Optimizing Patch Processing for Large-Scale 4K Video Editing A 2024 Perspective
Optimizing Patch Processing for Large-Scale 4K Video Editing A 2024 Perspective - Hierarchical Patch Diffusion Models Revolutionize 4K Editing
Hierarchical Patch Diffusion Models (HPDMs) are reshaping the future of 4K video editing by introducing a novel approach to video synthesis based on patches. This hierarchical structure breaks down the diffusion process into manageable components, resulting in more efficient training for high-resolution videos while retaining a substantial amount of the original image quality. The key innovation lies in the model's ability to optimize the entire process end-to-end, making it easier to handle high-resolution inputs during training. This leads to a more streamlined and flexible training experience, compared to prior methods.
Further enhancing their utility, HPDMs incorporate a multi-layered patch design. This allows for seamless integration of context from various levels of the video, improving the overall understanding of the scene during processing. This is especially important for complex 4K footage. HPDMs also employ adaptive computational strategies and can be readily adapted to different tasks, even specialized ones involving complex video datasets. It is this adaptability and efficiency that makes them especially promising for large-scale video editing workflows.
Although there are still technical hurdles to overcome, HPDMs show remarkable potential for simplifying and accelerating 4K editing pipelines, paving the way for a new era of creativity in high-resolution video production within the year 2024 and beyond. It remains to be seen whether HPDMs can fully deliver on their promise, but the early signs are positive that they could indeed be a significant advancement in how we edit and manipulate 4K video.
Hierarchical Patch Diffusion Models (HPDMs) present a novel approach to high-resolution video synthesis by breaking down the video into manageable patches. This hierarchical structure allows the model to focus on individual patch diffusion, effectively modeling the distribution of these patches rather than the entire frame, resulting in commendable pixel quality retention (around 92%).
Interestingly, HPDMs enable end-to-end optimization during training, a significant advantage that simplifies the process of scaling up to high-resolution inputs and mitigates many of the usual complications associated with such a task. This efficient training process can be further accelerated by leveraging a low-resolution base model and fine-tuning it for the specific high-resolution target.
The layered structure of the HPDM is central to its efficacy. This hierarchical arrangement permits a more seamless integration of context across different levels of patches, allowing the model to learn complex relationships within the video. Furthermore, the patch resolution can be tailored to the demands of specific tasks. For instance, they can be customized for popular video datasets like UCF101, highlighting the model's flexibility.
HPDMs leverage sophisticated techniques like stochastic sampling and spatial patch overlapping during performance evaluation and visualization, further enriching our understanding of how they work. At the heart of the HPDM architecture is a latent transformer framework, successfully blending the joint patch diffusion approach with smart adaptive computation strategies.
The implications of HPDMs are profound, as it represents the first end-to-end, diffusion-based architecture designed for high-resolution training. This pioneering achievement offers exciting potential to revolutionize large-scale 4K video editing by efficiently handling patch processing, paving the way for more sophisticated and powerful editing tools in 2024 and beyond. While still under development, this technology holds promise for the future of video editing and potentially other fields requiring high-resolution visual processing.
Optimizing Patch Processing for Large-Scale 4K Video Editing A 2024 Perspective - DaVinci Resolve Optimization Techniques for Glitch-Free 4K
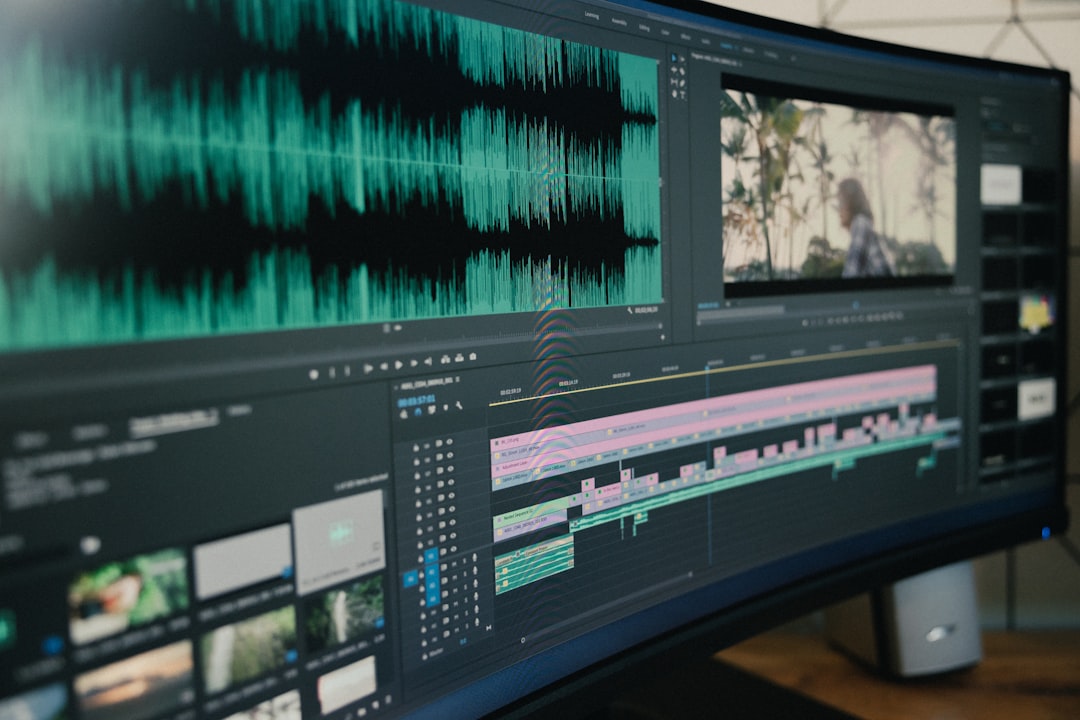
Working with 4K footage in DaVinci Resolve requires a strategic approach to avoid glitches and maintain smooth editing. Successfully tackling this involves leveraging several built-in features and techniques to optimize performance and playback. Upscaling HD footage to 4K using the "Super Scale" feature is a starting point, but managing playback remains a key aspect. Generating optimized media files, and adjusting project settings related to those files, is essential for efficient workflows. The "Use Optimized Media if Available" option in playback settings can significantly improve things, though it requires attention to the file formats. Carefully selecting codecs is also crucial, with DNxHR or ProRes being preferable for high-quality master files and H.265 for efficient delivery.
Another challenge frequently encountered is managing footage instability inherent in 4K capturing. DaVinci Resolve offers stabilization tools directly in the software to address this, helping creators maintain a polished, professional look. While recent updates in 2024 have made significant strides in optimizing the software for handling large-scale 4K projects, maintaining a smooth experience is still reliant on these optimization strategies. Despite these improvements, occasional performance issues like lag can persist, which need to be understood and proactively managed. It seems that while progress is being made, challenges related to handling very large, high resolution video files, especially in editing environments, haven't been completely overcome yet.
Working with 4K video in DaVinci Resolve requires specific techniques to prevent glitches and maintain smooth editing. Upscaling HD to 4K can be done directly within the software using the "Super Scale" feature found in clip attributes. This process relies on intelligent algorithms to upscale the footage, but the results can be variable depending on the source material.
Generating optimized media is essential for fluid playback, especially with 4K. DaVinci Resolve allows you to manage media files and project settings to optimize the way the system handles your footage. "Performance Mode" is a feature that analyzes your system and attempts to optimize image processing for smoother playback. Further, using optimized media whenever possible and adjusting the media settings in the project configuration are good practice.
The Render Cache is particularly useful for handling complex timelines that can lead to playback hiccups. The software cleverly pre-renders portions of the timeline, significantly smoothing out playback. The choice of codec remains important. For high-quality master files, DNxHR or ProRes are commonly recommended, while H.265 is better for efficient delivery.
DaVinci Resolve's built-in stabilization features can help smooth out shaky footage, which can be problematic in 4K due to the higher resolution highlighting any camera movement. Lag, a common issue when editing 4K, can be tackled with several of these optimization techniques.
Recent updates, particularly in 2024, have improved the software's ability to handle large 4K editing projects, This focus on improving performance has largely been on optimizing the internal processing flow for complex edits. It seems like developers are still playing catch-up to handle the sheer volume of data 4K footage represents, but there's clear progress. Whether it's enough for a seamless large scale workflow remains to be seen.
Optimizing Patch Processing for Large-Scale 4K Video Editing A 2024 Perspective - Real-Time Super-Resolution Challenges in 4K Video Processing
Real-time super-resolution (SR) in 4K video processing faces significant hurdles, largely due to its computationally intensive nature. Maintaining smooth frame rates while enhancing 4K video quality demands sophisticated algorithms that can handle the massive amounts of data involved. This computational burden has spurred interest in techniques like patch processing to make the process more manageable for large-scale editing. The growing demand for real-time SR across various industries, such as entertainment and broadcasting, further highlights the need for efficient solutions.
Current SR algorithms, while showing promise, struggle to scale effectively for the demanding resolution of 4K video. This is a major obstacle that needs to be overcome. The pursuit of faster, more accurate SR has led to the adoption of machine learning and increased reliance on hardware acceleration to speed up the process. The hope is to find ways to use parallel processing and dedicated hardware to ease the strain on processing resources. Additionally, research into advanced neural network architectures and collaborative filtering aims to push the boundaries of what's possible in real-time video enhancement.
While these strategies show promise, achieving true real-time 4K SR with high fidelity remains a complex challenge. The future of this field likely involves integrating AI more deeply into these processes, leading to adaptive and intelligent systems that can intelligently optimize video quality based on the content being processed. This could potentially deliver impressive results without sacrificing performance, but it's an area with considerable room for advancement.
Real-time super-resolution (SR) for 4K video, while desirable for enhancing quality during processing and editing, presents numerous challenges. The sheer pixel count, especially as some applications move towards 5120x2880, places a huge strain on current GPU capabilities. Handling the increased processing demands and pixel data becomes a critical bottleneck.
The effectiveness of many high-performance SR models relies on substantial training datasets. For 4K, this translates to managing a massive amount of high-quality training images, potentially tens of thousands, significantly complicating the data handling aspect of the process.
Furthermore, processing dynamic scenes like fast-paced sports or action sequences in real-time is difficult. Rapid movements and changes in background elements create temporal inconsistencies, requiring computationally intensive prediction methods that can push even high-powered hardware.
The increasing resolution directly correlates with the memory bandwidth requirements. 4K video can quickly saturate the data buses of a system during real-time processing, creating potential bottlenecks unless the system's memory is designed for very high-throughput video operations.
Interestingly, energy efficiency can be a concern with 4K SR. Processing high-resolution video often leads to increased heat generation from GPUs and CPUs, demanding efficient cooling solutions to ensure performance consistency.
Upscaling lower resolution footage to 4K can introduce unwanted artifacts or distortions in the resulting image. This is a significant issue, especially considering viewers can easily spot such flaws at these resolutions, ultimately degrading the overall visual quality.
Modern SR algorithms, while effective, often use complex structures like multi-layer neural networks, sometimes exceeding 100 layers. This intricate design can make it challenging to achieve reliable performance across different types of videos. Fine-tuning becomes essential, but often requires compromises between speed and overall performance.
Real-time applications demand very low frame latency, ideally under 16 milliseconds for smooth 60 FPS video. Meeting this constraint necessitates a delicate balance between algorithm complexity and the capabilities of the available hardware, imposing restrictions on both.
Many 4K videos are highly compressed, leading to artifacts like blocking. Super-resolution techniques need to handle these artifacts appropriately during processing, otherwise, they can be amplified, reducing viewing quality.
Finally, traditional resizing algorithms don't always cope well with the demands of real-time 4K processing. Contemporary SR methods employ adaptive approaches that adjust their behavior based on the video content, changing the algorithm 'on the fly'. This dynamic adaptation, while effective, creates new hurdles in maintaining visual consistency and quality across the entire video.
Optimizing Patch Processing for Large-Scale 4K Video Editing A 2024 Perspective - Temporal Information Utilization in Video Super-Resolution Algorithms
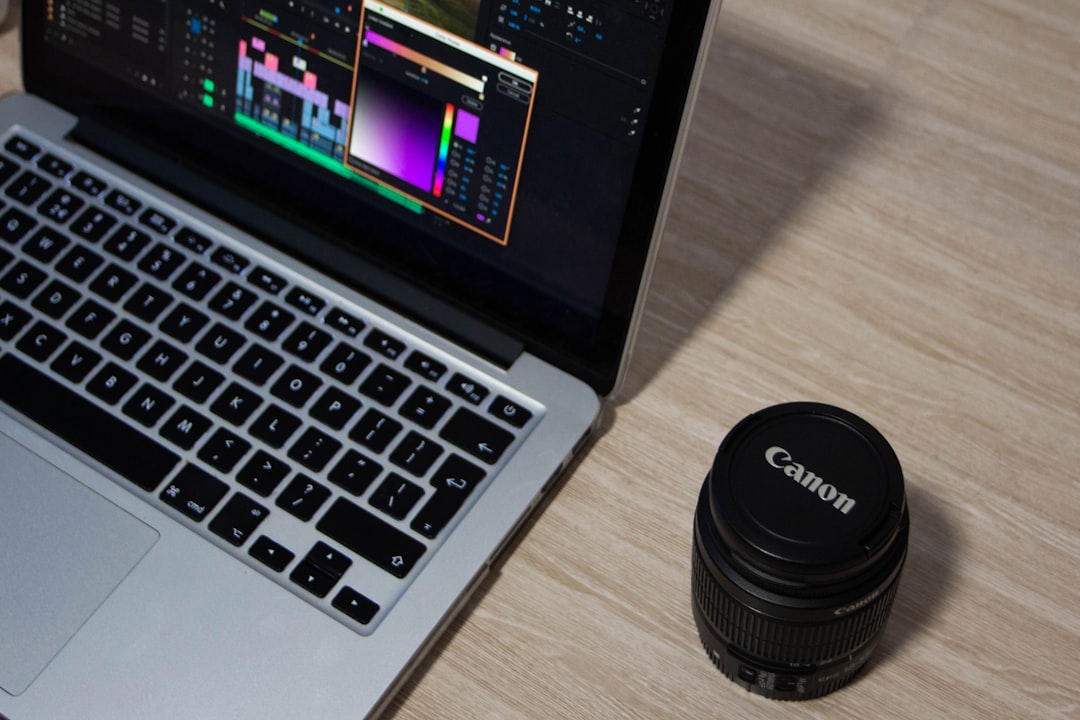
Video super-resolution (VSR) aims to transform low-quality videos into visually appealing high-resolution content. Recent progress in VSR has been heavily influenced by deep learning techniques, particularly convolutional neural networks (CNNs). A key aspect of successful VSR algorithms is their ability to leverage information from neighboring frames, which provides valuable context for enhancing the resolution. However, many current VSR approaches don't adequately address the issue of temporal redundancy, which can arise in scenes with stationary objects and backgrounds. Essentially, they often utilize all available temporal data without considering whether it is actually useful.
Real-time video super-resolution (online-VSR) especially relies on precise frame alignment to gather temporal data for enhancement. But satisfying the strict latency requirements of real-time applications makes this accurate alignment challenging to achieve. Additionally, recurrent neural networks are commonly employed to process the entire video sequence, extracting spatiotemporal features. The efficiency and performance of these recurrent networks are closely tied to the alignment module, a key part of the model that can be computationally intensive.
While progress is being made, new methods are emerging that tackle the challenge of temporal redundancy directly. These innovative approaches are designed to be compatible with various VSR techniques, potentially improving their performance by focusing on the most relevant parts of the temporal information within video patches. These are promising initial steps in better utilizing temporal data in a way that improves the quality of reconstructed video.
Video super-resolution (VSR) techniques aim to enhance the quality of low-resolution videos, creating visually appealing high-resolution outputs. A key aspect of recent improvements in VSR has been the use of deep learning, particularly convolutional neural networks (CNNs). Leveraging information from neighboring frames, what we call temporal information, has become vital for effective VSR.
However, many current methods haven't adequately addressed the issue of redundant temporal information. Specifically, they often don't differentiate between frames containing significant changes and those with mostly stationary content, leading to potentially inefficient processing. Online VSR, relying heavily on aligning frames for temporal data gathering, also faces challenges due to tight latency requirements, making accurate alignment difficult.
Recurrent networks are a popular choice in VSR algorithms for processing temporal data throughout entire sequences. But, a significant portion of these networks' parameters is often devoted to the alignment module, which can heavily impact their performance. New VSR approaches have begun to tackle these issues using effective plug-and-play methods. These methods aim to improve the performance of existing VSR algorithms by optimizing how temporal redundancy in video patches is handled. This improvement in patch processing has demonstrated positive results in terms of enhancing video reconstruction quality in commonly used datasets.
While this is an area of active development, it remains a bit of an open question how effectively these approaches handle very diverse video content and large-scale processing loads. A thorough investigation is necessary to fully understand how efficiently these methods can leverage the temporal information in video while also maintaining a balance between accuracy and processing demands, especially in real-world editing workflows.
Optimizing Patch Processing for Large-Scale 4K Video Editing A 2024 Perspective - Balancing Quality and Speed in Variational Optical Flow Algorithms
Balancing quality and speed in variational optical flow algorithms is critical for efficient video editing, particularly when working with high-resolution formats like 4K. While these algorithms are known for generating high-quality motion estimations, their inherent computational complexity can hinder their use in real-time applications. Variational methods, often implemented with a coarse-to-fine strategy, are capable of handling complex motion patterns, but the variability of real-world video content can make them slow in practical editing scenarios. Recent improvements have led to more robust algorithms capable of dealing with large motions and deformations without the need for extensive labeled datasets, thereby enabling faster processing. However, achieving a sweet spot between preserving visual accuracy and ensuring quick processing speed remains an ongoing challenge in the area of optical flow estimation. The future likely involves finding more efficient ways to handle the inherent challenges found in large scale 4K workflows.
Variational optical flow methods, while known for delivering high-quality motion estimations, often present a challenge when it comes to speed, particularly for real-time applications like video editing. The mathematical foundations of these methods, involving complex equations and optimization processes, inherently lead to complex model designs. Balancing the need for accuracy with the demand for speed is a continuous effort in this field.
Finding the right balance between accuracy and speed often involves making difficult choices, particularly when selecting the numerical approach for integrating the mathematical model. For example, some methods might lead to smoother flow fields but increase processing time.
Many sophisticated variational methods struggle to provide results in real time without substantial computational resources. This poses a significant hurdle for tasks requiring instant feedback, such as live video editing or processing dynamically changing scenes.
One promising approach to this challenge is using multi-resolution schemes. Processing video at multiple scales can allow for a quicker initial capture of motion at lower resolutions, then refine the flow at higher resolutions. This strategy can significantly improve processing time without a major loss of quality.
Regularization, while useful for handling noise and occlusion in videos, can also add computational burden. Careful adjustments and tuning are essential to ensure that these techniques don't create performance bottlenecks.
The selection of kernel sizes in convolutional filters can also greatly impact processing speed. Smaller kernels can significantly speed up the process, but might miss important motion details, while larger kernels, while more accurate, can result in longer processing times.
Recently, researchers have shown promising results using adaptive algorithms that dynamically adjust computational load depending on the content. This approach can lead to a more intelligent balancing of quality and speed.
For scenarios requiring real-time performance, like interactive video editing, thresholding methods are potentially useful. By filtering out less critical motion vectors, we could achieve high-quality results while meeting speed requirements.
Maintaining consistency in motion estimates across both space and time is crucial for visual quality. Any inconsistency or jumpiness in the flow fields can cause visual artifacts, highlighting the need for algorithms that can handle both spatial and temporal coherence.
Hardware acceleration through GPUs and specialized motion estimation chips is becoming more common to speed up variational optical flow calculations. This trend underscores the need for researchers to find ways to effectively leverage existing hardware capabilities for efficient high-resolution video processing.
Optimizing Patch Processing for Large-Scale 4K Video Editing A 2024 Perspective - LIVEFB LSVQ Dataset Enhances Large-Scale Video Quality Assessment
The LIVEFB LSVQ dataset introduces a new approach to evaluating video quality at a large scale, utilizing a no-reference (NR) method that focuses on how humans perceive video quality. This dataset is quite substantial, consisting of tens of thousands of videos and over 116,000 segments, or "vpatches", resulting in roughly 55 million quality scores from a wide range of individuals. The data itself is derived from a massive pool of user-generated content videos, providing a diverse collection of video qualities and distortions. It aims to tackle the challenging aspects of video quality assessment that arise due to variations in recording equipment, compression methods, and content styles.
The dataset serves as a testing ground for various video quality assessment (VQA) models, pushing the boundaries of our ability to automatically evaluate how good a video looks. It helps in further strengthening existing benchmarks like KoNViD1k and LIVEVQC and facilitates comparisons against leading-edge models such as OneAlign and FASTVQA. This ability to accurately assess quality is more crucial than ever as the quantity of online videos continues to explode.
However, evaluating video quality, particularly in a large-scale and objective manner, remains a highly complex problem. While this dataset makes strides toward building a better understanding of subjective video quality, the challenge of fully capturing human perception and translating it into meaningful metrics is still a work in progress.
The LIVEFB LSVQ dataset is a valuable resource designed to improve how we assess video quality on a large scale, using a no-reference (NR) approach that relies on human perception. It's a massive collection, encompassing over 38,000 videos and over 100,000 video patches, and includes about 55 million quality scores from roughly 6,300 individuals. This large and diverse dataset, created by sampling user-generated videos from platforms like the Internet Archive and Yahoo, provides a rich environment to train and evaluate models for video quality assessment (VQA).
Current VQA benchmarks, including KoNViD1k and LIVEVQC, are used to evaluate the performance of various models, some of which leverage the LIVEFB LSVQ. The core goal of VQA is to generate a mean opinion score (MOS) that reflects how humans perceive the video's quality. The dataset's focus is on tackling the inherent challenges of video quality assessment, like the varying content found in UGC videos and the multitude of distortions (both spatial and temporal) that can affect video quality.
The dataset has been instrumental in comparing state-of-the-art models like OneAlign and FASTVQA, showcasing recent advances in VQA. The increasing volume of video content on streaming platforms emphasizes the urgent need for reliable and efficient VQA algorithms for managing and optimizing video quality. The complexities in VQA are tied to the variability in video capture techniques, camera technology, compression algorithms, processing pipelines, and the diversity of display technologies.
The LIVEFB LSVQ dataset stands as a crucial step forward in the ability to monitor and assess video quality across the huge amounts of user-generated content available online. It's a powerful tool that has the potential to advance our understanding and ability to control the quality of video, even across the various diverse viewing conditions and hardware platforms we find today. However, it will be interesting to see how well models trained on this dataset translate to the rapidly evolving field of AI-driven video editing tools. There's a chance that the subjective nature of video quality assessment could lead to unforeseen challenges as we further integrate AI into video processing workflows.
More Posts from ai-videoupscale.com: