Using AI to Enhance Urban Skateboarding Footage A Technical Analysis of Sights on Scene
Using AI to Enhance Urban Skateboarding Footage A Technical Analysis of Sights on Scene - Deep Learning Networks Transform Urban Rail Slides Into Training Data
Deep learning techniques are transforming how we utilize footage of urban rail slides. By employing sophisticated algorithms, primarily Graph Convolutional Networks, the intricate movements and interactions of skateboarders during these maneuvers can be captured and processed. This opens up the possibility of creating valuable datasets that reflect the dynamic nature of skateboarders navigating urban rail infrastructure.
Such data can be leveraged in a variety of ways. For instance, it can improve the accuracy of urban transit system classification and help address challenges arising from population growth and the associated increase in transportation needs. While the primary benefit is likely to be in enhancing urban transit, the potential for other applications related to urban planning and infrastructure design is a promising future possibility. The application of these AI methods to urban skateboarding footage potentially contributes towards a more efficient and sustainable urban transit system by leveraging a seemingly niche activity for larger urban challenges. However, there are some concerns and questions around the potential overreliance on AI-driven solutions without considering other perspectives or potential biases in the data used to train these systems.
Urban rail slides, where skateboarders use the infrastructure's edges as a canvas for their movements, are a goldmine of high-definition footage. These videos are packed with unique textures and perspectives that are often missing in more traditional training datasets. It's intriguing how deep learning networks can be trained to recognize intricate patterns of movement and skateboarding style—details that might be too subtle for us to easily discern.
This rich data from urban skating environments, when used to train AI models, can provide a broader perspective. It allows the inclusion of environmental nuances like lighting and weather conditions, factors which often significantly impact video quality and the outcomes of various tricks. Furthermore, we can leverage the wealth of data from these urban skating videos to refine deep learning models. They can then become more adept at distinguishing the subtle differences in how tricks are performed, which could be particularly valuable for skateboarding coaching and skill evaluation.
However, transforming this raw skating footage into a structured, usable dataset for AI is no simple task. It necessitates sophisticated algorithms for parsing and annotating the videos. This underlines the need for robust computational infrastructure to handle the complex processing involved.
Interestingly, the sheer volume and depth of data derived from these urban rail environments present a fascinating possibility: simulating different skating scenarios. This opens up avenues to provide valuable insights for skateboarders of all levels, from recreational riders to competitive athletes. We can also imagine that AI systems, fueled by diverse urban skating data, could create detailed profiles for optimal skating techniques tailored to different rail types and locations.
But there are hurdles to overcome. Urban skating footage is often noisy, with pedestrians or vehicles moving within the frame. These moving elements can complicate the learning process for our algorithms. Consequently, careful filtering and refinement of the training data are crucial to ensure accuracy and reliability.
The continuous evolution of skating trends and urban design means urban rail slides create a dataset that is perpetually dynamic. AI models trained on this data can adapt over time, improving their ability to understand and respond to the changing landscape of urban skateboarding. It is exciting to consider that the blending of deep learning and urban skating analysis can not only assist in technique evaluation, but could also lead to augmented reality experiences that could revolutionize how people interact with and enjoy skateboarding.
Using AI to Enhance Urban Skateboarding Footage A Technical Analysis of Sights on Scene - Motion Capture Analysis From Olympic Park Security Footage
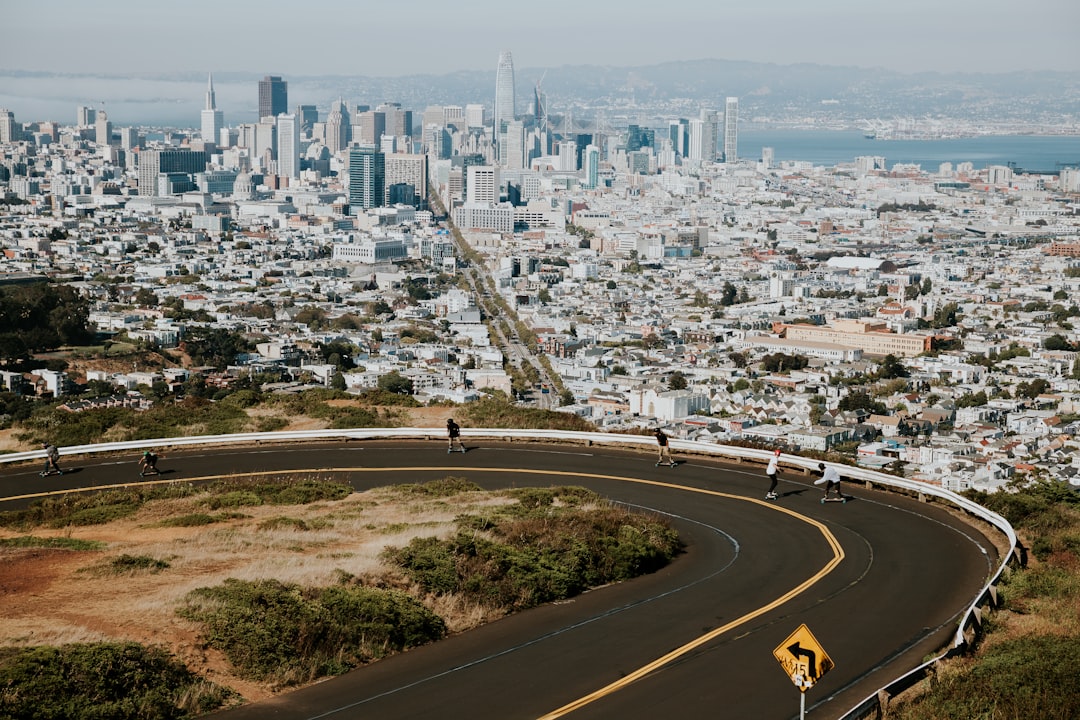
Analyzing Olympic Park security footage through motion capture presents a novel way to blend security and sports analytics. This approach leverages AI to provide real-time insights into athletic movement, giving athletes a detailed understanding of their performance. Traditionally, motion capture was a time-consuming process, requiring specialized equipment and manual analysis. The shift towards AI-powered, markerless systems makes this type of analysis more accessible to a wider range of athletes, from Olympic hopefuls to recreational enthusiasts. While this development helps optimize training and performance, it also raises the broader potential of motion capture technology in sports science and beyond. However, it's important to acknowledge that with the increased reliance on AI systems, we must critically examine the potential for biases in the data they use and understand their inherent limitations. The technology is still developing, and there are open questions about the ethical implications of using it.
Motion capture analysis, particularly when applied to Olympic Park security footage, presents a unique opportunity to understand skateboarding in urban environments. High-frame-rate cameras, often capturing up to 120 frames per second, are crucial for capturing the rapid movements inherent in many skateboarding tricks, allowing for a detailed look at previously hard-to-analyze actions.
More advanced motion capture methods often incorporate markers and sensors placed on skaters. This approach can provide a more detailed view of not only trick execution but also a skater's biomechanics, potentially yielding insights into ways to minimize the risk of injury. However, the urban environment introduces various challenges, like varying light conditions and unique angles, which can heavily affect the quality of the data gathered. By studying footage captured across different weather patterns and times of day, we can better understand how these environmental variables affect both skating performance and visibility.
AI models trained on this data have shown a remarkable ability to adapt over time. They learn from patterns in motion, including things like the wear and tear on different surfaces. This ability allows for the possibility of predicting optimal skating techniques for different terrains, an intriguing concept that could have broad implications. Beyond simply documenting movement, motion capture can identify anomalies, such as falls or failed tricks, offering valuable data for assessing risk and developing proactive safety measures.
Furthermore, it's possible to connect this data with urban design. By studying how skateboarders interact with existing structures like railings and curbs, we can gain a deeper understanding of how urban spaces can be designed to enhance both safety and encourage skateboarding. A comparative analysis of motion capture data from different locations can provide insights into how local culture and infrastructure shape specific skating styles.
However, employing surveillance footage in motion capture analysis raises important ethical questions regarding privacy and consent, particularly in public spaces. It's crucial to be mindful of how data is collected and used, ensuring that individual rights are respected. The detailed biomechanical data from motion capture can significantly influence the development of training protocols for skateboarders. Coaches can use these insights to create tailored training programs that address the specific strengths and weaknesses of individual skaters.
And while still in its developmental phase, it's exciting to consider a future where real-time motion capture analysis is possible during skateboarding sessions. Imagine a system that provides immediate feedback, enabling skaters to adjust their technique for optimal performance as they skate. Such a capability could significantly change how skateboarding coaching is done and how skaters approach practice.
Using AI to Enhance Urban Skateboarding Footage A Technical Analysis of Sights on Scene - Street Spot Recognition Through Computer Vision at LA Arts District
"Street Spot Recognition Through Computer Vision at LA Arts District" explores how AI and computer vision can revolutionize our understanding of skateboarding locations within urban settings. By using sophisticated algorithms to analyze street view imagery, we can extract key features and gain a more comprehensive understanding of the urban environment and its influence on skating culture. The LA Arts District, with its distinctive architecture and vibrant street art, provides a compelling case study. It showcases how the physical characteristics of a neighborhood influence both skateboarding styles and how skateboarders interact with the space. Through this approach, researchers can unveil hidden patterns in urban environments that might be missed by traditional methods, ultimately leading to potentially better urban design principles and a more nuanced understanding of how skateboarding and cities interact. It is crucial, however, to carefully consider the possibility of biases within AI-driven analyses and the inherent risk of oversimplifying the multifaceted nature of skateboarding within urban culture.
Urban visual intelligence, a concept that combines AI and visual data analysis, is gaining traction in urban studies, particularly with the rise of street view imagery. Over 6,800 research papers in the past year alone highlight the growing interest in applying computer vision (CV) to urban environments. CV techniques, a subset of AI, are particularly adept at extracting information from images, and recent advancements have made it possible to automatically glean insights from street view data, making it a more valuable resource for urban research.
This AI-driven approach to urban analysis offers a more detailed and nuanced understanding of urban spaces than traditional manual methods, which can be quite time-consuming and subjective. Street view imagery (SVI), readily available through services like Google Street View, has become a popular tool in this field. Researchers are using CV to explore how the physical environment influences human perception, which can be quite helpful for urban planning and geographic information science.
AI-driven approaches offer a more objective way to analyze how people perceive and interact with urban spaces, particularly relevant in contexts like skateboarding. Applying AI to skateboarding footage, especially in areas like the LA Arts District, can yield valuable insights into the relationship between urban spaces and skateboarding culture. While this technology holds great promise, the integration of video data from these complex urban environments can be challenging. The abundant visual "noise" from pedestrians and vehicles can make it difficult for algorithms to focus on skater movements alone.
However, the potential benefits are significant. AI algorithms can not only track skateboarders' movements but also recognize the unique characteristics of the urban structures they utilize. This opens up intriguing possibilities for future urban design that's tailored for skateboarding, making cities more enjoyable and functional for this vibrant community. Researchers have also found that lighting conditions and shadows are critical factors in video quality, which in turn impacts the ability of AI models to extract useful information.
Furthermore, motion capture systems, when combined with CV, offer an even deeper understanding of skaters' biomechanics. They can analyze not only the execution of tricks but also how skaters interact with the textures and surfaces they ride on, potentially revealing insights into preferred materials for skateboarding. AI systems trained on this data show remarkable adaptability as urban environments change over time, demonstrating the dynamic relationship between skating culture and the built environment.
The LA Arts District, with its diverse textures and color palettes, presents a varied landscape for video analysis. The quality of footage depends heavily on the time of day and weather conditions, making it crucial for algorithms to be trained on diverse datasets to ensure they can generalize well to these variations. Street spot recognition algorithms aren't just useful for sorting through massive video datasets but can also enhance real-time performance analytics, offering skaters immediate feedback on their skills and techniques.
The ability of AI to recognize and classify urban skateboarding environments, including features like ledges, rails, and gaps, opens up new ways to document and catalogue street spots. This could prove invaluable for skateboarders searching for optimal locations for practice and innovation. As this technology evolves, researchers are exploring the possibilities of real-time performance analysis, providing instantaneous feedback to skaters about their performance—something previously unheard of in the sport. These advancements in AI and computer vision have the potential to change how skateboarding is practiced and enjoyed in urban environments.
Using AI to Enhance Urban Skateboarding Footage A Technical Analysis of Sights on Scene - Machine Learning Models Track Board Flip Mechanics in Real Time
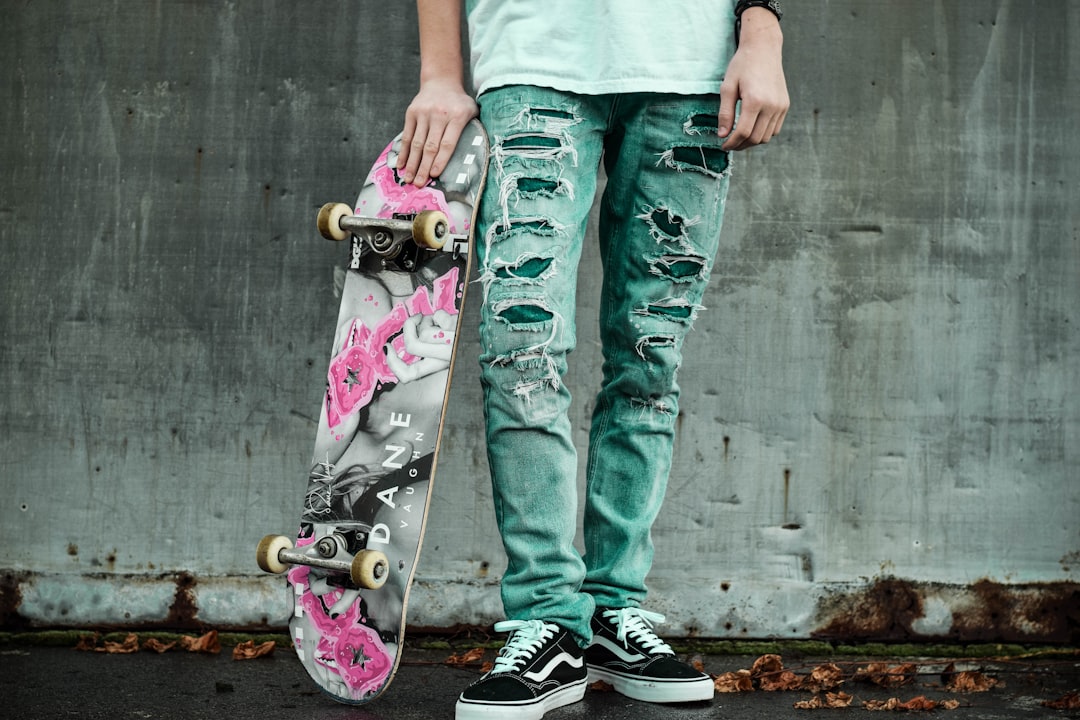
Machine learning models are now being used to analyze the mechanics of skateboard flips in real time. This provides a new way to study the details of skateboarding movements. These models use sophisticated algorithms to dissect the patterns of motion during flips, allowing for instant feedback on how well a flip is performed and the skateboarder's technique. The use of high-speed video captures the fine details of a skater's actions. These models can also consider how factors like lighting or weather can impact performance. However, the busy, complex nature of urban environments can lead to some unwanted noise or clutter in the data, and this requires careful handling to make sure the results are precise. In the end, real-time tracking of this type doesn't just make performance assessment better, but also has the potential to change how coaches work with skaters. This could allow for a much better understanding of flipping techniques and the connection between skateboarding and the built environment in cities.
Machine learning models are being explored to track the mechanics of board flips in real-time. They rely on advanced physics-based simulations to predict the trajectory of a skateboard during tricks, offering potential for immediate feedback during training. This could be a real game changer for learning new skills more effectively. These models are capable of dissecting factors like the speed of rotation, the flick angle, and the impact of landing. This level of detail can offer insights not just into skating techniques but also into the design of equipment to improve performance and safety.
High-speed cameras, capable of processing tens of thousands of frames per second, are vital for capturing the lightning-fast nature of skateboard tricks. This data fuels machine learning systems, allowing them to learn and predict movements with more accuracy, even in complex, fast-paced environments. It's really fascinating to see how they learn to interpret the nuances of trick execution.
One interesting development is the ability of these real-time tracking models to adapt to the distinctive features of different urban surfaces. This means they can develop a better understanding of how textures and materials impact board control and stability during flips. This has direct implications for skateboarding biomechanics studies.
Furthermore, these AI systems, because they can analyze flip mechanics in various urban settings, can learn to understand the broader context. They can grasp how local architecture influences skateboarding practices across different cultures and geographical locations. This capability to see things from a more 'contextual' perspective is pretty impressive.
Convolutional neural networks (CNNs) are also being employed to differentiate the subtle differences in movements during tricks. This leads to a much more refined skill assessment than might be possible with human observation alone. It's likely this kind of detailed evaluation could lead to more tailored coaching approaches.
Adding environmental variables like wind speed and humidity into model training can improve their predictive abilities concerning flip mechanics, as these things can have a significant impact on a skater's performance. It shows how the models are starting to capture more of the real-world complexity of skateboarding.
While the potential of these machine learning models is very exciting, we should also consider the computational requirements. These models can be quite demanding, needing substantial GPU resources, which could limit accessibility for average skaters and coaches.
The precision of these real-time mechanics tracking systems also holds promise for injury prevention strategies. By analyzing patterns that typically precede common skateboarding injuries, skaters could potentially adjust their techniques to mitigate risk.
Finally, envisioning the integration of data from these machine learning models into skateboarding apps holds potential for greater community engagement. It could lead to fun ways for skaters to challenge each other based on quantified skill metrics.
It will be very interesting to see how these tools evolve and what impact they have on skateboarding culture.
Using AI to Enhance Urban Skateboarding Footage A Technical Analysis of Sights on Scene - Urban Architecture Detection Creates Digital Skate Maps
AI is increasingly being used to analyze urban architecture, leading to the development of specialized digital skate maps. These maps are created by employing advanced AI techniques like deep learning and data fusion to extract detailed information about the built environment, particularly features relevant to skateboarding. This information enables a more precise understanding of skateable spaces within cities, which can be incredibly helpful for urban planning initiatives with a focus on skateboarding. The development of these maps relies on comprehensive datasets that capture changes in the urban landscape over time. This time-lapse view of changes is valuable for developing more efficient and sustainable urban planning practices that account for the needs of the skateboarding community.
However, it's important to acknowledge the challenges that come with using AI in this way. Urban environments and skateboarding styles are constantly evolving, which raises questions about the long-term accuracy and adaptability of these AI models. It's crucial that these systems are developed in a way that considers the complexities and dynamism of urban skateboarding culture. The challenge remains to ensure that AI-driven solutions provide accurate information while also respecting the nuances of skateboarding's social and cultural impact within cities.
The intersection of urban architecture detection and skateboarding is fostering the development of detailed digital skate maps. This approach utilizes deep learning and data fusion techniques to extract information from the built environment, focusing on skateboarding-relevant features like rail heights and surface conditions. Essentially, these AI models are learning to "read" the urban landscape through the lens of a skateboarder, creating a rich dataset that goes beyond simple visuals.
Currently, the largest available street-level scene change detection dataset is being leveraged, facilitating comprehensive urban mapping for skateboarding. These models can track changes in skate spots over time, reflecting the dynamic nature of cities and allowing maps to be continuously updated. This dynamic approach could prove especially helpful for skaters who want to find the best and most recently updated locations.
This research underscores how urban design and skateboarding culture are intertwined. By analyzing how skateboarders interact with urban elements, insights can be gained on how to incorporate skate-friendly design elements into future urban planning. This has implications for fostering skateboarding communities and creating more skate-friendly public spaces.
Furthermore, these systems can utilize algorithmic spot recognition to provide personalized recommendations for skaters based on individual skill levels, past activity, and environmental conditions. The algorithms are essentially learning to understand what makes a "good" skate spot, factoring in preferences and context. This personalization aspect holds potential for a more engaging and enriching skateboarding experience.
Interestingly, researchers are starting to examine the audio component of skate footage as well, with sound analysis revealing information about surface conditions and urban soundscapes. The 'feel' of a skate spot isn't just visual—it's also auditory. Understanding the relationship between the acoustic environment and skateboarding performance could provide additional insights into how skaters choose their spots and execute tricks.
These AI-driven approaches are also helping to better understand how different urban features influence trick execution. This understanding can be utilized to improve skateboarding coaching and skill development, potentially allowing skaters to refine their techniques based on specific urban elements. However, as cameras are increasingly utilized in public spaces to collect skate footage, it's important to consider the privacy implications. Balancing the benefits of data collection with ethical considerations for individual privacy is crucial.
These skate maps not only catalog skate locations but also allow for a feedback loop. Performance data from skaters can be used to refine the AI models, creating a cycle of improvement. The feedback allows algorithms to get better at recognizing and interpreting different skateboarding movements, which leads to a more refined analysis of skater skills and styles.
This intersection of AI and skateboarding has the potential to influence future skateboarding competitions as well. The maps and data gathered could provide a more nuanced and objective way of evaluating skater performance, going beyond subjective human observation and offering quantifiable metrics relevant to specific urban elements. This type of data-driven approach could lead to a more sophisticated scoring system in competitive skateboarding.
In conclusion, using AI to analyze urban environments through the lens of skateboarding is showing exciting potential for better urban planning, improved skater performance, and a deeper understanding of this urban subculture. While the technology is still developing, the advancements in urban architecture detection and data-driven mapping methods promise to be a valuable tool for both skateboarders and urban designers in the years to come.
Using AI to Enhance Urban Skateboarding Footage A Technical Analysis of Sights on Scene - Camera Movement Stabilization for Fast Following Shots
Capturing the dynamic energy of urban skateboarding often involves fast-paced, following shots that can be prone to camera shake and instability. This can significantly detract from the viewer's experience and obscure the intricacies of the skateboarding maneuvers. Recently, AI-powered stabilization methods have emerged as a powerful tool to overcome these challenges. These methods leverage complex algorithms that analyze each frame of video, identifying and compensating for unwanted camera movement. The result is a smoother, more polished presentation of the footage, minimizing the effects of jitters, shakes, and motion blur. This is particularly important in urban environments, where the interplay of skaters with complex surroundings creates visually complex scenes that can be difficult to film without some degree of camera movement. The benefit is not just a more professional-looking aesthetic, but also a better experience for the viewer, who can more clearly see the details of the skateboarding actions. Interestingly, even those who film skateboarding with less sophisticated equipment can benefit from the advancements in AI-powered stabilization, potentially leveling the playing field in the production of high-quality skateboarding footage. While these improvements offer great potential, the ongoing development of these AI stabilization techniques raises important questions about the computational demands involved and potential biases that may arise from relying on a particular dataset to train the AI.
Capturing fast-paced skateboarding tricks presents unique challenges for camera stabilization. Skateboarding often involves high-G forces, with skaters experiencing accelerations exceeding 3 Gs during rapid maneuvers. This extreme motion demands very accurate stabilization to prevent distortion and ensure every detail is captured, a hurdle for many videographers.
Many modern cameras use gyroscopic stabilization, which involves sensors detecting and countering rotational motion in real-time. While helpful in fast-paced urban environments, it can struggle to keep up with extremely quick changes. However, camera hardware limitations sometimes get in the way of effective stabilization. For instance, the 'jello effect', caused by rolling shutters in many camera systems, warps vertical lines during quick movements and can be difficult to fix. This common problem is especially noticeable in urban skateboarding videos.
Active stabilization systems use algorithms to predict and correct for motion in real time, while passive methods depend on mechanical components like gimbals. When dealing with rapid movement, active stabilization can offer a smoother picture as it adapts more quickly. The frame rate chosen for the video recording is also important for stabilization. Higher frame rates (like 120fps or more) reduce motion blur, but they place a heavier load on the stabilization system.
Another challenge for videographers is the depth of field when trying to shoot in fast following shots. Wide apertures lead to a narrow depth of field, making it harder to stabilize when a skater moves in and out of focus. Maintaining a clear picture of the subject becomes tricky for filmmakers under those conditions.
Furthermore, the environment itself can have a huge impact on both the skater and the camera. Uneven surfaces like cracks in the pavement or ramp edges create instability, requiring advanced stabilization technology that can anticipate such changes to ensure smooth footage.
More recently, AI has become part of camera stabilization systems. These algorithms analyze motion in real time and can differentiate between the desired motion of a skateboard trick and unwanted vibrations caused by the surroundings, offering much better stabilization than traditional methods.
While the technology is improving, the increased reliance on powerful processors and sensor hardware can lead to bulkier and heavier equipment, which can be less practical in the midst of an energetic skate session. Also, elements like weather and lighting add even more complexity to stabilization. Changes in the weather, like rain or sun, can cause problems with slippery surfaces, or camera settings like exposure and focus, which stabilization needs to consider to maintain a decent video.
It's clear that even with improvements in stabilization technology, maintaining consistent and accurate footage of fast-paced skateboarding in urban settings remains a significant technical challenge.
More Posts from ai-videoupscale.com: