Telequality Video Player Integrating AI Upscaling for Enhanced Streaming Experience
Telequality Video Player Integrating AI Upscaling for Enhanced Streaming Experience - AI Upscaling Technology Enhances Video Quality
AI upscaling has emerged as a powerful tool for refining video quality, leveraging machine learning to achieve results beyond traditional methods. These systems use complex algorithms, often based on deep learning, to analyze and enhance video frames. The outcome? Videos with greater detail, reduced noise, and a clearer overall picture, transforming lower quality sources into sharper, more defined visuals. This isn't just about making things look better; it's about addressing the limitations of traditional techniques like interpolation. AI upscalers can intelligently analyze and fill in missing information, producing smoother transitions and a more visually compelling experience. Examples like NVIDIA's RTX Video Super Resolution or Adobe's experiments show how AI can significantly enhance resolution and eliminate compression artifacts. The influence of AI isn't confined to pre-recorded content; it's also impacting the live streaming landscape. The ability to adjust video quality in real time, based on factors like network bandwidth, opens up exciting possibilities for seamless and adaptive viewing experiences. While this technology promises impressive results, the wider adoption and impact on the future of video consumption will require ongoing evaluation and scrutiny of both its capabilities and limitations.
AI upscaling is a fascinating field that utilizes convolutional neural networks (CNNs) to essentially "guess" what a higher-resolution version of a video might look like. These algorithms can take a standard definition video and make it appear much sharper and more detailed, even approaching 4K quality in some cases.
This isn't just simple pixel duplication. These systems use deep learning to learn complex visual patterns, like edges and textures, that traditional upscaling techniques often miss. They can learn from massive datasets of high-definition content, allowing them to recreate those intricate details and replicate visual characteristics that are difficult to achieve with older methods.
However, there's a trade-off. The upscaling process itself can generate visual distortions, like a halo effect around object edges. This indicates a need for fine-tuning the AI models to strike a balance between enhancing quality and reducing artifacts.
Furthermore, these systems can be trained to adapt to different video content. They can apply various enhancement techniques for things like animated movies versus real-life recordings, trying to produce an optimal viewing experience for each scenario.
The use of generative adversarial networks (GANs) is an intriguing recent development in video upscaling. GANs pit two neural networks against each other—a generator and a discriminator—to refine textures in upscaled footage and make it look more natural.
One major advantage of AI upscaling is its potential for real-time processing. This opens up possibilities for low-latency applications, which benefit from high-quality video streaming without introducing excessive delay. However, the computational demands are significant, presenting challenges for environments with less powerful hardware or slower internet connections.
It's important to consider that how a video is compressed can impact how well AI upscaling works. If a video has been excessively compressed before upscaling, the input quality is reduced, making it harder for the AI to recover and enhance lost detail.
There's a lot of ongoing research aimed at not only refining image quality but also improving processing speed. This is crucial for broadening how we can utilize AI to make video streaming experiences even better. As this technology continues to develop, it will be interesting to see how the balance between quality and processing limitations evolves.
Telequality Video Player Integrating AI Upscaling for Enhanced Streaming Experience - Real-Time Frame Analysis for Dynamic Improvements
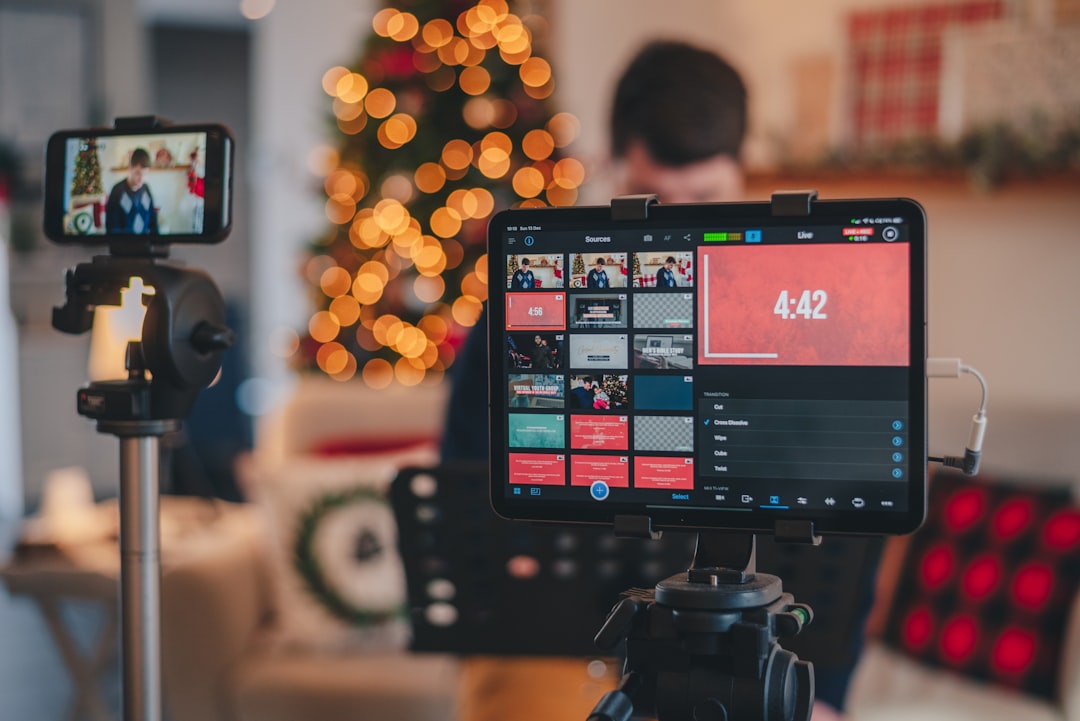
Real-time frame analysis is a crucial component in optimizing the dynamic aspects of video experiences. This approach, often powered by AI, involves continuously analyzing individual frames of a video stream. This continuous analysis allows for immediate adjustments to enhance video quality, adapt to changes in network conditions, and optimize the viewing experience. One important aspect is the ability to analyze viewer engagement and tailor content delivery in real-time. This can lead to a more personalized experience for viewers and more effective content strategies for creators. However, the need for balance between image quality improvements and the potential for creating visual distortions is a major factor to keep in mind. Real-time frame analysis is becoming more integrated into the field of streaming and, subsequently, its impact on the viewing experience will likely continue to evolve as this technology matures. This leads to questions about the implications for future video streaming and content consumption trends.
Real-time frame analysis is changing how we experience video, especially in dynamic environments like live streaming or surveillance. It's about making continuous adjustments to video quality based on what's happening in the moment, like fluctuating internet speeds. This is a significant shift from traditional techniques, which often process video once, leaving less room for real-time adaptation.
Interestingly, this approach can maintain a natural flow between video frames, preventing the jarring visual glitches sometimes seen with older methods. This temporal coherence makes for a more seamless and less disruptive viewing experience, a definite improvement.
One of the promising aspects is its potential to minimize streaming delays. By using predictive models, real-time frame analysis can anticipate what's needed for smoother delivery, which is crucial for anything from online gaming to video conferencing where timing is paramount.
What's also notable is its flexibility with hardware. This technology can leverage existing hardware efficiently, so even devices without top-of-the-line processors can handle advanced video improvements. This makes enhanced streaming more accessible compared to older techniques that frequently required more powerful hardware.
Furthermore, it offers the ability to customize video quality based on the type of content being watched. It can discern between, say, sports and movies and apply unique enhancements to each, resulting in a more tailored experience.
However, the original compression format used on the video can impact its effectiveness. Different compression methods can alter how well upscaling algorithms operate, reminding us that the entire video delivery chain matters.
Some systems also try to enhance video quality based on user behavior. For instance, if you switch to a larger screen, the video could automatically adjust to maintain sharpness without you having to fiddle with settings.
It's worth noting that real-time frame analysis is a great framework for ongoing improvements. As more video is processed, the system learns, making the enhancements continually better without needing constant manual tweaking.
It’s also not just for traditional video streaming. This technology has a lot of potential for mixed reality (MR) applications. It could enhance MR content in real time, potentially blurring the lines between the digital and physical worlds in things like gaming or training simulations.
One hurdle though is the lack of standardized ways to evaluate these systems. Without a consistent way to measure performance, comparing different approaches can be tough, which could potentially slow down the progress and development of the field.
Telequality Video Player Integrating AI Upscaling for Enhanced Streaming Experience - Bandwidth Optimization Without Sacrificing Quality
Optimizing bandwidth usage in video streaming is increasingly important as viewers demand higher quality content. AI techniques can enhance compression by identifying and removing redundancies that conventional methods often miss. This leads to better streaming without sacrificing visual clarity. However, striking the right balance can be tricky. The effectiveness of AI-based optimization often hinges on the initial video quality and the data rate it was encoded at. Moreover, viewer preferences are diverse, making it crucial for streaming systems to adapt to fluctuating network conditions while preserving the best possible video quality. Real-time video analysis has the potential to make high-quality streaming faster and more seamless, suggesting a promising future for how we consume video content. While these technologies offer great potential, it's important to remember that there can be trade-offs involved. It's crucial to continue evaluating these methods carefully to ensure that AI-driven optimization doesn't compromise other aspects of the streaming experience.
AI upscaling can be a tool for optimizing bandwidth without necessarily sacrificing the quality of the viewing experience. For instance, dynamically adjusting the frame rate based on the network connection can ensure smooth playback even when bandwidth is limited. This involves intelligently dropping frames while still keeping the video visually coherent.
Coupled with adaptive bitrate streaming, AI can make streaming more resilient to network fluctuations. By changing the bitrate dynamically, the system can switch between different quality levels, minimizing those annoying interruptions from buffering.
Interestingly, AI can go beyond just resolution improvements. It can also tackle the issue of compression artifacts. By analyzing the video's underlying patterns, AI can identify and try to minimize the effects of artifacts like blockiness or blurring, making the end result look better, even if the original video was heavily compressed.
The selection of a video codec plays a role in bandwidth optimization. While newer codecs like AV1 boast improved compression, combining them with AI upscaling could yield even greater bandwidth efficiencies and better viewing quality.
In the temporal domain, AI can refine how video information is spread across frames. Real-time frame analysis can not only upscale but also improve motion clarity. This is particularly important for genres with lots of rapid movement like sports broadcasts.
AI can incorporate frame interpolation, where new frames are generated from existing ones. This is a way to create smoother motion while lessening the perception of dropped frames, making the video appear better even if the actual frame rate isn't the highest.
Systems with advanced AI capabilities can adapt not just to network conditions but also user preferences. If a viewer prioritizes quality, the AI might adjust to use more bandwidth, maximizing clarity at the cost of slightly higher data consumption. On the other hand, bandwidth restrictions would drive the system towards prioritizing minimal buffering over ultimate sharpness.
It's also becoming clearer that different video content needs its own AI scaling approach. For action movies, the AI may focus on motion and sharpness, whereas for documentaries, the AI's task would be more about preserving fine detail and making up for any loss caused by compression.
AI-powered video streaming has the potential to learn and adapt over time. Systems can be trained on viewer interactions and content types, continuously refining their ability to optimize video delivery. This results in better viewing experiences as the system progressively gets better over time.
Despite its computational demands, AI upscaling is increasingly being optimized to work well on a wider variety of hardware. This means that users with less powerful devices can still enjoy enhanced streaming experiences without the need for expensive hardware upgrades. This is a positive development that could make high-quality streaming more accessible.
Telequality Video Player Integrating AI Upscaling for Enhanced Streaming Experience - Improved Performance on Low-Power Devices
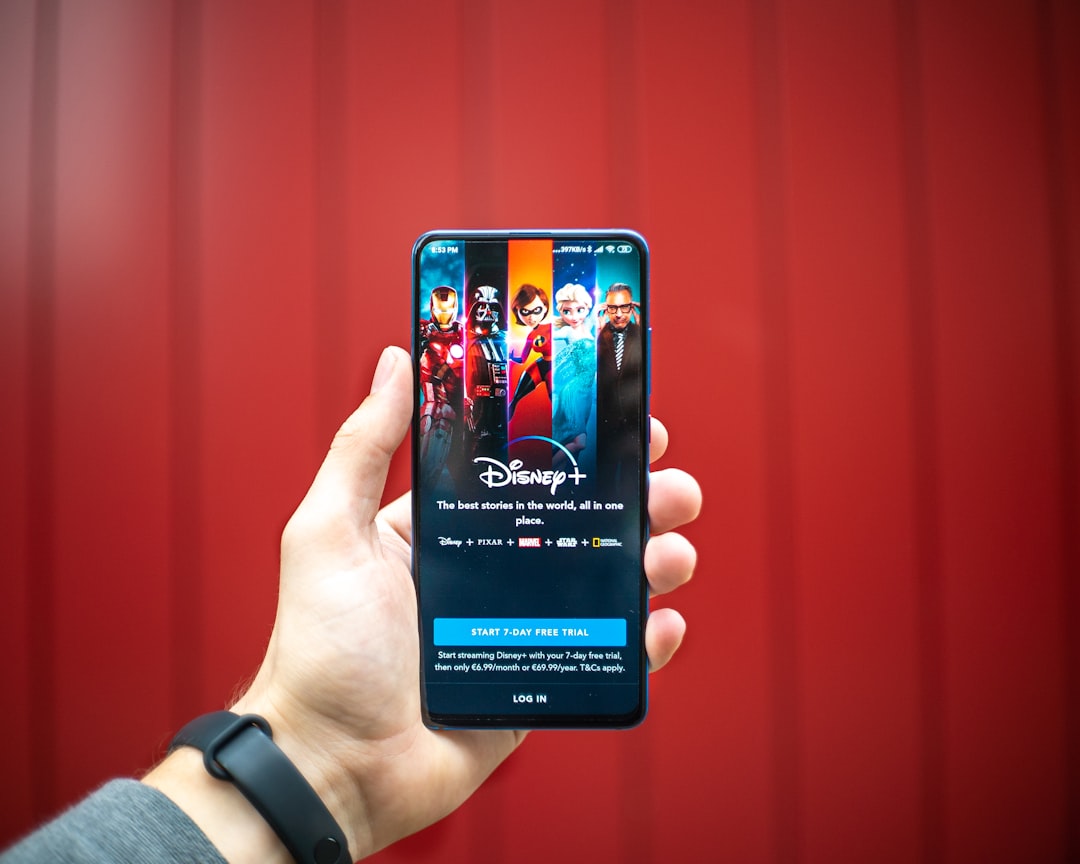
The Telequality video player's incorporation of AI upscaling is designed to enhance performance on devices with limited processing power, making high-quality streaming more widely available. These AI-driven methods refine video quality without demanding top-tier hardware, bridging the gap for those using older or less powerful devices. This is becoming increasingly relevant as viewers expect seamless streaming experiences. AI upscaling systems are continuously being refined to use resources effectively, leading to smoother playback and crisper visuals despite constraints on device power. The success of these optimizations, however, relies on the initial video quality and the limitations of the device itself, requiring ongoing assessments of their effectiveness and adaptability across a range of hardware. This ongoing development of AI upscaling signifies a shift towards more inclusive streaming experiences, where the quality of the video is not hampered by limitations of the viewer's device.
The integration of AI upscaling into video players is showing promise for improving the streaming experience on devices with limited processing power. These AI models are becoming increasingly adaptable, tailoring their operations to the capabilities of the specific hardware they're running on. This means devices with less powerful processors can still benefit from the upscaling process, as the AI adjusts its algorithms to avoid overloading system resources.
One notable aspect is the emphasis on efficient memory management. AI algorithms are now engineered to utilize techniques like memory compression, enabling the upscaling process without excessively taxing the device's resources. This is crucial for extending battery life on mobile devices or preventing performance hiccups on older computers. Additionally, leveraging GPU acceleration, where available, helps alleviate computational load on the main processor, further improving efficiency.
Furthermore, these systems strive to ensure output resolution consistency across varying video formats. This is important because devices with limited processing might struggle when handling fluctuations in quality from source content. By maintaining a consistent resolution, the video player reduces the processing burden, contributing to a smoother viewing experience.
Another aspect is the incorporation of streamlined compression techniques during the upscaling process. These techniques aim to reduce the data footprint of the video streams, optimizing bandwidth utilization on lower bandwidth connections. This is particularly advantageous for users on mobile networks or in areas with limited internet access. AI systems are also being fine-tuned to optimize video encoding for low-power devices, creating smaller, more manageable data packets that lead to smoother playback.
Additionally, AI upscaling can incorporate real-time adjustments to lower resolution or frame rates as needed, balancing quality with performance on devices with limited capabilities. This approach can prevent the device from being overwhelmed during particularly demanding scenes or when experiencing temporary drops in network connection.
Interestingly, some AI models are designed to learn user preferences over time, refining their performance on a given device. This personalized optimization could lead to a more tailored and ultimately improved streaming experience on low-power hardware. Another benefit for low-power devices is AI's growing ability to predict network conditions, allowing it to adjust video qualities in a way that minimizes streaming latency. This is especially vital for live content where delays can be detrimental to the viewing experience.
A further aspect is multi-resolution support. AI upscaling can allow a single video feed to be served at different resolutions concurrently, enabling low-power devices to receive a stream tailored to their capabilities without sacrificing overall quality or performance elsewhere.
While there's a lot of potential, it's important to remain mindful of the computational demands inherent in AI upscaling and the need to find optimal solutions for devices with varying levels of performance. The continuous refinement and optimization of AI upscaling algorithms is certainly an area to watch closely, as it could make high-quality video streaming more accessible across a wider range of devices.
Telequality Video Player Integrating AI Upscaling for Enhanced Streaming Experience - Versatile Solution for Live and On-Demand Content
The Telequality Video Player is designed to handle both live and on-demand video content effectively, making it a versatile tool for content creators and viewers alike. Its integration of AI upscaling aims to improve the overall streaming experience by enhancing video quality, especially when viewed on mobile devices where smooth, buffer-free playback is crucial. This player also simplifies the process of distributing and managing video content, potentially leading to more efficient workflows. The player's ability to adjust to different content formats suggests it can deliver a better viewing experience regardless of whether users are watching live streams or pre-recorded videos. However, the use of AI to enhance video naturally brings with it challenges related to potential visual artifacts and limitations on hardware processing power. Striking a balance between the benefits of AI upscaling and these inherent limitations is a critical factor to consider for optimal video streaming.
The Telequality Video Player is built to handle both live and on-demand content, making it a versatile tool for various streaming needs. It leverages AI upscaling to improve the overall viewing experience, especially for viewers with less than ideal network connections. The player is designed to provide smooth, buffer-free playback, especially on mobile devices, hoping to offer a consistent experience regardless of the platform.
One area of interest is how the AI within the player handles different types of content. For example, live sports and slower-paced documentaries will likely require different processing techniques to optimize the viewing experience. This dynamic adaptation suggests a level of intelligence in the system, adapting to the specific needs of various content types.
Reducing latency is another key aspect. Using predictive modeling via real-time frame analysis, the system aims to reduce delays during streaming, critical for interactive content like gaming or live sporting events. This capability offers exciting possibilities for those wanting real-time video communication and experiences.
In terms of video quality, the player strives to reduce those annoying compression artifacts that can affect clarity. By identifying and minimizing their effect, it can improve viewing pleasure even when dealing with heavily compressed video sources, potentially extending the lifespan of older or lower-quality content.
Furthermore, it can personalize the experience by adjusting video quality to suit individual viewers and the device they are using. Over time, the AI can learn about preferences and adapt, hopefully refining the experience without manual intervention. This adaptability extends to hardware capabilities as well, as the player aims to provide a high-quality stream on devices with limited processing power. This feature is notable given the varied devices used to access video content today.
The support for multi-resolution delivery is a strength, allowing the same content to be streamed at different resolutions. This caters to users with various internet speeds and device capabilities, allowing for greater inclusivity in streaming experiences. It also includes tools designed to manage memory usage effectively, potentially making the experience smoother on devices with limited memory, a common concern with high-quality video content.
Beyond this, the system tries to improve the temporal coherence, meaning it's capable of analyzing frame sequences, aiming for seamless transitions and reduction of distracting video glitches. While upscaling has been a focus, the system seems to go further in this context, aiming to address broader issues of continuity and visual quality.
While promising, questions remain about how the system handles various forms of compression and indexing to manage content, especially as it relates to viewer engagement metrics. Ongoing research into how the system performs in real-world conditions, and across varying types of content, will be needed to determine its overall impact and value in the video streaming landscape. With the ever-growing demand for higher-quality content, such tools are becoming more important.
Telequality Video Player Integrating AI Upscaling for Enhanced Streaming Experience - Machine Learning Techniques Reduce Playback Latency
Machine learning is increasingly being used to address a common problem in video streaming: playback latency. These techniques involve using adaptive algorithms to intelligently select the best bitrate for the current network conditions. This real-time optimization helps reduce the frustrating delays that can occur during streaming, leading to a smoother viewing experience. Furthermore, AI-driven video enhancement tools are being incorporated into the streaming process. These tools can improve the visual quality of video in real time, not only by upscaling the resolution but also by maintaining a smoother transition between frames. This is particularly important for live streams and other content where a seamless visual flow is crucial. The continued development of these AI technologies suggests a future where high-quality video experiences are readily accessible, even on less powerful devices. While this is a promising development, it's crucial that the performance of these machine learning techniques is continually assessed to ensure that they effectively meet the diverse needs of users and video content formats.
Machine learning is increasingly being used to improve the smoothness of video playback by predicting what's needed and adjusting accordingly in real-time. For instance, by anticipating which frames are about to be displayed, systems can proactively fetch that data, reducing the delays that can lead to noticeable lags, especially during live events like sports or online games. This predictive approach is crucial for maintaining a smooth, responsive viewing experience.
The effectiveness of these latency-reducing techniques often relies heavily on the analysis of large datasets. By studying past playback patterns and user behavior, AI models can learn to adjust to specific viewing environments, making the process of optimizing for low latency more precise. One aspect is dynamic buffering strategies. Instead of relying on a fixed buffer size, the AI can intelligently adjust it based on real-time network conditions, which is vital for maintaining a seamless experience, even when network speeds fluctuate.
Another area where machine learning plays a role is during the video encoding process. Algorithms can make changes on-the-fly, like adjusting the resolution or bitrate, to optimize quality while keeping latency low. Essentially, it's like a dynamic quality controller, ensuring that the video remains smooth without excessive delay. Some researchers have even developed algorithms specifically focused on minimizing latency. The idea is to prioritize the most important parts of the video stream, making sure viewers get the core visual information quickly, reducing the impact of delays.
It's also notable that these machine learning techniques can adapt to the specific capabilities of the device running the player. This is particularly important for users with less powerful devices that may not be able to handle the computational demands of complex video processing. By tailoring operations to the hardware limitations, the AI can still provide a low-latency playback experience, ensuring a broader audience can enjoy smooth streaming.
In addition, the insights from machine learning can help optimize the entire video delivery process. This includes determining the best network routes for data packets to travel, avoiding congestion points that cause delays. Further, incorporating feedback mechanisms allows these systems to continuously learn and improve over time. They can gather information about playback performance and user experience, making ongoing adjustments to refine their ability to minimize latency.
It's also interesting to see that AI can be trained to tailor the video processing approach to the type of content being viewed. For example, a fast-paced action movie will likely be handled differently compared to a documentary, adapting the processing strategy to best suit the particular content. This targeted approach is promising, as it suggests that future systems will be able to create not just a smooth streaming experience but one that's also more tailored to the viewer's expectations. The future of machine learning applications within video streaming seems likely to continue to emphasize adaptive adjustments in order to optimize the viewer's experience, though challenges associated with device limitations and ensuring consistent quality will likely remain an active area of research.
More Posts from ai-videoupscale.com: