Upscale any video of any resolution to 4K with AI. (Get started for free)
Adobe Media Encoder A Deep Dive into Video Compression Techniques for 2024
Adobe Media Encoder A Deep Dive into Video Compression Techniques for 2024 - H264 vs H265 Encoding Efficiency in 2024
In the video landscape of 2024, the choice between H264 and H265 encoding remains a crucial consideration, especially as higher-resolution streaming becomes more prevalent. H265, or High-Efficiency Video Coding, provides a compelling case for its use, excelling in compression. It can achieve similar visual quality to H264 using roughly half the bandwidth, a significant benefit for demanding formats. Yet, this enhanced efficiency comes at a cost: the more complex encoding process takes much longer, sometimes 10 to 20 times the time compared to H264. This can hinder workflows that prioritize quick turnaround.
While H265 boasts clear advantages, H264 retains its dominance in situations where broad device compatibility is vital and speedy processing is needed. Its widespread adoption across the industry, particularly in live streaming, gives it a lasting appeal. Adobe Media Encoder, reflecting this ongoing discussion, incorporates hardware acceleration for both codecs. This feature attempts to find a middle ground, maximizing the benefits of each format while alleviating some of their inherent limitations in terms of speed. Ultimately, the optimal choice between the two codecs depends on the specific demands of the project, balancing the need for high compression with real-world factors like time constraints and device reach.
H.264, also known as AVC, is a widely used codec that provides a reasonable balance between video quality and file size. It's a dependable standard for various applications like streaming and Blu-ray discs, demonstrating its versatility. However, H.265, or HEVC, has emerged as a more efficient compression codec. It offers a remarkable reduction in bitrate while sustaining high video quality, particularly valuable for high-resolution streaming formats like 4K and beyond. This efficiency translates to roughly half the bandwidth needed compared to H.264 for comparable quality during both encoding and transmission.
H.265’s enhanced adaptability stems from its flexible block sizes, reaching up to 64x64 pixels, which contrasts with H.264's fixed 4x4 block sizes. This flexibility allows H.265 to adapt more effectively to different video types, ultimately boosting its compression efficiency. The improved motion estimation in H.265 leads to more precise video transitions in the compressed format, contributing to its overall efficiency.
Despite its significant improvements, H.265 comes with a computational cost. Its encoding process is substantially more complex than H.264, resulting in encoding times that can be 10 to 20 times longer. This longer processing time can be a bottleneck in some workflows. Interestingly, even with these advantages, H.264 still holds a substantial market share, accounting for over 90% of use cases within the video industry. Its strong foothold is largely due to its compatibility across a wide range of devices, especially crucial for live streaming platforms.
Furthermore, H.264's simplicity and broad device support often make it the preferred codec when compatibility is paramount. For example, users or producers with less powerful hardware might experience issues with H.265 playback, making H.264 a safer choice. Conversely, nearly all modern cameras capturing 4K or higher video resolutions opt for H.265, emphasizing its storage efficiency for demanding formats.
Modern software like Adobe Media Encoder now supports hardware-accelerated encoding for both H.264 and H.265. This feature improves performance and reduces export times for both, making them more practical options within increasingly complex video pipelines. However, the inherent trade-offs between encoding speed and efficiency continue to guide the choices of developers, producers and engineers in their projects.
Adobe Media Encoder A Deep Dive into Video Compression Techniques for 2024 - Adaptive Bitrate Streaming Advancements
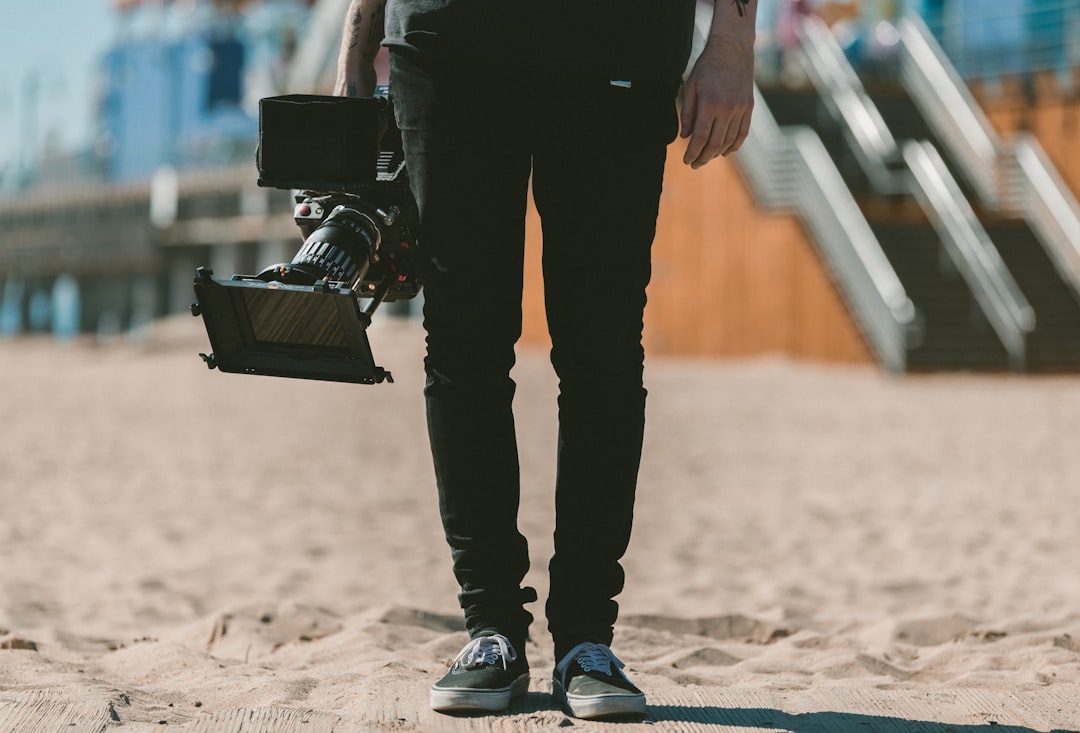
Adaptive Bitrate Streaming (ABR) is fundamentally changing how we experience video content. The core of ABR is its ability to adapt to the viewer's network conditions, dynamically adjusting video quality to maintain smooth playback even when bandwidth is limited. This is crucial in our increasingly diverse online environments, where users might be streaming from a high-speed connection or facing more challenging network conditions.
The evolution of ABR has seen a shift towards more sophisticated techniques for optimizing video quality based on real-time network analysis and user experience (QoE) data. Encoding techniques and algorithms are becoming increasingly complex to deliver the best possible viewing experience across various devices and network scenarios.
However, this progress comes with its own set of challenges. Designing an effective ABR system requires careful management of bitrate ladders—which are pre-defined sets of video resolutions and corresponding bitrates— and the entire transcoding process. Creating efficient bitrate ladders that cater to a wide range of viewing environments is a task requiring significant optimization. The encoding systems, like those found within Adobe Media Encoder, must also manage the increased computational load that these intricate ABR processes introduce. Ultimately, the success of ABR depends on a delicate balance between delivering a superb streaming experience and maintaining efficient resource utilization within the encoding pipeline.
Adaptive Bitrate Streaming (ABR) has become fundamental to the streaming experience we've come to expect, particularly for higher-resolution content where network conditions can be unpredictable. It works by intelligently adjusting the quality of the video stream based on a viewer's connection speed and bandwidth, minimizing buffering and ensuring a smooth playback experience. The core of ABR involves real-time bandwidth analysis, allowing it to dynamically adapt the video resolution to optimize for both quality and available network capacity.
This dynamic approach has proven to be highly effective, often leading to substantial bandwidth savings across the board. However, the effectiveness of ABR depends on a number of factors including having an optimized set of resolutions and bitrates (bitrate ladder), as well as using proper transcoding techniques that prepare videos in advance to cater to a range of quality levels. This pre-processing typically involves slicing and encoding videos into multiple versions of various quality levels to be easily selected during playback.
While ABR algorithms have evolved significantly, primarily driven by a growing need for more sophisticated network and viewer behavior prediction, they are ultimately limited by the underlying video encoding standards. For example, although H.265 provides more efficient compression, the added complexity can slow down encoding time, presenting hurdles for workflows that need to process content quickly.
Moreover, ABR relies on efficient implementation of streaming platforms and media players. The effectiveness of the system hinges on how seamlessly the chosen player can handle the multiple bitrate streams that ABR serves up. Compatibility can sometimes be a challenge, especially with older or less common devices or platforms.
The ongoing development of ABR technology, including incorporating machine learning and protocols like CMAF to reduce latency, suggests a continuous effort to refine the user experience. However, the transition towards a fully adaptive ecosystem remains ongoing. It's worth noting that the industry is still transitioning to new technologies while a large number of existing systems, especially in broadcast environments, are yet to be fully transitioned to a streaming model. Though ABR has proven beneficial to viewers by significantly improving streaming quality, continued work in optimizing ABR systems and the underlying encoding standards is crucial for the future of streaming. The quest to find the best possible balance between quality, efficiency, and viewer experience is an evolving research topic.
Adobe Media Encoder A Deep Dive into Video Compression Techniques for 2024 - AI-Powered Compression Algorithms
AI-powered compression algorithms are emerging as a potentially transformative force in video compression, particularly within the context of 2024's burgeoning video landscape. These algorithms, built upon the foundations of deep learning and neural networks, hold the promise of significantly improving compression ratios by identifying subtle patterns and redundancies that traditional methods often overlook. This ability to recognize complex data structures within videos allows for more efficient compression, particularly valuable for the increasing demand for high-resolution content.
Several companies, like Deep Render, are at the forefront of AI-driven compression technology, experimenting with unique solutions that aim to address some of the enduring problems inherent in the current video compression space. These new solutions involve techniques like refining the process of how video data is broken down (coding unit splitting) and incorporating content-aware models which intelligently adapt the compression method to the nuances of the video being encoded.
However, the adoption of these AI-powered methods isn't without its limitations. Many AI-based compression approaches require considerable computational resources, which can hinder their practical implementation in time-sensitive production workflows where speed is critical. Striking a balance between the superior compression achieved by AI and the need for manageable processing times will be key to broader adoption within the industry.
With the relentless expansion of internet video traffic, the necessity for innovative compression methods has become undeniably critical. The field of AI shows promise in optimizing how video data is handled, particularly for the ever-growing needs of streaming services. Nevertheless, successfully integrating AI-powered solutions into video compression pipelines requires a careful assessment of the trade-offs between the advantages in efficiency and the potential impact on processing speeds. Ultimately, the ongoing research into AI-powered compression will be crucial to finding the optimal balance between quality, speed, and efficiency in the evolving world of video compression.
AI-driven video compression is an emerging field that leverages neural networks to tackle long-standing challenges in the digital space. Deep Render, for instance, is developing a purely AI-based compression technology, suggesting a potential shift in the compression landscape. Companies like Apple, with their acquisition of WaveOne, are also showing increasing interest in these AI-powered solutions. This signifies that the industry acknowledges the potential of AI to optimize video compression further.
One of the core advantages of AI-based techniques is their ability to detect and remove subtle data redundancies that traditional algorithms often overlook, ultimately achieving higher compression ratios. Researchers at MediaKind, for example, have published work applying machine learning to enhance HEVC coding unit splitting, indicating a growing trend towards applying AI within existing compression standards. While some studies show that deep learning methods can match or even surpass the quality of human-designed algorithms, they also raise questions about the computational resources needed for their operation.
A new approach has emerged where AI models are used to augment conventional codecs. By integrating a small super-resolution model that adapts to specific content, video compression for internet delivery can be improved. Researchers at Microsoft have proposed a new framework that moves away from predictive coding in favor of conditional coding. This offers increased flexibility when encoding, potentially leading to improved efficiency.
The explosion of internet-based video traffic has been a major catalyst for advancements in video compression, pushing the boundaries of what's achievable. Integrating AI into this domain has the potential to reshape the video landscape, especially for streaming platforms. Deep neural networks can be integrated into different stages of the video processing pipeline, including pre-processing, coding, and post-processing.
However, the integration of AI into established workflows can be challenging. There's a learning curve involved in integrating AI models and training them with suitable data, which is especially true when integrating into existing codecs. The training process itself requires considerable computational power, which could lead to bottlenecks or latency issues in real-time applications. Still, it's intriguing that these AI-powered approaches can potentially be applied to improve the performance of almost any codec, effectively retrofitting existing systems with more efficient compression techniques.
AI-driven adaptive bitrate streaming is particularly promising. Algorithms that can predict user behavior and network conditions in real-time have the potential to revolutionize how streaming content is delivered. While AI-driven compression has shown tremendous promise, it is still an active area of research. Finding the ideal balance between compression quality, computational resources, and seamless integration into existing infrastructure will continue to shape how video content is encoded and shared in the future.
Adobe Media Encoder A Deep Dive into Video Compression Techniques for 2024 - Multi-Pass Encoding Strategies
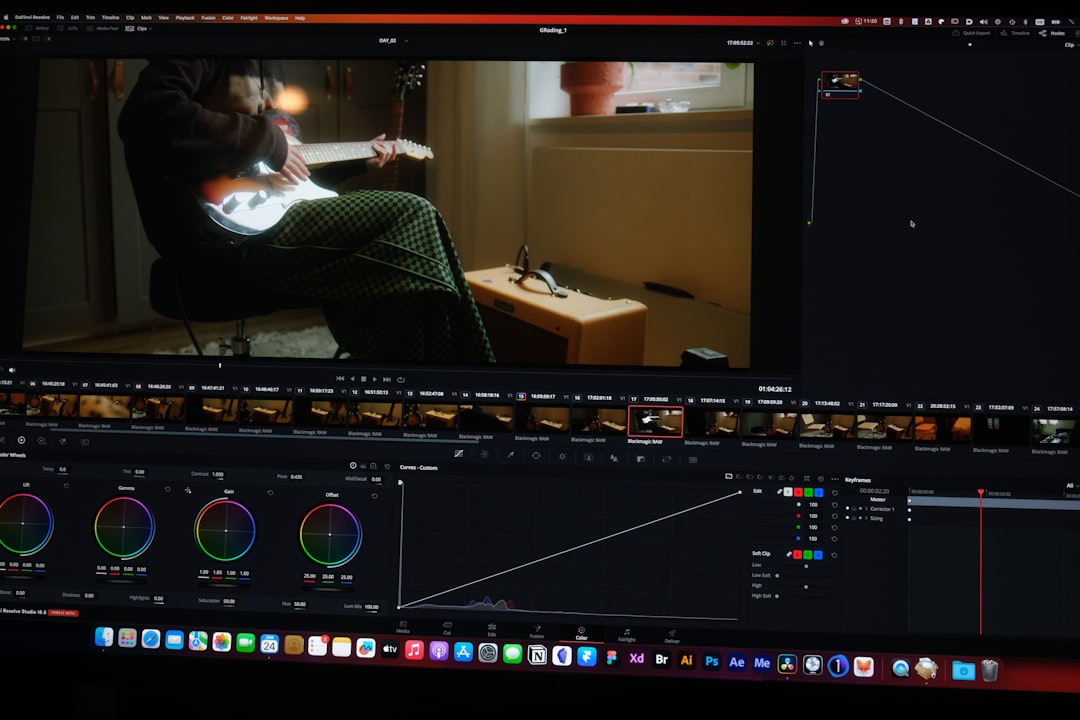
Within Adobe Media Encoder, multi-pass encoding offers a refined approach to striking a balance between video quality and file size. This technique involves multiple passes through the video data, allowing for more intricate adjustments to bitrate and other encoding settings. This leads to noticeable improvements in overall visual fidelity, which is especially useful for demanding video formats such as 4K and UHD. When optimizing for quality and efficiency is paramount, multi-pass encoding can prove valuable.
However, the inherent trade-off is the increased time required for processing. The longer encoding times might not align with every production schedule, particularly when speed is a priority. As video production demands continue to evolve, it's crucial for professionals to consider the advantages and limitations of multi-pass encoding, making informed decisions based on their project's specific needs. Striking the right balance between video quality and efficient encoding time is key for delivering high-quality video content in 2024.
Multi-pass encoding strategies offer a way to refine the video compression process by performing multiple passes over the source material. This approach, compared to single-pass methods, allows the encoder to gain a more comprehensive understanding of the video's content during the initial passes, enabling it to make smarter decisions about how to optimize the encoding in subsequent passes. The outcome is usually higher quality video at lower bitrates, a major benefit for demanding applications like broadcast and streaming.
A particularly interesting outcome of using multi-pass is the ability to achieve better results with Variable Bitrate (VBR) encoding. VBR intelligently adapts the bitrate allocated to the video based on the complexity of the scene in question. This means that action sequences or intricate detail receive more bits, while simpler scenes use fewer bits. The result is a more efficient use of bandwidth, leading to a generally better viewing experience.
Of course, there are downsides. The process of multi-pass encoding is significantly slower than its single-pass counterpart. Depending on the chosen configuration, encoding times can stretch out to several times longer. This added time can be a serious issue for workflows that need to turn things around quickly, leading to a necessary trade-off between the quality gains and the processing time required to obtain them.
The exact way that a multi-pass strategy is applied can be adjusted to fit different goals. For instance, a streaming project might focus on keeping the encoded video under a particular size to minimize buffering or delays. On the other hand, someone archiving video might emphasize preserving the finest details possible even if the encoded file is larger. This adaptability can be helpful, allowing producers to tune the encoding to meet the needs of their project.
In general, multi-pass encoding tends to produce consistent quality across a wide variety of devices. This is good news for viewers, as they can expect a clear, high-quality viewing experience regardless of whether they are using a high-end device or a less powerful phone.
The most common form is what's called two-pass encoding, but there are advanced techniques like adaptive multi-pass encoding that take things even further. In adaptive methods, encoding parameters are continuously adjusted based on the playback characteristics observed during streaming. This leads to an optimized experience that extends beyond the static approach of a regular multi-pass strategy.
Multi-pass encoding is more intricate than its single-pass equivalent. This complexity, however, provides a much greater level of control over the encoding process. Engineers can tweak various parameters to fine-tune encoding quality in specific areas of the video or for particular types of content. This allows for very specific and customized optimization of the encoded results.
It's worth noting that despite advances in hardware acceleration, it seems that the sequential nature of multi-pass encoding doesn't offer the same level of speed increases as single-pass workflows. Hardware acceleration is mostly helpful for processing stages where all operations can happen simultaneously, so it's not as beneficial for processes that need to be executed sequentially like multi-pass encoding.
The choice of video codec can impact the effectiveness of multi-pass encoding as well. A codec like H.265, with its more complex compression algorithms, can benefit greatly from multi-pass encoding because its intricate algorithm can make better use of the additional information gathered across multiple passes. This tends to be more efficient compared to simpler codecs.
Finally, it is worth noting that multi-pass encoding can increase the long-term resilience of video content. By increasing the quality and preserving a higher level of detail within the encoded files, multi-pass methods help make content remain valuable for a longer duration. This is especially useful for archival purposes, helping preserve historical records and ensuring they remain viewable even as technology evolves and changes.
Adobe Media Encoder A Deep Dive into Video Compression Techniques for 2024 - HDR Content Compression Techniques
HDR video introduces new hurdles and potential for video compression. Handling the wide range of brightness and color information inherent in HDR requires specialized techniques. Newer HDR compression methods have begun adopting approaches previously only seen in other fields, like using convolutional neural networks (CNNs) to adjust the bit depth of video data. This allows for a more nuanced representation of HDR's wide color spectrum, especially when converting standard definition material to higher-fidelity formats.
Additionally, more sophisticated methods like End-to-End Deep Video Compression are being tested. These utilize concepts like Rate-Distortion Optimization (RDO) to enhance compression efficiency by improving how motion is calculated within the video. Essentially, they're trying to adapt compression to the specific content of a video. This reflects the overarching trend of taking the methods that work well with standard dynamic range (SDR) content and applying them to the increasingly common HDR content.
While these advanced compression methods offer the promise of better quality and smaller file sizes, they often require significant processing power. This creates a tension between pushing the limits of HDR compression and the practical needs of production environments that are often driven by quick turnaround times. Finding the right balance between advanced compression techniques and manageable processing speed is one of the key issues for video compression going forward.
HDR content presents unique challenges for compression techniques compared to standard dynamic range (SDR) video. The expanded color space, with up to 10-bit color depth offering over a billion colors, requires more sophisticated methods to handle this increased data without significantly increasing file sizes. It's interesting that techniques like perceptual quantization, which consider how our eyes perceive luminance, are becoming more important. These methods prioritize allocating bits to the parts of the image we're most sensitive to, which can noticeably enhance quality without significantly increasing the file size.
HDR content also frequently utilizes scene-dependent compression techniques. This approach allows the compression process to dynamically adapt to the varying brightness and color levels across different sections of the video. Maintaining detail in both bright and dark areas is a crucial aspect of HDR, so it's not surprising that compression has evolved to better preserve those elements. Formats like HDR10 and Dolby Vision also rely on dynamic metadata that changes between scenes, further increasing the complexity of compression methods. The encoder needs to intelligently compress this added metadata without sacrificing the visual impact of the HDR.
While HEVC (H.265) is a common choice for HDR compression, other codecs like AV1 offer potential for even greater efficiency. AV1, with its open-source nature, is an intriguing prospect for streaming platforms hoping to widely distribute HDR content. One of the aspects of HDR content that can sometimes be overlooked is that it's often pre-processed with color grading adjustments to achieve a particular visual aesthetic. This can create some difficulties when compression comes into play. The desire to preserve the artist's vision in HDR can lead to greater computational demand during the compression process.
Encoding HDR content can be resource-intensive, requiring up to four times more processing power compared to SDR video. This can lead to longer encoding times and present bottlenecks in workflows where speed is important. Unfortunately, many of the HDR compression methods can double encoding times in relation to SDR video. This adds an extra challenge for content creators who need to deliver high-quality HDR without excessively long encoding durations.
It's also worth noting that some HDR compression techniques can sometimes unintentionally lose detail in extreme highlight or shadow areas. Peak brightness values can be unintentionally clipped during the compression process, affecting the visual experience. This highlights the fine line that HDR compression methods need to walk in order to achieve a high level of efficiency while minimizing data loss.
Lastly, not all devices are capable of fully displaying HDR content, which can impact the way it's perceived by the viewer. HDR compression approaches need to also take into account the target viewing environment to avoid compressing the content in ways that remove the desired visual impact if the device can't display it correctly. It's an important aspect of the overall HDR pipeline, demonstrating that video compression is highly dependent on the playback device's capabilities.
Adobe Media Encoder A Deep Dive into Video Compression Techniques for 2024 - Cloud-Based Encoding Solutions
In 2024, cloud-based encoding solutions have become increasingly popular, offering a more flexible and scalable alternative to traditional, on-site encoding methods. These solutions rely on the capabilities of cloud computing to handle demanding video processing tasks, letting users encode and convert videos without being restricted by their own hardware. The appeal lies in the efficiency and accessibility they promise.
However, these solutions come with challenges. Potential delays in processing, a reliance on consistent internet connections, and security concerns related to data storage all need to be considered. As the demand for higher-resolution video increases, video professionals need to carefully assess the advantages and drawbacks of cloud-based encoding when making choices in this evolving production environment. The key is to strike a balance between the benefits of cloud-based processing and its operational limitations.
Cloud-based encoding solutions, when considered in the context of video compression and tools like Adobe Media Encoder, offer some intriguing possibilities for 2024. It's fascinating how they can flexibly scale their resources. When demand increases, more processing power becomes available, which can be a lifesaver for teams dealing with fluctuating workloads. They don't need to invest in expensive hardware upfront; instead, they can simply use what they need, paying only for the resources consumed. This flexibility can make cloud solutions particularly appealing to smaller operations.
One of the more striking features of these systems is their ability to split encoding tasks across multiple servers. This parallel processing can dramatically cut down on the time it takes to compress a video, a major advantage compared to relying on a single workstation. However, this approach isn't without its downsides. Cloud-based services sometimes add latency, particularly during periods of heavy usage, which may not suit projects needing incredibly fast turnaround.
The ability to collaborate remotely is another factor that makes cloud solutions intriguing. It becomes much easier for teams spread across various locations to work on the same video files simultaneously, significantly streamlining production processes, particularly in today's remote work environments. It's interesting that some cloud encoding platforms automatically update their encoding capabilities, keeping pace with evolving standards and formats, relieving users of the need to manually upgrade their software.
Cloud providers often incorporate robust disaster recovery features. These built-in backups and redundancy measures mean that encoded files are much safer than when stored locally on a single server, reducing the risk of data loss from hardware failures. It's worth noting that some of the more advanced cloud encoding services are beginning to implement machine learning in their encoding processes. This AI can adapt encoding parameters based on the unique features of each video and past performance, potentially optimizing compression efficiency over time.
Moreover, several cloud services have built-in capabilities for producing various bitrate versions of videos on the fly. This is perfect for adapting to varying internet conditions encountered by viewers, leading to a smoother streaming experience. A lot of the newer cloud encoding services integrate with Content Delivery Networks (CDNs). CDNs facilitate streamlined distribution of compressed videos and ensure faster delivery to end-users around the world, which is especially important for audiences scattered globally.
These features of cloud-based encoding services are a fascinating example of how technology is changing video compression workflows in 2024. While they introduce new possibilities, it's critical to carefully consider the tradeoffs involved, such as latency issues, especially when choosing a service for a particular project.
Upscale any video of any resolution to 4K with AI. (Get started for free)
More Posts from ai-videoupscale.com: