Upscale any video of any resolution to 4K with AI. (Get started for free)
Optimizing HandBrake's Batch Conversion for AI Video Upscaling Workflows
Optimizing HandBrake's Batch Conversion for AI Video Upscaling Workflows - Configuring HandBrake's Output Settings for AI Upscaling
When integrating HandBrake into AI upscaling workflows, correctly configuring its output settings is paramount for maximizing video quality. Fine-tuning settings like resolution, bitrate, and file format can significantly influence the outcome of the upscaling process. The ability of HandBrake to handle batch conversions is particularly useful for AI-driven projects involving multiple videos. This speeds up the workflow for large-scale upscaling tasks.
While some dedicated AI upscalers offer advanced features, it's worth remembering that other tools, including free online upscalers and comprehensive software like DaVinci Resolve, also have useful capabilities for upscaling. However, their performance and features can vary widely. By correctly configuring HandBrake's output, you can ensure a smooth transition to your chosen AI upscaler and generate high-quality, upscaled videos. This is particularly important as it can impact the results of your post-processing steps in the upscaling workflow. There is a delicate interplay between HandBrake's role and the nature of the specific AI upscaling tool in use.
When preparing videos for AI upscaling with HandBrake, you have a lot of control over the output. You can tweak things like resolution, frame rate, and bitrate through different presets to get a balance between quality and file size that works for your needs. For instance, choosing the right encoder can dramatically affect the outcome. H.265 tends to compress better than H.264, offering a potential 50% reduction in file size without noticeable visual loss – which is generally beneficial for AI upscaling.
HandBrake's 'constant quality' setting, rather than fixed bitrate, can be important for upscaling as it provides more consistent quality in the source material. This consistency can be important for how the AI upscale algorithms work. Using certain container formats that support 10-bit color, you can maintain better color reproduction. This can be crucial for AI upscalers that rely on richer color information.
HandBrake's preview feature is helpful since you can test changes in real time and see the impact on the video before starting a large batch conversion. This iterative approach can really optimize your workflow. The audio codec choice is also something to consider for both quality and file size. Options like AAC or Opus might be preferable to MP3, especially if the audio is crucial to the final video.
Furthermore, you can use HandBrake's filtering capabilities to clean up the video before sending it off to the AI upscaler. Things like deinterlacing or noise reduction can significantly improve the final upscaled output, as they tackle visual issues that AI upscaling might amplify. Batch conversion is also a time saver that ensures consistency in output settings across multiple files. While HandBrake itself can offer a lot of control, you can always enhance the output with external filters or software, which can give you even more nuanced adjustments and potentially produce better results.
Optimizing HandBrake's Batch Conversion for AI Video Upscaling Workflows - Optimizing Video Codec Selection in HandBrake
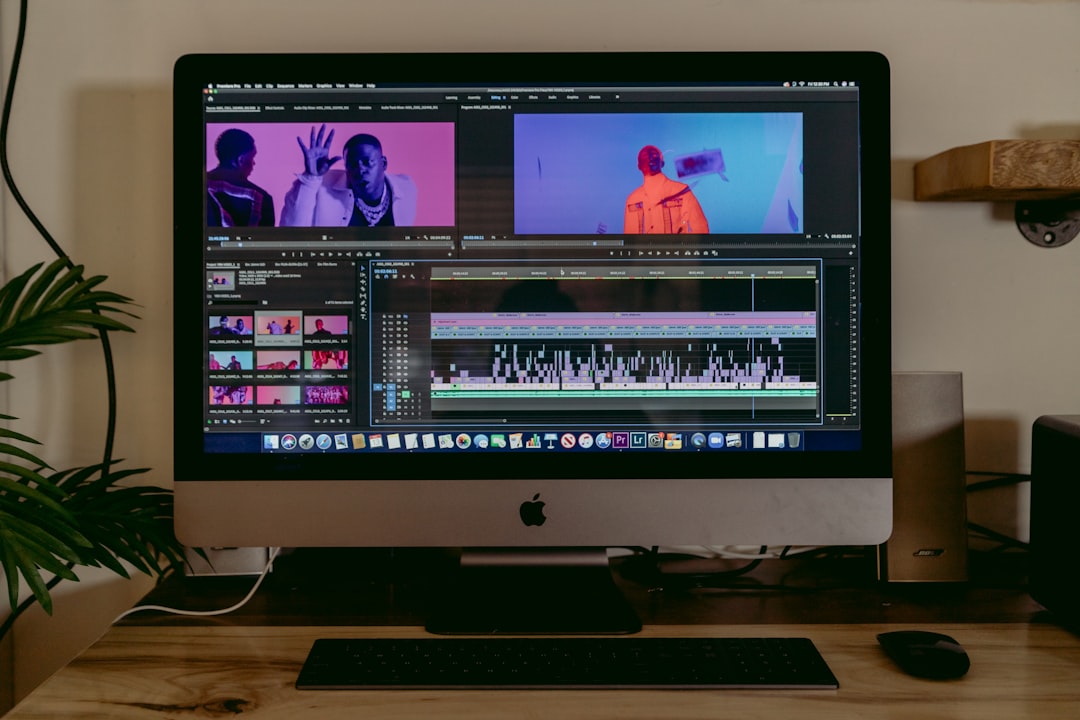
When preparing videos for AI upscaling using HandBrake, the choice of video codec plays a key role in determining the final output quality and efficiency. H.265 (HEVC) is often a good choice due to its ability to achieve high compression ratios, leading to smaller file sizes without sacrificing visual clarity. This is particularly helpful for AI upscaling workflows where large file sizes can be a bottleneck.
For high-quality video conversion, using the Apple TV 2160p60 4K HEVC Surround preset within HandBrake is a versatile starting point. It's also helpful to use the x265 codec with a CRF value of around 24. This helps balance quality and file size. It's important to keep in mind that maintaining video quality during the initial encoding stages is essential because upscaling can amplify existing compression artifacts.
HandBrake also offers deblocking options, which can be adjusted to help control how sharp or smooth the final image is. The goal is to retain sharpness while preventing unwanted artifacts that might arise from the conversion or upscaling process. By thoughtfully selecting a codec and adjusting settings like CRF and deblocking, you can maximize the quality of the video going into the AI upscaling stage, resulting in a more successful and visually appealing final result.
HandBrake's efficiency with the H.265 codec, also known as HEVC, is a key feature for AI video upscaling workflows. Its ability to compress video to roughly half the size of H.264 at the same quality level can be critical when dealing with large volumes of video data. This is especially true for situations where storage space and bandwidth are limited, which is often the case with AI upscaling projects.
When considering color, encoding videos with a 10-bit color depth can have a noticeable impact on the quality of upscaled results. This expanded range of color helps ensure the AI upscalers have access to more nuanced color information, enabling them to perform a more effective enhancement.
If preserving the original video quality is the utmost priority, HandBrake can be utilized with lossless codecs. This can be beneficial for AI upscaling, as it ensures the AI algorithms are working with a pristine, detail-rich source.
Beyond the codec choice, the container format plays a role in compatibility with AI upscalers. Formats like MKV can accommodate advanced codecs and features like 10-bit color. However, MP4 is a more universally supported format but might not work with all upscaling tools due to lacking some functionalities.
HandBrake's 'constant quality' setting is preferred to a fixed bitrate setting when it comes to upscaling. It helps to maintain a consistent quality level throughout the video, which can contribute to more predictable outcomes when the AI upscaling algorithms are applied. The upscalers seem to benefit from a steadier input source.
The audio codec choice matters in a similar way to video. More modern codecs like AAC and Opus can deliver a quality-to-file size ratio that might be better than the older MP3 format, especially when the audio is crucial to the final video experience.
HandBrake's filtering capabilities can improve the video prior to upscaling. Features like denoising and deinterlacing can address potential video artifacts that can hinder the upscaling process. Addressing these artifacts before the AI sees them leads to a cleaner, smoother, and more refined final result.
Testing settings with HandBrake's preview feature before running large-scale batch conversions is a useful technique. It allows a researcher or engineer to quickly see if the settings chosen are generating the desired quality level, which can be a valuable time saver and resource optimization strategy.
While faster encoding presets in HandBrake speed up batch conversions, it's important to recognize that they might lead to quality loss. Striking a balance between encoding speed and quality is vital for ensuring the results of AI upscaling are optimal.
Finally, carefully selecting the output resolution in HandBrake can minimize the work needed by the AI upscaler. If you're starting with a video that already has a high resolution, upscaling it further might be an unnecessary step that increases processing time without yielding a noticeable quality increase.
Optimizing HandBrake's Batch Conversion for AI Video Upscaling Workflows - Adjusting Frame Rate and Resolution for AI Processing
When using HandBrake to prepare videos for AI upscaling, adjusting the frame rate and resolution is essential for achieving optimal results. These settings, found within the "Video" tab, directly impact both the quality of the upscaled video and the efficiency of the AI processing. For example, increasing the frame rate can improve motion smoothness, while adjusting the resolution allows the AI to handle the video input without excessive demands. However, overly aggressive adjustments can lead to diminishing returns in quality relative to the time spent processing. The key is to find a balance that works best for the specific AI upscaling tools and desired outcome. By carefully fine-tuning these settings, you can enhance the quality of the final upscaled video and maximize the benefit of integrating HandBrake into your AI workflow.
When preparing videos for AI upscaling with HandBrake, adjusting frame rates and resolution can significantly impact how well the AI algorithms perform. While higher frame rates give the AI more data to work with, potentially leading to smoother transitions and better recognition of elements within each frame, simply cranking up the resolution isn't always a win. Sometimes, excessive resolution can bog down the AI process, especially if the upscaler isn't designed to handle a very high pixel count. This can lead to wasted processing time without much visible improvement in output quality.
Videos that are interlaced can present challenges for AI upscalers. HandBrake's ability to convert them into progressive scan format can really help, as AI upscalers tend to perform better on progressive videos, minimizing the impact of interlacing artifacts. There's a similar consideration with bitrate. It's easy to think that a higher bitrate is always better, but for AI upscaling, finding a balance between bitrate and resolution can lead to a better outcome in many cases. Pushing the bitrate too high might strain the AI algorithms needlessly.
The frequency of keyframes can play a surprising role in the AI upscaling process. More frequent keyframes can help the AI recognize scene changes better, which could result in improved quality in the final output. Likewise, temporal resolution - the number of frames analyzed over a specific time period - might be even more impactful than spatial resolution in some cases. Ensuring that the right number of frames are selected for processing can be a key optimization step.
It's important to note that the field order within interlaced frames can mess things up if it's not handled correctly. A misaligned field order can mislead the AI into misinterpreting movement in the video, resulting in artifacts that hurt the overall quality of the upscaled video. For videos with low frame rates, there's a technique that can be beneficial. Duplicating frames during preprocessing can lead to a smoother, more refined output. This approach essentially provides more input data to the AI, which can translate into a smoother, more fluid final video.
One advantage of using a higher color depth in HandBrake before AI upscaling is that it provides the upscaling algorithm with richer color information. Using something like 10-bit color can enable more nuanced adjustments, leading to improvements like a reduction in banding issues that can arise with color gradients.
HandBrake's real-time preview feature is a helpful tool for experimenting with encoding settings, but it's important to acknowledge that it relies on lower-priority system resources. This can have an impact on overall processing speed, so it's a tradeoff you have to be aware of when you're trying to optimize your workflow. This is especially true when you need to quickly iterate through different encoding settings before you launch a massive batch conversion.
Optimizing HandBrake's Batch Conversion for AI Video Upscaling Workflows - Managing Audio Tracks and Subtitles in Batch Conversion
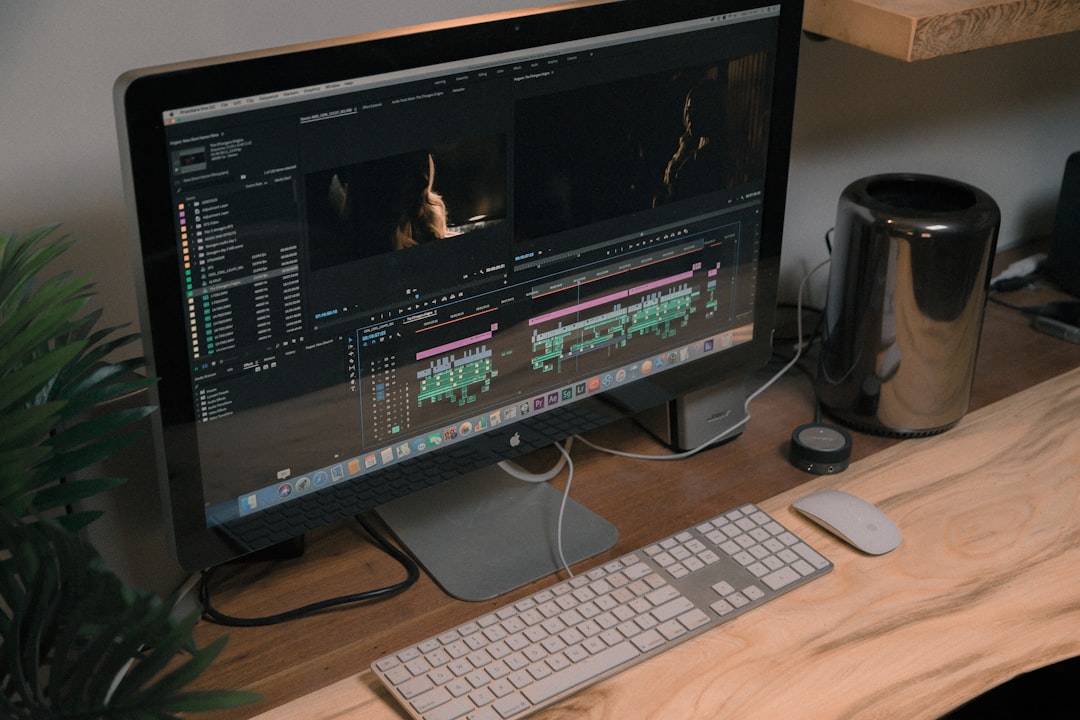
When optimizing HandBrake's batch conversion process, especially for AI video upscaling, carefully managing audio tracks and subtitles becomes crucial to get the desired output. You can simplify this by creating custom presets that keep only the specific audio track you want and remove any unwanted subtitles. HandBrake also has a "Selection Behavior" setting that lets you set defaults for audio and subtitles during batch encoding, saving time and effort for multiple videos.
It's important to note that during batch conversion, HandBrake only keeps the audio and subtitle tracks you've specifically selected; any extras will be ignored. This means you can tailor audio and subtitles exactly how you need them for each video. If you want an even more streamlined approach, you can also use HandBrake's command-line version, HandBrakeCLI, to automate transcoding across entire directories of videos. This makes the batch conversion process even smoother and more efficient. While some might find this simpler, HandBrake's main interface is still useful for those who want more control over individual video settings.
When working with HandBrake's batch conversion feature for AI video upscaling, managing audio tracks and subtitles can be a bit tricky but also quite powerful. Each subtitle is treated like its own track, which gives you the flexibility to pick and choose which ones get included in the final video. You can even decide between soft subtitles (which stay as a separate track) and hard subtitles (which are baked into the video itself). Each approach has its pros and cons in terms of viewer experience and file size.
HandBrake's ability to handle multiple audio tracks is handy when you want to offer different language options or include commentary. This is useful for making videos more accessible to wider audiences. However, your audio codec choice matters here too, as it impacts both quality and file size. Newer codecs like Opus often provide a good balance of quality and compression, making them attractive for modern streaming or AI upscaling scenarios.
Subtitle formats themselves have their own quirks. SRT is a popular basic format, while ASS allows for more styling options which could be better for engaging viewers. Batch conversion makes things fast, but sometimes this can force the same audio and subtitle choices on all your videos. This might not be optimal if your source material varies widely. Fortunately, you can fine-tune audio levels during conversion with HandBrake. It lets you normalize the volume across tracks, avoiding those sudden jumps in sound during playback.
Don't assume a higher audio bitrate always equals better audio. There's a point of diminishing returns where further increases don't produce a noticeable improvement in quality, potentially leading to larger files. You can sometimes get HandBrake to automatically extract subtitles from certain formats during batch conversion. This is a neat trick that saves time when working on a huge set of videos, making it easier to manage accessibility.
It's worth noting that if subtitles aren't configured correctly, HandBrake might skip over encoding settings related to them. This can lead to issues like missing or poorly-timed subtitles, which isn't what you want. You might need to experiment to find the best practices for your specific setup and workflows. Paying close attention to the source video's information and how the settings affect the output is a crucial element of successful video conversion within an AI upscaling process. It is easy to see that the current iteration of HandBrake needs further refinement in its subtitle handling and management to streamline workflow and improve quality.
Optimizing HandBrake's Batch Conversion for AI Video Upscaling Workflows - Leveraging HandBrake's Queue System for Large-Scale Projects
When dealing with a large number of videos, especially in AI upscaling projects, HandBrake's queue system becomes a valuable asset. It lets you add multiple video files to a processing list, automating the conversion process and saving you time. This is particularly helpful when you need to apply consistent settings—like resolution, codec, and format—across a whole batch of videos, which is vital for seamless integration with AI upscaling tools. The queue helps maintain order and efficiency, allowing you to schedule conversions and ensure consistency in the final outputs. This streamlines the entire process, making it easier to manage large projects. It's important to be mindful that relying solely on batch processing can sometimes lead to quality sacrifices if settings aren't carefully considered. Users should always monitor the results to ensure the queue's automation isn't causing unwanted changes to the video quality.
HandBrake's queue system is a valuable asset for managing numerous video conversion tasks within large-scale projects. It lets you line up a bunch of videos for processing, essentially creating a to-do list for HandBrake. This can save a lot of time, especially if you're working with many files that would otherwise need individual attention.
One benefit is that you can prioritize certain videos in the queue. This comes in handy when you're iterating through an AI upscaling workflow and need certain outputs faster than others. If some videos are crucial for later stages of the process, you can bump them to the top of the queue.
Furthermore, HandBrake does a good job of logging errors related to individual encoding tasks during batch processing. This makes troubleshooting much easier since you can quickly see which videos ran into issues and avoid having to sift through a bunch of unrelated output files.
You can also set up custom encoding presets and apply them to the whole queue. This ensures that all the videos are processed using consistent settings, which is really important for maintaining quality standards, especially if you're sending the output files to an AI upscaler.
It's also useful that you can set limitations on the number of simultaneous conversions. This is important for managing system resources, especially if you're working on a powerful machine with multiple cores or in a cloud environment with a certain number of instances allocated. Limiting the number of tasks keeps your computer from getting overloaded and helps prevent crashes or slowdowns.
If there's an issue with a video that's been through HandBrake and upscaled with AI, you can easily adjust the queue to re-encode just that specific video without repeating the whole process. This makes the workflow flexible and avoids unnecessary work, a significant advantage in time-sensitive situations.
Also, HandBrake utilizes multi-threading during batch conversions, which means it can use multiple CPU cores effectively. This leads to faster processing, especially for large projects. The queue system works with many different video formats and codecs, which is crucial for ensuring compatibility with various AI upscalers.
HandBrake also provides real-time progress information, allowing you to see the status of each task and get an estimate of completion times. This allows for better planning and resource allocation for larger projects. For users who need more automation, the command-line version of HandBrake (HandBrakeCLI) lets you automate the whole queue using scripts. This makes batch conversion processes more streamlined, particularly if you need to replicate the exact same workflow across multiple machines or different projects.
While the queue system has advantages, it's important to acknowledge that a highly customized workflow can result in some odd behaviors if not configured correctly, especially in more complex AI upscaling projects. Further experimentation and adjustments to workflows are likely to be needed to get the optimal balance of speed, resource utilization, and quality.
Optimizing HandBrake's Batch Conversion for AI Video Upscaling Workflows - Integrating HandBrake Output with AI Upscaling Software
Connecting the output of HandBrake to AI video upscaling software is a crucial step in improving video quality for various applications. HandBrake is very useful for batch processing and offers a wide array of settings for preparing videos. However, it's not primarily designed for large resolution increases. Therefore, it makes sense to use HandBrake's output settings with specialized AI upscaling tools, such as those from Topaz Labs or Aiseesoft, to achieve better results. Matching HandBrake's output with the specific requirements of these upscalers—like using compatible codecs, bitrates, and resolutions—can greatly improve the outcome of the upscaling process. While HandBrake offers a strong starting point, it's often the integration with dedicated AI upscaling programs that truly transforms the quality of a video. There's often a trade-off to consider as well in terms of the quality of the upscaling vs. the time it takes to get there, and this is part of the design and development challenges facing users.
When integrating HandBrake's output with AI upscaling software, several aspects become crucial for optimizing the workflow. One challenge is the potential processing overhead introduced by large-scale batch conversions. Depending on your chosen resolution and codec, the load on your system can vary significantly, possibly leading to bottlenecks when the AI upscaler is involved.
The choice of color depth during the HandBrake encoding step can also affect the AI upscaling outcome. Switching to a 10-bit color depth provides a wider range of color data, allowing the AI upscalers to work with richer information and potentially minimize artifacts like banding that can be prominent in lower color depth outputs. This can be particularly important for videos with a lot of subtle color variations.
HandBrake's preview feature provides valuable feedback while you're tweaking encoding settings. However, using it consumes system resources, which can slow down the process, especially when preparing for large-scale batch conversions. There's a bit of a trade-off between real-time feedback and overall speed, and you might need to think carefully about the specific context of your task.
Interestingly, the frequency of keyframes influences how AI algorithms interpret scene changes. More frequent keyframes can help the AI recognize transitions more accurately, potentially leading to higher-quality upscaled results. The AI seems to benefit from having more consistent scene change information to process.
Another point to consider is how HandBrake handles interlaced video formats. Interlacing can introduce artifacts that often confuse AI upscaling software. Fortunately, HandBrake's ability to convert interlaced videos into progressive format can significantly simplify the processing and enhance the quality of the output. It's certainly worth taking advantage of if you are working with a lot of interlaced video.
The choice of audio codec has a significant impact on the overall file size and audio quality of the final output. Using newer audio codecs like Opus can be advantageous in AI workflows because they often provide high quality at lower bitrates compared to traditional choices like MP3. For AI upscaling, smaller file sizes can be a big advantage in some situations, allowing for better resource usage and faster processing.
HandBrake's batch conversion feature lacks the ability to apply unique settings to individual files within the same queue. This can pose a challenge if the source videos have varying quality or require different settings. As a result, extra vigilance is often necessary to ensure consistent output and achieve the desired outcomes across all videos.
The format of the output container also needs to be considered. Containers like MKV are generally more flexible and support a wider range of features, including advanced codecs and higher color depth. However, MP4, while more widely compatible, may prevent some upscaling optimizations that are possible with other containers. So it depends on your priorities in each specific project.
HandBrake's audio normalization feature is especially useful for batch processing. It's designed to prevent sudden, unwanted changes in audio levels across multiple files. This can be critical for maintaining a consistent and enjoyable experience for viewers, especially if you have a project involving diverse sources of video.
Finally, when running extensive video processing tasks with HandBrake, managing system resources is crucial. Controlling the number of simultaneous video conversions ensures the system isn't overloaded, particularly on multi-core processors or in a cloud-based environment. This is a practical step you can take to ensure the stability of your processing throughout complex AI upscaling projects.
Upscale any video of any resolution to 4K with AI. (Get started for free)
More Posts from ai-videoupscale.com: