Upscale any video of any resolution to 4K with AI. (Get started for free)
How Video Upscaling Technology Enhances MP4 Conversion Quality in 2024
How Video Upscaling Technology Enhances MP4 Conversion Quality in 2024 - Frame Interpolation Brings SD Videos to Life Through MP4 Processing at 120fps
The ability to insert new frames into a video sequence, a process known as frame interpolation, has progressed considerably. This is particularly evident in recent techniques like the VFIMamba model, which excels at creating intermediate frames for a smoother, more fluid representation of motion at higher frame rates, like 120 frames per second. While this enhances the visual quality of low-resolution videos, its impact goes beyond simple aesthetics. Frame interpolation is also integral for a range of tasks, like restoring old video or producing slow-motion effects.
With a growing need for content in higher frame rates, new approaches are being developed. They aim for efficiency without sacrificing visual quality, tackling the inherent limitations of earlier methods. Ultimately, frame interpolation provides a potent means to improve the overall viewing experience and address the inherent challenges associated with viewing standard-definition videos in a modern context. This is especially pertinent as the quality of our viewing environments and expectations increase. While frame interpolation can improve the look of a low resolution video, there are limitations to the overall resolution that can be achieved by this process.
Frame interpolation, a method often involving optical flow analysis, creates new frames between existing ones, potentially generating up to 240 additional frames within a single second of original video. This ability to effectively increase the frame rate can greatly diminish the appearance of motion blur, especially in sequences with rapid movement, making it valuable for content like sports and action films.
The impact of this processing can be especially noticeable when applied to standard definition (SD) videos. It elevates the perceived smoothness of the footage, making it more comparable to higher resolution formats like HD and 4K, a significant improvement in viewing quality.
However, generating these interpolated frames at high rates like 120fps can strain computational resources. Powerful GPUs are often essential to perform frame interpolation efficiently and prevent noticeable lag or degradation in the quality of the output.
There's a delicate balance in frame interpolation, as the process can introduce artifacts like ghosting or an unnatural appearance of motion, especially when dealing with complex movements or rapidly changing scenes. This underlines the intrinsic trade-off between achieving higher frame rates and maintaining optimal visual fidelity.
Modern frame interpolation techniques are increasingly incorporating machine learning, allowing them to learn from extensive datasets and generate frames more precisely than traditional approaches based on established algorithms.
The utility of this technology expands beyond entertainment, as it has applications in fields such as medical imaging and scientific simulation. The ability to boost frame rates in these domains provides researchers and medical professionals with better tools for analysis and diagnosis.
Frame interpolation can be a powerful tool for revitalizing older video content. It offers a means to improve the visual quality of classic movies and TV shows without extensive and costly remastering.
Furthermore, it finds utility in virtual reality (VR), where it helps to create smoother transitions between frames and reduce feelings of motion sickness often caused by viewing lower frame rate videos in immersive environments.
As frame interpolation continues to evolve, a major focus is on reducing the delay, or latency, involved in real-time video streaming applications. If researchers can successfully minimize latency, it will enhance the fluidity of live video streaming, making it an even more seamless and enjoyable experience.
How Video Upscaling Technology Enhances MP4 Conversion Quality in 2024 - Advanced Denoise Filters Remove Video Grain From Legacy MP4 Files
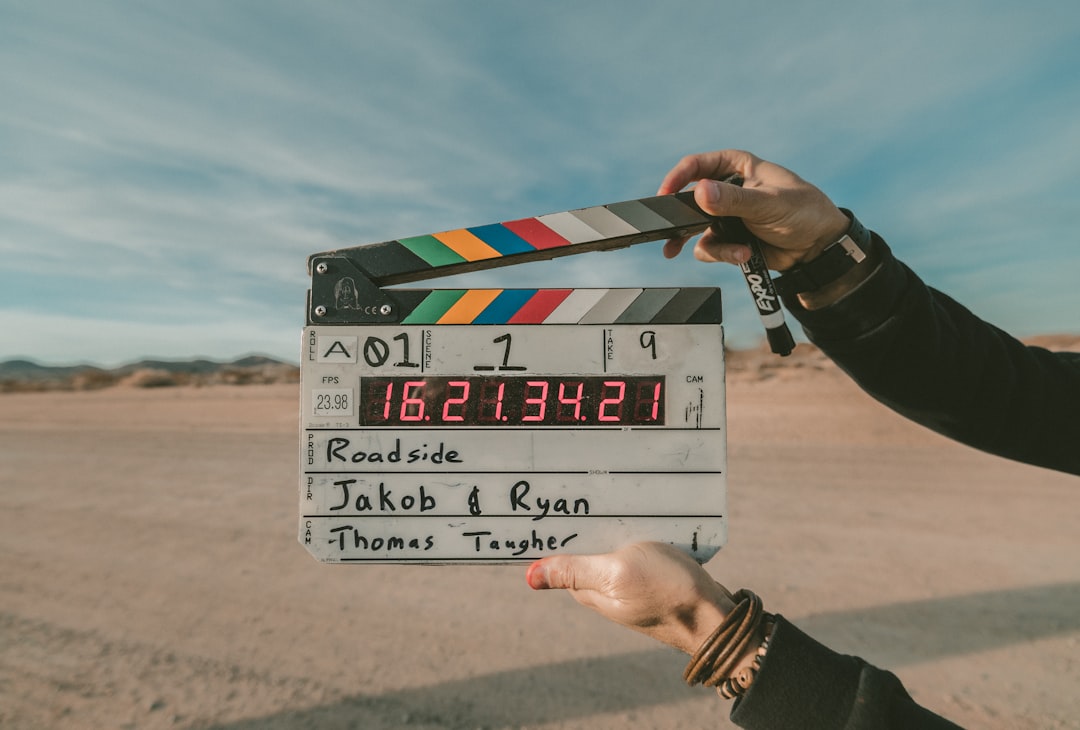
Older MP4 files often suffer from visual noise and grain, particularly those recorded in less-than-ideal conditions. Thankfully, newer video processing techniques are tackling this issue head-on with advanced denoise filters. These filters, increasingly powered by artificial intelligence, are capable of automatically identifying and removing various types of noise and unwanted artifacts. This automated approach makes improving the visual quality of older videos far more accessible compared to older methods.
Some video editing software packages, like Filmora, now offer customizable denoise features. This allows users to fine-tune the smoothness of the resulting video. This is especially helpful for older video content, such as footage recorded in low light or with high ISO settings, where noise is often more pronounced.
This trend of incorporating more powerful denoise features within standard editing software signifies a broader shift. Video editing tools are becoming more comprehensive, providing a wider array of tools that go beyond basic editing. For those who want to enhance older video content, these tools are proving quite useful. While challenges remain in balancing noise reduction with preserving the underlying details in a video, these new technologies represent a leap forward in the capabilities of readily available video editing tools. The overall effect is a path towards cleaner and more visually appealing video content, even for older, lower quality videos.
Advanced denoising filters, employing sophisticated algorithms, are proving quite effective at tackling the noise and grain often found in older MP4 files. This can lead to a cleaner, sharper image, enhancing the overall viewing experience without introducing new visual defects. These filters often incorporate adaptive techniques, intelligently analyzing each frame to differentiate between actual video detail and noise. This targeted approach helps preserve essential information within the video, which is crucial when dealing with older compressed files.
Many legacy MP4s were created with older camera technologies and compression methods, resulting in noticeable artifacts. These denoising filters can specifically target and remove these artifacts, bringing the video closer to modern quality standards. The integration of machine learning has boosted denoising to a new level. These AI-trained algorithms can identify and remove noise patterns that older, traditional filters often miss, significantly enhancing the quality of the video enhancement.
However, the performance of these filters can differ depending on the type of noise or grain in the video. For instance, salt-and-pepper noise responds differently to a filter than Gaussian noise, requiring specialized techniques for optimal results. One interesting consideration is the computational cost of real-time denoising. Higher quality filters can be resource-intensive, often relying on modern GPUs to work efficiently without negatively impacting video playback, especially with higher resolution content.
Beyond basic quality improvements, a surprising benefit of these advanced denoisers is their ability to help with facial recognition in videos. By minimizing noise around facial features like eyes and mouths, the clarity increases, proving useful in security and identification contexts. Video restoration is a rapidly expanding application of denoising. It aims not only to enhance quality but also to revitalize historical footage suffering from decay and compression artifacts, making it more enjoyable for contemporary audiences.
Furthermore, using denoise filters can improve the efficiency of subsequent stages in video editing. By starting with clearer footage, editors can save time during post-production, as they spend less time correcting noise and grain issues. For filters to be fully useful, seamless compatibility with a range of software and editing suites is essential. Many of the newer filters are built to integrate with popular editing platforms, making them accessible for professional and casual content creators alike.
How Video Upscaling Technology Enhances MP4 Conversion Quality in 2024 - Machine Learning Models Now Handle 8K Video Resolution Without Motion Blur
Machine learning models have reached a new level of sophistication, now capable of handling 8K video resolutions without introducing the distracting artifacts of motion blur. This advancement represents a significant leap forward in video processing, especially when compared to older techniques that often struggled with maintaining clarity during fast-paced scenes. The development of AI-powered solutions, like some purportedly superior video upscaling models, illustrates this improvement. These newer models can take lower resolution video and upscale it to 8K, while also enhancing the overall smoothness of motion by increasing frame rates. Furthermore, these new techniques efficiently address previously troublesome issues like blurring and the appearance of digital noise, helping to produce much cleaner and sharper video content. It seems clear that as the expectation for high-quality video viewing continues to grow, these machine learning innovations will continue to shape the future of video fidelity across different devices and platforms. While these developments are exciting, some caution is warranted as the algorithms involved can still be imperfect, sometimes producing other, potentially less obvious, artifacts. However, the potential for higher quality video is clearly here, even for formats as demanding as 8K.
The ability of machine learning models to handle 8K video resolution without introducing motion blur is quite remarkable. These models achieve this by considering the temporal coherence of video sequences. In essence, each generated frame is not just a simple upscaling of the original; it's also designed to seamlessly blend with the surrounding frames, maintaining a natural flow of movement. This is crucial for eliminating artifacts like motion blur, a common issue in upscaled videos.
However, training these models for such high resolutions is a considerable undertaking. They require enormous datasets capturing a wide range of motion and lighting conditions to ensure that they can handle diverse video content effectively. This presents a challenge since the models need to generalize well, producing consistent quality across vastly different source materials.
8K video is computationally intensive. A single frame at this resolution has four times the pixel information of a 4K frame. This necessitates specialized hardware like powerful GPUs and optimized algorithms to ensure that upscaling happens in a reasonable timeframe. It highlights the importance of computationally efficient model architectures and implementation to prevent lengthy processing times.
Furthermore, despite the advancement of machine learning in this domain, challenges remain. Quantization artifacts, stemming from the inherent compression used in many video formats, can become more prominent at 8K. Pre-processing steps are crucial to minimize these issues and improve the overall visual quality.
Interestingly, researchers have begun to incorporate perceptual loss functions into the training process. These functions evaluate quality based on how humans actually perceive video, rather than a simple pixel-by-pixel comparison. This paradigm shift is leading to more realistic and visually pleasing upscaled videos.
Some of the latest models even transcend traditional frame interpolation. They can synthesize completely new frames that better accommodate complex movements, contributing to a much smoother and more natural presentation of motion without creating artificial blur.
While still in the experimental stage, real-time processing of 8K videos using these techniques is gaining momentum thanks to improvements in edge computing. The prospect of dynamically adjusting video quality on the fly in streaming applications is exciting, but will require balancing quality with resource usage.
The rise of 8K video processing is also impacting video encoding. We're likely to see more efficient codecs that handle these high data rates without sacrificing quality, enhancing the overall user experience by minimizing buffering or delays.
Another area of exploration is adaptive training methods. These allow models to modify their learning rate during training based on the complexity of each frame. This adaptation can lead to better handling of challenging video sequences, improving the final output quality.
Beyond entertainment, the capabilities for processing 8K video hold promise in fields like surveillance, medical imaging, and training simulations. In these domains, high-fidelity video can provide more detailed information, improving the accuracy and efficacy of the applications they support.
How Video Upscaling Technology Enhances MP4 Conversion Quality in 2024 - Smart Edge Detection Preserves Details During MP4 Format Conversions
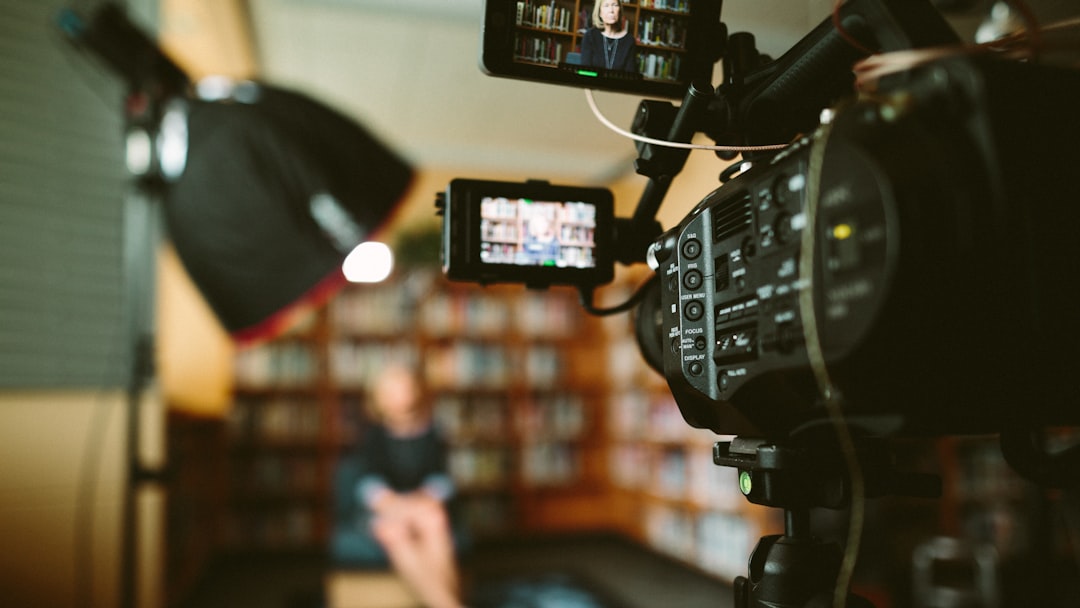
When converting videos to the MP4 format, some details can be lost due to the compression process. "Smart Edge Detection" is a technique that helps mitigate this issue. It leverages algorithms to specifically identify and enhance the boundaries, or edges, within each frame of a video. By refining these edges, the resulting video appears sharper and more defined, leading to a noticeable improvement in overall image quality.
The importance of edge detection has grown alongside the broader trend of upscaling video in 2024. As upscaling technologies strive to create higher-resolution versions of videos, preserving the original level of detail becomes even more crucial. Edge detection helps to ensure the fine details that define the objects and textures in a scene are not blurred or lost during the conversion or enhancement process. While this technology generally improves the visual output, the computational burden of accurately identifying and processing complex edges can still present a challenge, especially in scenes with a lot of intricate detail.
Despite these computational constraints, Smart Edge Detection is an important tool for optimizing MP4 conversions. As the demand for higher-quality video continues to increase, further refining these edge detection techniques will likely be a key part of pushing the boundaries of what's achievable in video processing. It's likely that the ability to process even more complex scenes with less distortion and artifacting will continue to be a priority in the future.
During MP4 format conversions, maintaining the intricate details of a video is crucial for preserving its overall quality. This is where "smart edge detection" comes into play. These algorithms focus intently on identifying and preserving the essential components of a video frame, such as the boundaries of objects and the textures within them. It's essentially a way to keep the important visual information from getting lost during the conversion process.
Interestingly, many smart edge detection methods use what's known as adaptive filtering. Instead of applying a uniform filter across the entire frame, the algorithm dynamically adjusts its approach based on the content of each specific area. This ensures that the filtering process is sensitive to where fine detail needs to be protected and where it can be more aggressively modified to improve the overall video quality.
Furthermore, these modern algorithms often incorporate a spatial-temporal analysis. This means that instead of just focusing on individual frames, they analyze multiple frames simultaneously to better understand the dynamics of motion within the video. This approach can reduce the appearance of artifacts that can occur during conversions, particularly those related to movement, and improves the smoothness of moving objects.
A surprising but beneficial aspect is their resilience to noise. In older MP4 files, which often suffer from noise or grain, these algorithms can effectively distinguish between true video details and noise. This separation allows them to maintain sharpness while reducing the unwanted noise that can detract from a video's clarity.
The mathematical underpinnings of these methods are quite sophisticated, leveraging techniques like gradient-based analysis or even machine learning to further refine their ability to separate visual details from noise or background clutter. It’s fascinating how these algorithms are evolving to become ever more nuanced and effective in preserving intricate detail.
Fortunately, improved hardware allows many of these algorithms to operate in real-time. This capability makes smart edge detection valuable for applications such as live streaming. This means that viewers can enjoy enhanced video quality without experiencing noticeable lag or delays during the conversion process.
Another key element is what's referred to as "multiscale analysis". These algorithms are capable of processing both broad and fine details simultaneously. This feature is especially useful when working with video content that includes a wide range of visual elements, such as landscapes with intricate patterns, as well as objects up close.
It's been suggested that effectively preserving details during conversions might also lead to improved efficiency in video compression. The idea is that when vital features are retained, the video can be compressed more effectively without compromising the overall image quality. This could be an important development, especially given the ever-increasing demand for high quality video content that needs to be efficiently stored and transmitted.
However, there are limitations. While smart edge detection excels in dynamic videos, it can occasionally struggle with video content that lacks motion or has a very consistent structure. In such cases, the algorithms may overlook subtle details that are not highlighted by changes in the visual content over time.
Finally, the applications of smart edge detection extend beyond general entertainment. In fields like medical imaging, the preservation of fine details is vital for diagnosis. Similarly, autonomous vehicles rely on accurate edge detection to navigate complex surroundings. These broader applications highlight the versatility and increasing importance of this technology across various domains.
How Video Upscaling Technology Enhances MP4 Conversion Quality in 2024 - Automated Color Correction Adjusts Video Tone Mapping in Real Time
In the realm of video editing in 2024, automated color correction has become a vital tool for refining the visual appeal of videos. This technology utilizes artificial intelligence to automatically adjust aspects like brightness, contrast, and saturation in real time. Essentially, it fine-tunes the video's tone mapping, helping to create the desired mood and atmosphere within each scene. This real-time capability is a significant improvement over traditional methods, as it enables editors to instantly see the effect of color changes and refine the look of the video as they work.
The benefits of this approach are clear. It simplifies the color grading process, allowing content creators to achieve professional-looking results without needing extensive color correction experience. Automated color correction brings consistency to videos, helping to maintain a unified look across different scenes and clips. This streamlining of the process can lead to increased efficiency, especially for individuals or teams working on projects with tight deadlines.
However, the automation aspect can also present some challenges. While the algorithms are designed to intelligently adjust colors, it's possible that they can sometimes overcorrect, leading to a loss of the original artistic intent behind the footage. Overuse of these automated features can result in videos that look unnatural or overly stylized. Careful attention and some level of human intervention are still often needed to achieve the perfect balance.
Despite these limitations, automated color correction has firmly established itself as an essential aspect of contemporary video editing. As we move forward, the development of increasingly sophisticated algorithms will likely refine the process further, making it even easier to achieve a high level of visual quality in videos. As the demand for high-quality, easily consumable video content grows, the ability to refine colors and video tone quickly and dynamically through real-time processing will be increasingly critical across different video production and consumption platforms.
In the ever-evolving landscape of video processing, automated color correction has emerged as a powerful tool for enhancing video quality in real time. This fascinating area of research blends computational techniques with artistic sensibilities to refine video tone mapping dynamically. Let's explore ten intriguing aspects of this technology that might spark a researcher's curiosity.
First, these automated systems often employ what is called dynamic color grading. They rely on algorithms to analyze video content in real-time, adapting color adjustments as the scenes change. This ensures visual consistency and helps maintain the desired aesthetic throughout the entire video. Second, they often incorporate histogram equalization techniques. This approach helps to evenly distribute the brightness and contrast of a video, preventing issues like overexposed or underexposed areas, which often can negatively affect the visual appeal. Third, the integration of machine learning is becoming increasingly common in these systems. These models are trained on vast datasets of video content, enabling them to "understand" color distributions in diverse environments and make more intelligent adjustments. This results in a more nuanced approach to color correction that often mimics the skills of experienced professional colorists.
Fourth, automated color correction systems often make use of video metadata. This metadata, which includes information like the camera used, lighting conditions during filming, and even specific lens characteristics, can be valuable in optimizing the tone mapping process for each project. This personalized approach helps ensure that the results are consistent with the creator's intent and the nature of the video content. Fifth, the advancement of cloud computing and edge processing technology has made real-time color correction during live streams a reality. This is exciting since it allows broadcasters to enhance the visual quality without straining the capabilities of local devices or requiring specialized hardware. This helps ensure seamless and visually-appealing broadcasts for various applications.
Sixth, these systems can handle the intricacies of color space conversions. This means that footage originally captured in one color space, such as Rec. 709, can be smoothly transitioned to others, like DCI-P3 or Rec. 2020, without sacrificing color accuracy. This is important because it enables the creators to share their work in a wider range of formats. Seventh, the automated processes can help prevent issues related to tone mapping, including banding or posterization. This is crucial in situations where there are many subtle gradients or color variations in the scene, as these issues can be quite distracting to viewers. Eight, in advanced implementations, these systems might utilize spectral analysis techniques. They examine the distribution of colors throughout the visible spectrum, enabling them to ensure that the adjustments maintain accurate and visually pleasing representations of colors.
Ninth, although primarily automated, many of these systems still allow for a level of user customization. This enables editors to make fine-tuning adjustments after the initial automated color correction has been completed. This is a smart combination of automation and manual control, allowing for creative input while streamlining the color grading process. Finally, it is important to recognize that the applications of automated color correction are not limited to standard video formats. They are being used in increasingly complex environments, including virtual reality and augmented reality applications. In these settings, real-time color adjustments are critical for creating a sense of realism and immersion, furthering the potential of these environments.
The field of automated color correction is dynamic, evolving with improvements in machine learning and computer processing. As both hardware and algorithms become increasingly powerful, we can anticipate that these techniques will continue to transform the way we experience video content across various platforms and applications.
How Video Upscaling Technology Enhances MP4 Conversion Quality in 2024 - Neural Networks Enable Stable Frame Rate Conversion Without Quality Loss
Neural networks are increasingly vital in video processing, especially when it comes to smoothly converting frame rates without sacrificing visual quality. These networks excel at creating new frames, called interpolation, using sophisticated machine learning techniques, leading to smoother motion in videos. Older approaches to frame interpolation were often hampered by things like unnatural-looking motion or blur. However, neural networks, utilizing convolutional and recurrent network structures, are generating better results. There's also growing interest in generative adversarial networks (GANs) for frame rate upscaling. GANs have the potential to create even more seamless transitions between frames, proving helpful in things like video streaming or creating virtual reality experiences. As this area of research continues, the focus is on optimizing these techniques to deliver higher frame rates while preserving the standards of modern viewing experiences.
Neural networks are proving increasingly valuable in frame rate conversion, particularly for enhancing video quality without introducing noticeable degradation. They surpass traditional methods by meticulously analyzing motion vectors within a sequence, leading to a better understanding of scene dynamics. This approach leverages temporal coherence, a crucial factor in maintaining the smoothness and fidelity of motion when inserting new frames.
The incorporation of adaptive learning further refines the interpolation process. Neural networks can modify their behavior based on the content and motion of each scene, enabling more accurate and contextually relevant frame generation. This dynamic adaptation helps to minimize the occurrence of artifacts and unnatural motion that often accompany traditional interpolation techniques.
However, this improved accuracy comes with a computational cost. While frame rate conversion has always been resource-intensive, advancements in neural network architectures, particularly those utilizing convolutional layers, have significantly improved processing efficiency. This is crucial for enabling real-time applications without sacrificing output quality, which is critical for seamless user experience.
Deep learning models are particularly effective at reducing common visual artifacts like ghosting and blurring. These models can learn optimal motion characteristics from large training datasets, leading to interpolated frames that appear smoother and more visually appealing.
While primarily focused on entertainment, these neural network-driven frame rate conversion techniques have applications in other fields. Medical imaging, for instance, benefits from higher frame rates for more detailed analysis and diagnosis. Similarly, sports analytics and simulations utilize this technology to capture and analyze motion in intricate detail.
Recently, the use of generative adversarial networks (GANs) has gained momentum for frame interpolation. These models are capable of synthesizing entirely new frames, effectively enhancing the quality and realism of video sequences. This holds a lot of promise, but it's early days still.
However, relying on machine learning also introduces a caveat: the dependence on robust and diverse training data. If the training data fails to encompass a wide range of motion scenarios, the model's ability to generalize may decline, leading to less desirable interpolation results.
Furthermore, the latency involved in real-time frame rate conversion remains a challenge. While processing power has increased, generating interpolated frames fast enough for live broadcasts can still be difficult, occasionally disrupting the viewing experience. Addressing this latency remains an ongoing area of research.
Interestingly, there's a growing trend toward integrating neural networks with edge computing. This means running the networks on the devices themselves, making it possible to achieve near-instantaneous frame rate conversions on devices like smartphones or smartwatches. This could reduce dependence on cloud services and increase the quality of video experiences across various platforms and environments.
Lastly, when utilizing frame rate conversion for sensitive content, such as older film or video art, a crucial consideration is the need to carefully balance the enhancement of the original material with the preservation of the original artist's intent. If these advancements are not applied with care and awareness, valuable visual details and stylistic choices may be inadvertently lost or distorted.
These ongoing developments in neural network-driven frame rate conversion suggest that we can expect to see more advanced and powerful upscaling techniques in the years to come. The future of video quality may depend heavily on the innovative applications of machine learning.
Upscale any video of any resolution to 4K with AI. (Get started for free)
More Posts from ai-videoupscale.com: