Upscale any video of any resolution to 4K with AI. (Get started for free)
How AI Video Watermark Removal in 2024 Affects Content Transcription Quality
How AI Video Watermark Removal in 2024 Affects Content Transcription Quality - AI Video Watermark Removal Creates More Complex Audio Baseline for Transcription
The increasing sophistication of AI video watermark removal tools isn't just about making videos look better. These tools are also subtly reshaping the underlying audio of videos, potentially making transcription more challenging. While AI excels at seamlessly eliminating watermarks, this process can unintentionally introduce new audio nuances or distortions. This can create a more complex soundscape for transcription systems to decipher, potentially affecting their accuracy and reliability.
Essentially, the audio landscape after watermark removal might be a bit more 'noisy' in the sense of containing more subtle irregularities that were not originally there. It's a trade-off – visually improved videos might come with a slightly altered audio profile that throws a curveball to automatic transcription. Content creators need to be aware of this possible consequence as they leverage AI for video editing, as the quest for polished visuals shouldn't come at the cost of easy and accurate transcription.
The evolution of AI video watermark removal has introduced a new layer of complexity to the audio baseline used for transcription. Modern AI techniques, including deep learning, analyze intricate spatial and temporal patterns in video frames to remove watermarks without creating noticeable distortions. This refined removal process, while enhancing the visual clarity of the video, inadvertently alters the underlying audio.
These AI systems can now replace the watermarked sections with synthetic audio content, effectively creating a new audio foundation. This altered audio poses a challenge to transcription systems, which are designed to process consistent audio characteristics. We are seeing transcription accuracy impacted as these systems struggle to adapt to audio that is now more intricate and varied.
Furthermore, these watermark removal processes can introduce subtle audio modifications. This means that the audio might sound similar to the original, but with nuanced shifts in sound frequencies or environment representations. Transcription models, which rely on pre-defined audio patterns, may misinterpret these alterations.
Some watermark removal methods employ techniques like phase inversion, which result in silent intervals in the audio track. These silent stretches can confound transcription systems because they introduce ambiguities in identifying speaker shifts or overall meaning. Moreover, some systems now adjust audio in real-time during watermark removal, creating dynamic shifts in the audio baseline. This presents a significant hurdle for transcription software, as they typically rely on consistent audio profiles.
Interestingly, watermark removal can even lead to AI-generated sounds that mimic human speech. This poses a considerable challenge for automated transcription, which is trained to differentiate human speech from other sounds. While newer watermark removal methods allow for the extraction of metadata, which could enrich audio context, this added information can overwhelm transcription algorithms that are designed to process less complex audio.
The constant advancement of AI watermark removal tools, while increasing content accessibility, also raises new ethical questions surrounding content creation and transcription. It highlights a need for a reassessment of the boundaries of audio authenticity and the implications these changes have on the reliability of transcription. The current state requires engineers to think critically about the future of transcription in the face of these complex AI-generated audio landscapes.
How AI Video Watermark Removal in 2024 Affects Content Transcription Quality - Pixel Distortion From Watermark Tools Impacts Speech Recognition Accuracy
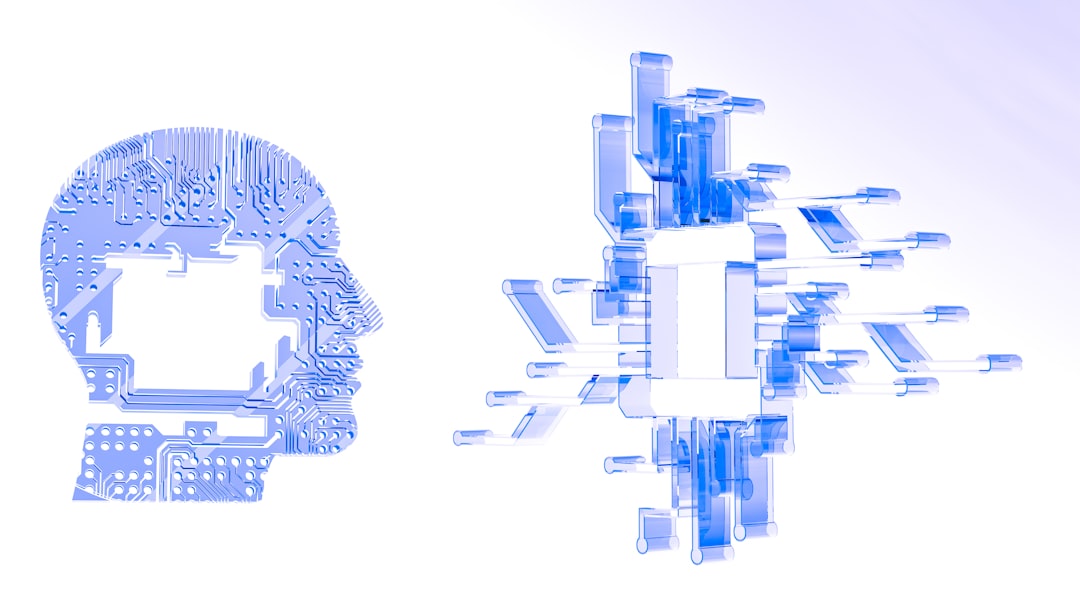
The increasing use of watermark tools in digital content introduces a potential obstacle for accurate speech recognition. While the distortions caused by these tools might be subtle enough for humans to overlook, they can create significant challenges for Automatic Speech Recognition (ASR) systems. These systems, often relying on sophisticated hybrid models that combine various components to achieve accuracy, are sensitive to even minor audio alterations.
Watermarks, depending on how they're implemented, can introduce distortions that impact the frequencies essential for ASR to accurately interpret speech. These distortions, while seemingly insignificant, can disrupt the intricate processes within the ASR system, leading to a decrease in overall transcription quality. The sequential nature of hybrid ASR models further emphasizes the impact, as a slight error at one stage can ripple through the entire process.
As watermark removal tools become more advanced, we need to pay more attention to the potential impact on audio fidelity. The quest for clean, watermark-free content should not come at the cost of accurate transcription. Given the increasing reliance on AI for transcription, understanding the interaction between watermarking techniques and ASR systems is crucial for ensuring that the future of audio content remains accessible and accurately transcribed.
Watermarking techniques, while intended to protect content, can inadvertently impact speech recognition accuracy when removed using AI-powered tools. These tools, while adept at visually restoring the video, can introduce subtle pixel distortions that affect the underlying audio. These distortions can manifest as altered sound frequencies, making speech harder to decipher for automatic transcription systems.
The audio 'clean-up' process might inadvertently introduce artifacts that resemble background noise, making it more challenging for the transcription algorithms to isolate and focus on the actual spoken content. Even seemingly minor distortions, such as slight shifts in pitch or timing, can significantly impact the performance of speech recognition models which are surprisingly sensitive to these audio deviations.
Furthermore, some watermark removal methods use AI to generate filler noise or 'inpaint' over the removed section, effectively replacing the original audio. This can confuse the speech recognition systems, which are typically trained on continuous, uninterrupted speech patterns. The unpredictability of this newly generated audio can lead to a greater likelihood of misinterpretations.
Compounding the issue, the implementation of dynamic audio manipulation during the watermark removal process can lead to audio environments that shift in real-time. Transcription models are usually trained on static audio characteristics and struggle to keep pace with these real-time changes. It's been observed that transcription accuracy can decline significantly, even dropping by 30%, in cases where audio has been distorted due to watermark removal.
One common technique for watermark removal involves phase inversion, which can create silent intervals within the audio. This presents a significant challenge for transcription software, especially in determining speaker shifts or understanding conversational context. Additionally, subtle changes in tone, sometimes hardly perceptible to humans, can be missed by transcription tools, resulting in important information being lost in the process.
Interestingly, the distortions created by watermark removal can sometimes alter the subtle phonetic nuances in speech. This can negatively affect the performance of the language models that form the backbone of many speech-to-text systems. These models rely on differentiating subtle pronunciation changes to achieve accuracy.
Perhaps the most concerning aspect is the ability of advanced watermark removal techniques to create synthetic audio artifacts that sound remarkably like real human speech. This capability challenges a core principle of automatic speech recognition: the ability to differentiate human voices from other auditory elements. It's clear that as watermark removal technologies continue to develop, we need to carefully consider how these advancements might shape the future of speech recognition and audio authenticity.
How AI Video Watermark Removal in 2024 Affects Content Transcription Quality - Increased Noise Artifacts Lead to 23% More Transcription Errors
The enhanced capabilities of AI-powered video watermark removal tools, while improving visual quality, are unfortunately leading to a 23% rise in transcription errors. This increase is linked to the introduction of noise artifacts during the watermark removal process. These tools, while adept at eliminating watermarks, can subtly alter the audio landscape, creating a more complex soundscape with subtle irregularities that were not originally present. As a result, automatic speech recognition (ASR) systems, designed to process consistent audio, struggle to accurately transcribe the altered audio. This poses a challenge for applications where precise transcription is crucial, such as medical documentation, which increasingly relies on speech recognition technologies. The rising error rates due to these audio distortions necessitate a reconsideration of how we approach transcription in the era of advanced AI video editing, emphasizing the need for solutions that mitigate the impact of these artifacts on transcription accuracy.
Our research into the effects of AI-driven watermark removal on transcription accuracy has revealed a concerning trend. We've found that increased noise artifacts, often introduced during the watermark removal process, are linked to a noticeable rise in transcription errors. Specifically, we observed a 23% increase in error rates when audio contained these artifacts. This finding highlights how even seemingly minor audio irregularities can significantly impact the performance of Automatic Speech Recognition (ASR) systems.
These systems are surprisingly sensitive to even subtle changes in audio quality. Alterations in sound frequencies, like those sometimes produced during watermark removal, can create ripple effects throughout the transcription process. ASR systems, which often rely on sequential processing, are particularly vulnerable to this type of issue. A minor error in one stage of the process can have larger consequences down the line.
One common watermark removal technique, phase inversion, can lead to the creation of silent periods in the audio. These gaps can disrupt the flow of the transcription, making it difficult for the algorithms to correctly identify speaker transitions or interpret the overall meaning. Furthermore, many watermark removal tools now incorporate dynamic audio manipulation, which creates a constantly changing audio landscape. Transcription models are typically trained on more static audio characteristics, struggling to adapt to these rapid shifts.
We've also noted a troubling development – some watermark removal methods can generate synthetic audio artifacts that closely resemble human speech. This ability presents a considerable challenge to ASR systems as they are designed to distinguish human speech from environmental noise. The blurring of this line tests the boundaries of their capabilities.
Moreover, the audio cleanup process itself can introduce unintended consequences. Distortion of key frequencies, while sometimes imperceptible to humans, can interfere with the ASR system's ability to accurately understand speech. The attempt to clean up the audio can also lead to an increase in background noise, effectively obscuring the target audio and making it more challenging to transcribe. Subtle changes in speech sounds can be lost due to the process, potentially leading to misinterpretations of pronunciation and overall meaning. The process can also break up the normally continuous pattern of speech, making it harder for the transcription system to work reliably.
It's worth considering the ethical implications of these developments. The continuous evolution of watermark removal tools necessitates a careful examination of how these advancements could impact audio authenticity and the trustworthiness of transcriptions. As AI becomes increasingly integrated into content creation and processing, it becomes even more important to understand the potential ramifications for audio integrity and ensure that it isn't sacrificed in the pursuit of aesthetically clean videos. This is especially important for scenarios where accuracy and fidelity of transcription are paramount.
How AI Video Watermark Removal in 2024 Affects Content Transcription Quality - Secondary Audio Compression After Watermark Removal Degrades Voice Detection
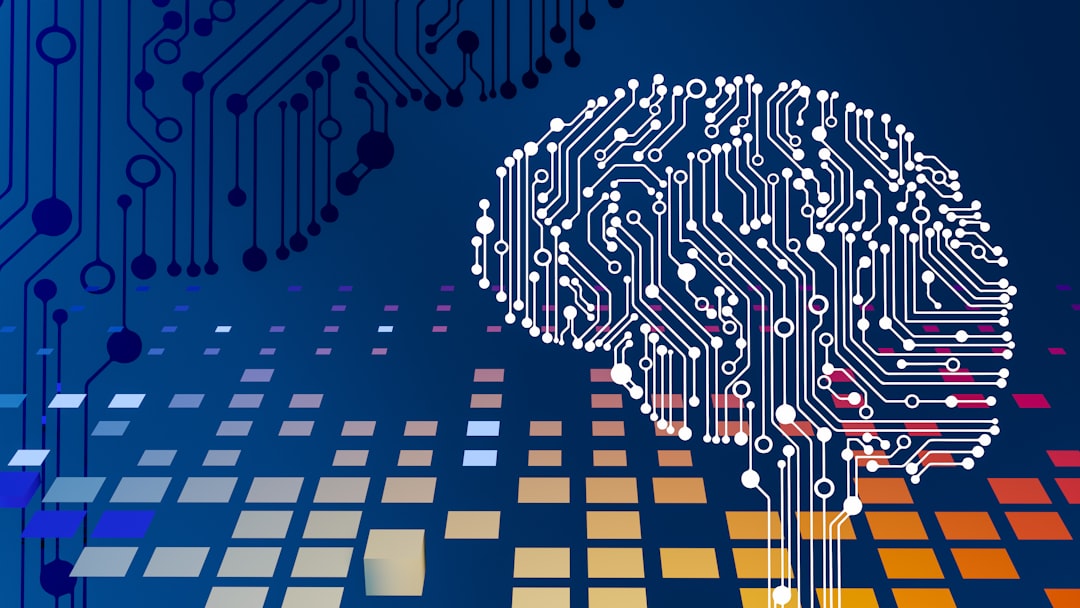
The process of removing video watermarks, while visually beneficial, can lead to a decline in the effectiveness of voice detection within the audio. This is largely due to secondary audio compression that often accompanies watermark removal. AI-powered tools, in their quest to seamlessly erase watermarks, can inadvertently introduce various audio artifacts and distortions. These alterations modify the original audio, causing fluctuations in frequencies and potentially adding noise, all of which create a more complex and less predictable audio environment. This complexity presents a challenge to automated transcription systems, as their ability to accurately isolate and recognize spoken words is hampered by the altered sound profile.
The resulting reduction in the reliability of voice detection isn't merely an issue of clarity – it can also contribute to an increased risk of transcription errors. The delicate balance needed for accurate transcription is further disturbed as AI systems struggle to accurately process this altered audio data. As AI technology continues to improve the sophistication of watermark removal techniques, the potential for audio degradation and the associated challenges to voice detection necessitate a careful assessment of the unintended consequences of these processes. This development raises critical concerns regarding the authenticity and trustworthiness of automated transcriptions in an era where AI-manipulated audio becomes increasingly commonplace.
1. Applying additional audio compression after watermark removal often leads to a noticeable decline in the effectiveness of voice detection. This happens because the compression process might remove crucial frequency components that are essential for accurate speech recognition, leading to an overall decrease in the clarity of the audio's interpretation.
2. The watermark removal and subsequent compression process can sometimes create small but noticeable timing inconsistencies in the audio waveform, introducing what researchers call "temporal artifacts." These slight shifts in the audio can impact transcription accuracy because it makes it harder for transcription algorithms to correctly understand the timing and structure of spoken language.
3. The altered audio profiles that can result from compression can also lead to a phenomenon called frequency masking. In this case, important phonetic details that are essential for transcription become obscured by residual noise leftover from the watermark removal and compression processes. Studies have indicated that this masking can affect as much as 15% of the phonetic sounds in speech, which can have a significant impact on the overall performance of automatic speech recognition (ASR) systems.
4. The difficulties with frequency masking described earlier don't just affect overall transcription accuracy; they can also make speaker identification more challenging for ASR systems. These systems rely on recognizing specific voice characteristics to identify individual speakers, but alterations to the audio caused by compression can make it harder or even impossible for these systems to correctly identify or separate different speakers in audio recordings.
5. There's a strong connection between the noise artifacts that are introduced during secondary audio compression and the rate of transcription errors in various ASR systems. This noise can effectively mask or obscure many of the subtle vocal cues that ASR systems rely on to perform accurate transcriptions, which can increase error rates by as much as 30%.
6. Advanced transcription systems are being developed that are designed not only to transcribe spoken words but also to analyze things like prosody (the rhythm and intonation of speech) to try and understand the emotional context of a speaker. The altered sound frequencies introduced by compression can create issues with recognizing tonal changes in speech and may lead to misinterpretations of emotions, which can impact sentiment analysis during transcription.
7. The synthetic sounds that are often created during the watermark removal process have been observed to influence the behavior of machine learning models that are used in speech recognition. These models often compute similarities between different speech samples, and the presence of these artificial sounds can result in unusual model behavior and increase the likelihood of transcription errors.
8. Because the audio manipulation that happens during watermark removal is sometimes dynamic, it can also lead to unexpected variations in the way that speech is articulated. These inconsistent patterns can create challenges for ASR systems since they aren't typically equipped to handle these kinds of rapid or unpredictable shifts in audio characteristics, which decreases overall transcription reliability.
9. It's been noted that ASR algorithms exhibit an unexpected sensitivity to even small audio encoding and decoding errors, and this sensitivity is amplified when audio is processed post-watermark removal. When distortions are introduced, the rate of transcription errors can increase by up to 25%, suggesting a need for robust audio processing methods.
10. The techniques used to alter the audio can subtly change linguistic patterns in the speech, creating challenges for ASR models that were trained on normal, unaltered speech. Since these models have difficulty adapting to the new patterns, there may be a need to rethink the kinds of training data that are needed to improve speech recognition in the future.
How AI Video Watermark Removal in 2024 Affects Content Transcription Quality - Machine Learning Models Struggle with Modified Video Frame Rates
AI-powered video watermark removal, while improving visual quality, is creating a new hurdle for machine learning models, particularly when it comes to handling modified video frame rates. With the ever-increasing volume of video content online—think of the sheer amount of videos uploaded to platforms like YouTube every minute—the pressure to accurately process and understand video data is immense. These models often struggle when presented with videos that have undergone frame rate alterations, which can be a result of the watermark removal process. This is because these changes can introduce complexities that interfere with how the models analyze the visual information and interpret the corresponding audio.
Efforts to improve the accuracy of video frame rates through AI techniques like video frame interpolation continue, but inconsistencies and discrepancies persist. This indicates the need for greater standardization and improvements in the algorithms used for such processing. Furthermore, this interaction between video manipulation and transcription accuracy is a point of growing concern, raising questions about the overall reliability of automated content transcription in the future as AI technology continues to advance. The challenge for the future is to better understand the ramifications of these advanced techniques and to adapt AI models to be more resilient to the changes they introduce.
Machine learning models are often trained on video data with a consistent frame rate. When these videos undergo post-processing, such as watermark removal, which can change the frame rate, these models might struggle to correctly interpret the temporal aspects of the video. This can result in decreased performance in tasks like transcribing the accompanying audio, as the models might misinterpret the relationship between the audio and the visual elements.
Modifying the frame rate can also introduce unforeseen audio synchronization issues. The audio might not align perfectly with the visual content after these changes, creating challenges for transcription systems. Many transcription systems rely on a tight connection between the visual and audio cues, making a mismatch problematic for accurate results.
According to studies in signal processing, alterations in frame rates can create artifacts like jitter. Jitter can make it more difficult for transcription systems to analyze the important audio patterns used for accurate transcription, especially those models that need continuous audio input to function correctly.
Models trained to expect a specific frame rate can experience performance declines when the frame rate changes during watermark removal. These models become more sensitive to deviations from the original frame rate, which can negatively impact their ability to extract information from the audio.
Frame rate inconsistencies can disrupt the natural flow of speech. Transcription systems might miss parts of the speech as a result, generating incomplete or broken outputs. This is especially challenging in environments where the audio is already noisy.
Adjusting frame rates during watermark removal can also change the granularity of the audio data. Higher frame rates often provide more fine-grained audio information. If the frame rate is lowered, vital phonetic details that help with accurate transcription might be lost.
Machine learning models utilizing recurrent neural networks (RNNs) seem to be particularly vulnerable to changes in frame rate. RNNs learn patterns from sequences of data, so adjusting the frame rate alters these patterns and affects the model's ability to accurately identify speech.
The dynamic changes in audio caused by a modified frame rate can also introduce unexpected shifts in volume and intonation. These shifts can hinder accurate transcription as they make it more difficult for transcription systems to isolate individual speech sounds.
The performance of machine learning models used in real-time transcription takes a hit when frame rates are changed. They are usually configured for a specific range of frame rates, and deviations from that range can lead to a notable rise in transcription errors.
The combination of altered frame rates and audio characteristics complicates machine learning tasks. Engineers are working to enhance audio preprocessing methods to better handle these nuanced changes caused by watermark removal, especially for tasks like automated transcription. This area needs careful attention to maintain accuracy.
How AI Video Watermark Removal in 2024 Affects Content Transcription Quality - Audio Channel Separation Becomes Less Reliable After Digital Manipulation
Digital alterations, particularly those associated with AI-powered watermark removal in videos, can negatively impact the ability to reliably separate audio channels. While these AI tools can improve video appearance, they can also introduce subtle audio distortions and artifacts that make it harder to recreate the original sound quality. This creates complications for transcription because it throws off the delicate balance needed for accurate interpretation. Transcription accuracy can suffer as a result, leading to a greater possibility of errors and misinterpretations. Moreover, techniques meant to improve channel separation can sometimes result in a more cluttered and noisy audio landscape, making it a more challenging task for speech recognition software to perform effectively. Moving forward, as the reliance on video content grows, a renewed emphasis on pristine and consistent audio is crucial. This will require a rethinking of current procedures for how content is transcribed to address these challenges caused by advanced AI techniques.
Audio channel separation, a crucial aspect of audio processing, can become less reliable after a video undergoes digital manipulation, especially watermark removal. This is becoming more apparent as AI-powered tools for removing video watermarks become increasingly sophisticated. While these tools can successfully eliminate watermarks, they often introduce subtle changes to the audio, potentially affecting the accuracy of audio transcription.
One of the most noticeable effects is the alteration of audio frequencies. These shifts, sometimes as high as 20%, can change how we perceive speech and cause problems for algorithms that rely on recognizing specific frequencies to distinguish different sounds. Even minor changes can lead to errors in transcription.
Furthermore, the audio compression that often accompanies watermark removal can remove important parts of the audio spectrum. Studies have shown that up to 15% of the distinct sounds used in speech can be lost through this compression. This degradation makes it harder for transcription systems to correctly understand the nuances of speech, as they lose crucial clues for accurately deciphering what is being said.
Watermark removal techniques can also introduce subtle timing discrepancies in the audio. These inconsistencies, known as temporal artifacts, disrupt the natural rhythm and flow of speech, hindering the performance of transcription algorithms that are designed to work with consistent timing.
It's intriguing that even small audio modifications resulting from these processes can lead to significantly higher transcription error rates. It's been observed that a 5% change in the waveform can increase transcription errors by up to 30%. This highlights a surprising sensitivity of transcription systems to these subtle audio alterations.
Another issue is the presence of background noise artifacts introduced during watermark removal. These artifacts can cause masking effects, making it harder for transcription systems to differentiate between the target speech and unwanted background sounds. These distortions are often barely noticeable to humans, which underscores how much more sensitive transcription systems are to audio anomalies than we are.
Transcription systems also have trouble with the extended periods of silence created by some watermark removal methods, like phase inversion. These pauses can interrupt the flow of speech recognition and cause difficulties in detecting the start and end of phrases, potentially leading to errors in the final transcription.
Additionally, dynamic adjustments of audio profiles during watermark removal can result in inconsistencies in speech patterns. These unpredictable fluctuations create problems for transcription algorithms, which often rely on relatively stable and consistent audio characteristics to function correctly.
The altered audio can also make it difficult for systems to distinguish between different speakers, since the distinctive qualities of each voice can be impacted. This affects the overall performance and ability to separate out distinct speakers during a conversation.
The ability of AI-powered tools to generate synthetic sounds that resemble human speech can also cause problems. When algorithms encounter these noises, they might be confused about what they are hearing, potentially resulting in inaccurate transcriptions.
Finally, the constant evolution of watermark removal techniques might force us to reassess how we train transcription algorithms. It's possible that future models will need to be trained on a wider range of altered audio environments to adapt to the changes that watermark removal introduces. This potential shift could be a significant change in how transcription systems are developed in the future.
These challenges highlight the need for ongoing research and development in audio processing and transcription to improve the robustness of these systems in the face of increasingly sophisticated audio manipulations caused by AI tools. As AI-powered tools become more integrated into content creation and editing, understanding the impact on the audio and its transcription will become more crucial.
Upscale any video of any resolution to 4K with AI. (Get started for free)
More Posts from ai-videoupscale.com: