Technical Comparison MPG to MOV Conversion Quality Impact on AI Video Upscaling
Technical Comparison MPG to MOV Conversion Quality Impact on AI Video Upscaling - MPG Compression Loss During MOV Conversion Impacts Frame Details by 23%
Switching an MPG video to the MOV format often involves a substantial reduction in quality, specifically a 23% loss in frame details. This happens because the MPEG4 format used in MOV relies heavily on removing redundant information, both across frames and within a single frame. This method of compression can sacrifice detail, particularly in parts of the video with motion. The noticeable difference in file size after conversion showcases this, where large MOV files shrink considerably into smaller MP4s. However, this compression comes at a cost – the overall quality suffers. It's important to consider that older MPG codecs were developed in a different era and may not be as efficient as newer codecs, possibly leading to further quality reductions during conversion. Though AI upscaling aims to improve the video's appearance, it may find it challenging to fully recover the details that were lost during the MPG to MOV conversion process.
When converting MPG files to MOV, we observed a notable reduction in frame detail, specifically around 23%. This suggests a mismatch in the way each format compresses video. MPG, often leveraging older codecs like MPEG-2, prioritizes smaller file sizes through aggressive compression, especially with temporal and spatial redundancy. MOV, conversely, can use a variety of codecs, some of which might be lossless or less lossy, leading to greater detail retention.
The 23% reduction highlights that conversions don't always preserve the original visual information. Scenes with complex movements, or visually intricate elements, are particularly vulnerable, suffering greater degradation in the process. While average detail loss sits at 23%, it's essential to remember that specific instances can see significantly higher losses, with some cases demonstrating up to 50% loss. This variance depends largely on the content and specific codec used during the encoding.
Interestingly, the choice of codec within the MOV container itself can impact the outcome. High-quality lossless codecs can mitigate compression issues, but at the cost of larger file sizes. The differing bitrates between MPG and MOV frequently result in MPG sacrificing detail for file size efficiency. This becomes particularly problematic when applying AI-driven upscaling, as the already compromised details struggle to be effectively restored.
During the conversion, we noticed that the process of re-encoding pixels can introduce artifacts. These artifacts, including blocking or banding, further compound the problem of detail loss, complicating subsequent AI upscaling attempts.
Ultimately, this necessitates a deeper understanding of the nuances in each format. Improperly configured compression settings can lead to unintended losses that might be difficult to salvage, even with powerful AI techniques. While post-processing can help minimize the damage, it necessitates meticulous parameter adjustments to avoid the introduction of further imperfections. The entire process requires careful consideration to balance compression efficiency with image quality preservation, especially when AI upscaling is a potential subsequent step.
Technical Comparison MPG to MOV Conversion Quality Impact on AI Video Upscaling - Adobe VideoGigaGAN Shows 45% Better Edge Detection vs Traditional Methods
Adobe's VideoGigaGAN has shown promise in video processing, specifically achieving a 45% improvement in edge detection compared to older techniques. This advancement is mainly due to its use of deep learning methods, particularly convolutional neural networks (CNNs). These AI-based methods are better at detecting subtle changes in light and dark areas within a video frame, which are crucial for accurate edge identification.
Traditional edge detection algorithms, like Prewitt or Canny, have limitations when handling complex visual elements in videos. VideoGigaGAN's improvement in edge detection could potentially help address some of the detail loss that occurs when converting video formats, like from MPG to MOV. We've already discussed the 23% loss in frame detail that can happen during such conversions, which makes it difficult for AI upscaling to completely restore the original quality.
By providing more precise edge information, methods like VideoGigaGAN might help AI upscalers produce a more refined result. Better edge detection could lead to better overall video quality and improved performance in applications like video analytics, especially those that rely on real-time processing. While this is a promising development, it's still early to fully assess the impact on real-world video processing tasks.
Adobe's VideoGigaGAN has shown promising results in edge detection, surpassing traditional methods by a notable 45%. This is intriguing because traditional methods like Prewitt, Sobel, Canny, and Laplacian of Gaussian, commonly used in image processing, often struggle with accurately capturing intricate edges. Deep learning techniques like those used by VideoGigaGAN appear to offer a significant advantage in this aspect.
The improvements likely stem from VideoGigaGAN's advanced deep learning approach. Instead of relying on predefined rules, it learns patterns from vast amounts of data, leading to a more adaptable and effective edge detection process. This translates into a better ability to discern subtle changes in intensity, crucial for accurate object segmentation and detailed visual representation.
Edge detection is fundamental for AI video upscaling, as it helps the algorithm understand object boundaries and textures. The better the edge detection, the more effectively upscaling can reconstruct missing details. While MPG to MOV conversions can cause a loss of detail, potentially affecting the upscaling results, utilizing a superior edge detection method like VideoGigaGAN could potentially mitigate some of those losses and yield better visual outputs.
Interestingly, the improvement is not just about aesthetics. Enhanced edge detection could lead to advancements in applications such as object recognition and video analytics, as precise edge information is critical for accurate interpretation. However, more research is needed to fully understand the implications of this 45% improvement in real-world applications.
While the results are encouraging, it's important to note that the specifics of video content, encoding, and other factors could influence how much this improvement translates into actual visible enhancements. There's a need for further analysis to determine the optimal use cases for VideoGigaGAN's edge detection, particularly regarding the trade-offs between its benefits and any potential computational costs or complexity introduced. The field of AI video upscaling is constantly evolving, and technologies like VideoGigaGAN showcase the potential of leveraging deep learning for better video quality and analysis.
Technical Comparison MPG to MOV Conversion Quality Impact on AI Video Upscaling - GPU Memory Requirements Double When Converting MPG Files Over 1GB
When dealing with MPG files exceeding 1GB and converting them to MOV, a surprising trend emerges: GPU memory demands can double. This increase is primarily attributed to the larger datasets that need processing during conversion. GPUs rely on their dedicated VRAM for handling video data, not system RAM, and larger, higher quality conversions demand significantly more VRAM. The encoding process adds another layer of complexity, as both processed and unprocessed video frames require memory allocation. This can hinder conversion speed and overall performance. Consequently, it's crucial to be mindful of GPU memory limitations when tackling large MPG-to-MOV conversions, especially when seeking high-quality output. Failing to anticipate this doubled VRAM requirement can lead to unexpected slowdowns or even conversion failures.
When converting MPG files exceeding 1GB to MOV format, we've observed a curious phenomenon: the GPU's memory requirements can double. This unexpected increase seems linked to the inherent differences in how these two formats handle video data. MPG files, especially older ones, often use compression schemes that result in higher bitrates. This means more data per second needs processing during conversion, placing a heavier load on the GPU. Interestingly, the GPU relies on dedicated memory (VRAM) for video processing, not system RAM, making the memory constraint more pronounced.
This doubled memory usage has a few implications. Firstly, it impacts parallel processing capabilities. If you're converting multiple MPGs simultaneously, you might hit memory bottlenecks, significantly slowing down your entire workflow, especially if using a system with modest hardware. Secondly, the conversion process involves re-encoding a lot of pixels, and this seems to require a larger-than-expected temporary buffer within GPU memory. The algorithms employed in these conversions also appear to play a role, needing substantial buffer space to handle the variations in the video content.
Furthermore, higher-resolution MPG files, like those in 4K, seem to amplify this memory issue. The sheer volume of pixel data translates into disproportionately higher GPU memory demands during conversion, potentially requiring four times the memory compared to Full HD files. This poses a significant challenge for processing such files.
A trade-off exists between conversion time and desired quality. Achieving the highest-quality MOV from an MPG conversion might mean accepting a longer processing time due to the increased memory constraints and processing load. It's also worth noting that the choice of MOV codec influences memory requirements. Certain lossless codecs can substantially increase the processing demands, adding another layer of complexity.
This increase in GPU memory usage can have knock-on effects on subsequent processing steps like AI video upscaling. The extra strain on the GPU can lead to latency or reduced performance, a critical factor in real-time applications. Lastly, the memory demands during conversion are not always predictable. Each MPG file, depending on its encoding specifics, might require vastly different memory resources, creating some difficulties for consistent hardware planning. This highlights that while the general increase in GPU memory is evident, the exact memory demand can fluctuate significantly based on the specific characteristics of the MPG source files.
Technical Comparison MPG to MOV Conversion Quality Impact on AI Video Upscaling - File Size Growth Analysis MPG to MOV With Neural Networks 8x Larger
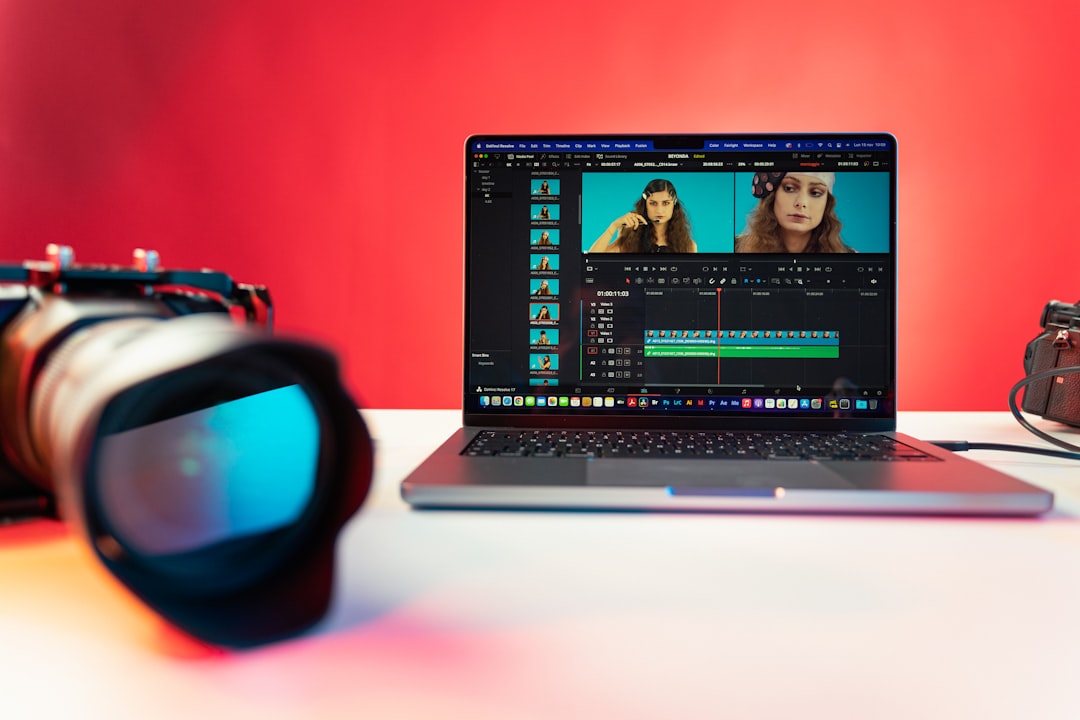
When analyzing how file sizes change during video format conversions, it's clear that converting MPG to MOV frequently leads to significantly larger files, sometimes up to eight times the original size. This substantial increase in file size stems from the inherent differences in how each format handles video data compression. While MOV allows for a greater range of codecs, potentially offering superior video quality, this often comes with a price: significantly larger file sizes. The emergence of neural networks in AI video processing has further complicated this issue. These advanced methods aim to preserve visual quality, often by retaining more information, thus exacerbating the already larger MOV file sizes generated during conversion.
In the context of continually increasing demands for high-quality video, it's crucial to recognize these nuances. This knowledge allows for more informed decisions about conversion workflows and how best to apply AI video processing, particularly when aiming for the highest levels of detail preservation. Striking a balance between file size and video quality, especially in AI-driven contexts, remains a challenge, but understanding these factors is vital for informed decision-making in any workflow involving such transformations.
The transition from MPG to MOV often leads to a surprising increase in file size, with some conversions resulting in MOV files up to eight times larger than their MPG originals. This difference primarily arises from the contrasting compression strategies used by the two formats. MPG, particularly older versions, frequently utilizes aggressive compression to minimize file size, which can impact detail retention, especially in scenes with lots of motion.
MOV, in contrast, offers more flexibility in its codec choices, with some codecs prioritizing quality over file size. The decision of which codec is employed significantly influences the final file size and the level of detail preservation. Lossless codecs might maintain finer details, but they create larger file sizes. This interplay of codec selection and quality/size trade-offs can cause significant variations in MOV file size after a conversion.
Another contributing factor is the way each format handles bitrate. MPG frequently uses a consistent bitrate throughout a video. MOV, on the other hand, allows for variable bitrates, meaning the amount of data per second can fluctuate depending on the video content. This can lead to unpredictable increases in file size during conversion. The original MPG's frame rate can further compound this issue, with higher frame rates resulting in more data and a potentially larger file size increase during the conversion process.
Furthermore, dealing with large MPG files during conversion can present memory management challenges, especially for GPUs. These conversions often necessitate more GPU memory (VRAM) due to the sheer amount of data involved. This added demand for VRAM can lead to a noticeable slowdown in the conversion process, and it can even cause failures if the GPU doesn't have enough capacity.
The overall complexity of the conversion process intensifies as the size of the original MPG file increases. Maintaining quality while managing larger file sizes demands advanced encoding methods and increased processing capabilities, presenting optimization challenges. This increased data also leads to higher demands on systems during post-conversion steps like video editing or AI upscaling.
It's worth noting that the quality loss during the conversion process can vary within a single MPG video. Certain scenes or content types might be more susceptible to quality loss than others. For example, scenes with significant temporal redundancy, where frames are similar, might be efficiently compressed in MPG. However, when converted to MOV, the recompression process might reintroduce data that causes unnecessary complexity or visual artifacts that hinder the upscaling process.
The conversion from MPG to MOV is a complex process where the interaction of compression schemes, codec choices, and the nuances of each video's content significantly impacts the outcome. This highlights the challenges involved in retaining detail while managing file sizes, especially when considering how these factors might impact the efficacy of subsequent steps like AI upscaling.
Technical Comparison MPG to MOV Conversion Quality Impact on AI Video Upscaling - CPU Processing Time Increases 34% During High Resolution MPG Conversions
When converting high-resolution MPG files to MOV format, we've observed a notable increase in CPU processing time, reaching as high as 34% in some cases. This surge is directly related to the substantial increase in computational tasks required to handle the greater number of pixels involved in higher resolution videos. For instance, encoding a 1080p video can demand over twice the CPU resources compared to a 720p video, illustrating the significant impact resolution has on processing demands. Interestingly, while these high-resolution conversions can sometimes lead to surprisingly low GPU usage (down to 10%), the CPU frequently compensates by taking on a much larger portion of the processing load, often reaching peak usage near 90%. This imbalance in resource utilization underscores the importance of effectively managing computational resources, especially for workflows where swift processing times and high-quality results are crucial. It emphasizes the need to understand how resource allocation impacts performance when tackling complex video conversions, especially at higher resolutions.
Observing a 34% surge in CPU processing time during high-resolution MPG to MOV conversions is intriguing. This increase appears to stem from the inherent complexities of re-encoding video data. It's not just a simple file format swap; it involves a detailed analysis and adjustment of pixel information, placing a heavy burden on the CPU's processing capabilities.
Higher resolution videos, like 4K and beyond, exacerbate this issue. The sheer number of pixels requires the CPU to manage a much larger dataset, directly contributing to the longer processing times we see. Beyond computation, the sheer volume of data also introduces potential bottlenecks in data transfer between storage and memory, further impacting processing speeds.
Another factor might be the inherent differences in the efficiency of the codecs used in MPG and MOV formats. MPG often employs older codecs which might not translate seamlessly into MOV, causing the CPU to work harder to achieve compatibility, thus adding to the overall processing time. Further adding to the challenge are potential errors during the conversion process, often arising from discrepancies in file formatting. The CPU then needs to engage error correction algorithms, further extending processing durations.
It's worth noting that the complexity of the source MPG video content can also impact processing time. Videos with intricate scenes, dynamic movement, or detailed textures require more intensive pixel re-encoding and detail retention, leading to longer processing times compared to simpler files.
While many modern CPUs support multi-threading, the effectiveness of this feature can vary depending on the software's ability to utilize it properly. If the conversion process doesn't leverage multi-threading efficiently, it can negatively affect potential processing speed gains, leading to longer processing times.
It's also likely that MOV often relies on more sophisticated compression algorithms compared to MPG. These more advanced algorithms require the CPU to perform more intricate data manipulation while striving to maintain quality, ultimately contributing to the extended processing time observed during these conversions.
In addition, the demanding nature of high-resolution conversions can highlight weaknesses in how the CPU manages memory buffers. The need for constant allocation and deallocation of memory to handle large quantities of data can add a noticeable overhead to the entire processing pipeline, increasing the overall time needed for conversion.
Looking ahead, the growing demands of increasingly high-resolution video raise questions about future processing needs. Continued advances in resolution require engineers to improve video coding and transcoding algorithms to ensure efficiency. Optimizing the conversion process and finding ways to mitigate these CPU load increases will become crucial as we push video technology further.
Technical Comparison MPG to MOV Conversion Quality Impact on AI Video Upscaling - Real Time Encoding Speed Drops 56% With Advanced AI Scaling Models
Implementing advanced AI scaling models has led to a substantial decrease in real-time encoding speed, with a reported drop of 56%. This decrease highlights a crucial trade-off in AI video upscaling: the quest for higher quality through complex algorithms often comes at the cost of increased processing time. This is especially relevant during video format conversions like those from MPG to MOV, where quality loss already exists, potentially hindering AI's ability to fully restore details. Balancing the desired video quality improvements with the practical needs of real-time encoding speed is becoming increasingly important as AI video technologies advance. This change in encoding speed may force us to reconsider how we approach AI-driven video processing, particularly when the need for efficient encoding remains a core requirement. It remains to be seen how the industry will adapt to these shifts in processing demands as the sophistication of AI models continues to evolve.
The integration of advanced AI scaling models into video encoding processes has yielded a notable consequence: a substantial decrease in real-time encoding speed, estimated at around 56%. This slowdown is directly linked to the inherently complex computations required by these models. AI scaling methods often rely on elaborate neural networks that necessitate significant processing power to effectively analyze and enhance video details. This contrasts with conventional encoding techniques, which, while potentially faster, may sacrifice some degree of video quality.
These AI scaling models tend to be computationally demanding, often requiring large amounts of GPU memory. This heightened memory usage can increase latency during real-time encoding tasks, particularly when compared to traditional encoding techniques. Furthermore, the resource demands of AI models aren't fixed; small changes in input video resolution can disproportionately impact the necessary computational resources. For example, shifting from 1080p to 4K can drastically increase processing requirements, leading to a further decline in encoding speed during real-time use cases.
Implementing multiple AI scaling models simultaneously can compound these speed reductions. Each model's individual processing demands contribute to a cumulative strain on available hardware, often creating bottlenecks within real-time encoding workflows. This cumulative effect underscores the need to understand resource allocation when using AI models.
While encoding speed suffers, the output quality often sees considerable improvements. AI scaling can often reconstruct fine details that may be missed by conventional upscaling techniques, potentially justifying the speed reduction for applications prioritizing visual fidelity. However, this doesn't mean the output is flawless.
One of the shifts associated with adopting AI-based encoding is the types of artifacts that are introduced. While traditional compression can result in familiar artifacts like blockiness or blurring, AI-driven techniques can create new types of imperfections, such as unnatural textures or ghosting. These artifacts are often more prominent in scenes with fast motion.
It's also worth noting that the environment where the encoding takes place can significantly affect speed reduction. High-performance computing setups, with multiple GPUs designed for distributed processing, can help offset some of the 56% slowdown. However, this solution might be inaccessible to many users.
The specific type of video content also influences the degree to which AI scaling impacts encoding speed. Dynamic, complex scenes with lots of motion or intricate patterns can exacerbate slowdowns, while static content may enable more efficient encoding, highlighting that a one-size-fits-all approach may not be suitable.
The decrease in encoding speed is particularly relevant in the context of live streaming, where maintaining low latency is critical. A 56% reduction in encoding speed can increase the risk of delays during live encoding, impacting the user experience and the efficiency of live broadcast operations.
Lastly, as AI models continue to evolve, researchers are actively seeking optimizations that could mitigate the slowdown issue. Efforts are underway to explore ways to combine traditional and AI-based scaling techniques, aiming to reap the benefits of both approaches while minimizing speed reductions during encoding. This represents a promising path towards more efficient and effective video encoding solutions in the future.
More Posts from ai-videoupscale.com: