Upscale any video of any resolution to 4K with AI. (Get started for free)
New AI Upscaling Techniques for High-Quality Video Playback in 2024
New AI Upscaling Techniques for High-Quality Video Playback in 2024 - AI-Powered Resolution Enhancement Techniques in 2024
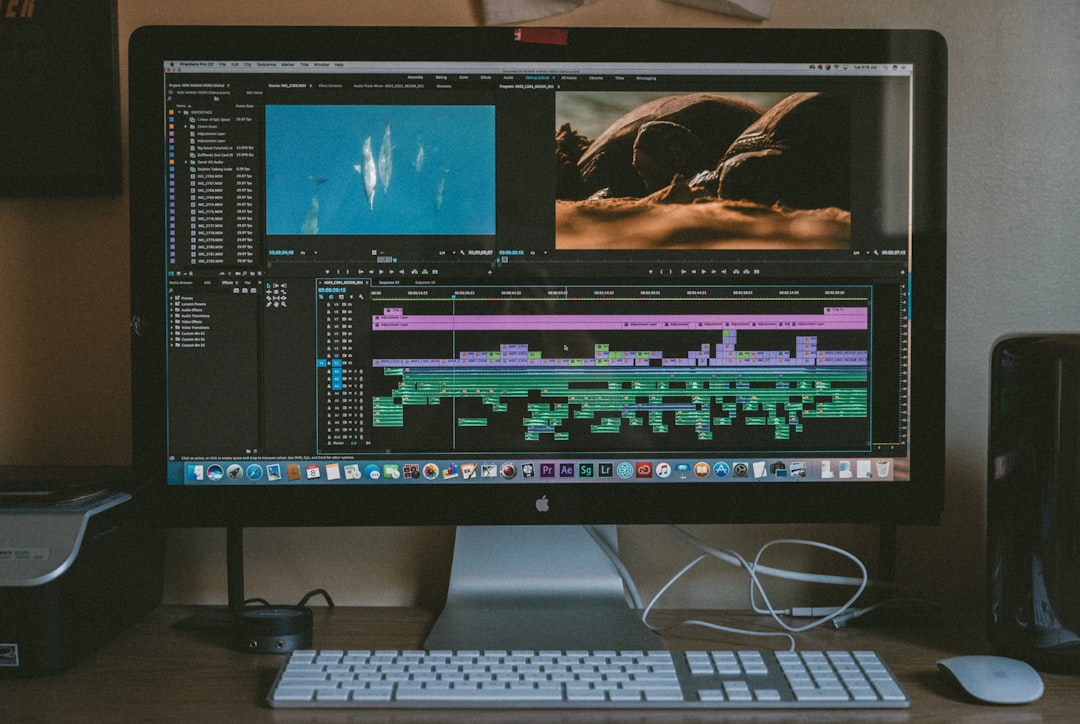
The landscape of AI-driven resolution enhancement has evolved rapidly this year, showcasing impressive advancements in upscaling video content. Tools are now able to upscale video to resolutions as high as 8K, a feat previously unimaginable. Techniques within these AI upscalers, such as those employed by VideoProc Converter AI and TensorPix, effectively minimize visual artifacts and smooth out motion blur while achieving these higher resolutions. This has opened doors for improving the quality of older video formats, particularly home movies, where Topaz Video Enhance AI has shown promising results.
Beyond just boosting resolution, these technologies have become more user-friendly. Features like batch processing make upscaling multiple videos much faster and easier. Yet, this rapid development also begs the question of the tradeoffs between artificial enhancements and the integrity of the original content. While the results can be impressive, discerning the difference between genuine detail and AI-generated enhancements remains a point of discussion in the field.
The field of AI-driven video resolution enhancement has seen a surge of practical applications in 2024. Several readily available tools now leverage AI to upscale videos, primarily focusing on increasing resolution by factors of 2x, 4x, or even 8x. Services like TensorPix and CapCut offer online upscaling, showcasing how cloud-based processing with GPUs can speed up the process. Products like VideoProc Converter AI demonstrate the ability to drastically enhance older, low-resolution content, effectively bridging the gap between standard-definition and 4K or even 8K playback. Software like Topaz Video Enhance AI takes a more sophisticated approach, applying techniques to reduce motion blur and artifacts, particularly beneficial for restoring the clarity of older home videos.
The trend towards user-friendly interfaces is undeniable. Most current tools are designed for ease of use, allowing users to easily upload, process, and download batches of enhanced videos. Movavi's inclusion of AI upscaling in their 2022 release demonstrates a broader movement within video editing software towards incorporating these features. Pixop, while not as widely known, has also contributed to the field by focusing on high-quality upscaling outcomes.
While much of the focus is on resolution enhancement, some tools, such as Topaz Video AI, venture beyond simply upscaling. Their features demonstrating frame-rate conversion (e.g., 24 fps to 60 fps) highlight that these AI techniques can also enhance the cinematic experience by creating smoother visuals and reducing camera shake. It remains to be seen if this intersection of AI-driven video manipulation and cinematic enhancement will lead to further exploration and potential innovation. The practicality and accessibility of these technologies in both professional and casual use cases is notable. It is certainly a space to watch for future developments.
New AI Upscaling Techniques for High-Quality Video Playback in 2024 - Frame Rate Interpolation Advancements for Smoother Playback
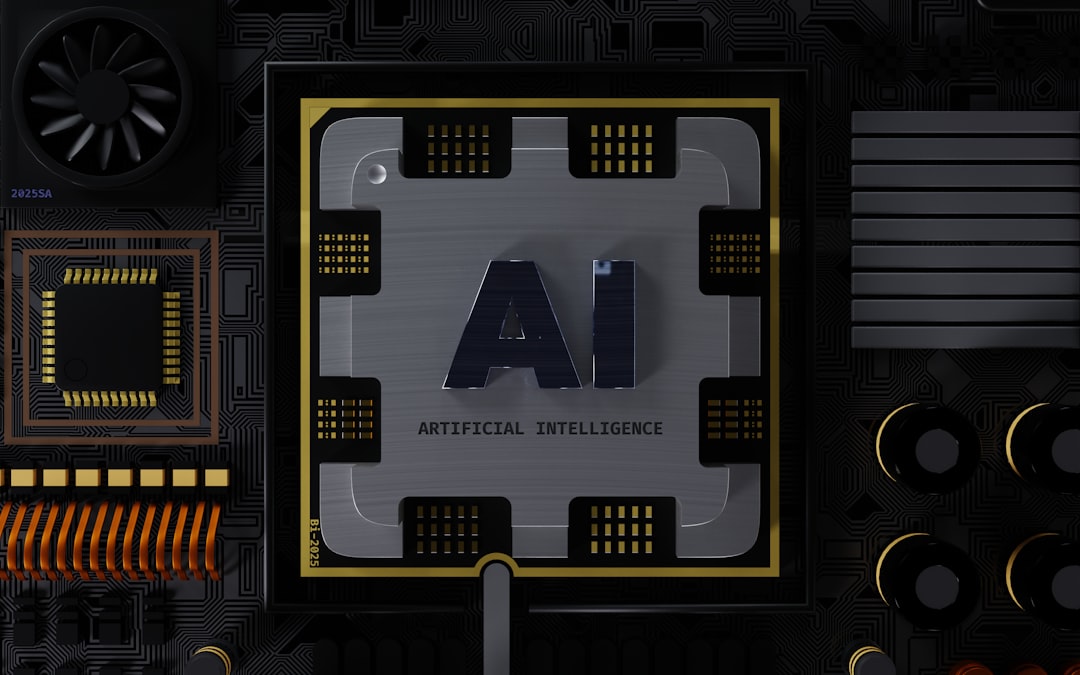
The field of video playback is seeing a significant shift with the integration of advanced frame rate interpolation techniques. AI is now at the forefront of this change, enabling tools to generate artificial frames within a video sequence. This results in a smoother visual experience, especially noticeable when upscaling from lower frame rates like 24 frames per second (fps) to higher rates, such as 60 fps. The impact is particularly evident in action-packed content where it minimizes the appearance of motion blur. This smoother, more fluid playback translates into a heightened sense of immersion for the viewer.
While the improvements are undeniably beneficial, concerns linger about the fine line between enhancing the visuals and manipulating the original content. The implications for the future of cinematic experiences are being debated, particularly regarding the potential for an over-reliance on artificial enhancement and the possible loss of authenticity in the original footage. As these technologies continue to evolve, it will be vital to understand how they affect our perception of film and video.
AI-driven frame rate interpolation is transforming how we experience video by enhancing the smoothness of motion. It works by creating new frames in a video sequence, effectively increasing the frame rate. This can take a video originally captured at 24 frames per second (fps) and make it appear as if it were shot at 60 fps, leading to a much smoother visual flow.
The algorithms behind this are becoming increasingly sophisticated. Techniques like optical flow are used to analyze motion patterns within the video and predict where objects will be in the next frame, enabling the creation of believable, in-between frames. The integration of machine learning further refines this process. AI models trained on massive datasets are learning to understand motion patterns more accurately, producing higher quality interpolated frames that are closer to how the real-world motion would appear.
Of course, evaluating the quality of these synthetic frames is important. Metrics like PSNR and SSIM can help measure how well the interpolated frames match the original video, giving us a quantitative sense of any artifacts introduced by the AI upscaling. However, the pursuit of smoothness comes with computational challenges. Real-time frame rate interpolation is demanding, requiring powerful processing hardware to handle the workload without introducing noticeable delays.
The impact of frame rate interpolation is already evident in several areas. Broadcasting sports, for instance, benefits tremendously as viewers can more easily track fast-moving objects and unpredictable camera angles without distracting blurring. This trend is also reflected in consumer demand for smoother playback, with more TVs and streaming services embracing frame rate interpolation. But, we need to be wary of potential pitfalls. While reducing motion blur is the goal, interpolation can introduce artifacts like ghosting if not implemented carefully.
Thankfully, it also allows older video formats, recorded at lower frame rates, to be experienced at higher frame rates and resolutions, potentially helping preserve and revive a vast library of older content. Looking ahead, live streaming could become a primary beneficiary. Imagine a future where online events are smoothly broadcasted with a visual quality that matches traditional broadcasts. The possibility of AI-enhanced live streams raises exciting questions about how we will interact with moving images online. There's a clear trend towards smoother and more engaging video experiences, and frame rate interpolation is playing a major role in achieving this goal.
New AI Upscaling Techniques for High-Quality Video Playback in 2024 - Real-Time Upscaling Solutions for Streaming Platforms
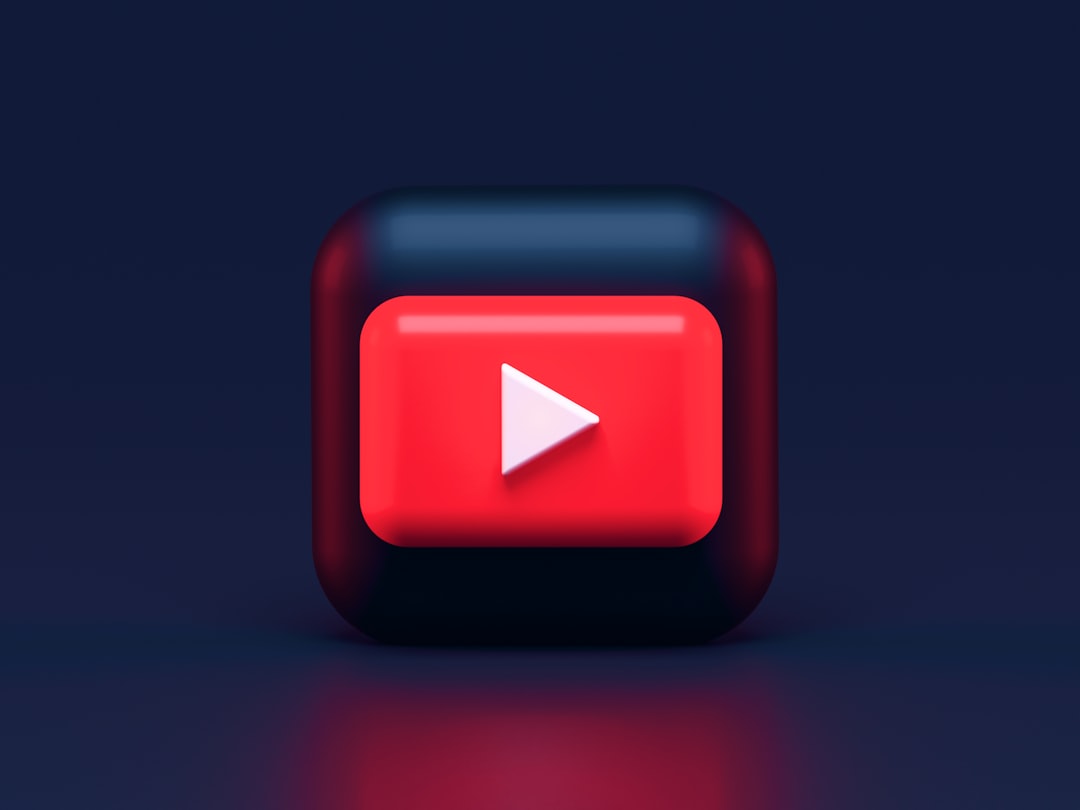
The demand for high-quality video streaming is driving the development of real-time upscaling solutions. Streaming services are increasingly under pressure to deliver sharp, detailed video, and AI-powered upscaling is emerging as a key technology to achieve this. Services like TensorPix demonstrate how cloud-based processing, combined with powerful GPUs, can efficiently upscale lower-resolution videos into higher-resolution formats, like 4K. Tools such as VideoProc Converter AI excel at converting standard-definition or low-quality videos into significantly more appealing 4K versions, significantly enhancing older content. Additionally, some tools, such as Topaz Video Enhance AI, are tackling problems beyond just resolution boosts. They can help restore older video by removing motion blur and artifacts, opening the door to enhanced playback of home movies and historical footage.
This surge in AI-based upscaling tools raises interesting questions about the relationship between visual enhancement and preserving the integrity of original footage. The line between enhancing viewing pleasure and artificial manipulation of video content remains a topic of debate. The rapid evolution of these upscaling technologies highlights the complex landscape of AI's growing influence on the way we view and consume streaming content.
Streaming services are increasingly relying on real-time upscaling to provide a better viewing experience. These solutions have become quite efficient thanks to the power of modern GPUs, which allow for the on-the-fly processing of video without noticeable delays. This is a big change from older methods that needed pre-processing.
There's growing evidence that upscaling can actually improve the *perceived* quality of video, beyond just the numerical resolution. Viewers often find that upscaled content appears sharper and more detailed, suggesting that these AI algorithms might be creating information that wasn't originally there. This challenges how we traditionally measure video quality.
Many current AI upscaling methods make use of convolutional neural networks (CNNs). These networks are trained on massive datasets of images, allowing them to learn how to reconstruct details that were lost in the original, lower resolution source.
Some upscaling techniques are using a hybrid approach, combining AI with more traditional image processing methods. This combination can not only enhance the resolution but also things like color accuracy and contrast, leading to a richer visual outcome.
Interestingly, some advanced upscaling solutions are becoming adaptive to the type of content they are processing. For instance, an algorithm might upscale animation differently from a live action movie. This suggests these systems are becoming more sophisticated in how they approach visual styles.
However, upscaling isn't without its potential issues. These algorithms can sometimes introduce noticeable artifacts, like bright edges or ringing effects. Finding a balance between enhancing the visuals and preserving the original content integrity requires careful tuning of the algorithms.
From a practical standpoint, real-time upscaling can allow streaming platforms to deliver lower resolution content and then have it upscale on the user's device. This can help save bandwidth and improve accessibility while still providing a high quality viewing experience.
The upscaling process is influenced by the quality of the source material. Upscaling very low quality or compressed videos might not produce satisfactory results. Therefore, the original quality of the video you're trying to upscale is important for the outcome.
We're also starting to see user feedback mechanisms in some of these tools. Viewers can now provide direct feedback on the upscaling quality. This feedback loop could help refine the algorithms over time, and adapt to the specific preferences of users.
There's also a growing discussion about the ethical implications of upscaling, especially when it comes to older videos or archival footage. With the ability to dramatically alter visual quality, we have to consider how this affects the authenticity and original meaning of content. This is something that we'll need to consider as upscaling technology continues to advance.
New AI Upscaling Techniques for High-Quality Video Playback in 2024 - Neural Network-Based Texture Reconstruction in Video Processing
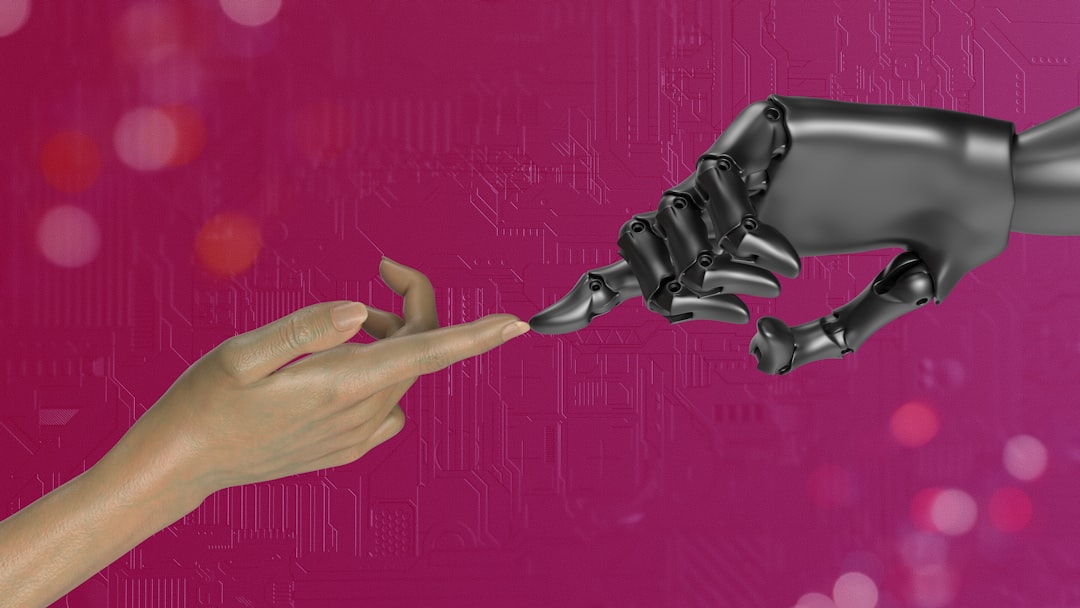
Neural networks are playing an increasingly important role in video processing, especially in the context of upscaling. Their ability to reconstruct textures is crucial for generating high-quality, detailed video from lower-resolution sources. This is particularly relevant in 2024 as AI-driven upscaling techniques continue to mature. Neural networks can now recreate fine details and intricate patterns within video frames, effectively bridging the gap between low and high resolutions.
Furthermore, recent developments in this area focus on handling complex video content. New methods address long videos with dynamic scenes, like those with frequent shifts in location or action, by intelligently processing the spatial and temporal characteristics of the footage. This addresses a significant challenge in upscaling, as older methods often struggled with preserving visual consistency during such changes. Another promising development involves refining how videos use reference frames during upscaling. Techniques like reference-based super-resolution provide more flexibility in aligning textures, thereby achieving smoother visual transitions in the output.
However, as with any technology that manipulates visual content, concerns remain about balancing enhanced visual fidelity with the integrity of the original video. While neural networks can generate compelling detail, it's important to consider the implications of these enhancements. The industry is actively navigating the complexities of this issue, striving to ensure that upscaled videos enhance the viewing experience without sacrificing the fundamental character of the source footage. Striking this balance will likely remain a key area of exploration and debate in the evolving field of AI video enhancement.
Neural network-based texture reconstruction has become a key player in video processing, particularly in improving video resolution and quality. These techniques, often utilizing powerful neural networks like Generative Adversarial Networks (GANs), are capable of rebuilding intricate details in real-time, a remarkable feat considering the computational intensity involved. However, the effectiveness of these networks is deeply connected to the quality and variety of the datasets they are trained on. For instance, a neural network trained primarily on action movies might excel at upscaling action scenes but struggle with other genres like animation. This points to the critical role of careful dataset selection in determining model performance.
Interestingly, some AI systems for texture reconstruction can analyze the video content in real-time and adapt their upscaling approach accordingly. For example, these systems might choose different upscaling strategies for static backgrounds versus rapidly moving objects, resulting in a more refined and suitable enhancement. Additionally, these networks can be leveraged to recover details that were lost during the compression of a video. This suggests that some of the negative effects of lossy compression, like a decrease in visual clarity, can be partially mitigated through the application of these AI models.
Despite these advancements, there's a growing discussion about whether the textures produced by AI are "genuine" or artificially generated. It's often hard for viewers to determine the difference between real details and those created by the AI, raising ethical concerns about how these techniques might be used in media production. Although neural networks can create incredibly realistic textures, they are not flawless. They can still produce artifacts like ghosting or blurring, and ongoing research focuses on improving models to reduce these unwanted side-effects while preserving crucial details.
Some research has shown promising results using neural style transfer in texture reconstruction, which basically allows merging the content of one video with the stylistic characteristics of another. While this offers exciting possibilities for enhancing aesthetics, it also introduces complex questions related to manipulating creative content. Achieving top-notch texture reconstruction requires substantial computing power, often relying on powerful GPUs. This leads to a trade-off between visual quality and computational cost, demanding constant optimization and the development of faster hardware.
When reconstructing textures in video, temporal coherence is essential. The neural networks need to consider how the textures change over time to avoid flickering artifacts between frames. Newer algorithms often leverage past frames to predict how textures should look in the current frame, ensuring a smooth and consistent visual experience. Some upscaling tools now incorporate user feedback mechanisms, allowing adjustments based on viewer preferences. This ability to incorporate real-time feedback and adjust the upscaling process could lead to more customized and refined techniques over time.
New AI Upscaling Techniques for High-Quality Video Playback in 2024 - Adaptive Upscaling Algorithms for Varying Content Types
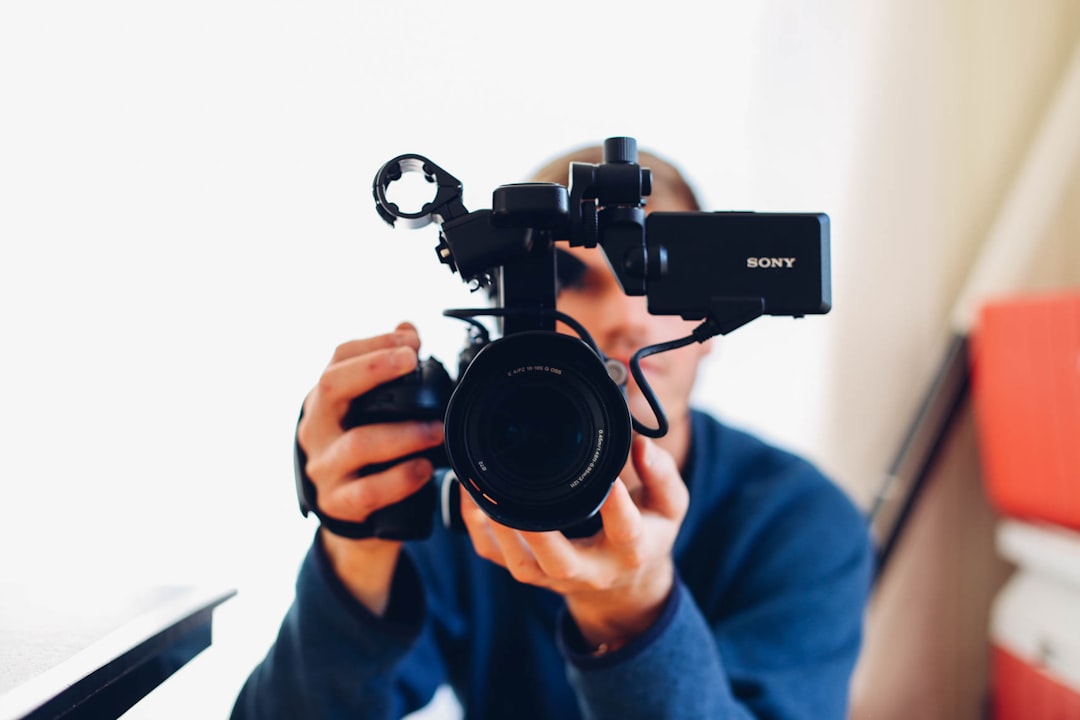
In 2024, AI upscaling algorithms are evolving to become more adaptive to the nuances of different content types. These algorithms are becoming more adept at analyzing the unique characteristics of images and videos, whether it's a photograph, an animated sequence, or a live-action film. This adaptability leads to upscaling that's not just about increasing resolution, but also about preserving specific textures and maintaining sharp edges in a way that respects the original content's stylistic intent. The results can be impressive, leading to a more nuanced and visually pleasing experience.
However, this sophistication comes with a caveat: there is always the possibility that adaptive upscaling introduces artifacts or unintended changes to the visual style of the original material. The delicate balance between pushing visual quality and respecting the character of the original content is a challenge that continues to be explored by developers in this area. As these techniques become even more sophisticated, it will be crucial to ensure that enhancements are both impactful and respectful of the content being manipulated.
AI upscaling techniques are becoming increasingly sophisticated, adapting to the diverse types of content found in videos. One exciting area of development is the use of algorithms that can intelligently adjust their approach based on what's being upscaled. For instance, an algorithm might handle a still landscape shot differently than a fast-paced action sequence, aiming to optimize detail and avoid artifacts specific to each. This "content-aware" approach allows for more fine-grained control over the upscaling process.
These adaptive algorithms can also make real-time changes during playback. As a scene shifts from dark to light, or as camera movement increases, the upscaler can automatically adjust its parameters to maintain a consistent level of quality. This dynamic nature helps keep the output looking sharp and detailed, even in complex visual situations.
Many of these techniques rely heavily on deep learning models. These models are trained on a large variety of content, including live-action videos, animations, and even text overlays. The AI essentially learns to identify the unique characteristics of different content types, and then applies the most effective upscaling techniques for each one. This allows the algorithm to better preserve texture and fine detail based on the source material.
Another area of focus is how algorithms can intelligently leverage reference frames. Instead of simply upscaling every frame independently, advanced techniques look at related frames to guide the process. This is particularly important in action scenes or fast cuts, as it can help to create smoother visual transitions. The goal is to minimize issues like blurring and flickering that can occur during abrupt changes in movement.
However, maintaining visual consistency over time, something we call temporal coherence, remains a challenge. Adaptive algorithms strive to keep the texture and appearance of upscaled objects constant across frames, reducing visual distractions. Some algorithms even let users customize upscaling strategies based on the type of content. This opens up possibilities for filmmakers to emphasize specific scenes or optimize for faster processing when necessary.
Additionally, newer upscaling methods go beyond standard RGB color data, using multispectral analysis. This involves considering other aspects of the image like luminance and chrominance separately, which can lead to better color accuracy and fidelity. There's also promise in the ability to repair some of the visual damage that occurs when video is compressed. By recognizing the typical patterns of compression artifacts, algorithms can sometimes recreate missing pixel data to help improve the overall image.
It's also interesting to see upscalers incorporating metrics related to human visual perception. This means that the upscaling process isn't just guided by technical error calculations, but also by how humans perceive differences in image quality. This can lead to more satisfying visual outcomes.
On the flip side, a critical concern with adaptive upscaling is the possibility of algorithmic biases. If an algorithm is primarily trained on certain genres, it may not work as well on others. This suggests that the training datasets used to develop these algorithms are very important. Ensuring that upscalers can handle the diverse range of video content we encounter today will require careful attention to these potential biases. As we continue to see improvements in these AI techniques, balancing the potential benefits with the challenges they present will be an important ongoing discussion.
New AI Upscaling Techniques for High-Quality Video Playback in 2024 - Edge Computing Integration for Faster AI Video Upscaling
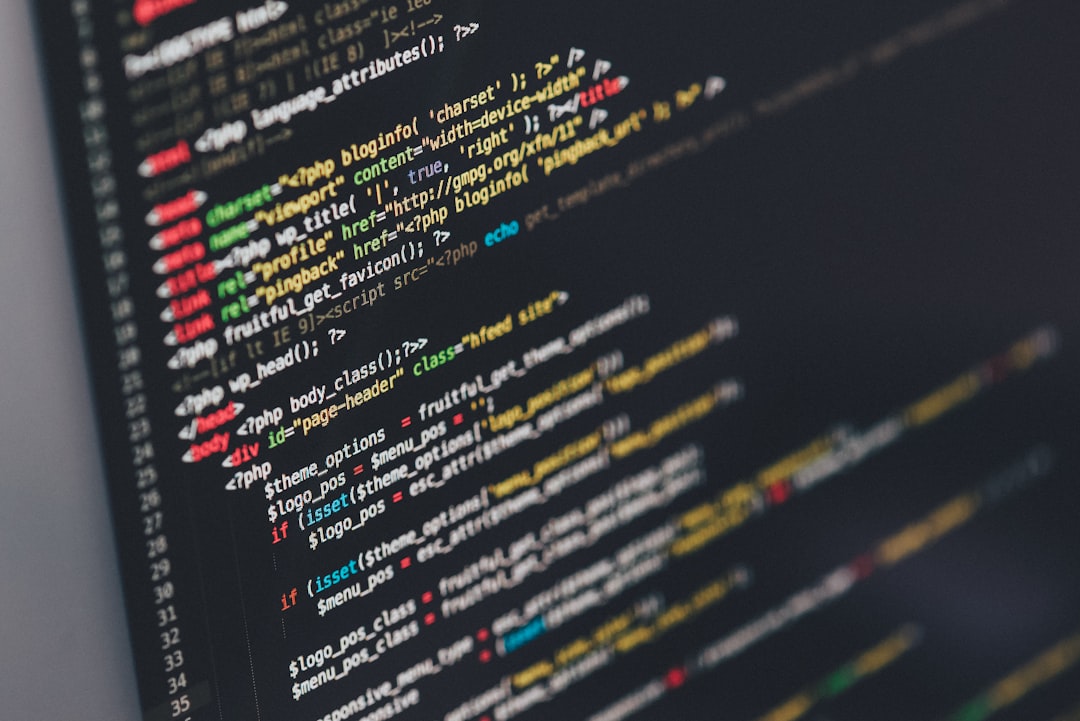
The integration of edge computing is bringing about a notable shift in the speed and efficiency of AI-driven video upscaling. By placing computing power closer to where the video is being viewed, edge computing can minimize the delays inherent in sending video data to remote servers for upscaling. This means faster processing times and smoother playback, particularly for real-time applications.
We're starting to see examples of this in action, such as the AI-based video upscaling features built into web browsers like Microsoft Edge. These features use AI and machine learning to effectively increase video resolution, but they do so while taking advantage of the processing power found on your computer's graphics card. This approach can lessen the burden on cloud services and reduce the time needed to upscale videos, thereby resulting in a more fluid viewing experience.
However, it's crucial to consider the implications of pushing processing to the edge. One of the challenges is carefully balancing the benefits of enhanced visual quality with the need to maintain the integrity of the source video. This delicate dance between improving the look of videos and preserving the original nature of the footage is a critical consideration as these upscaling technologies mature. We can expect more fine-tuning of the algorithms used in edge computing upscalers to help address this.
In the broader picture, the marriage of edge computing and AI video upscaling is enabling wider access to high-quality video content. It makes higher resolution and smoother video potentially available in a variety of contexts – a trend that could reshape how we consume and enjoy video moving forward.
Integrating edge computing into AI video upscaling presents a compelling path forward, especially for real-time applications. By moving processing closer to the source of the video, we can potentially minimize the delays often associated with cloud-based AI upscaling. This reduction in latency is particularly important for experiences where real-time response is vital, like live sporting events or interactive video streams. It would be fascinating to see if this approach enables smooth 4K or 8K streaming in situations where that wasn't previously feasible due to the limitations of network infrastructure.
Another intriguing aspect of edge computing is its potential to enhance privacy. Processing videos closer to the device generating them limits the amount of data that needs to be transferred to remote servers. This could be a significant benefit in contexts where the content being upscaled might involve sensitive information. However, it remains to be seen how effective this approach truly is for preserving user privacy, particularly with the continued growth and integration of data across multiple systems.
One notable advantage is the ability to allocate processing resources more dynamically. Rather than relying on a fixed set of resources in a central location, we can adjust the level of processing power as needed. This could be invaluable for handling a wide variety of video content, adapting to changes in resolution, and ensuring that the system doesn't become overloaded. But, how exactly the performance gains would manifest in real-world situations is still an area that requires further exploration.
Edge computing's efficiency in utilizing bandwidth is also worth exploring. It seems plausible that by minimizing the amount of data that needs to be sent over the network, we can improve network performance for users, especially those dealing with limited bandwidth or inconsistent network connectivity. While this may help the video experience, it's vital to ensure the upscaling quality isn't compromised in exchange for bandwidth efficiency. There's a fine balance to strike.
Another possibility is that integrating local knowledge into the upscaling process could help preserve specific aspects of the video, such as its color grading or style. This would be especially helpful in enhancing older video formats that have a unique visual character. It remains unclear, however, whether this type of local adaptation would introduce unwanted artifacts or bias into the output.
The potential for scalability across different devices is also attractive. With edge computing, we could conceivably deliver AI-enhanced video across a wide range of platforms and devices, leading to a more consistent experience. It would be interesting to explore if this approach would make it more feasible to provide high-quality upscaling on mobile devices with limited processing power, for example.
From a cost standpoint, deploying AI upscaling on edge devices could reduce the reliance on expensive data centers, potentially decreasing the overall operational expenses associated with video processing. It's intriguing to speculate how cost-effective this would be in practice, considering the need to deploy edge nodes with sufficient processing power.
Furthermore, the development of new machine-learning protocols designed for edge computing has the potential to streamline the deployment of AI models in this space. These new protocols may make it much easier to apply complex AI techniques to upscaling video content at the edge, leading to potentially wider adoption of this approach.
Moreover, edge computing seems to offer an opportunity to allow AI algorithms to better respond to dynamic changes in video conditions, such as sudden shifts in lighting or rapid movement. Adapting the upscaling in real-time could lead to a higher-quality experience compared to static algorithms. However, this approach will likely come with new challenges of complexity and algorithm design.
Finally, training AI models using localized data could potentially lead to upscaling results that are more relevant to specific communities and demographics. This potentially enables the personalization of video processing in ways that were previously unthinkable. Yet, concerns about potential bias and fairness in training data still remain.
In conclusion, the integration of edge computing into AI video upscaling presents a number of exciting potential benefits, from improved latency and user privacy to increased scalability and reduced costs. It is likely that this field will witness significant advancements in the near future, although further investigation is needed to address the challenges and fully realize these benefits.
Upscale any video of any resolution to 4K with AI. (Get started for free)
More Posts from ai-videoupscale.com: