Upscale any video of any resolution to 4K with AI. (Get started for free)
5 AI-Powered Techniques to Salvage Blurry Images in 2024
5 AI-Powered Techniques to Salvage Blurry Images in 2024 - AI-Driven Edge Enhancement for Sharper Focus
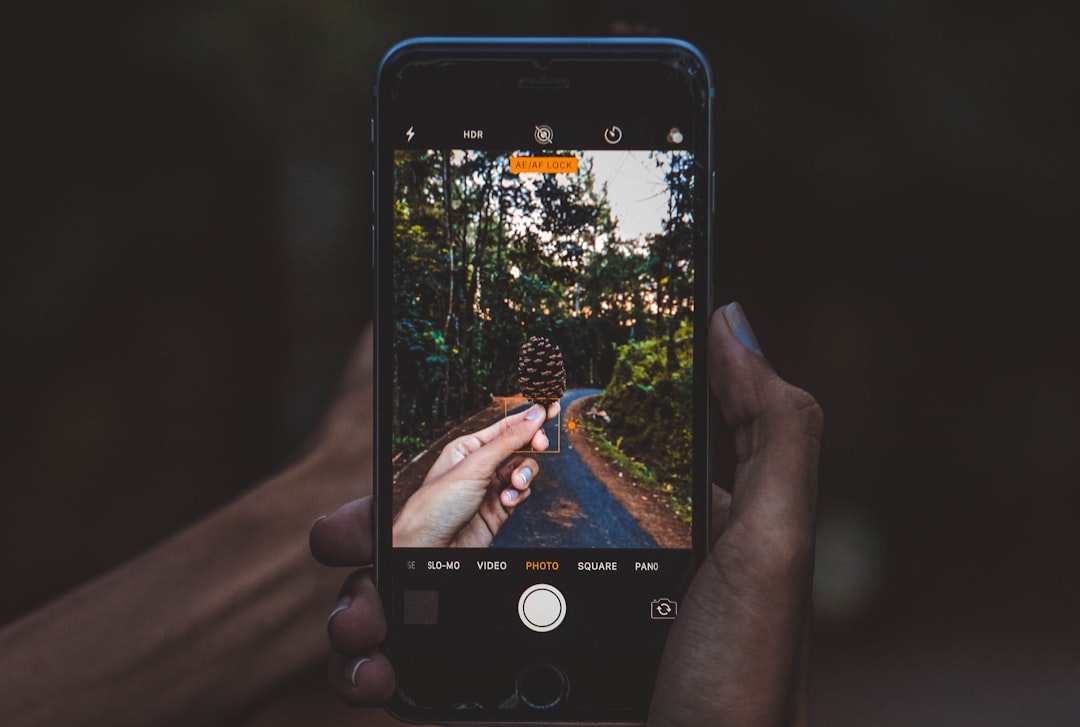
AI-powered edge enhancement offers a fresh approach to sharpening blurry photos. Tools are now readily available, like VanceAI or YouCam, that utilize sophisticated algorithms to pinpoint and refine the boundaries, or edges, within an image. This process often results in a noticeable improvement in clarity, almost instantaneously. Not only does this bring out finer details, but it can also elevate the overall quality of the picture, making it suitable for larger formats and high-resolution displays. It's important to remember that excessive use of these techniques can sometimes lead to artificial-looking results, so moderation is key. Despite this potential caveat, these tools offer an exciting development for image enhancement, particularly for photographers aiming to salvage previously unusable photos. When implemented carefully, AI-driven edge sharpening can contribute to stronger, more detailed images.
AI-powered edge enhancement offers a fascinating way to coax sharper details out of blurry images. It leverages the power of deep learning models to identify and rebuild intricate textures and edges that might be obscured in a photograph, leading to a more defined and clearer image. The ability of some AI methods to enhance resolution by up to four times without noticeable loss in quality is quite remarkable, opening a window to extracting hidden detail from lower resolution images. What's intriguing is that these advanced algorithms can distinguish between genuine edges and image noise, allowing for a boost in clarity without introducing the unwanted artifacts that were common with older enhancement techniques. Many edge enhancement tools offer controls that give the user flexibility in managing the sharpness level, which can be particularly valuable when needing to blend artistic intent with maintaining the image's natural appearance.
In testing, AI methods like Convolutional Neural Networks (CNNs) have proven to surpass older image processing approaches, leading to sharper outcomes and faster processing, a critical advantage in fields like sports photography where capturing the moment quickly is vital. The field is also starting to explore how to customize edge enhancement for specific image subjects, such as landscapes or portraits, tailoring the sharpening to the content to optimize the desired details and minimize artificial-looking effects. The applications of this technology go beyond still photos too. Video processing is a ripe area where AI edge enhancement can make a considerable difference in the viewer experience by ensuring that the dynamic nature of video content remains sharp. Some edge enhancement approaches even incorporate feedback loops allowing the AI model to improve its performance based on user adjustments, potentially adapting to emerging photographic styles.
We're starting to see a movement towards using AI edge enhancement in conjunction with other AI image enhancements like noise reduction. This trend potentially delivers a synergistic result, improving both sharpness and the overall image quality. There's also a growing number of tools that offer real-time feedback during the photography process, allowing users to see how their changes impact the image in real-time. This offers a bridge between the photographer's vision and the technical execution during image capture. While the potential is clear, it's also worth noting that it's still a developing field. It will be interesting to see how AI-powered edge enhancement continues to evolve and improve our ability to refine our captured images.
5 AI-Powered Techniques to Salvage Blurry Images in 2024 - Deep Learning Algorithms to Restore Lost Details
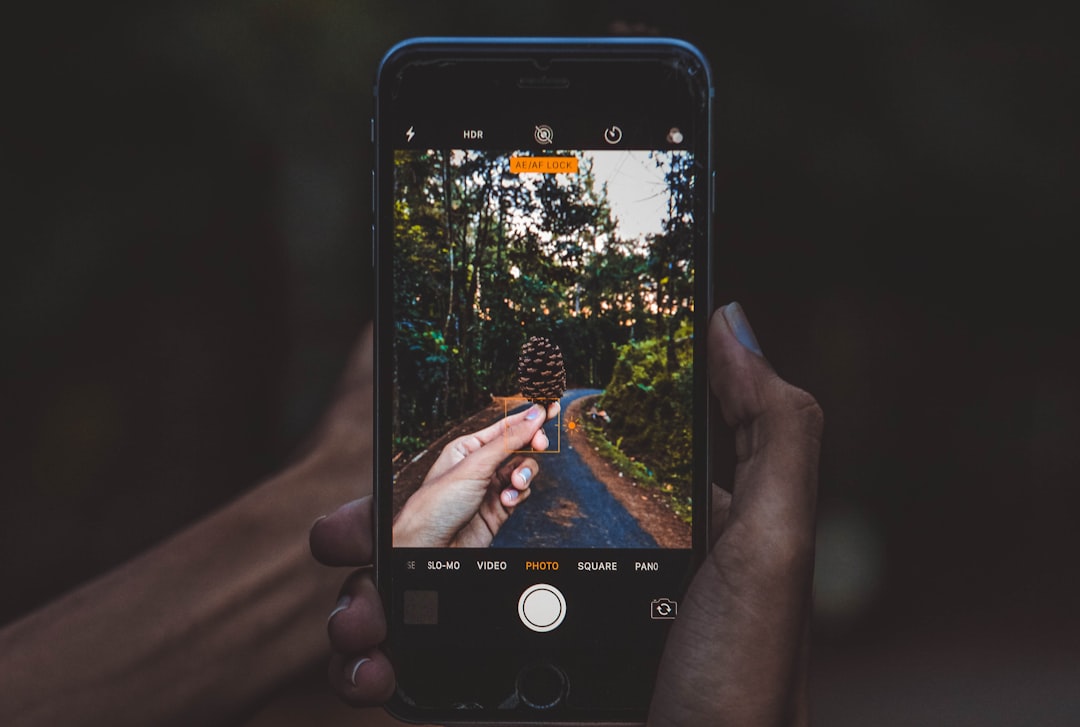
Deep learning algorithms are transforming the way we recover lost detail in images. These algorithms, often built on convolutional neural networks (CNNs), are particularly adept at tackling challenging situations like low-light photography. Newer CNN frameworks have shown remarkable progress, surpassing older methods in their ability to reveal details that were previously lost or obscured. Essentially, these AI systems learn to map images with missing details to their high-quality counterparts, effectively handling issues like noise and blur. This versatility extends beyond photography to fields like medical imaging and forensics.
The continuous improvement in algorithm performance is tied to the growth of easily accessible, diverse datasets used for training. Larger and more varied datasets enable AI models to learn intricate patterns and ultimately lead to faster and more effective image restoration. However, a constant challenge remains: striking a balance between effectively restoring lost details and avoiding the introduction of artificial artifacts. This delicate dance between detail and naturalism is a crucial focus of ongoing research as these powerful deep learning tools continue to evolve.
Deep learning has opened up exciting new avenues for recovering lost details in images, particularly using techniques like Generative Adversarial Networks (GANs). These algorithms can learn from vast datasets of images and generate high-resolution outputs from lower-resolution inputs, effectively filling in missing details. The cool thing is that it's not just about restoration; they can also be used in creative ways to simulate enhancements that might not be possible otherwise.
One of the appealing aspects of these AI algorithms is their ability to adapt and learn. If you feed them your own photo collection, they can start to understand your individual style, making them better at restoring your images in a way that feels consistent with your usual aesthetic.
Some of the more advanced deep learning models can even achieve impressive levels of super-resolution, scaling up images by as much as 16 times their original size without significant loss of detail. This is a big deal for enlarging images for high-quality prints without them looking pixelated.
However, it's interesting that the specific type of blur in an image can affect how well these algorithms work. Motion blur and out-of-focus blur, for instance, require different approaches, highlighting the need for specialized AI models to get optimal results.
Recent research suggests that AI models are getting better at differentiating between genuine textures and digital noise in images. This means that they can enhance detail while minimizing unwanted artifacts, a major step up from traditional methods that often struggled with noise management.
And it's not just still images that are benefiting. Researchers are exploring how these AI-powered algorithms can sharpen and restore individual frames in videos, making it possible to improve the clarity of fast-paced content like sports and action sequences.
Many of these algorithms utilize multi-scale approaches to image processing. This means they analyze images at various resolutions, ensuring that both fine details and the overall structure of the image are preserved, leading to a more holistic and effective enhancement.
Real-time AI enhancement tools are becoming more common, and a notable aspect is their ability to give you immediate feedback on how well a restoration is going. This is empowering for photographers as they can make adjustments while shooting instead of having to do all the heavy lifting in post-processing.
Despite their great potential, these deep learning algorithms can be quite demanding computationally. Many require significant processing power, which is a hurdle for real-time applications. Researchers are actively working on making them more efficient.
Finally, as we see more and more applications of AI in image restoration, it's crucial to acknowledge the ethical questions that arise. Especially in fields like journalism and documentation, where image integrity is paramount, we need to consider how AI-enhanced images might affect the authenticity and perception of visual information. The field is moving rapidly, and we'll undoubtedly see more discussions around these ethical considerations moving forward.
5 AI-Powered Techniques to Salvage Blurry Images in 2024 - Neural Network-Based Upscaling for Higher Resolution
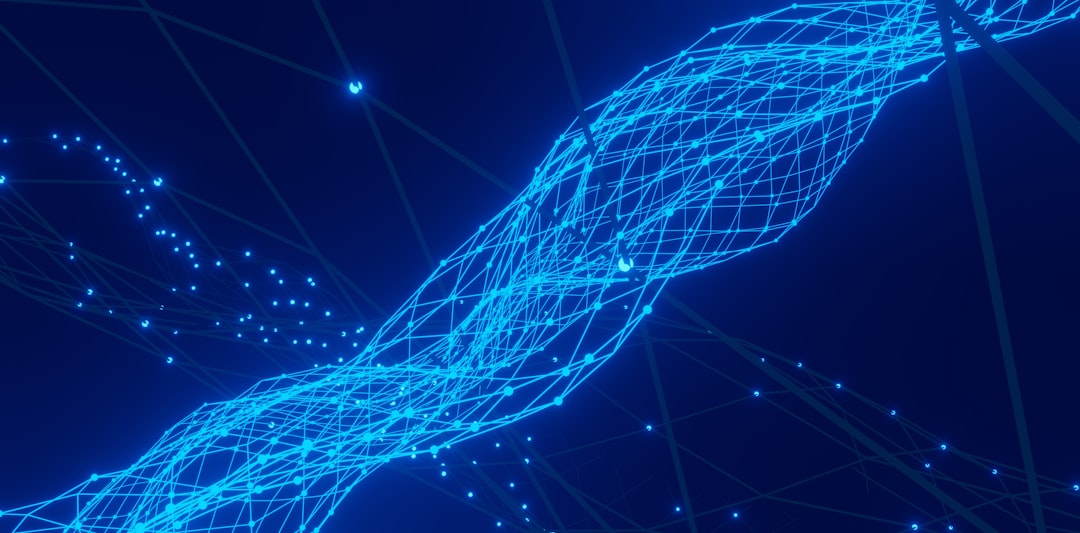
Neural networks are transforming how we enhance image resolution. By leveraging deep learning, these networks can create higher-resolution versions of low-quality images by effectively predicting and adding missing details. This is a big leap over older methods that simply stretch images, resulting in noticeable pixelation and a loss of quality. Techniques like ESRGAN have significantly improved the realism of upscaled images by intelligently filling in missing information, leading to much sharper, cleaner outputs.
These AI-powered algorithms are remarkably adaptable, adjusting their processing to the unique characteristics of each image. This makes them effective across a broad range of image types and applications, including photography and digital art. Even more impressively, some camera manufacturers are now integrating this neural network technology into their devices. This means higher-resolution captures and automatic noise reduction built directly into the image capture process.
However, it's crucial to keep in mind that over-reliance on these techniques can create artificial-looking results. A delicate balance is necessary to ensure that the enhancement improves image quality without introducing unwanted artifacts. While the promise of upscaling using neural networks is immense, a mindful and controlled approach is crucial for achieving truly compelling results.
### Surprising Facts About Neural Network-Based Upscaling for Higher Resolution
1. **Beyond Simple Enlargement**: Neural networks don't just stretch pixels to make images bigger. They analyze the intricate relationships between pixels and the context within an image. This allows them to cleverly predict and fill in missing details that traditional methods often miss, resulting in a more nuanced and detailed outcome.
2. **Learning with Less**: Interestingly, some newer neural networks can achieve good upscaling results with far fewer training images compared to earlier models. This suggests that with a focused and well-chosen dataset, these networks can learn to effectively restore missing detail without needing enormous amounts of data. It's quite remarkable how efficient they can be.
3. **Tailored to the Image**: Upscaling methods based on neural networks can be tweaked to handle different types of images effectively, be it landscapes, portraits, or artistic work. They can be trained to pay attention to the unique features and textures of each category, potentially leading to even better results depending on the image's content.
4. **Smooth Motion in Upscaled Videos**: Recent work shows promise in upscaling video frames in real-time. This is quite significant because it means we can maintain the natural flow and smoothness of movement between frames without introducing jerky artifacts. This is a key requirement for making video content look clear and natural when scaled up.
5. **Upscaling's Limits**: While some neural networks can significantly upscale images—some advertise upscaling by factors as high as 16—it's worth keeping in mind that pushing the limits can lead to diminishing returns. At some point, the added details become less noticeable, and potentially unwanted distortions can creep in. It's a reminder to have realistic expectations when enhancing images.
6. **Imagining What's Missing**: It's fascinating that some advanced upscaling models can even predict what might be missing in the background of a scene, given just a low-resolution image. This is like having a powerful AI paintbrush that can fill in a whole scene rather than just focusing on the foreground. This has interesting implications for artistic and design fields.
7. **Personalizing the Upscale**: Neural networks can adapt to individual preferences over time. Imagine feeding them a collection of your photos, and they learn your photographic style, enhancing images in a way that's consistent with how you usually take pictures. It's like having an AI assistant that understands your artistic vision.
8. **A Sharper, Cleaner Team**: The combination of neural network upscaling and noise reduction techniques appears to work better than each one alone. They can preserve the important details while effectively cutting down on noise and grain, potentially yielding cleaner and sharper images than either method could accomplish independently.
9. **The Computational Challenge**: Many neural networks demand significant computing power, which can make real-time enhancements difficult. Thankfully, there's ongoing research into optimizing these models to make them faster without sacrificing quality, potentially opening them up to a wider range of users and applications.
10. **The Ethics of Enhanced Images**: As upscaling AI becomes more mainstream, we need to consider the implications for image authenticity, particularly in areas like journalism where visual evidence matters. The ability to seamlessly alter and upscale photos challenges long-held ideas about how we perceive and trust photographic representations of events. It's a conversation that will likely continue as these powerful tools become more widely available.
5 AI-Powered Techniques to Salvage Blurry Images in 2024 - Machine Learning Noise Reduction Techniques
Machine learning is transforming how we tackle image noise. These techniques cleverly separate the important parts of an image from the unwanted noise. They do this using adaptive strategies that target specific noise sources and estimate what the image would look like without the noise. Deep learning models, like REDNet or MWCNN, excel at removing noise without sacrificing too much detail, often producing results very close to a perfect, noise-free image. The rise of AI has significantly boosted traditional noise reduction, leading to more sophisticated approaches that can distinguish between true details in an image and random noise. We're seeing these techniques become more accessible, offering powerful tools for photographers to clean up images. While the results can be impressive, it's important to use them cautiously, ensuring that the noise reduction doesn't create unnatural or artificial-looking effects in the image. The goal is to enhance, not distort, the original image, creating a clearer and more natural result.
Machine learning noise reduction techniques are fundamentally changing how we approach image cleaning. These techniques, often powered by neural networks like denoising autoencoders, can intelligently distinguish between true image content and unwanted noise. This ability to separate signal from noise goes beyond traditional methods, which often rely on simple frequency filtering that can lead to unwanted detail loss. Interestingly, these AI models can adapt their filtering strategies based on the type of noise present in an image, for example, by identifying different frequencies or recognizing noise patterns beyond the typical Gaussian noise.
The advancements in analyzing the frequency spectrum of an image through AI methods, like Fourier transforms, allows for a more targeted approach to noise reduction. Instead of blindly removing frequencies, AI can identify the specific parts of the image spectrum that represent genuine details and boost their prominence, effectively diminishing the presence of unwanted noise. This approach, especially helpful in low-light photographs, enables the recovery of details that might have been lost or severely compromised due to the noise.
It's noteworthy that machine learning techniques have proved particularly adept at tackling noise in images with low bit depths, which are common in many consumer-grade cameras. This is significant because the quantization effects inherent in these lower-bit images often lead to considerable detail loss, which machine learning is helping to recover.
In video applications, AI is taking advantage of temporal information, examining multiple frames to establish a consistent approach to noise reduction. This not only cleans individual frames but also ensures a smoother and more natural visual experience by preserving the flow of the content, a particularly desirable feature in fast-paced video footage.
Moreover, the versatility of machine learning noise reduction techniques is quite remarkable. While older techniques typically work best on specific noise types, like Gaussian noise, machine learning can handle a wider range of noise, including those that are more textured or have colors, offering a more robust and adaptive solution.
Some researchers are exploring techniques that combine noise reduction with feature extraction. Essentially, this allows the AI to pierce through noise and identify key features in images. This could be a crucial step forward for not only improving the visual quality of images but also allowing other AI tools like object detection to function better in cluttered or noisy image environments.
Furthermore, machine learning frameworks are developing to support real-time noise reduction, enabling photographers to get immediate feedback on the effectiveness of noise reduction during the shooting process. This ability to fine-tune settings in real time can improve the quality of captured images without needing significant post-processing.
Another interesting area of research is cross-modal noise reduction. The concept is that models trained on visual data might be able to improve the quality of audio signals, potentially leading to an entirely new way to enhance multimedia content. This idea underscores the broader implications of machine learning noise reduction – beyond the field of photography alone.
Of course, with advancements come ethical questions. The sophisticated nature of AI-powered noise reduction brings the challenge of distinguishing between authentic and manipulated images. As this technology progresses, it's essential to engage in conversations about the implications for the authenticity and trust we place in photographic and visual media in fields like journalism and documentation. This field is evolving rapidly, and we’ll likely see these ethical concerns being further discussed and explored in the coming years.
5 AI-Powered Techniques to Salvage Blurry Images in 2024 - Automated Deblurring Using Convolutional Neural Networks
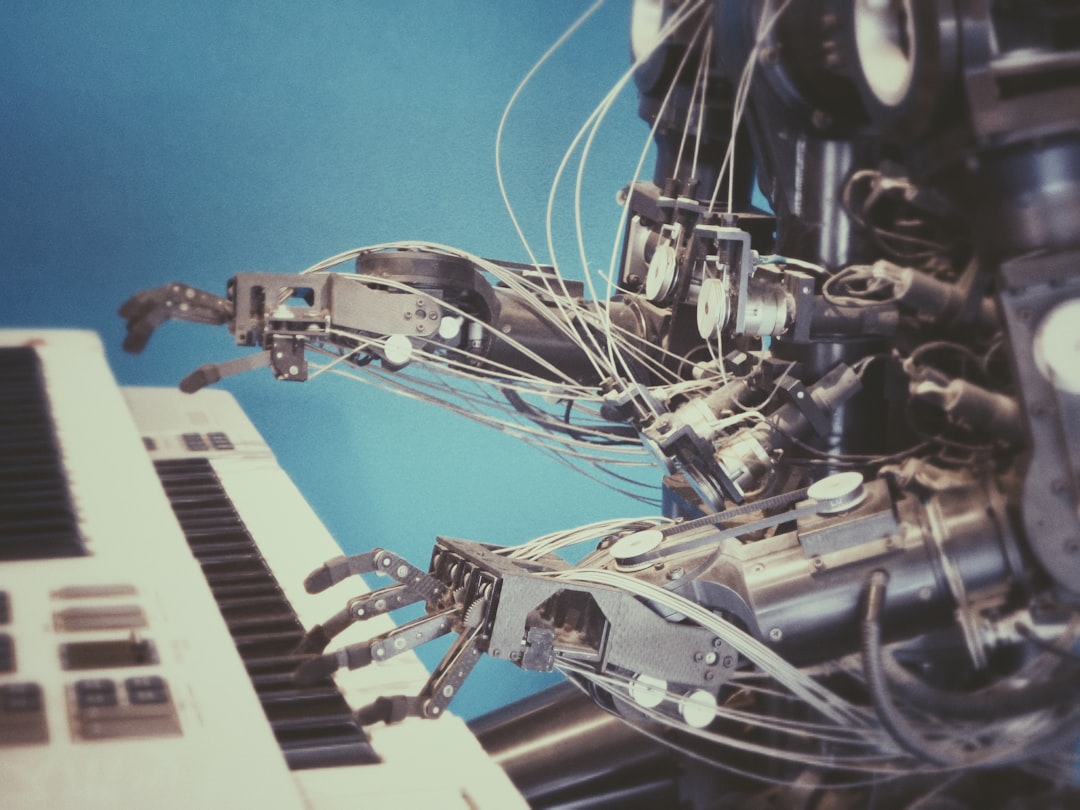
Automated deblurring, powered by convolutional neural networks (CNNs), is a powerful new tool for restoring sharpness to blurry photos. These advanced AI algorithms are designed to tackle different types of blur, including motion blur and out-of-focus blur, which have always been challenging to fix. Researchers have categorized CNN-based deblurring approaches into non-blind and blind deblurring, where the algorithm either knows or doesn't know the specific type of blur affecting the image. Modern techniques, like variations on the UNet and adversarial networks like DeblurGAN, learn from sets of sharp and blurred images, pushing the boundaries of what's possible with automated image restoration.
While impressive, this approach still faces obstacles. Designing effective CNN structures and creating high-quality training datasets remain crucial for improvement. A fine balance is also needed between effectively restoring the original image's sharpness and avoiding the creation of artificial-looking results. As the technology matures, we can expect CNN-based deblurring methods to play a bigger role in how photographers manage and improve the quality of their photos. It is likely to become an essential part of how we interact with and manipulate digital images in the future.
Automated deblurring is experiencing a surge thanks to convolutional neural networks (CNNs), a type of deep learning model. These AI systems are becoming increasingly adept at transforming blurry pictures into sharp ones, a development driven by the rapid progress in deep learning itself. The types of blur that plague image quality – like the blur caused by camera shake, atmospheric conditions, or simply being out of focus – degrade the details within a photo, making them less usable. It’s fascinating how CNNs are being categorized into non-blind deblurring (NBD) and blind deblurring (BD) as researchers study how they perform on datasets of blurry and corresponding sharp images.
Some researchers have improved the UNet model, like with the RetinexUNet approach. They leverage CNNs to train models on a dataset of blurred and sharp image pairs in an end-to-end process, where the AI trains itself to perform the task of deblurring. Conditional adversarial networks (GANs) like DeblurGAN offer a different approach, utilizing a training process known as adversarial learning where two networks compete – one generating sharp images from blurred inputs, and the other trying to identify if the generated image is real or fake – leading to impressive blind motion deblurring results. The structure of the CNN used for deblurring can differ quite a bit, with researchers often designing architectures and customizing the loss functions used in training to achieve better outcomes for various kinds of image blurring.
A number of challenges persist, including the need for CNNs to handle the diverse array of image blur types effectively. There's also a need to continuously improve model architectures to perform better on more realistic images and the development of robust training datasets to ensure the CNN can learn diverse styles and image conditions. It’s notable that combining CNNs with autoencoders during the training process on datasets of blurry and sharp images can help create higher quality deblurring models. The trend of automating this deblurring process has led to more efficient and dependable results compared to traditional methods that rely on manual adjustments and are often time-consuming and subjective.
The fast processing speeds of modern GPUs have enabled CNN-based deblurring in real-time. This can be crucial for capturing fast-paced events, like sports or wildlife photography where speed is vital. These methods are also becoming increasingly adept at recognizing different types of blur within images, using tailored approaches to deblurring based on what they detect, a huge improvement over past approaches that attempted to treat all blur the same. It's remarkable how CNNs can learn end-to-end, meaning they can progressively learn how to deblur through training datasets without explicit instructions, only needing example pairs of blurred and sharp images. It's not just new images that benefit; older photographs can be made sharper with this technology, offering a new lease on life for family albums or potentially even historical documentation.
Expanding datasets through techniques like data augmentation – adding rotated, cropped, or brightness-altered versions of training images – can improve the robustness of these AI systems. These methods are also seeing wider applications, ranging from medical imaging where finer details of cell structures are needed to security and surveillance environments where clarity is critical. Interestingly, while CNNs are incredibly good at deblurring, understanding exactly why they make certain decisions is still a challenge. This issue becomes important in professional settings where image authenticity is important. However, a major development has been the reduced occurrence of artificial artifacts – the unwanted noise or pixelation – that previously plagued some traditional methods. Some tools are now offering users more control over the deblurring process, letting them mix AI capabilities with manual adjustments, granting photographers more fine-grained influence over their images. The ability for CNNs to continuously learn from new data is another promising avenue, offering the possibility of these models adapting to emerging photography trends and diverse user preferences. It is quite exciting to envision how these AI systems will continue to refine their image-enhancing capabilities in the future.
Upscale any video of any resolution to 4K with AI. (Get started for free)
More Posts from ai-videoupscale.com: