7 Key Stages of AI Video Quality Enhancement From Basic to Professional Results
7 Key Stages of AI Video Quality Enhancement From Basic to Professional Results - Raw Video Analysis Through Machine Learning Pattern Recognition
The application of machine learning, especially deep learning, to raw video analysis is fundamentally changing how we engage with visual content. AI systems are now capable of learning intricate patterns from vast amounts of video data, becoming increasingly adept at identifying, tracking, and interpreting the events and objects within a video. This process often involves supervised learning techniques, a method that requires substantial labeled datasets for effective training. However, creating and managing these datasets can be quite challenging. Further complicating the process is the analysis of long-form video content, demanding advanced algorithms and techniques to effectively manage and analyze the sheer volume of video data created daily across various fields. These challenges notwithstanding, the integration of machine learning is pushing the boundaries of video analysis, allowing us to refine the quality and accessibility of visual information in new and exciting ways. The potential for enhanced video understanding and usability through machine learning is substantial and continues to evolve.
Machine learning, particularly using convolutional neural networks (CNNs), offers a new approach to understanding raw video data. These networks can be trained to spot irregularities in videos that might escape human notice, proving valuable for applications such as surveillance where identifying accidents or uncommon behaviors is key. However, unlike traditional frame-by-frame methods, machine learning can analyze entire video sequences, better grasping the overall context and movement patterns for more powerful predictions.
This ability to understand the flow of events relies significantly on good-quality input. Low-resolution video or poor lighting can severely hinder performance. Fortunately, some recent innovations in unsupervised learning are starting to lessen the need for huge amounts of labeled video data. This could accelerate deployment in real-world situations.
A fascinating aspect is 'temporal information extraction', where the models focus on changes visible within video frames over time. This allows for more accurate action recognition and event forecasting, with potential for fields such as sports analysis and security. Combining audio and visual cues from a video has also been shown to greatly improve accuracy in event recognition because sounds often give a richer understanding of the situation.
Moreover, analyzing the high-dimensional data within a video allows us to uncover patterns hidden at first glance. This detailed analysis could yield insights into behaviors or trends across various settings. The recent developments in hardware acceleration, especially GPUs, make real-time processing feasible, paving the way for applications like live security and autonomous vehicles.
It's crucial to carefully select the relevant features that we input to these machine learning models. If we don't do this effectively, the resulting analysis can be deceptive. So, pre-processing and feature engineering play vital roles. Lastly, the growing trend of 'transfer learning' has opened doors for us to adapt models trained on vast video datasets for specialized tasks. This process significantly reduces the effort and resources required in developing useful solutions for video analysis, making it accessible to more researchers and developers.
7 Key Stages of AI Video Quality Enhancement From Basic to Professional Results - Frame Rate Optimization And Motion Smoothing
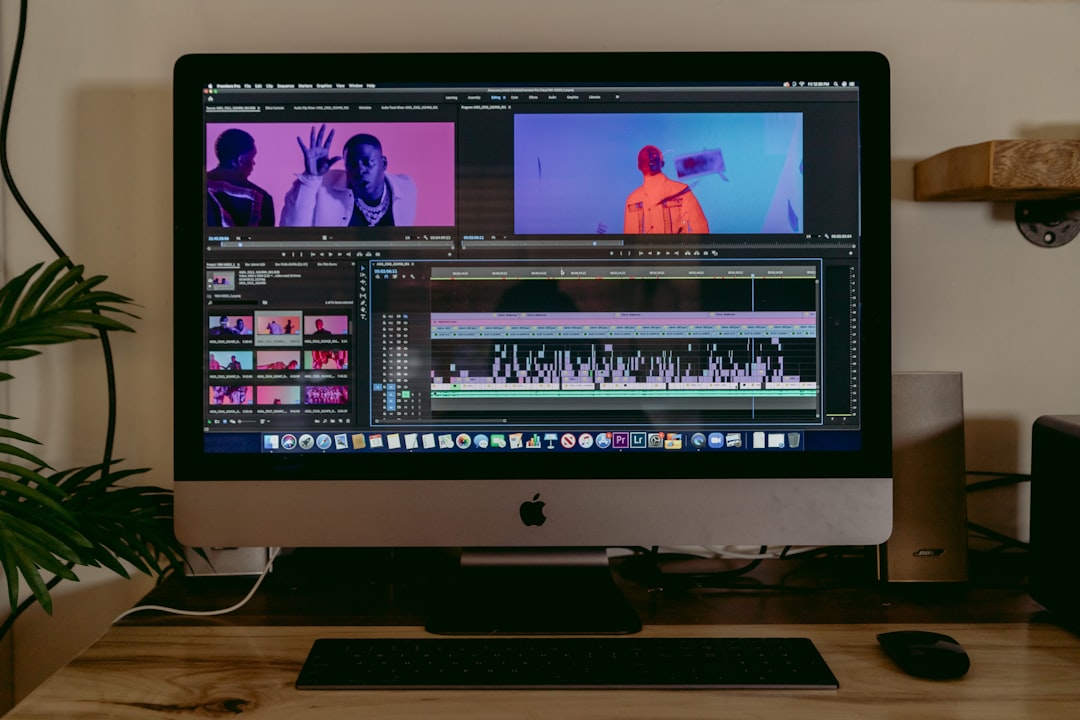
Frame rate optimization and motion smoothing are crucial aspects of enhancing video quality using AI. These techniques significantly improve the viewing experience by addressing issues like jerky or choppy movements. AI algorithms, specifically frame interpolation, can generate new frames between existing ones, making the video appear smoother and more fluid.
For example, techniques like RIFE Video Interpolation can successfully increase frame rates, even from lower resolution video, pushing past 30 frames per second. This ability to smoothly increase frame rates has a clear impact on video quality. There are various software programs designed to optimize frame rates, offering features like converting a 24 fps video to a smoother 60 fps for a more cinematic feel. This is accomplished by generating in-between frames to make the transition between frames appear smoother.
While some software aims for a smoother viewing experience, other AI tools focus on expanding the capabilities of video. Some can significantly increase frame rates, making super slow-motion playback smooth and realistic. This demonstrates the transformative potential of AI in video manipulation, pushing the boundaries of what's possible in achieving fluidity and a better viewing experience.
AI-powered video enhancement is increasingly focusing on optimizing frame rates and smoothing motion, leading to more fluid and engaging viewing experiences. Increasing the number of frames per second (fps) can significantly reduce motion blur, especially noticeable in fast-paced action sequences. This can lead to a more satisfying and clear viewing experience. However, enhancing frame rate isn't without its drawbacks.
While motion smoothing can greatly improve the perceived smoothness of videos, it can also introduce unintended aesthetic changes, a phenomenon sometimes called the "soap opera effect". This over-smooth aesthetic can clash with the artistic choices made by filmmakers, especially when dealing with cinematic works originally shot at 24 fps for a particular visual style. This leads to an interesting question: is improving fluidity worth compromising the creative intentions of the original work?
Different content types naturally have varying optimal frame rates. While cinema often favors the lower frame rates (24 fps) to maintain a distinct visual language, sports broadcasts commonly use 60 fps to capture rapid movements clearly. This highlights the need to tailor the optimization process based on the specific nature of the video.
The human eye's perception of motion varies with different frame rates. What might be acceptable for standard video (say 30 fps) may require a significantly higher rate (60 fps or even higher) for action-heavy sequences to avoid jerky motion. This understanding of human perception is vital in optimizing for various types of content.
Techniques like optical flow estimation are often utilized to create additional frames between existing ones in motion smoothing. While this allows for interpolating motion, the quality and accuracy of the interpolation can vary significantly depending on the complexity of the scenes involved. It seems like there is still room for improvement in these algorithms.
It's also important to consider the 'temporal cadence' of the video – how the balance between motion and stillness is perceived over time. This is essential for maintaining narrative flow and viewer attention. For instance, abrupt changes in frame rate within a video might impact storytelling and could result in an unnatural viewing experience.
One surprising issue is that very high frame rates can sometimes introduce their own motion artifacts, like judder or stutter, particularly when dealing with video content that wasn't originally produced at those high rates. This indicates the complexity involved in this enhancement process and highlights the need for advanced correction techniques during the upscaling process.
Furthermore, achieving consistency in frame rate optimization across diverse displays remains a hurdle. An enhanced video that looks pristine on a high-end screen might induce visual fatigue or discomfort on a lower-quality display. This necessitates careful calibration for diverse viewing conditions.
Looking ahead, new display technologies, like variable refresh rate (VRR), show promise in allowing for dynamic frame rate adjustments. This could provide the best of both worlds: dynamically tailoring frame rates to specific content, viewer preferences, and device capabilities for a tailored and comfortable viewing experience while retaining the fidelity of the original motion. This emerging technology could solve some of the current challenges in AI-based video quality enhancement.
7 Key Stages of AI Video Quality Enhancement From Basic to Professional Results - Resolution Upscaling From SD To 4K Using Neural Networks
AI-powered resolution upscaling transforms standard definition (SD) video into 4K by employing neural networks. These networks essentially learn the patterns within the lower-resolution video and then predict the missing high-resolution pixel data, effectively filling in the gaps to create a sharper, more detailed image. This process involves experimenting with different neural network architectures to optimize the upscaling process, aiming for the highest possible quality while minimizing artifacts. We're seeing a rise in tools and platforms that allow users to leverage these capabilities for their own video enhancement needs, offering options to control and fine-tune the upscaling process.
However, the pursuit of ultra-high resolutions through AI raises concerns about the potential alterations to the original video content. The neural network-generated details might not perfectly reflect the original source, potentially leading to a loss of the artistic intent embedded within older film or video recordings. While the results of AI upscaling can be impressive, mimicking a near-cinematic quality for older footage, we must consider the balance between enhancing visual clarity and preserving the unique characteristics of the original content. It's a delicate dance between improving accessibility and maintaining the integrity of the original creative work. Finding that sweet spot is an ongoing challenge in this rapidly evolving field.
Neural networks are becoming increasingly sophisticated in their ability to upscale video resolution, particularly from standard definition (SD) to 4K. This process involves the network learning to predict the missing high-quality pixel information based on the lower resolution input. However, training these networks demands substantial resources, often requiring many hours of video data to establish reliable patterns. This highlights the computational intensity associated with achieving high-quality results.
Beyond simply increasing the number of pixels, these neural networks can also enhance the overall perceived sharpness and detail. Viewers can often discern details that were previously obscured in the original SD source, leading to a more immersive viewing experience.
Interestingly, the architectural design of the neural network itself plays a significant role in the quality of the output. For example, generative adversarial networks (GANs) have shown promise in achieving superior upscaling compared to more traditional convolutional neural networks (CNNs). Understanding these architectural nuances is crucial for optimizing upscaling quality.
One of the more compelling challenges in AI-powered upscaling is the ability to effectively remove artifacts. These artifacts, often resulting from compression or noise in the original SD video, can detract from the enhanced resolution. Neural networks are increasingly adept at detecting and correcting these imperfections, significantly improving the visual fidelity of the upscaled content beyond a simple resolution increase.
A recent trend in resolution upscaling involves integrating temporal information, leveraging the flow of events across multiple frames to enhance the quality of individual frames. This is based on the idea that understanding the context of motion can help accurately predict details within a particular frame, leading to a more accurate and detailed output.
It's also worth noting that the viewing environment itself significantly influences the perceived quality of an upscaled video. Studies indicate that viewers are more sensitive to the differences in upscaled content when viewing on larger screens and closer distances. This implies that both content creators and display technologies need to consider these factors when creating and displaying upscaled videos.
However, it's not all smooth sailing. Even neural networks can introduce their own artifacts during the upscaling process, meaning there's a careful balancing act between enhancing resolution and preserving the integrity of the source material. This underscores the complexities involved in this technology and the importance of calibration and testing for optimal outcomes.
The nature of the content also influences the quality of the upscaling process. Animated videos, with their unique style and often less complex textures, may upscale differently than live-action footage, which inherently has a wider range of details and textures.
Furthermore, handling fast-paced or high-motion content remains a challenge for many of the existing upscaling algorithms. Fast movements can result in blurring or distortion of details, suggesting that refinements are still needed to effectively handle these scenarios.
Fortunately, recent advances in hardware capabilities, especially GPUs specifically designed for deep learning, have dramatically sped up the upscaling process. We're now moving toward the possibility of real-time upscaling, offering the potential to enhance content on the fly rather than relying on lengthy offline processing. This opens up many opportunities for more dynamic and interactive video experiences.
7 Key Stages of AI Video Quality Enhancement From Basic to Professional Results - Color Correction And Dynamic Range Enhancement
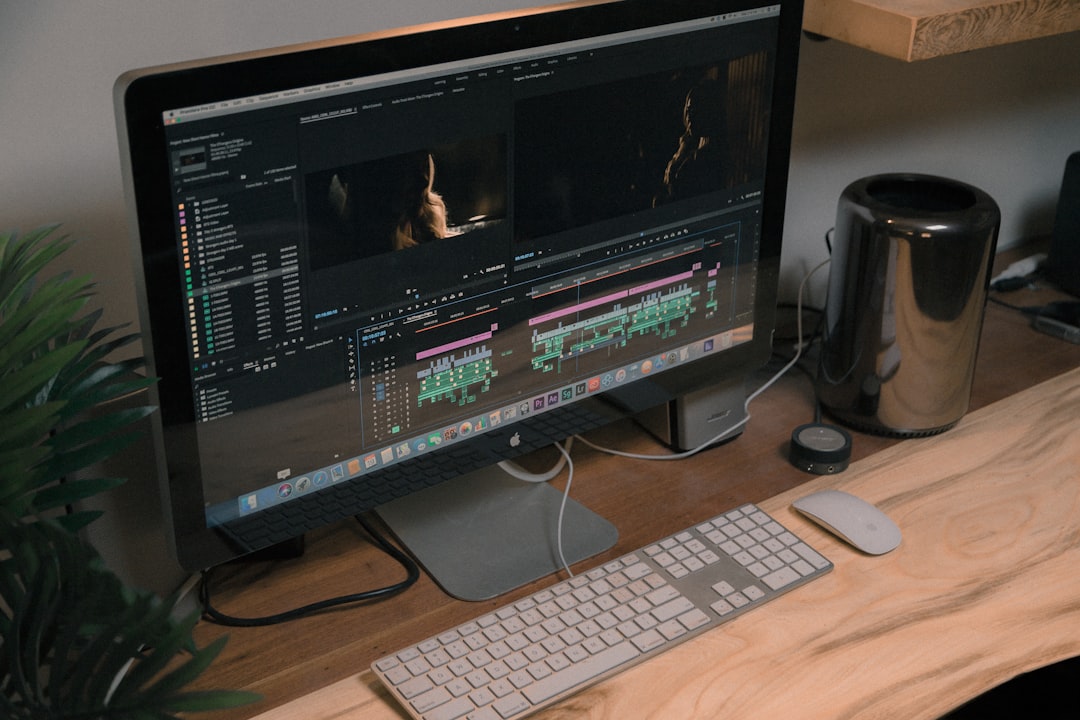
AI video enhancement increasingly relies on color correction and dynamic range enhancement to deliver truly high-quality results. Modern AI tools now offer sophisticated automated color adjustments, simplifying the process for everyone from novice users to professional editors. This automation brings professional-level color grading within reach of a broader audience, enriching the aesthetic appeal and detail of videos.
By enhancing color accuracy and expanding the dynamic range, these tools are able to reveal details often hidden in shadows or washed out in bright areas. This results in a more nuanced and visually rich viewing experience. While the accessibility and ease-of-use of AI-driven color tools are undeniably beneficial, some may worry about the potential loss of a uniquely artistic vision. The traditional approach of a skilled colorist carefully refining a video’s color palette might be replaced by algorithmic choices, potentially leading to a homogenization of video aesthetics. This is a growing point of debate in the video production community.
The evolution of AI in video enhancement continues to push boundaries. The tools allow for a wider range of creative choices, which is exciting. But, as with any technology that streamlines complex processes, it's important to weigh the benefits of automation against the loss of more personalized and artistic techniques. The future of video enhancement lies in a careful balance between the potential of AI-powered tools and the importance of preserving an artist's unique vision.
Color Correction And Dynamic Range Enhancement
The human eye's ability to perceive a wide range of light and color, spanning about 20 stops of dynamic range, greatly exceeds the capabilities of most camera sensors, which typically top out around 14 stops. This disparity presents a challenge for video content creators, as it requires careful color correction to create visuals that mimic the way we naturally perceive the world.
Furthermore, the choice of color space can significantly influence how colors are presented. Different color spaces, such as sRGB, Adobe RGB, and DCI-P3, have varying ranges of colors, with DCI-P3 offering a wider gamut, often utilized for cinematic productions to capture richer color palettes. During color correction, it's crucial to consider these color space differences to ensure the final video accurately reflects the content's intended look.
Look-Up Tables (LUTs) play a critical role in color correction. They essentially map one color space to another, enabling quick transformations of the video's overall appearance. LUTs can dramatically alter the mood of a scene, essentially shifting a bright sunny day to a somber, film noir style without needing reshoots. This malleability showcases the power of color correction beyond just fixing technical errors.
However, color perception is not always straightforward. Simultaneous contrast, a phenomenon where surrounding colors influence how a specific color is perceived, creates complexities for color correction. This means even if the color itself remains the same, its perceived appearance can change based on the context of the surrounding scene, emphasizing the intricate and nuanced nature of the process.
The bit depth of a video influences the level of detail in color transitions. Higher bit depth, such as in 10-bit video (with 1024 shades per channel), allows for smoother gradations compared to 8-bit video (with 256 shades). This is particularly critical when enhancing dynamic range, as it ensures smooth and accurate transitions between various shades.
Color correction is often used for artistic effect, extending beyond simple color adjustments. Color grading can subtly influence the mood of a scene or highlight narrative aspects, functioning as a powerful tool for visual storytelling.
Gamma correction, a process that adjusts the luminance of a video, significantly impacts how shadows and highlights are displayed. Adjusting the gamma curve can reveal more detail in both very dark and very bright areas of an image, contributing to a considerable improvement in the overall quality of the enhanced video.
Unfortunately, the wide array of display technologies brings another level of complexity. Different monitors and TVs have different methods for displaying color and dynamic range, like HDR versus non-HDR. A video that looks perfect on one display might appear very different on another, creating a need for more adaptive color correction methods.
While dynamic range enhancement aims to capture the entire range of light in a scene, there are inherent technical limits. In scenes with extreme contrast, clipping can occur, where details are lost in very bright or dark areas. Careful planning during filming and thoughtful color correction in post-production can minimize this impact.
AI tools are increasingly involved in color correction, capable of analyzing frame-by-frame color attributes and applying corrections automatically. However, they frequently lack the artistic subtlety and nuance a skilled colorist brings to the process. This illustrates the ongoing tension between the potential of automation and the value of human creativity in color correction, suggesting both human and AI will continue to have vital roles in this process.
In essence, color correction and dynamic range enhancement are not simple tasks but complex processes that involve careful consideration of various factors. This field constantly evolves as new technologies emerge and push the boundaries of what's possible in video enhancement. The goal is always to find the best balance between preserving the original intent of the content while enhancing it to meet the diverse requirements of viewers and display technologies.
7 Key Stages of AI Video Quality Enhancement From Basic to Professional Results - Noise Reduction And Artifact Removal Through Deep Learning
Deep learning has brought about a notable shift in how we approach noise reduction and artifact removal in video enhancement. AI systems, particularly those utilizing deep neural networks, are capable of identifying and addressing noise and artifacts – issues stemming from compression or other sources that can significantly hinder visual quality. The strength of these methods lies in their versatility, effectively tackling noise and artifacts across various video formats and types.
However, this technological leap isn't without its complexities. While the algorithms can enhance signal quality impressively, it's crucial to be aware that the fine-tuning process can, at times, introduce new artifacts. This suggests the need for careful calibration and ongoing refinement in these techniques to ensure the best results. The goal is to achieve a delicate balance between enhancing video quality and avoiding the unintended introduction of new issues.
The promise of significantly improved video quality through deep learning is undeniable, bringing us closer to a future where noise and artifacts are a thing of the past. But it's important to acknowledge that achieving this ideal is a continuous process, a conversation among experts who strive to continually refine these technologies and better understand their limitations. It is a field that presents both remarkable potential and intricate challenges as the pursuit of pristine video continues.
Deep learning has emerged as a powerful tool for tackling noise reduction and artifact removal in video, mirroring its success in other signal processing fields like EEG analysis and medical imaging. While deep learning offers promising solutions, it also presents intriguing challenges and nuances we need to understand.
One surprising aspect is the sheer diversity of noise types in video. Simple techniques struggle with this variability, as noise can manifest in different forms (e.g., Gaussian, salt-and-pepper, shot noise), demanding more sophisticated approaches. Successfully tackling these varying noise profiles requires deep learning models trained on diverse noise types to be truly effective.
Furthermore, the sequential nature of video introduces the vital need for temporal consistency in noise reduction. If each frame is treated in isolation, the resulting video can exhibit flickering artifacts, defeating the purpose of enhancement. Algorithms need to consider the flow of the video over time to maintain a smooth and natural visual experience.
Generative models, especially GANs, have shown promise in this area. They can learn to produce a clean version of the video, helping remove noise and artifacts more effectively by creating a counterpart that looks more like a noise-free version of the original. This generative approach allows for a more holistic and intuitive understanding of the video's underlying structure.
Excitingly, advancements in hardware and algorithm optimization have made real-time noise reduction increasingly feasible, enabling live video enhancements like those needed in surveillance systems or live sports broadcasts. This shift toward real-time capabilities offers tremendous opportunities for interactive and dynamic video experiences.
A current trend involves combining noise reduction and deblurring in a single framework. This joint approach seems to outperform treating each issue separately since artifacts from one can complicate the other. This integrated approach to tackling multiple issues simultaneously showcases the ingenuity of deep learning methods.
However, reliably distinguishing meaningful features from background noise can be tough for neural networks. Creating training datasets that showcase a wide range of noise conditions and video contexts is critical for effectively training these models.
Adaptability is another key factor. Some advanced techniques leverage adaptive filtering, adjusting the intensity of noise reduction based on the video's local characteristics. This allows them to preserve sharp details while effectively cleaning up noisy areas, ensuring the final output maintains a balance between clarity and smoothing.
Interestingly, standard quality metrics, such as PSNR, may not accurately capture the perceptual quality changes introduced by noise reduction algorithms. Newer perceptual quality metrics that better align with how we experience visual differences are becoming more relevant for effectively evaluating model performance.
A subtle yet significant problem is the potential for noise reduction techniques to introduce their own visual artifacts, such as unusual shadows or halos around objects. This underscores the delicate balance needed in algorithm design, showcasing the importance of careful fine-tuning to avoid inadvertently diminishing video quality.
Finally, the effectiveness of these deep learning models is strongly linked to the variety and richness of the training datasets. Models trained solely on very noisy content might struggle with cleaner video, highlighting the ongoing need for datasets that encompass a wide range of noise levels and video styles to improve model robustness and applicability across different content.
The field of deep learning for video noise reduction and artifact removal is ripe with exciting possibilities. While substantial progress has been made, many nuanced and challenging aspects remain, requiring ongoing research and development. Ultimately, the goal is to leverage deep learning's power to enhance video quality while maintaining visual integrity and preserving the intended artistic expressions within the video content.
7 Key Stages of AI Video Quality Enhancement From Basic to Professional Results - Sharpness Enhancement And Detail Recovery
Within the broader scope of AI video enhancement, sharpening and restoring details are pivotal steps in elevating the visual experience. AI algorithms are adept at deciphering the intricate patterns within video data, particularly low-resolution or compressed content, and then reconstructing the missing details. This process goes beyond simple sharpening, aiming to enhance the video with a richer texture and sharper visual elements, ultimately creating a more engaging viewing experience. However, achieving this goal isn't without its pitfalls. In the pursuit of perfect sharpness, some AI tools can introduce subtle but noticeable artifacts, creating a tension between desired enhancement and the integrity of the original video. It's a continual effort for developers to refine these tools to find the right balance between adding clarity and preserving the artistic style or intent of the source material. The ongoing refinement of these techniques showcases a constant evolution in video enhancement technology, where the search for optimum visual quality remains a key pursuit.
Sharpness enhancement and detail recovery are fascinating aspects of AI video enhancement, revealing both the potential and limitations of current technologies. Our perception of sharpness isn't just about pixel resolution; our brains also process contrast and edges, making it a complex challenge for AI. It turns out that manipulating the frequencies in video, particularly the higher frequencies that correspond to fine details and edges, is a common way to improve sharpness. This manipulation is often done through techniques like the Fourier Transform, which can alter the spatial frequency information of the video.
However, a common pitfall is overdoing the sharpness enhancement. Too much sharpening can lead to the appearance of artificial halos around edges, which can diminish image quality. This emphasizes the need for a delicate balance – a constant struggle within the development of these algorithms. Traditional image processing methods like Laplacian and unsharp masking are still relevant in AI approaches, where they can be used to selectively sharpen specific areas, yielding more nuanced results compared to a blanket application of sharpness across the entire image.
Beyond single frames, advanced AI techniques are starting to utilize temporal information across multiple video frames to improve detail. By recognizing and analyzing patterns of change across frames, it's possible to infer and even reconstruct details that are hard to discern within individual frames. This temporal approach can lead to a noticeable improvement in the clarity and sharpness of videos, especially during motion. Interestingly, deep learning models can now dynamically focus their enhancement efforts on specific parts of a frame, for instance, prioritizing faces or important objects within a scene, thereby enhancing details in the most impactful areas.
Of course, the viewing conditions heavily influence how we experience sharpness enhancement. Large screens and close viewing distances can highlight any limitations in the sharpening methods used. This requires AI video enhancements to be tailored to the expected viewing environment. We're also seeing more sophisticated approaches that incorporate edge-preserving methods, which can help maintain natural textures within an image while still sharpening it. These techniques, like joint bilateral filtering, are helping to prevent an artificial appearance, preserving authenticity.
The specific hardware used for video playback is also a factor in sharpness perception. High-resolution displays can potentially amplify any sharpening artifacts, demanding extremely fine-tuned adjustments. Maintaining temporal coherence—that is, applying sharpness enhancements consistently across video frames—is crucial for preventing distracting flicker or jumps in image quality. Without a consistent application of sharpness, we can end up with a disjointed viewing experience that hinders the audience's immersion in the content.
In conclusion, sharpness enhancement and detail recovery are becoming more sophisticated with the advancements in AI. However, it is a field with nuances and challenges that require ongoing research and refinement. The goal remains to improve visual quality while preserving the integrity of the original video content and respecting any artistic intentions behind its creation.
7 Key Stages of AI Video Quality Enhancement From Basic to Professional Results - Final Quality Control And Export Parameters
The final stage of AI video enhancement, encompassing Final Quality Control and Export Parameters, is crucial for ensuring the finished product meets desired quality standards before sharing or distributing. This involves thoroughly examining and fine-tuning various video quality metrics. These include elements like the final resolution, the frame rate, and the level of compression applied. These details directly affect the viewing experience, and getting them right is vital to keep viewers engaged.
Beyond the technical adjustments, this stage often relies on perceptual metrics. These tools help gauge how the human eye and brain perceive the video quality after enhancement. The goal is to strike a balance between delivering a high-quality output and creating files that are not overly large and difficult to handle.
Export settings are equally important. The way you choose to export the video can have a big impact on its quality when viewed on different devices and platforms. One thing to keep in mind is compression, as it can sometimes cause visible issues like artifacts, undermining the positive effects of the AI enhancement process. These issues are more noticeable if you haven't optimized compression levels appropriately.
As the world of video continues to change, having a firm grasp of quality control and export parameters becomes ever more crucial for delivering high-quality video that captivates your audience. Understanding and mastering these final steps is how you ensure that all the AI video enhancement work delivers the intended results and viewer experience.
Final Quality Control and Export Parameters hold a crucial position in the AI video enhancement pipeline, often overlooked but critical for achieving professional results. It's where the fruits of earlier processing stages are refined, optimized, and prepared for distribution. However, this final stage is filled with its own set of intricacies and unexpected hurdles that can impact the overall quality if not carefully addressed.
One area of continuing research is the choice of quality assessment metrics. Traditional metrics like PSNR, while simple to compute, often fail to capture the nuances of how we perceive visual quality. New methods, such as SSIM, strive to more accurately align with our visual system by factoring in the structure of images rather than just focusing on simple pixel differences. This more perceptual-focused approach is becoming increasingly important.
Maintaining perfect sync between audio and video is another concern, especially as videos get more complex with multiple audio layers and edits. Even a tiny delay can significantly disrupt the viewer's experience and can be very difficult to fix in later stages. It's a testament to how subtle aspects of video can drastically alter how we perceive it.
The selection of video codecs also impacts the final product's quality and file size. More modern codecs like H.265 can offer better compression rates, allowing for higher-quality videos at smaller sizes. However, they require more computational resources for encoding and decoding, a trade-off we need to consider for the specific applications. Similarly, decisions about using a variable bit rate (VBR) instead of a constant bit rate (CBR) affect how bits are allocated throughout the video. VBR allows for optimization by dedicating more bits to demanding scenes, leading to smaller file sizes without significantly affecting the viewing experience.
Color space conversion during export can be a significant source of errors. Transforming video between color spaces, like from Rec. 709 to Rec. 2020 for high dynamic range (HDR) displays, can lead to unexpected color shifts if done improperly. This underscores the importance of meticulous attention to detail to preserve the initial artistic intent during these transformations. And choosing the appropriate export format (like MP4, MOV, or ProRes) directly affects compatibility, playback smoothness, and quality. While MP4 is commonly supported, formats like ProRes might offer higher quality through reduced compression, albeit with significantly larger file sizes.
Modern AI techniques are also increasingly being incorporated into noise management during the export process. This helps reduce artifacts that might have been introduced during editing or from inherent compression issues, resulting in cleaner, smoother-looking video. Tools that allow for real-time quality control during export are also beginning to appear. These can identify problematic areas like bitrate issues or color distortions on the fly, allowing for on-the-spot corrections to save time and resources in post-production.
The shift toward cloud-based rendering solutions is notable. Cloud-based export allows us to offload the computational demands to powerful servers, accelerating the export process and also improving scalability for larger collaborative projects. This cloud-based approach is becoming increasingly significant as video production becomes more complex and collaborative. Similarly, the rise in hardware acceleration through GPUs is revolutionizing how we export high-resolution videos. These advancements allow us to produce the increasingly popular 4K and beyond resolutions much more quickly and efficiently.
These advancements in final quality control and export are a testament to the continued refinement and improvement of the AI video enhancement pipeline. It's a delicate balancing act to achieve the highest quality output while balancing considerations like file size, compatibility, and artistic intent. The future seems to be moving towards more integrated workflows with more powerful hardware and cloud-based approaches which might greatly streamline this phase.
More Posts from ai-videoupscale.com: