 185 Free Step-by-Step Super Scale Guide - Fine Tuning Edge Detail and Noise Reduction Parameters
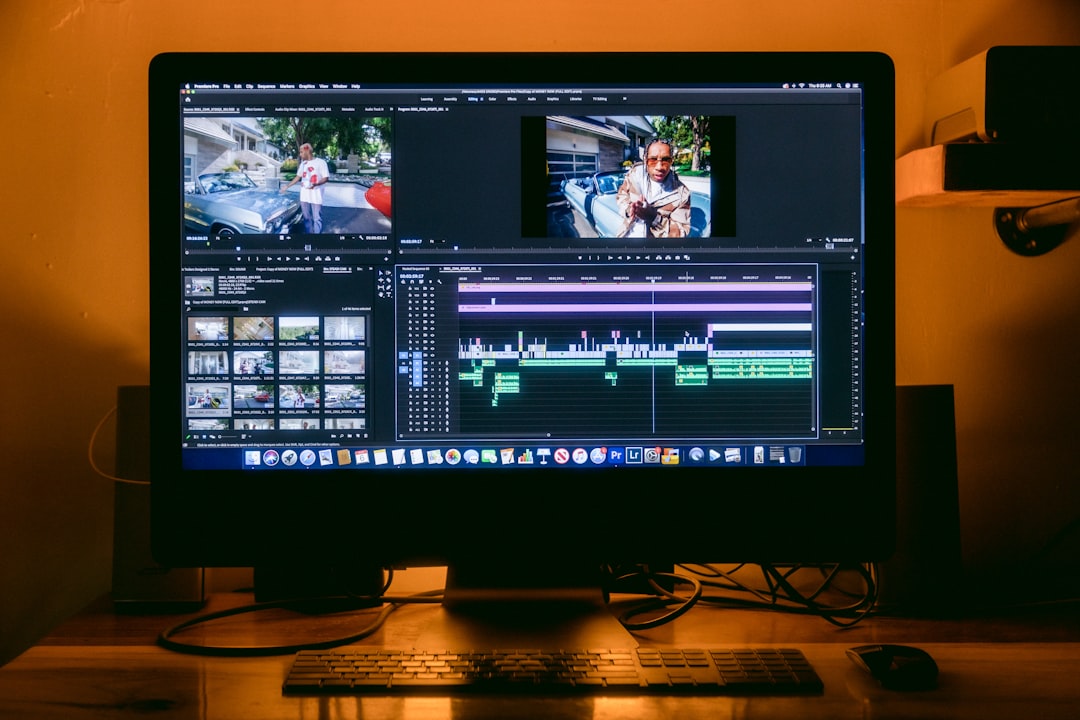
When upscaling video using DaVinci Resolve's Super Scale, fine-tuning the edge detail and noise reduction settings is vital for achieving optimal results. While Super Scale uses clever algorithms to improve image sharpness, incorrect settings can introduce unwanted artifacts or lose crucial details. It's best to refine sharpness and noise reduction levels *before* starting the upscaling process. This is because any noise or grain present in the original 1080p footage can become more noticeable after it's upscaled to 4K. Moreover, tools like Temporal Noise Reduction or specialized noise-reduction plugins can be valuable for pre-processing the video, allowing the AI algorithm to operate on a cleaner signal. Ultimately, the quality of the final 4K output depends not only on these adjustments but also on the quality of the original 1080p source material and the capabilities of the display used to view it.
When refining the edge detail and noise reduction parameters within DaVinci Resolve's Super Scale, we encounter a fascinating interplay of algorithms and image characteristics. It's intriguing how some noise reduction algorithms can now differentiate between noise types—like the color artifacts (chroma noise) or the variations in brightness (luminance noise)—and tailor their reduction accordingly, instead of a uniform approach across the whole image. This selective noise treatment could be a valuable tool in preserving a more natural look in the image.
Maintaining sharpness during the upscaling process is paramount. Effective upscaling techniques try to isolate and preserve edge detail while trying to discriminate between true detail and background noise. If this is done correctly, we can lessen the likelihood of halo effects or undesired blurring around objects. It’s remarkable how these methods seemingly distinguish between a sharp edge and noise, resulting in cleaner and sharper upscaled footage.
The initial bit depth of the footage being upscaled has a significant influence on how effectively we can reduce noise and enhance edge detail. Footage captured at higher bit depths contain more data about the image, giving us a finer degree of control over color and luminance values. This can lead to smoother gradients and a more natural look when making adjustments during the upscaling process. It's still unclear to me whether Super Scale can improve the bit depth during the process, or if we're simply refining what’s already there.
One interesting aspect of noise reduction is that it often operates in the frequency domain. What that means is that algorithms look at the range of frequencies (similar to sound) in an image and target specific frequency components that relate to noise. This type of frequency-based filtering allows for a more targeted approach to noise reduction while ideally preserving essential details within the image. It would be interesting to understand what specific frequencies are being targeted by Super Scale.
Adjusting noise reduction often has a subtle interplay with how edges are treated in the image. It seems that pushing noise reduction too far can lead to softened edges, creating an undesirable blur. This dynamic interaction between parameters necessitates a degree of finesse, as operators must navigate a delicate balance between reducing noise and maintaining a sharp image. This trade-off is perhaps the most challenging aspect to the Super Scale process and highlights the importance of the operator’s skills and eye.
Temporal noise reduction leverages the information available across multiple frames in the video to enhance image quality. By looking at how noise changes over time in nearby frames, it becomes possible to differentiate between actual image content and fleeting noise. This is particularly useful in footage with a lot of movement. It’s amazing to imagine how these algorithms process and interpret the frames in order to achieve this result.
It's convenient how DaVinci Resolve provides real-time feedback when changing the edge and noise reduction parameters. This enables users to immediately see how their adjustments alter the image. This sort of immediate visual feedback minimizes guesswork and speeds up the process of obtaining desired results. It would be great if there were some sort of quantifiable measure of image quality in real-time so we could evaluate our process more objectively.
For users who frequently apply the same types of adjustments to similar types of video content, it's possible to build and save their own presets. A preset encapsulates all of the choices made in the Super Scale process. This provides a starting point for future projects and makes the upscaling process even quicker, since they can then fine-tune those presets based on the needs of the particular project. This approach would also be beneficial for research, so we can better compare the upscaling results of different preset configurations.
Improvements in software continue to advance noise reduction capabilities. Machine learning is playing a critical role in noise reduction, by training models on enormous datasets of video images. These more advanced models are adept at adapting to different video characteristics, improving overall clarity while potentially minimizing the creation of visual artifacts. It's intriguing to imagine what this new breed of noise reduction might accomplish in the future.
Finally, depending on the processing capabilities of the editing system, users can opt to use either the central processing unit (CPU) or the graphics processing unit (GPU). GPU acceleration can accelerate the time it takes to apply adjustments. This can be particularly helpful when making adjustments to a large number of clips, where render times can become significant. A better understanding of how GPUs interact with the specific algorithms employed in Super Scale would be valuable for researchers and users who have access to multiple machine configurations.
How to Upscale 1080p to 4K Using DaVinci Resolve 185 Free Step-by-Step Super Scale Guide - Optimizing Export Settings for Maximum Quality
When exporting your upscaled 4K video from DaVinci Resolve, the export settings become crucial for maintaining the quality you've achieved through Super Scale. The choice of codec plays a key role; options like H.265, H.264, DNxHR, and ProRes each offer different compression characteristics. Picking the right codec balances visual fidelity with file size. You'll also want to consider the bitrate—a higher bitrate generally results in better quality but larger file sizes.
It's important to make sure the "Use Timeline Settings for Output Scaling" option is active in the Deliver panel. This ensures the 4K resolution you've established in your project settings is carried through to the final export, avoiding unexpected changes in resolution or aspect ratio.
Bear in mind that some codecs, especially lossy ones, can introduce compression artifacts that may negate some of the improvements achieved during the AI upscaling. This emphasizes the importance of testing and selecting codecs that best maintain the sharpness and detail obtained through Super Scale. Ultimately, finding the optimal balance between codec choice, bit rate, and resolution is essential to produce a high-quality 4K export that preserves the enhanced visual fidelity you've worked to achieve.
When optimizing the export settings for the best possible quality after using DaVinci Resolve's Super Scale, several factors come into play. One of these is the bit depth of your original 1080p footage. Higher bit depth footage contains more information about the colors in your image and allows for more fine-tuned adjustments during the upscaling process, potentially yielding a smoother and more natural look for your final 4K output.
Noise reduction algorithms in Super Scale often work by analyzing the different frequencies (like tones in sound) within the image and targeting specific frequencies that correspond to noise. This frequency-based filtering approach can selectively remove noise while attempting to preserve important image details, ultimately resulting in cleaner images.
Maintaining a balance between sharpening the edges and reducing noise is a delicate process. Pushing noise reduction too far can lead to a loss of sharpness, blurring edges, and creating a less desirable appearance in your video. There’s a tricky interplay between these two adjustments that requires a skilled eye and a good understanding of the image characteristics in order to arrive at the best outcome.
If you're upscaling videos with clips that have inconsistent frame rates, it's worth ensuring they're all set to the same frame rate before starting the upscaling process. This can minimize the risk of encountering playback issues or choppy sections in the final output, preventing frustration during the export phase.
DaVinci Resolve's Super Scale provides valuable real-time feedback as you adjust the noise reduction and edge detail settings. The instant visual results give you a good idea of how your changes impact the image. This instantaneous feedback speeds up the entire process and helps you reach your desired results quickly. It’s interesting to wonder if there might be a way to get a more objective assessment of the quality improvements by incorporating some form of quantitative metric.
While the Neural Engine powering Super Scale utilizes advanced AI, the effectiveness of these AI models can vary depending on the specific nature of the content you are trying to enhance. Models trained on vast amounts of data, such as landscapes, may not perform as well with scenes involving quick motion or other elements. This highlights the dependence on how well the AI is trained for specific image content.
The upscaling process can be computationally intensive, impacting how long it takes to render your video, especially if you're working on larger projects or aiming for higher-resolution output. This means it’s important to consider the capabilities of your computer’s hardware to make sure it can handle the task in a reasonable amount of time.
One of the aspects that often becomes noticeable after upscaling is the appearance of compression artifacts from the original footage. This suggests that it's vital to start with the best-quality footage you can for the upscaling process to work most effectively.
Your selection of the export codec can either enhance or hinder the benefits you see from the upscaling process. The choice you make can significantly influence the quality of your exported 4K video and potentially undo some of the visual benefits that were achieved through Super Scale. Trying different codec options will help you find a balance between file size and quality.
Saving presets for your upscaling settings allows you to save your adjustments and use them as a starting point for other projects. This can help streamline the upscaling process, allowing you to focus on specific adjustments that might be needed for a particular project, or to simply carry your workflow forward from a prior session. It's useful for both efficiency and potentially comparative analysis during the research process.
While Super Scale offers a compelling path for upscaling 1080p to 4K, it's important to understand the nuances of the process and how the various controls can be used to achieve the best results possible. Continually experimenting with the different settings is a key element of exploring the capabilities and limitations of this tool for different image types.
How to Upscale 1080p to 4K Using DaVinci Resolve 185 Free Step-by-Step Super Scale Guide - Memory and Performance Management During 4K Upscaling
Upscaling 1080p footage to 4K using DaVinci Resolve's Super Scale feature introduces some interesting challenges related to how your computer's resources are used. Since Super Scale relies on sophisticated AI to create new pixel information, it can place a heavy demand on your computer's processor and memory. This can lead to longer processing times, especially when dealing with projects that contain a lot of footage or complex effects. How efficiently your computer can handle this process is vital to ensuring the upscaling process goes smoothly.
Managing your settings, and being mindful of how those settings interact with your system's performance, becomes especially crucial when dealing with the demands of 4K upscaling. Understanding how your computer's components are utilized during the Super Scale process is essential to avoid potential problems such as slow rendering or noticeable image artifacts. If you aren't careful, settings can lead to unexpected quality issues in your final 4K video. For the best results, consider the capabilities of your hardware when embarking on a 4K upscaling project. By understanding the interplay between your hardware and the AI algorithms driving Super Scale, you can make informed decisions that contribute to a more streamlined workflow and higher-quality final videos.
Delving into the inner workings of DaVinci Resolve's Super Scale feature reveals some intriguing aspects related to memory and performance during 4K upscaling. For instance, the amount of RAM needed can dramatically increase as the resolution being processed goes up. Upscaling from 1080p to 4K often calls for at least 16GB of RAM, though professionals frequently use 32GB or more to ensure a smooth experience.
Interestingly, the time it takes to upscale a video can vary quite a bit based on the complexity of the scene. Scenes with rapid movement or lots of intricate details tend to take considerably longer, sometimes even doubling the processing time compared to relatively static scenes. This really emphasizes the importance of having a good understanding of your project before you start.
Another fascinating aspect is how both the GPU and the CPU contribute to the performance of the upscaling process. While GPUs are crucial for the visual aspects and optimizing how things look in real-time, the CPU still has a significant role to play in managing memory and handling various processing tasks. If either of these components is insufficient for the workload, it can create performance issues.
It's worth mentioning that Super Scale doesn't magically improve the quality of any imperfections that already exist in the original 1080p video. Compression artifacts that might be in the original footage can become more prominent after the upscaling due to the way the resolution is being changed. This means the flaws are potentially more noticeable rather than hidden by the process.
Furthermore, fast disk access speeds are often crucial for handling the data involved in upscaling 4K video. The constant reading and writing of large files can benefit greatly from using SSDs instead of traditional hard drives. SSDs significantly reduce read/write times, improving the efficiency of the whole workflow.
DaVinci Resolve has a caching system to help with previews and render times. By configuring these cache settings appropriately, we can potentially reduce memory pressure and get quicker feedback while using Super Scale. However, if errors exist in any clips that are upscaled, there's a chance they can spread throughout the editing process. The scaling process can amplify discrepancies in color and luminance, leading to issues that require meticulous attention during the editing stage.
Working with clips that have different frame rates can further complicate things. When mismatched frame rates are processed during upscaling, it might require the software to use more processing resources to ensure everything plays smoothly. This can potentially lead to slower workflows.
One helpful feature is the dynamic resolution adjustments built into DaVinci Resolve. This allows users to modify resolution settings within the project timeline, providing a means to manage resource allocation and potentially reduce memory strain when using multiple high-resolution files. Additionally, saving presets for Super Scale adjustments can streamline performance because the system doesn't have to recalibrate settings for every clip. This type of optimization can help free up memory for the upscaling process itself.
Understanding these aspects of memory and performance management during the upscaling process with Super Scale provides a more complete understanding of how DaVinci Resolve functions. This insight can help optimize workflows and ensure a smooth and efficient experience. By understanding the potential limitations and the factors that affect performance, users can potentially optimize their editing process for the best possible results.
Upscale any video of any resolution to 4K with AI. (Get started for free)
More Posts from ai-videoupscale.com: