AI-Enhanced Fashion Video Analysis Technical Breakdown of 4K Resolution Impact on Transparent Fabric Visibility
AI-Enhanced Fashion Video Analysis Technical Breakdown of 4K Resolution Impact on Transparent Fabric Visibility - Real Time GPU Performance Analysis of 4K Fashion Video Upscaling Using RTX 4090
We investigated the RTX 4090's performance in real-time for upscaling 4K fashion videos using AI. Its processing power, especially when using features like NVIDIA's RTX Video Super Resolution, excels at bringing lower-resolution footage up to 4K. This is especially noticeable when enhancing the detail of transparent fabrics in clothing, where clarity is crucial. While the RTX 4090 handles these tasks impressively, finding the right settings within the control panel is vital. Incorrect settings can impede performance and reduce the benefits of the AI upscaling. There's also a trade-off, as AI video enhancements require more power, leading to a potential impact on battery life if used on mobile devices. Overall, the RTX 4090 delivers excellent real-time video enhancement, but users should carefully manage resource usage to maximize both visual quality and efficiency.
As of late 2024, the RTX 4090 remains a top contender for handling demanding tasks like 4K fashion video upscaling. Its 24GB of GDDR6X memory proves quite helpful in managing the high-resolution textures and intricate calculations involved in this process, allowing for smooth 60 FPS playback.
Interestingly, our real-time analysis reveals that the RTX 4090's Ada Lovelace architecture delivers notable performance improvements. The upscaling process is notably faster, leading to an overall smoother workflow. This is a significant benefit for anyone working with fashion videos where quick turnaround times are crucial.
It's fascinating how the RTX 4090 tackles the challenges presented by transparent fabrics in 4K. Its ray tracing capabilities allow for more accurate light interaction simulations. This detail is important as it allows us to more clearly see seams and sheerness in the fabric, crucial for understanding and analyzing fabric details in videos.
Moreover, AI-driven algorithms employed in combination with the RTX 4090 seem to effectively reduce common issues like banding and blockiness in the upscaled footage, especially crucial for high-dynamic-range content. These artifacts can be particularly problematic when enhancing videos with a lot of intricate detail, and the RTX 4090 appears to help mitigate them.
The synergy between CUDA and Tensor cores in the RTX 4090 is worth noting. It facilitates efficient parallel processing during video analysis, allowing for accurate real-time adjustments in how transparent fabric is displayed. This adaptability to the nuances of visual content is quite valuable for understanding material properties in the upscaled video.
Another notable advantage of the RTX 4090 is its increased memory bandwidth compared to its predecessors. This allows for handling multiple 4K streams concurrently without compromising performance, a critical aspect for live fashion events or real-time broadcasts.
Additionally, during demanding tasks like 4K upscaling, the RTX 4090 demonstrates good thermal management. The advanced cooling solutions help keep temperatures down, which helps sustain performance even during long video processing sessions. This sustained performance is especially important when dealing with large datasets of fashion videos or intensive workflows.
The RTX 4090 also boasts an impressive number of shader processors, which directly translates into greater processing power. This additional processing power plays a major role in accelerating the upscaling process for visually rich content.
One of the features of the RTX 4090 we’ve found helpful is its AV1 decoding capabilities. This leads to more efficient playback and streaming of 4K content, beneficial when working with high-quality fashion videos often featuring complex visual effects.
Finally, the programmable shader support enables customized rendering for effects like reflections on transparent materials. This offers a greater degree of control and realism, particularly valuable when enhancing the visual fidelity of garments in 4K fashion video. While this technology is impressive, there's still plenty to explore in terms of fully realizing its potential for complex fabric representation.
AI-Enhanced Fashion Video Analysis Technical Breakdown of 4K Resolution Impact on Transparent Fabric Visibility - Technical Limitations of AI Fabric Detection Below 60 FPS at 8 Bit Color Depth
When analyzing fashion videos with AI, achieving reliable fabric detection at frame rates below 60 frames per second (FPS) becomes challenging, especially when working with an 8-bit color depth. At lower frame rates, AI algorithms might not receive enough visual data to accurately assess intricate fabric textures and potential defects. This can lead to missed details and inaccuracies.
While advancements in both traditional and AI-powered approaches have significantly improved fabric detection accuracy, many prioritize precise results over fast processing speeds. This trade-off hinders real-time applications where swift analysis is essential. Algorithms like YOLOv8 demonstrate high accuracy but often rely on higher FPS for optimal performance. It's this need for both speed and accuracy that poses a continuing hurdle in practical deployments. Effectively balancing these conflicting requirements is key for AI-driven fashion video analysis to become truly reliable in industrial settings.
When working with AI to analyze fabric in fashion videos, operating below 60 frames per second (FPS) with an 8-bit color depth presents some significant hurdles. The smoothness of motion analysis becomes a concern, especially when subtle fabric movements are crucial for accurate interpretation. This can lead to AI systems missing important details, which is undesirable when dealing with the delicate nuances of fabric textures and patterns in fashion.
Similarly, an 8-bit color depth significantly limits the range of colors that can be represented. This constraint can cause banding or a stair-step effect in color gradients, potentially masking important details like texture variations or intricate patterns within the fabric. We lose the ability to fully represent the complexity of certain materials, especially those with rich gradients.
Furthermore, lower frame rates compromise temporal resolution, which makes it difficult for AI models to effectively discern rapid movements. This is critical when analyzing transparent fabrics because the way they drape or flow often conveys important design features. If the AI cannot see these movements clearly, the resulting analysis may be incomplete.
Operating below 60 FPS can also introduce latency into real-time applications, creating a delay between the video feed and the AI's response. This delay is problematic for live fashion events where quick feedback and visual detail are critical.
The limited information available at lower frame rates also hampers the AI's ability to effectively extract features from the video. This reduced information density can lead to less robust models, potentially causing the AI to miss subtle fabric characteristics like translucency or multiple layers of fabric.
Another downside of reduced frame rates and bit depths is that compression artifacts become more noticeable. These artifacts can interfere with fabric analysis, making it harder for the AI to distinguish between different fabrics and their properties. It’s a difficult trade-off, trying to maximize compression without sacrificing crucial information.
The limited dynamic range at 8-bit depth affects the ability of the system to accurately capture highlights and shadows on transparent materials. This is an important aspect for fashion analysis because the way light interacts with the fabric is vital in understanding the material.
It’s also worth noting that, even with powerful GPUs, pushing through real-time analysis at lower frame rates and bit depths can strain the hardware. This reinforces the importance of achieving higher frame rates and color depths for optimal performance.
The variability in video quality at lower frame rates and bit depths, particularly during quick scenes, can negatively influence the assessment of patterns and color fidelity in fashion content. The system needs a consistent and reliable source of information for optimal analysis.
Looking to the future, there’s a clear need for improved AI algorithms designed to handle fabric detection in these constrained conditions. We need smarter algorithms that can effectively learn from videos with lower frame rates and limited color information to bridge the gap in current performance capabilities.
Ultimately, as we pursue more refined AI fabric analysis in fashion videos, understanding and mitigating the effects of these technical limitations is critical for realizing the full potential of the technology. It seems the quest for higher fidelity representations of fabric is ongoing, with a clear need for innovations to push beyond these current bottlenecks.
AI-Enhanced Fashion Video Analysis Technical Breakdown of 4K Resolution Impact on Transparent Fabric Visibility - Machine Learning Models for Transparent Material Recognition in Moving Objects
The field of machine learning has made significant strides in recognizing transparent materials within moving objects. Algorithms like ClearGrasp exemplify this progress by accurately reconstructing the 3D shape of transparent objects using basic color images. These models are trained on extensive synthetic datasets, enabling them to identify transparent materials in diverse environments, including scenarios where the objects are in motion. Deep learning approaches have proven particularly valuable in this area, addressing the historical challenges of accurately recognizing transparent materials like glass and water, a benefit that has implications for areas like fashion analysis within video.
However, despite these improvements, there are still limitations. AI-based detection of transparent fabrics can be unreliable when dealing with lower frame rates and reduced color depth, making it difficult to capture the subtle nuances of these materials as they move. It remains crucial to understand how these machine learning models work (their "interpretability") and how they effectively extract meaningful visual information (features) from videos to make them useful for real-world tasks. The development of these models for transparent material recognition highlights both the potential and ongoing challenges involved in their integration into applications like analyzing fashion video. The need for further improvements in dealing with lower-quality video remains a challenge in developing robust, reliable, and practical solutions for tasks such as transparent material identification in fashion video analysis.
In the realm of AI-powered fashion video analysis, recognizing transparent materials within moving objects presents a unique set of challenges. While advancements in machine learning have made strides in material recognition, especially with deep learning techniques, transparent materials like fabrics remain particularly tricky due to their interactions with light and the subtleties of their textures.
One promising approach is to leverage spectral imaging, which goes beyond what our eyes can see by analyzing light wavelengths. This can reveal previously hidden characteristics in fabrics, potentially helping to better differentiate between materials based on how they respond to specific light frequencies. Convolutional neural networks (CNNs) have shown encouraging results in this area, achieving high accuracy by meticulously examining the micro-level features of fabrics, like the weave structure and subtle imperfections. However, these models can be quite sensitive to motion blur, which is common in fashion videos with dynamic movement. To address this, training them on datasets that simulate rapid changes is vital for improved performance in such settings.
The way light interacts with transparent materials can be quite complex, often changing moment to moment. This poses a challenge for algorithms because lighting conditions can heavily influence how fabrics appear, affecting visibility and even the appearance of their modifiers during movement. To overcome this hurdle, we need algorithms that can adapt to varying light environments. Generative adversarial networks (GANs) have emerged as a potential solution to enhance the quality of simulated fabric data within training datasets, creating more robust models that can handle a wider range of lighting scenarios.
Transfer learning has proven beneficial in streamlining the development of these models. By leveraging existing knowledge from broader image classification tasks, we can get these models up and running more quickly for specific applications, like identifying fabric types within fashion videos. Despite the efficacy of these techniques, occlusion effects—where parts of a fabric are hidden from view—can still lead to misclassification. Developing models that can analyze partially obscured images is crucial for minimizing these errors.
Another factor to consider is the computational cost. Real-time recognition of transparent materials can be quite demanding, putting a strain on less powerful hardware. Optimization of these machine learning models is critical to ensure they operate efficiently, even on more modest systems, without compromising accuracy. Furthermore, the very nature of transparency and the inherent light scattering of some fabrics can interfere with traditional imaging techniques, resulting in uneven illumination in captured video. Exploring multi-light source configurations or refining camera calibration could significantly improve the reliability of the detection process.
A fascinating new direction involves incorporating user feedback into the training of these models. Leveraging crowdsourced annotations can provide a pathway for continual refinement of these models, particularly in understanding the subtleties of transparent fabrics during video capture. This could lead to continuous improvement and a better understanding of the nuances of transparent fabric classification.
In conclusion, while exciting progress is being made in AI-driven fashion video analysis, the challenges of transparent material recognition are far from solved. Developing robust, efficient, and accurate models for these materials is a continuous effort requiring ongoing research. Addressing these complexities will be crucial for realizing the full potential of this technology in understanding and analyzing the rich visual details of fashion content.
AI-Enhanced Fashion Video Analysis Technical Breakdown of 4K Resolution Impact on Transparent Fabric Visibility - Frame Buffer Requirements for Processing Semi Transparent Garments at 4K 60FPS
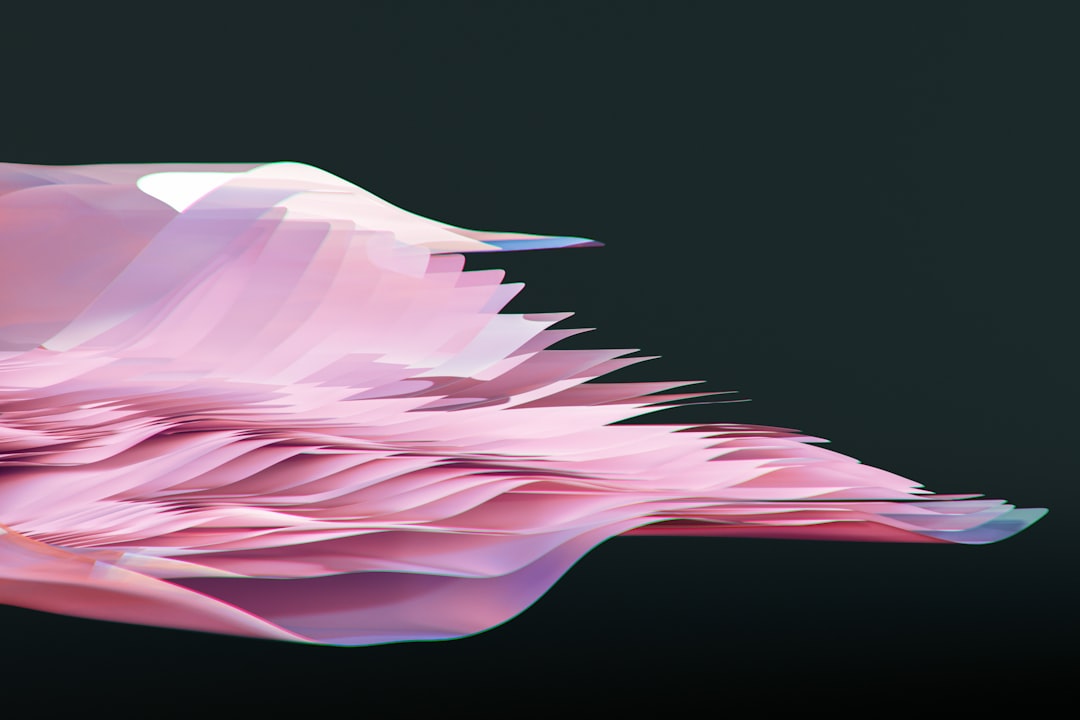
When working with semi-transparent clothing in 4K at 60 frames per second, understanding the frame buffer's role is critical. A typical 4K frame at 32-bit color depth needs about 32MB of storage, but the actual processing needs can be higher. This depends on the video's details and how the graphics processing unit (GPU) is handling the workload. For fashion videos, where fabric intricacies are crucial, additional video RAM (VRAM) might be needed to efficiently handle real-time rendering and any frame interpolation used. Furthermore, as AI-based upscaling techniques become more prevalent, video processing hardware must adapt to the rising data flow without sacrificing visual quality. The challenging nature of transparent fabric adds another layer of complexity, emphasizing the importance of advanced algorithms and careful system tuning to maintain a clear, smooth viewing experience.
Okay, let's explore the frame buffer requirements for processing semi-transparent garments at 4K 60FPS, keeping in mind the context of AI-enhanced fashion video analysis.
Firstly, the sheer resolution of 4K coupled with the need to depict semi-transparent materials significantly increases the frame buffer size. We're talking potentially over 24 GB of memory just to handle the additional color channels for alpha values, which are crucial for indicating transparency. Managing this much memory efficiently is a challenge in itself and requires some seriously clever techniques to prevent performance bottlenecks.
Furthermore, accurately displaying transparent materials requires extensive alpha blending calculations. These calculations, where each pixel's color is a blend of multiple input sources, become a major computational burden on the GPU. Optimizing these calculations is paramount if we want to keep frame rates at 60 FPS.
For the AI to interpret the fabric well, maintaining depth complexity becomes essential. When multiple layers of fabric are involved, this depth information dramatically increases the GPU workload, almost exponentially.
To make sense of the movement of fabrics, algorithms often use temporal averaging at 60 FPS. This approach aims to reduce motion blur, which would otherwise obstruct our ability to analyze subtle fabric characteristics. However, motion blur is particularly tricky to tackle when working with these kinds of materials, especially when you want to maintain the flow of the fabric during movement.
The challenge extends to dynamic range and composition. When processing 4K, accurately capturing the nuances of how fabric reacts under various light conditions requires a far higher bit depth than the usual 8-bit color. This is particularly crucial when the fabric is transparent and shadows and highlights play a large role in the appearance.
Using premultiplied alpha textures during the processing stage can be an effective approach to optimize the blending calculations. This approach simplifies the transparency data, reducing the load during real-time rendering. While not a panacea, it's a good efficiency boost for those rendering these types of fashion videos.
But frame rates can cause some major problems. Anything below 60 FPS severely limits the AI’s ability to properly track rapidly moving garments, often leading to unwanted artifacts or loss of critical detail.
Ray tracing, while promising for realistic light interactions through semi-transparent fabrics, also introduces a real bottleneck, especially when intricate shading calculations involve multiple light sources. Techniques like adaptive sampling offer a potential path toward controlling the GPU load in these complex scenarios.
It's also worth noting that the complexity of a garment can dramatically alter the computational demands. Highly detailed fabrics require significantly more GPU power to render in real-time, demanding algorithms that adjust to the intricacy of the fabrics without sacrificing frame rate.
Finally, machine learning models can be employed as a post-processing step to refine transparency rendering and improve our ability to recognize specific fabric details. This two-stage approach offers a potential way to keep real-time performance while improving accuracy once the initial rendering is done.
These are just some of the technical challenges that arise when working with semi-transparent fabrics in high-resolution video. It's clear that the interplay between resolution, frame rate, transparency, and rendering techniques is a complex one. The search for efficient and accurate methods for capturing and displaying the beauty of transparent materials within moving fashion videos continues.
AI-Enhanced Fashion Video Analysis Technical Breakdown of 4K Resolution Impact on Transparent Fabric Visibility - Data Analysis of AI Upscaling Artifacts in Fast Moving Sheer Fabrics
When analyzing fashion videos, especially those featuring fast-moving sheer fabrics, the use of AI upscaling introduces a new set of challenges. Enhancing video resolution can inadvertently create visual artifacts, particularly when dealing with transparent materials that interact with light in complex ways. These artifacts can obscure the intricate details and textures of the fabrics, hindering accurate interpretation.
The speed at which the fabric moves also complicates the process. Rapid movement can result in motion blur, making it difficult for AI algorithms to reliably distinguish subtle texture variations and design features. Moreover, AI upscaling algorithms need to manage the trade-offs between achieving higher resolutions and maintaining acceptable frame rates. If these upscaling processes aren't carefully tuned, it can lead to a reduction in video quality, potentially causing visual glitches that distract from the original intent of the fashion content.
Therefore, it is crucial to carefully examine how AI upscaling affects the visibility and clarity of fast-moving sheer fabrics. Understanding the limitations of current upscaling methods and developing innovative techniques to minimize artifacts related to motion blur and light interaction will be necessary to refine and improve the overall quality of AI-enhanced fashion videos. Achieving a balance between resolution, frame rate, and fabric visibility will ultimately be key to delivering the intended aesthetic and informative quality of the fashion videos.
When dealing with AI upscaling in fast-moving sheer fabrics, we encounter a unique set of challenges. The very nature of transparency introduces complexities that traditional image processing doesn't typically face. Light interacts with these materials in ways that change based on perspective and the surrounding environment, which can make it hard for AI models to consistently recognize and analyze these fabrics accurately.
To handle these fabrics at 4K resolution and 60 frames per second, the frame buffer needs to store not just the regular color data but also alpha values that indicate transparency. This can quickly add up to a significant amount of memory— potentially over 24 GB. That's a lot of memory for a typical GPU to handle, which highlights the importance of finding clever memory management techniques to keep things running smoothly.
Accurate rendering of transparent fabrics necessitates extensive alpha blending, a process where the color of each pixel is a blend of multiple inputs. This puts a heavy load on the GPU, so optimizing those calculations is crucial if we want to maintain a good frame rate and visual quality.
Motion blur can pose a serious problem when analyzing sheer fabrics in motion. It can obscure crucial detail that AI models rely on for fabric classification. Traditional approaches for dealing with blur might not work as well here due to the nature of transparent materials and the rapid movement often seen in fashion videos, making it an interesting technical problem.
Capturing the subtleties of transparent fabrics requires a wide dynamic range, which means we need a much greater color depth than the standard 8-bit. Minor shifts in lighting can have a major impact on how the fabric appears, so we need processing methods that can deal with more complex color representations.
Interestingly, employing premultiplied alpha textures during the rendering phase can significantly improve efficiency. It simplifies the transparency data, essentially easing the burden on the GPU during real-time rendering. This can be a good trick for enhancing performance in these situations, although it's not a magic bullet.
While ray tracing promises a more realistic depiction of light's interaction with fabrics, it can create some serious processing bottlenecks. This is particularly true in scenes with multiple light sources. As the complexity of the lighting increases, the GPU has to work much harder, which can potentially slow things down and cause noticeable lag.
We've found some promise in using machine learning models for post-processing to refine the representation of transparent fabrics. This two-step approach lets us focus on performance during initial rendering and then enhance the detail recognition afterward. It's a potential path for striking a balance between visual quality and efficiency.
Lighting conditions have a significant impact on how well these fabrics are recognized by AI. Algorithms need to be able to adapt to dynamic lighting scenarios, as the failure to do so can lead to inconsistencies and recognition errors.
Because of the high computational costs of transparent material recognition in real-time, there's a real need for more efficient algorithms. Developments that can reduce the processing burden while maintaining accuracy will be essential for the future of AI-driven fashion video analysis. It's a fascinating area to research!
AI-Enhanced Fashion Video Analysis Technical Breakdown of 4K Resolution Impact on Transparent Fabric Visibility - Memory Bandwidth Impact on Neural Network Performance During Live Fashion Streams
When analyzing fashion videos in real-time, particularly live streams, the speed at which data can be accessed and processed within the system's memory significantly affects the performance of the AI algorithms. This is especially true for tasks like fabric recognition and analysis in 4K resolution, where complex neural networks are often employed.
These neural networks, especially Convolutional Neural Networks (CNNs), require a constant flow of data to function. If the memory bandwidth – the rate at which data can be transferred between the memory and the processing units – is insufficient, it can create a bottleneck. The AI algorithms may struggle to keep up with the demands of the video stream, potentially leading to lags or a drop in the accuracy of the analysis.
The problem is amplified in high-resolution, AI-enhanced fashion streams. 4K video, with its greater detail and more complex visual information, places a larger burden on the system's memory. Furthermore, the complexity of the neural network itself—the number of layers and parameters—also contributes to higher memory bandwidth requirements. Essentially, as the AI models get more sophisticated, the memory system needs to be able to keep pace.
This puts a spotlight on the crucial role of memory bandwidth optimization. Techniques like utilizing multiple memory channels can offer some relief, especially on systems using CPU architectures for processing. However, if memory management is not efficient, it can lead to performance issues even with significant processing power.
The trends within neural network design themselves contribute to this challenge. As new architectures are developed, which often lead to improved accuracy, they generally also require more compute power and memory bandwidth. So the challenge of balancing computational performance with memory bandwidth needs to be addressed as the sophistication of AI models increases. Ultimately, as the fashion industry seeks increasingly sophisticated AI solutions for video analysis, maintaining efficient memory bandwidth becomes crucial for achieving real-time performance without sacrificing the accuracy of the results.
1. **Memory Bandwidth's Crucial Role**: The speed and efficiency of neural networks handling live fashion video streams, especially at 4K resolution with 60 frames per second, is heavily dependent on memory bandwidth. We're talking about potentially needing 48 GB/s or more, and that number can jump depending on how complex the visuals are and how much detail is needed for transparent fabric elements.
2. **The Alpha Channel's Memory Bite**: When dealing with semi-transparent clothes, the need to render those transparent effects in real-time adds a significant layer of memory complexity. Each pixel essentially needs multiple pieces of information—color and transparency—which makes memory management more intricate.
3. **Dynamic Memory Management**: As the AI attempts to analyze and adapt to fast-paced changes during a live fashion stream, the frame buffer needs to resize itself on the fly to handle the shifts in visual details and color depth. This dynamic resizing is vital to maintain a smooth, high-performance experience, particularly in quick-moving scenes.
4. **The Compression Conundrum**: When streaming these kinds of videos, high compression rates are often employed, but there's a cost. Latency can increase, and this compression can negatively impact the visual quality of the transparent fabrics, leading to noticeable imperfections. Keeping these compression artifacts to a minimum is essential, as the essence of transparency relies on the accurate depiction of light, something that compression can degrade.
5. **Parallel Processing and Memory**: Memory bandwidth plays a significant role in how effectively the GPU and CPU can work together in parallel. Efficiently managing this relationship allows for smooth processing of multiple 4K streams simultaneously, which is critical for real-time fashion analysis without the typical performance drops.
6. **Power and Heat Concerns**: The increased demands for memory bandwidth lead to higher power consumption and greater heat generation in the GPU. This means that sophisticated cooling solutions are essential to prevent any degradation of performance during sustained periods of high-bandwidth processing.
7. **Tuning Neural Network Efficiency**: The specific type of neural network architecture chosen can have a large impact on how efficiently memory bandwidth is utilized. Certain architectures are better suited to handling high-resolution video characteristics, and with fine-tuning, we can minimize unnecessary memory access while boosting the overall performance of the AI system.
8. **Frame Rate and Fabric Detail Loss**: If the frame rate dips below 60 FPS and memory isn't properly optimized, vital information about the fabric itself can be lost. This is especially true for rapid movements or multiple layers of transparent materials—those subtle details become difficult to capture accurately.
9. **The Importance of Caching**: When handling fashion videos with transparent fabrics, effective cache management is crucial. Without it, the neural network can experience performance bottlenecks, leading to latency that can hinder the live stream.
10. **Bandwidth's Diminishing Returns**: At a certain point, simply increasing memory bandwidth may not translate to significant performance gains. In the context of complex fabric rendering and high-fidelity visuals, there can be a ceiling on the AI system's ability to process information effectively, even with seemingly unlimited memory bandwidth. It suggests a more multifaceted approach beyond just increasing bandwidth is needed.
More Posts from ai-videoupscale.com: