Upscale any video of any resolution to 4K with AI. (Get started for free)
Topaz Video AI v330 New Stabilization Model and Enhanced Video Processing Capabilities Unveiled
Topaz Video AI v330 New Stabilization Model and Enhanced Video Processing Capabilities Unveiled - Revamped Stabilization Model Tackles Camera Shake
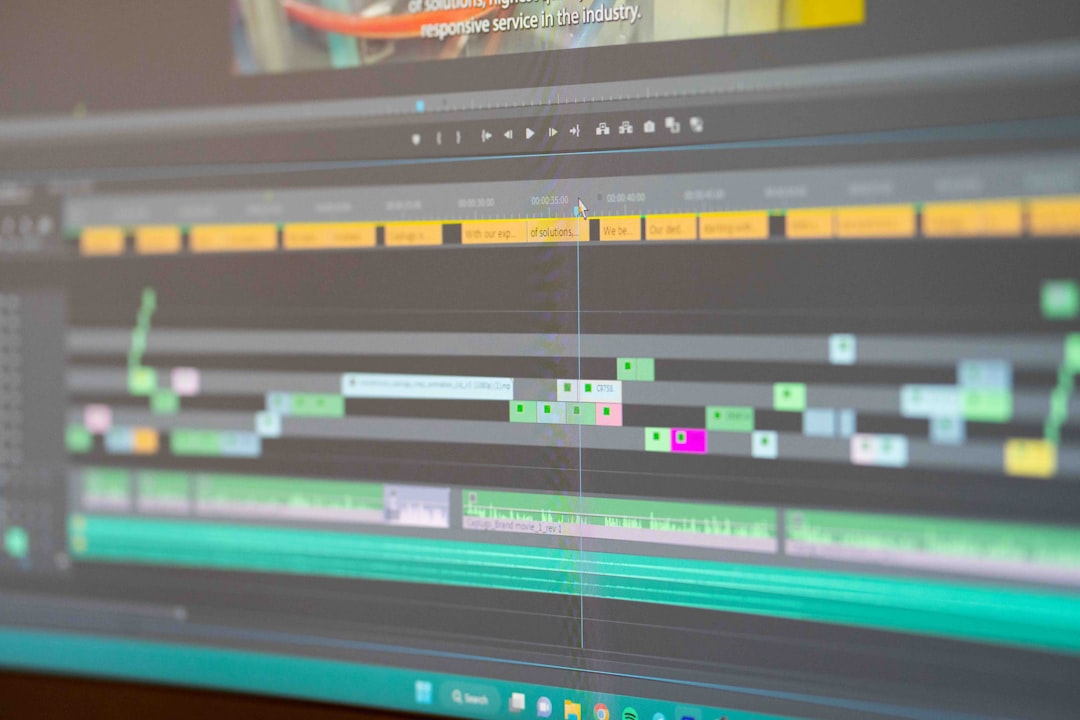
Topaz Video AI v330 boasts a redesigned stabilization model that takes aim at shaky video footage. This new approach employs sophisticated neural networks to stabilize the original frames, effectively reducing camera shake and jitter. It's worth noting that this model operates without the constraint of a pixel-perfect match between input and output files, offering flexibility in handling varied footage. While this update boasts some new features - like an auto-crop tool and adjustable stabilization levels - it's too early to tell how much they actually improve the overall user experience. This latest iteration claims to be more efficient than previous versions and can handle processing offline. It will be interesting to see if this latest version truly sets the benchmark for video enhancement technology as the company claims.
The new stabilization model in Topaz Video AI v330 takes a different approach to addressing camera shake compared to previous versions. While earlier iterations focused on improving general video quality, this latest model specifically targets camera movement, making it more efficient and accurate. The core of this approach is a sophisticated algorithm that analyzes camera motion in real-time. This algorithm differentiates between intentional camera movements and unintended shakes. For example, a smooth pan across a scene wouldn't be altered, while sudden jolts during a handheld shot would be smoothed out. The algorithm relies heavily on machine learning to anticipate and counteract specific types of motion artifacts, which are common in handheld shooting.
A notable improvement is the model's ability to handle extreme zoom levels. Typically, zoom magnifies camera shake, making stabilization more challenging. This new model overcomes this by intelligently analyzing the zoomed image to determine the best stabilization adjustments without sacrificing detail. This addresses a long-standing problem for filmmakers using high-zoom lenses, who previously had to choose between stable footage or high zoom levels.
It’s also important to note that unlike traditional stabilization methods which often crop the frame to minimize shake, this model retains the original image's resolution. The model analyzes the footage's motion characteristics and optimizes the output to ensure stability while minimizing cropping. This approach preserves the artistic framing of the original shot and maintains maximum detail.
The new stabilization model's potential benefits extend beyond improved visual quality. It allows creators to work faster by reducing the amount of time they spend correcting shaky footage in post-production. While the model offers a noticeable improvement, it's still important to understand that no software can completely eliminate camera shake. There are situations, particularly those involving rapid, erratic movements, where the model may struggle. In these cases, the user might need to manually adjust stabilization settings. Nonetheless, this new model represents a significant advancement in the field of video stabilization and will likely become a valuable tool for filmmakers and video editors.
Topaz Video AI v330 New Stabilization Model and Enhanced Video Processing Capabilities Unveiled - Frame Rotation Technique Enhances Smoothness
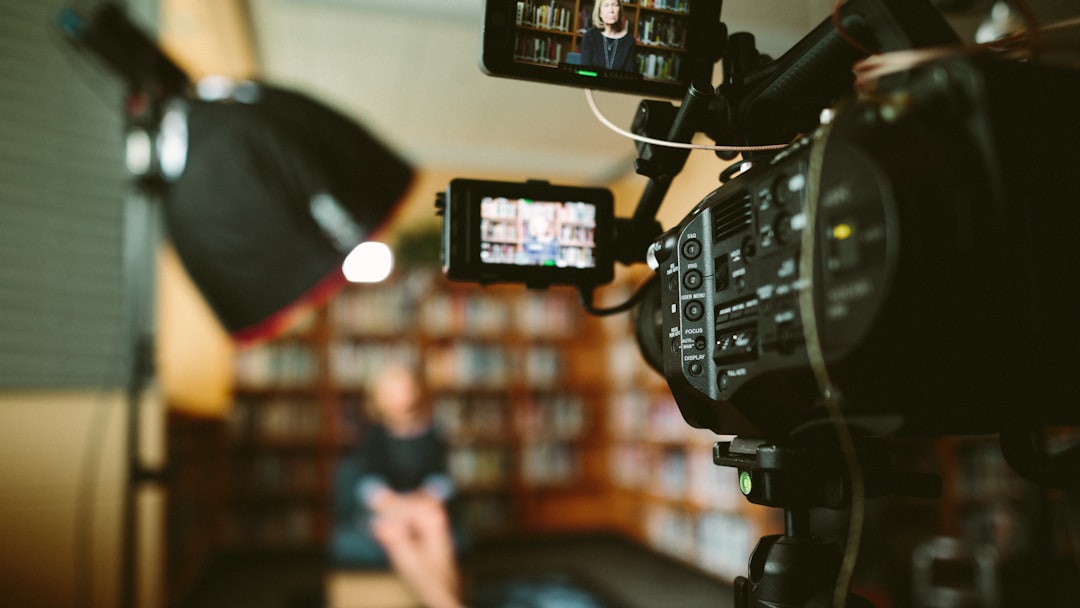
Topaz Video AI v330 features a new stabilization model that utilizes a frame rotation technique. This technique aims to enhance the smoothness of video by stabilizing individual frames. By rotating these frames, the model can stabilize subjects in the video, making them appear more steady in the final output. This approach, however, can result in some pixel discrepancies between the original footage and the stabilized version.
While the new model's primary focus is on reducing camera shake, the update also introduces some additional features, including an autocrop tool and a rotation option within the cropping tool. These new features offer filmmakers increased control over their editing process and greater flexibility in addressing various camera shake scenarios. Although the new stabilization model is a notable advancement, it's important to remember that no software can entirely eliminate camera shake.
The new stabilization model in Topaz Video AI v330 utilizes a Frame Rotation Technique, which is a departure from previous stabilization methods. This technique introduces some intriguing capabilities, although the long-term effects on the overall video enhancement process remain to be fully evaluated.
One notable aspect is the model's ability to detect and correct even minute frame shifts. This precision, measured in fractions of a pixel, contributes to a noticeable increase in the perceived smoothness of the final video. It's interesting to see how this fine-tuning addresses the common problem of shaky footage, particularly in handheld recordings. Additionally, the model employs a dual-axis approach to stabilization, correcting both horizontal and vertical camera movement simultaneously, potentially improving the overall quality of the stabilized video.
Furthermore, the real-time motion tracking used in the model helps minimize the appearance of artifacts that often occur with delayed stabilization. This is achieved by making adjustments as the video is processed, minimizing the time lag that often leads to visible distortions.
The model's integration with lens correction algorithms is particularly noteworthy. By correcting for distortion, it aims to ensure that zoomed shots maintain their accuracy and visual fidelity while also being stabilized. This is particularly relevant for filmmakers working with wide-angle or telephoto lenses where distortion can become a major issue.
Another interesting feature is the model's ability to adapt to varying frame rates within the same footage. While this may seem like a minor point, it is actually quite significant because it enables the model to function consistently even if the frame rate fluctuates during the recording process.
The model also leverages advanced machine learning to predict potential motion artifacts, proactively adjusting the frame rotation to minimize their impact. This proactive approach aims to maintain video quality even when dealing with camera shakes or sudden movements.
One of the key benefits touted for this technique is the reduction of motion blur. The precise frame rotation adjustments aim to minimize the blurring that occurs when the camera shakes, resulting in a clearer, sharper output.
Moreover, the Frame Rotation Technique preserves the original resolution of the footage rather than relying on destructive editing methods. This ensures that the output maintains its full artistic intent and avoids compromising the original composition.
The model also aims to simplify the keyframing process for video editors. By taking care of the stabilization automatically, it allows editors to focus on more creative tasks, minimizing the need for repetitive adjustments for camera shake.
Lastly, the model's ability to work effectively with older footage offers an opportunity to enhance previously unusable material captured with older or less stable equipment. This could be a valuable feature for filmmakers and archivists who seek to restore and preserve older footage.
It will be fascinating to see how this new model develops and the real-world impact it has on the video stabilization process. While the model holds promise, it's crucial to remember that no software can entirely eliminate camera shake. There will inevitably be instances, particularly those involving rapid or erratic movements, where manual adjustments may still be needed. Ultimately, the success of this new technique will depend on how effectively it balances precision with real-world usability.
Topaz Video AI v330 New Stabilization Model and Enhanced Video Processing Capabilities Unveiled - Advanced Video Processing Features Expanded
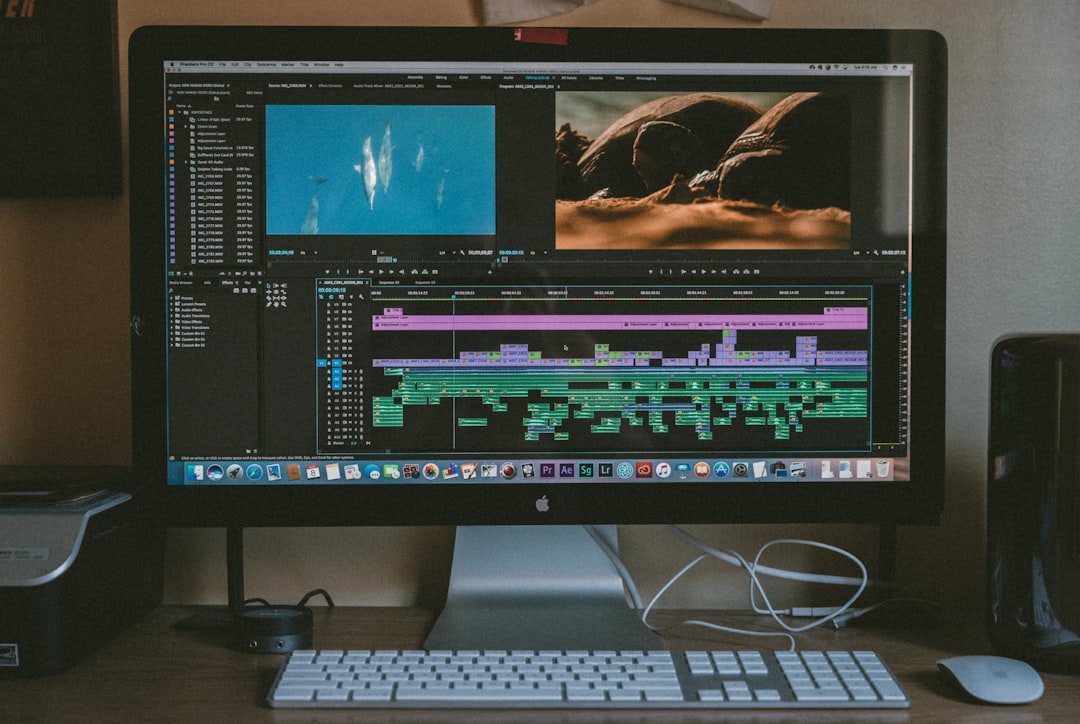
The "Advanced Video Processing Features Expanded" section focuses on the latest improvements in Topaz Video AI v330. This version pushes the boundaries of video editing by implementing a new architecture that supports a range of neural networks. These networks are specifically designed for tasks like upscaling, deinterlacing, motion interpolation, and, of course, shake stabilization.
This version also prioritizes workflow efficiency. It includes parallel processing for faster processing and command line support, catering to users who want more control over the technical details of their video editing process. This should allow for a wider range of user needs to be met. In terms of technical details, Topaz Video AI v330 has improved its compatibility with various codecs and GPUs, making sure the software performs well across different hardware setups.
While these advancements are exciting, it's important to acknowledge that even with these new features, there are limitations. The effectiveness of these advanced processing features may vary depending on the complexity of the video footage being processed. It will be interesting to see how these features perform in real-world scenarios and how well they truly address the specific challenges of video editing.
The latest version of Topaz Video AI, v330, introduces a new stabilization model based on frame rotation. This technique aims to stabilize individual frames by rotating them, creating a smoother overall video experience. This approach retains the original framing, maintaining visual detail, though it may result in minor pixel inconsistencies. It's interesting how the model meticulously analyzes and adjusts individual frames to achieve stabilization, even at a fraction of a pixel level. This precision is especially noticeable in handheld footage, where subtle shakes can become magnified.
The model's adaptability to varying frame rates within a single piece of footage is also impressive. This feature ensures consistent stabilization across different recording speeds, providing flexibility for filmmakers working with a mixture of formats. The integration of lens correction algorithms within the stabilization process is noteworthy. It effectively combats distortion, particularly helpful for productions using wide-angle or telephoto lenses. This enhances visual fidelity, crucial for maintaining professional-quality visuals.
What really sets this model apart is its proactive approach to motion artifact correction. It utilizes machine learning algorithms to predict and counteract such artifacts in real-time, significantly reducing lag that often results in visual distortions. This proactive approach is a clear step up from traditional stabilization methods that typically rely on post-processing corrections.
While the model is a significant improvement in video processing technology, it still has some limitations. For example, it struggles with extremely erratic camera movements. Users may need to manually adjust stabilization settings in such scenarios, highlighting the ongoing need for human oversight, especially in more complex filming conditions. Nonetheless, it will be interesting to see how this model develops and its real-world impact on video stabilization.
Topaz Video AI v330 New Stabilization Model and Enhanced Video Processing Capabilities Unveiled - Local Workstation Performance Optimization

Topaz Video AI v330 includes improvements designed to boost performance on local workstations, especially when stabilizing video footage. This version uses advanced neural networks and sophisticated algorithms to speed up processing, aiming for a more streamlined workflow for video editors. Features like parallel processing and command line support give users more control over their editing, but how beneficial this is really depends on the specific video content being worked on. It also plays nicely with different codecs and GPUs, suggesting it can work well on various hardware configurations, though users should not expect miracles. How effectively these features enhance video quality and streamline editing is something that remains to be seen as the software matures.
The new stabilization model in Topaz Video AI v330, while promising smoother video, introduces some significant performance demands on local workstations. It leverages advanced neural networks for stabilization, resulting in complex processing tasks that can push CPUs and GPUs to their limits. The complexity of these algorithms, with their multi-layered processing and matrix operations, can lead to longer rendering times, even on well-optimized systems.
To achieve seamless video editing workflows, we need to consider the delicate balance between hardware and software. Matching the editing environment with specific codecs and formats can avoid bottlenecks that hinder efficiency. The new features in v330 might require careful integration with existing workflows.
One key consideration is RAM, which plays a crucial role in video stabilization. High-resolution footage, especially with extensive frame analysis, can consume significant memory. Optimizing RAM utilization could improve processing speeds.
While v330 offers offline processing, slow disk read/write speeds can impede the effectiveness of this feature. Using high-performance SSDs becomes essential to avoid bottlenecks during data transfer and processing phases.
Another factor is the reliance on machine learning, which introduces performance overhead. Older hardware lacking support for the latest instruction sets could lead to slower processing speeds. The trade-off between improved video quality and system performance needs careful consideration, especially if upgrading hardware isn't feasible.
Intensive video processing can also cause thermal throttling, impacting performance and potentially damaging components over time. This emphasizes the importance of adequate cooling solutions in high-performance workstations.
While the model adapts to variable frame rates, it can still present challenges. Changes in frame rate might lead to dropped frames or synchronization issues during stabilization, highlighting the need for careful workflow planning.
The user interface may require manual adjustments to fine-tune settings depending on the footage, suggesting that fully automated systems aren't foolproof. Ongoing engineering oversight might be necessary to manage these nuances.
Finally, while sophisticated algorithms are designed to eliminate motion artifacts, they can sometimes introduce new ones due to their complexity. The trade-offs between different stabilization techniques require careful consideration, as removing one artifact may result in the emergence of another, potentially complicating post-production.
Topaz Video AI v330 New Stabilization Model and Enhanced Video Processing Capabilities Unveiled - Improved Codec Support for H264, H265, and AV1
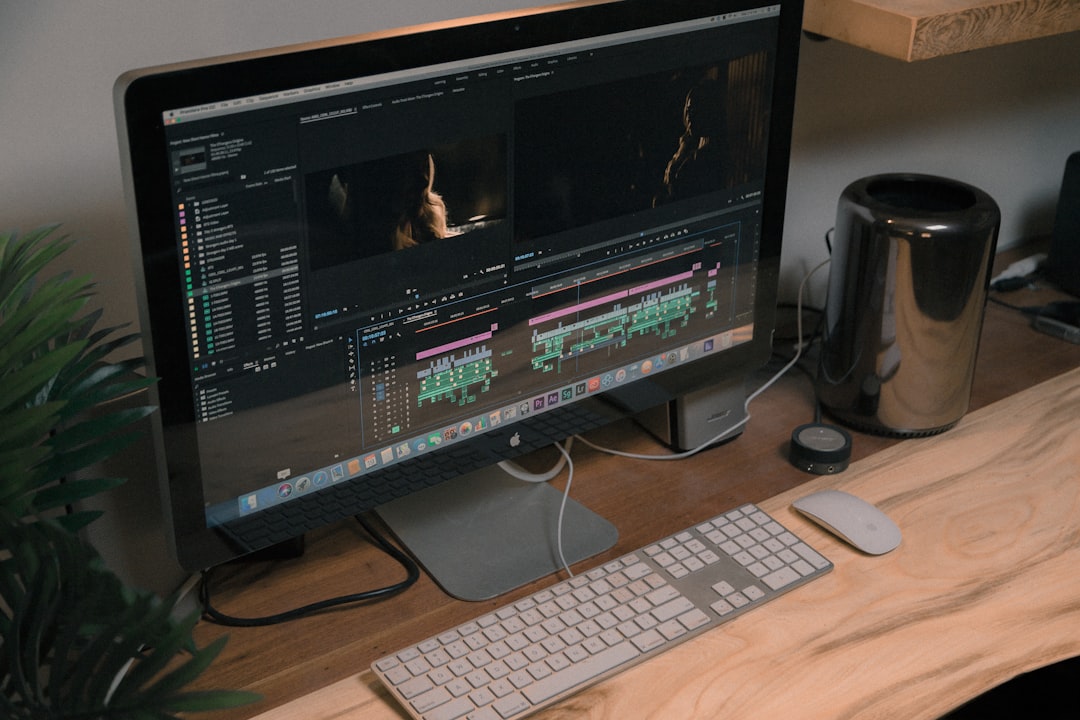
Topaz Video AI v330 boasts improved support for H264, H265, and AV1 codecs, expanding the software's video processing capabilities. H264 continues to be a popular choice, striking a balance between quality and file size. H265, known for its efficiency and smaller files, comes with the downside of requiring more processing power. AV1 stands out with its advanced compression capabilities, offering greater efficiency without a drastic increase in decoding complexity. This updated codec support broadens the software's compatibility with various systems and hardware, reflecting the evolving video landscape where efficient codec performance is crucial for both quality and smooth workflows. However, the practical benefits of these improved codecs might vary depending on the specific footage and hardware setup.
Topaz Video AI v330 has made strides in codec support, integrating H.264, H.265, and AV1 into its workflow. This improvement is promising, as each codec brings unique benefits and drawbacks, making the selection process crucial for various projects.
H.264 remains the workhorse of video compression, commonly used for online and mobile platforms. While it offers decent compression, its files tend to be larger compared to newer codecs. H.265, also known as HEVC, improves upon H.264 with more efficient compression and smaller file sizes, but demands more computational power for both encoding and decoding.
AV1, however, is where things get interesting. This codec is a frontrunner in compression technology, achieving impressive compression gains without significantly increasing decoding complexity. It outperforms H.264 and H.265 in this regard, making it potentially ideal for large, high-resolution videos. However, this benefit comes at a price - AV1 decoding requires significantly more processing power compared to older codecs.
Beyond mere compression, each codec has its own unique characteristics. For example, AV1 is designed to handle unreliable networks, ensuring smooth streaming despite potential packet loss. H.265 and AV1 also have incorporated features to withstand transmission errors, making them valuable for live broadcasts where signal stability is a concern. Furthermore, AV1 boasts scalability, allowing for different resolutions and bitrates within the same stream, making it more versatile than its predecessors.
Despite its technological prowess, AV1's adoption has been slower than expected. Licensing complexities surrounding H.264 and H.265 have slowed their widespread use, and AV1, being royalty-free, has captured the attention of companies like Netflix and Google. They see AV1 as the future of video compression due to its efficiency and lack of licensing fees. However, widespread adoption hinges on hardware acceleration, and while H.264 and H.265 are widely supported by modern hardware, AV1 still requires some catch-up in this regard.
It’s also crucial to consider the inherent differences between codecs. Each codec produces unique artifacts under certain compression settings. While H.264 may introduce block artifacts, H.265 might result in banding. While AV1 strives to minimize such issues, it’s not entirely artifact-free, especially at lower bitrates.
Finally, content encoded with AV1 is seen as more future-proof. As higher resolutions like 8K gain traction, AV1's efficient handling of bandwidth without compromising quality becomes increasingly significant.
Topaz Video AI's inclusion of these codecs is a positive step for users who are seeking more control and customization in their video workflows. It's certainly a step forward in the evolution of video compression, but it's important to consider the various factors, including processing power, licensing, and artifact generation, to determine the optimal codec for specific needs.
Topaz Video AI v330 New Stabilization Model and Enhanced Video Processing Capabilities Unveiled - TensorRT Models Boost NVIDIA Machine Performance
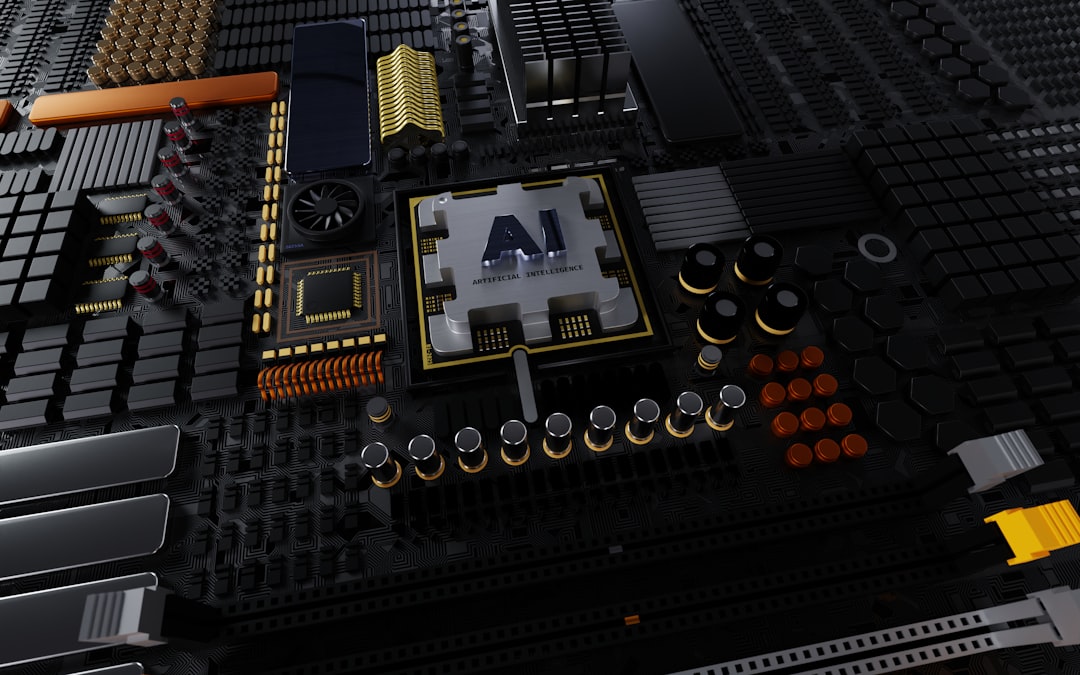
Topaz Video AI v330 boasts performance enhancements through the integration of TensorRT models, specifically designed to accelerate processing on NVIDIA hardware. These models significantly boost video processing speeds, resulting in faster exports and smoother playback, particularly beneficial for editing high-resolution footage. This improvement, however, relies on complex computational techniques, raising concerns about potential compatibility issues and potential bottlenecks in processing efficiency. The trade-off between efficiency and complexity in video processing necessitates careful consideration for optimal performance.
The new version of Topaz Video AI (v330) incorporates NVIDIA's TensorRT, a powerful framework that optimizes deep learning inference performance. This integration significantly boosts processing speeds, particularly for video stabilization. TensorRT utilizes mixed precision calculations, enabling the software to utilize both FP16 and INT8 formats, which can result in inference times that are up to 8x faster compared to using FP32 alone. This approach optimizes the processing of neural networks, making the software faster and more efficient.
One of the advantages of TensorRT is its ability to dynamically optimize models to accommodate varying input shapes, allowing for flexible data processing. This adaptability is particularly useful for real-time applications that require processing of data with changing resolutions or formats. The framework also fuses layers in a neural network, reducing memory bandwidth requirements and increasing throughput, thus enabling faster execution times without sacrificing accuracy. By fully utilizing the parallel processing capabilities of NVIDIA GPUs through CUDA, TensorRT accelerates model inference and reduces latency, making it ideal for applications requiring real-time performance.
TensorRT supports advanced operations, including convolutions, activation functions, and normalization layers. This versatility allows for efficient deployment of models with complex architectures across diverse domains. Its integration with ONNX (Open Neural Network Exchange) format enables seamless deployment of models trained in various frameworks, such as PyTorch or TensorFlow, streamlining the machine learning workflow and enhancing the usability of pre-trained models. TensorRT offers a plugin architecture, allowing developers to introduce custom layers beyond built-in operations. This provides flexibility for researchers and engineers working with specialized networks.
The framework also includes profiling tools to help identify performance bottlenecks and optimize algorithms. This analytical capability is invaluable for fine-tuning models for maximum efficiency. To minimize device memory overhead, TensorRT employs sophisticated memory management techniques. It dynamically allocates and frees memory as needed, ensuring that GPU resources are utilized effectively. This is particularly important for tasks like video stabilization that require significant processing power. TensorRT is designed to be compatible with various NVIDIA GPU architectures, enabling engineers to optimize models for a wide range of hardware and maximize performance across different applications and deployment environments.
It remains to be seen how effectively this integration with TensorRT enhances video quality and streamlines editing in real-world scenarios. While there is promise, it's crucial to carefully evaluate the trade-offs between different stabilization techniques and how they impact performance and final output.
Upscale any video of any resolution to 4K with AI. (Get started for free)
More Posts from ai-videoupscale.com: