Upscale any video of any resolution to 4K with AI. (Get started for free)
AI-Enhanced Video Conversion Exploring the Intersection of Machine Learning and Format Transformation in 2024
AI-Enhanced Video Conversion Exploring the Intersection of Machine Learning and Format Transformation in 2024 - AI Transcoding Revolutionizes Video Format Conversion
AI transcoding is revolutionizing how we convert video formats. It's no longer just about switching file types; AI uses machine learning to fine-tune videos for optimal viewing on a wide range of devices. This means better quality and a smoother experience, especially when videos are meant for diverse platforms. The core of AI transcoding relies on neural networks, allowing for more sophisticated optimization than traditional methods. This approach is particularly useful in cloud-based transcoding services, where AI can enhance image clarity and overall video quality without significant manual intervention. The implications are vast, as it's shifting the landscape of video production and distribution in ways akin to the internet's impact on the field. This suggests that AI transcoding might unlock new avenues for creative expression and technical exploration in the video space. However, it's worth noting that while this technology holds great potential, its widespread adoption and impact on the field are still unfolding.
AI transcoding has become a fascinating field, leveraging machine learning to intelligently adjust video compression and formatting. We're seeing algorithms dynamically adapt to network conditions, for instance, adjusting compression in real time to ensure a smooth streaming experience without user intervention. Deep learning models are also improving bit rate management, with some systems achieving impressive reductions in file size – up to 50% – while preserving image quality, a feat difficult for traditional methods.
Beyond simple format conversions, AI is tackling more nuanced challenges. These systems are able to automatically analyze and adjust color discrepancies between different formats, which ensures that the visual integrity is consistent regardless of the original content’s characteristics. Further, recent work on frame interpolation using AI allows us to smoothly convert video to formats with higher frame rates, avoiding unnatural, jerky motion.
This progress is tied to the sophistication of the algorithms. For example, “content-aware” algorithms prioritize important details in complex scenes, meaning the fine textures and edges that make up the richness of a scene are preserved with higher fidelity. There's even research exploring how these systems can predict viewer behavior to tailor the encoding process, which could have implications for improving audience engagement metrics.
Interestingly, some systems are hybridizing traditional compression techniques with machine learning to dynamically optimize settings based on the target device, further improving compatibility and access across the vast array of devices used today. Generative models are also being investigated as an intermediate step in transcoding, potentially smoothing the process of converting between vastly different video resolutions or qualities. We see applications of this emerging in areas like augmented and virtual reality, where low-latency and high-quality are crucial.
The future of AI-enhanced transcoding looks tied to cloud computing. By leveraging cloud infrastructures, we can scale processing capabilities dynamically based on media traffic. This is enabling us to deliver video experiences with lower latency, a crucial requirement for global access to online media. The evolution of AI in video conversion is quite profound, and while the potential applications seem vast, there is a need for continued research and testing to ensure quality and efficiency of these systems.
AI-Enhanced Video Conversion Exploring the Intersection of Machine Learning and Format Transformation in 2024 - Neural Networks Enhance Video Processing Efficiency
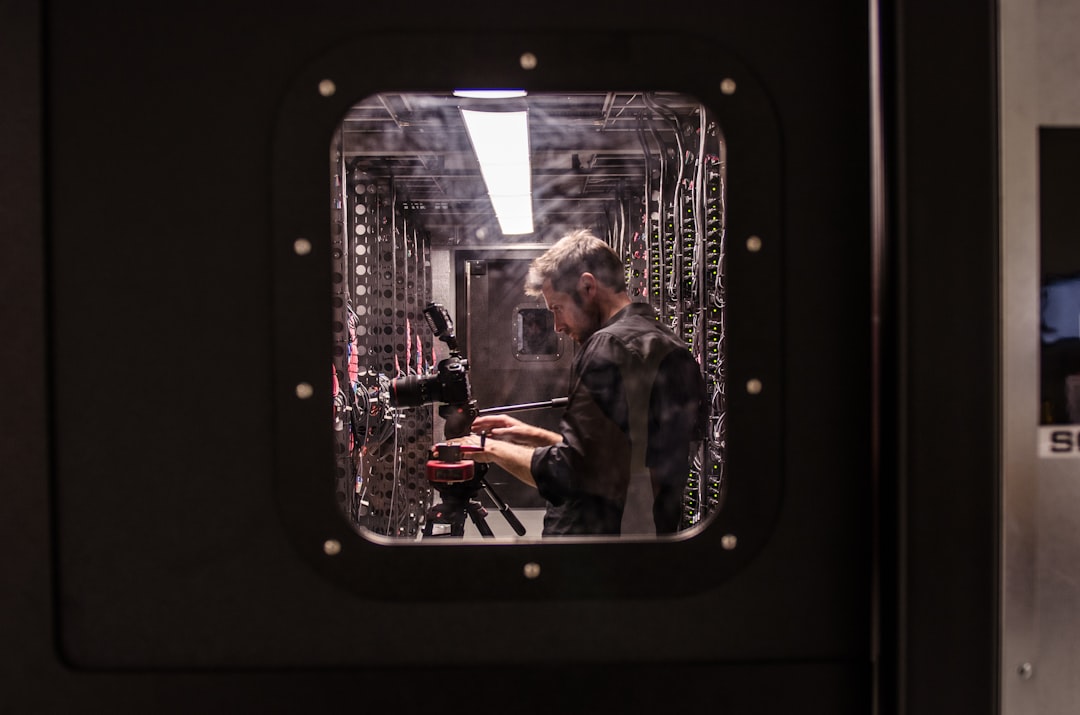
Neural networks are proving crucial in improving the efficiency of video processing, especially within the newer video coding standards like HEVC and VVC. They achieve this by refining various stages of the encoding process, such as how frames are predicted and quantized. This optimization allows for better compression without noticeable loss of visual quality. As we deal with increasingly higher-resolution and larger volumes of video data, the use of deep learning techniques has become increasingly important. They offer ways to speed up the processing involved in video handling, including compression and display. Furthermore, neural networks play a role in a newer field known as intelligent video coding, where the encoding process incorporates a deeper understanding of the video's content. This intelligent encoding produces more compact representations of the visual information, potentially leading to better overall video quality. It's clear that deep learning and traditional video compression methods are working together in a new way, signifying a significant shift in how video is encoded and shared. However, there's a clear need for continued research and development to fully realize the potential of this approach, as it's a fast-changing field.
Neural networks are increasingly being integrated into video processing pipelines, particularly for tasks like compression and format conversion, offering several potential advantages over traditional methods. One of the most promising aspects is the potential for significantly faster processing speeds. In some instances, neural networks have demonstrated real-time transcoding capabilities, converting video formats in seconds—a stark improvement over the minutes or even longer it can take using conventional approaches.
Maintaining high visual fidelity during compression is another area where neural networks show potential. Research indicates that they can achieve higher compression rates while maintaining comparable, if not superior, image quality when compared to traditional techniques. This is especially relevant as video resolutions and file sizes continue to grow. Interestingly, these systems can also learn from the videos they process, effectively refining their encoding strategies over time. This adaptive learning allows for continuous improvement in both the efficiency and output quality of the process.
Further, neural networks are capable of performing more in-depth scene analysis, helping to preserve subtle details that might otherwise be lost in the compression process. This is especially important for intricate scenes with complex textures and fine details, which are crucial for maintaining the richness of video content. Furthermore, they're being explored for dynamic bitrate adjustment, where the bitrate is altered during playback based on real-time factors such as network conditions and user behavior. This can provide a more seamless viewing experience while also optimizing bandwidth utilization.
The ability to perform sophisticated inter-frame prediction is another strength of these systems. By intelligently estimating the changes between frames, they can effectively reduce data usage without compromising the fluidity of motion, making them well-suited for high frame rate content. We also see the emergence of techniques that leverage generative models in the transcoding process. This offers a smoother pathway for converting between vastly different video qualities or resolutions, which is crucial for applications like live broadcasts and interactive gaming where seamless transitions are essential.
Additionally, neural networks can help automate tasks like color calibration, ensuring consistency across different video formats. This is a significant benefit for situations where videos from multiple sources need to be integrated, such as during live events or collaborative projects. Error correction mechanisms are also being implemented, enabling the systems to identify and rectify distortions or artifacts in real-time. Finally, the integration with cloud-based systems enhances scalability, allowing for flexible processing capabilities that can handle varying media traffic, contributing to a seamless experience for users across platforms and devices.
While these advancements are exciting, it's worth noting that many of these applications are still in their early stages. Further research and development are necessary to fully understand the capabilities and limitations of these systems, especially in terms of ensuring long-term reliability and output quality. The intersection of neural networks and video processing is a rapidly evolving field with the potential to redefine how we capture, process, and experience video content.
AI-Enhanced Video Conversion Exploring the Intersection of Machine Learning and Format Transformation in 2024 - Enhanced Video Coding Project Explores AI-Driven Codec Improvements
The Enhanced Video Coding (EVC) project, led by the Moving Picture, Audio and Data Coding (MPAI) group, is exploring the use of artificial intelligence to improve existing video codecs. The core idea is to enhance the performance of traditional codecs, including the MPEG5 EVC, by incorporating AI-based tools into the encoding process. This means potentially replacing or improving how elements like motion compensation and quantization are handled. The ultimate goal is to create more efficient compression methods while preserving, or even improving, the overall quality of the video.
Further, there's a growing interest in developing new standards that take full advantage of AI's capabilities. The MPAI's exploration of End-to-End Video Coding (EEV) represents a shift towards using fully neural network-based systems to handle video encoding. This ambitious approach offers a unique opportunity to optimize the entire coding process, but also presents challenges in terms of standardization and implementation.
While the use of AI in video compression shows significant potential for improved efficiency and quality, the field is still in its early stages. There are ongoing challenges related to reliability, ensuring optimal performance across diverse hardware and software, and addressing concerns about potential biases or inaccuracies introduced by the AI systems themselves. Despite these hurdles, the potential for AI-driven codec improvements is substantial, and continued research and collaboration within the standardization community will be crucial in shaping the future of video compression technology.
The integration of AI into video coding, particularly within the MPAI's Enhanced Video Coding (EVC) project, is leading to exciting developments. They are trying to improve existing codecs like MPEG5 EVC by swapping out or supplementing current tools with AI-powered equivalents. This approach, often referred to as AI-Enhanced Video Coding (MPAI-EVC), reflects a broader interest in Intelligent Video Coding (IVC), a field that’s been around since the 80s and involves using video content understanding to create more efficient representations of visual data. The Evidence project under the MPAI is another example of this trend. They are exploring the use of neural networks to potentially replace or refine existing video coding components.
It seems that there's a growing movement to fully embrace neural network-based solutions, as evidenced by MPAI’s exploration of End-to-End Video Coding (EEV) standards. AIVC (Artificial Intelligence-based Video Codec), one specific example, is a fully neural network video codec. Its two conditional autoencoders handle motion compensation and coding, optimizing the compression process using a single rate-distortion optimization loop. This single-process architecture is a departure from traditional video coding methods. It's interesting that this shift towards neural networks in video encoding isn't just a minor tweak, but reflects a broader trend toward more sophisticated AI in video compression.
The fusion of machine learning and format conversion in video coding is certainly an active area. It seems like it has the potential to lead to a significant improvement in both compression efficiency and video quality. The standardization work done by the MPAI is critical to guide the development and use of these new technologies, especially when it comes to AI and machine learning-influenced approaches. However, one might wonder how the adoption of AI-driven codecs will impact the existing landscape, particularly the ecosystem of established standards and implementations. The challenges ahead will likely include ensuring compatibility with existing hardware and software and validating the long-term stability of these AI-driven systems, as the field is rapidly evolving. While exciting, the implementation of these cutting-edge technologies will require careful research and validation to ensure that the benefits of AI-driven video compression are realized without sacrificing the quality and reliability of the video stream.
AI-Enhanced Video Conversion Exploring the Intersection of Machine Learning and Format Transformation in 2024 - Machine Learning Algorithms Optimize Video Files for Multi-Platform Compatibility
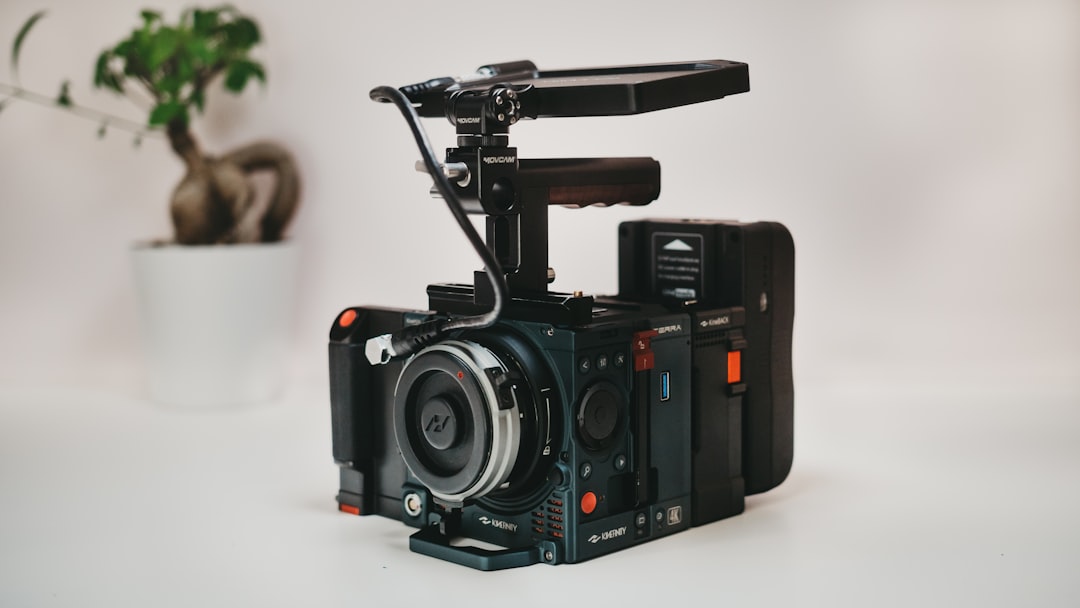
The increasing variety of devices and streaming platforms used to watch videos has made multi-platform compatibility a major concern. Machine learning algorithms are now being used to optimize video files, tackling the challenges that arise when a video needs to work well on a wide range of devices. These algorithms are able to examine video content and make intelligent choices about how to compress the data to ensure minimal loss of quality.
We're seeing this play out in new video compression techniques like H.265, VP9, and AV1, which are able to drastically reduce file size without causing a noticeable drop in video quality. Moreover, the use of deep learning within these algorithms lets them identify and remove redundant information in ways traditional methods couldn't, resulting in more efficient compression. This is especially important as the demand for high-quality video streaming continues to grow, creating a need for ways to transmit those videos effectively even over limited bandwidth connections. Machine learning seems to be poised to become a crucial tool in addressing this challenge, helping to make video formats more versatile and easily accessible across devices. There are still questions and challenges as this technology becomes more widely adopted. It will be interesting to see how it changes video distribution and consumption in the coming years.
Machine learning is increasingly woven into the fabric of video optimization, particularly for achieving seamless compatibility across a wide range of devices. These algorithms are able to dynamically analyze video content and intelligently adjust compression levels in real-time based on factors like network conditions. This dynamic adaptation is a significant departure from conventional static compression methods, promising a more robust and responsive viewing experience.
Interestingly, these systems are also becoming more 'content-aware.' They can distinguish between different aspects within a single frame, applying higher compression ratios to less important areas while prioritizing visual fidelity in critical regions. This granular approach leads to a substantial improvement in both file size and perceived quality. It's fascinating how these models are starting to explore connections between video encoding and viewer behavior, even attempting to predict which portions of a video will be most engaged with by the audience. This raises intriguing possibilities for optimizing encoding based on likely viewing patterns.
Another area of recent advancement is frame rate interpolation. By leveraging machine learning to estimate the motion between frames, videos can be effectively upscaled to higher frame rates, leading to a much smoother and less choppy playback experience, something which has previously been challenging to achieve. Furthermore, the systems can now perform dynamic error correction, essentially acting as a continuous quality control mechanism to identify and repair any distortions or artifacts that emerge during the compression and transcoding processes.
Generative models are also beginning to play a larger role, particularly in upscaling low-resolution content to higher resolutions. This process allows for seamless conversions to higher qualities and opens up possibilities for enhancing the viewing experience in real-time applications like live streaming. We're also seeing the emergence of hybrid approaches, combining traditional video coding techniques with machine learning-driven strategies. These hybrid systems offer a more flexible and fine-grained level of control over the transcoding process, which helps optimize for different device types and playback environments.
It's notable that machine learning is now being used to automate processes like color calibration across diverse video formats, thus ensuring visual consistency regardless of the content's origin or playback device. This is a substantial improvement over manual techniques. The ability of these algorithms to optimize bitrate management is quite noteworthy. They can achieve remarkably efficient compression, in some cases reducing file size by up to 50% while maintaining comparable quality. This is crucial in today's environment of growing video consumption and bandwidth limitations. These advantages are further enhanced by integrating machine learning with cloud-based processing, which allows video transcoding systems to scale dynamically based on user demands and network traffic, improving the overall experience, especially for global audiences.
While these developments are undoubtedly encouraging, there's a constant need for careful evaluation of these systems. Reliability, long-term stability, and the potential for unforeseen biases introduced by the AI components are all areas requiring continuous investigation. However, given the advancements we're seeing, it's clear that AI is significantly reshaping video optimization and compatibility, paving the way for more efficient and accessible viewing experiences.
AI-Enhanced Video Conversion Exploring the Intersection of Machine Learning and Format Transformation in 2024 - AI Integration Accelerates Video Production Workflows
AI is rapidly changing how videos are made, streamlining workflows and making production more efficient. AI can now automate a lot of the repetitive parts of video creation, like editing and adjusting colors, which helps speed up the entire process and cut costs. This lets video professionals concentrate on the creative parts of their work. Beyond that, AI can analyze the content of videos, which can be used to personalize the experience for viewers, keeping them engaged. AI can even help with editing by recognizing elements within the video, like objects and scenes, which moves the process more toward automation from the traditionally very manual tasks. This trend of AI-powered tools is altering the landscape of video production in a way that’s comparable to how the internet completely reshaped creative industries. While there are promising advances, it remains to be seen how these technological shifts will fully unfold and impact the creative processes and the video production industry in general.
The integration of AI, specifically machine learning, is significantly accelerating various aspects of video production workflows. It's becoming increasingly apparent that these systems can automate repetitive tasks like editing, color correction, and sound mixing, which traditionally have consumed a considerable amount of time and resources. While the potential for automation is noteworthy, a more interesting aspect is how AI allows for a deeper analysis of video content. Algorithms can distinguish between different types of scenes—like action-packed moments versus still shots—and apply specific processing techniques based on what they're seeing. This tailored approach has led to considerable gains in efficiency, though we're still early in understanding the full impact.
One of the most visible applications of AI in video production is its ability to adapt to varying network conditions. Systems can dynamically adjust video quality on the fly to ensure smooth playback, even when network bandwidth is limited. This dynamic approach is particularly helpful for live broadcasts, where the potential for disruptions is greater. Furthermore, it seems AI-based encoding approaches are leading to improvements in visual fidelity. It's possible to achieve comparable or even higher image quality with smaller file sizes compared to traditional methods. This is a significant benefit given the ever-increasing demand for high-quality video streaming, especially as network conditions can be inconsistent.
Another benefit relates to motion compensation, a crucial component in any video encoding process. AI is allowing for more accurate prediction of movement between frames, which in turn leads to smoother playback. This is especially relevant in action-packed sequences, where maintaining clarity and reducing visual artifacts is critical. The ability to intelligently analyze individual scenes, particularly in high-motion segments, is another way AI improves the workflow. Machine learning models seem capable of prioritizing the preservation of crucial elements within a scene, meaning that the most critical details are more likely to remain sharp during encoding, a task that has traditionally been challenging.
We're also witnessing the integration of error correction mechanisms into these workflows. AI-powered systems can detect and fix distortions in real time during the conversion or transcoding stages, which reduces visual artifacts that can mar a video viewing experience. There's also a growing use of hybrid encoding techniques, blending traditional compression strategies with AI-driven approaches. This seems to offer more fine-grained control over the entire transcoding process, resulting in greater compatibility with the multitude of devices used to view video. This is further boosted by the rise of generative AI models, which can efficiently upscale lower-resolution video content to higher resolutions with minimal compromise in quality.
Perhaps the most intriguing aspect of this trend is the emerging ability for some AI systems to predict viewer behavior. It seems these systems can analyze content and make inferences about how viewers might interact with a video, offering the potential to optimize encoding in a way that better matches viewing patterns. It's an area that requires further research and study, but it suggests the potential for video content to be dynamically tailored to individual audiences in a way that isn't possible with traditional methods. While these advancements are incredibly promising, it's important to acknowledge that the integration of AI into video workflows is still an evolving field. It will be crucial to continue researching the reliability, robustness, and potential biases these systems may introduce to the broader video production ecosystem. Overall, the use of AI in video production is reshaping how we create, optimize, and distribute video content, suggesting a future where video experiences are more personalized, accessible, and efficient.
AI-Enhanced Video Conversion Exploring the Intersection of Machine Learning and Format Transformation in 2024 - Generative AI Expands Accessibility in Video Synthesis and Modification
Generative AI is rapidly expanding the ease with which videos can be created and manipulated, opening up video production to a broader range of individuals. AI models, like those developed by OpenAI and Google, can generate complex and high-quality video content simply from text descriptions. This shift makes video creation more accessible, empowering individuals who may not have traditional filmmaking skills or resources. This democratization of video production not only encourages creativity but also potentially addresses issues of inclusivity by providing tools for more diverse voices to be heard in the digital space. The potential to integrate generative AI with language models could significantly improve the quality and capabilities of video generation, ultimately leading to richer, more engaging, and widely accessible video experiences. However, with such power comes the responsibility to use these tools ethically, raising concerns about potential misuse and the need for careful consideration of the impact on video content and its creators.
The field of generative AI is significantly expanding the accessibility and capabilities of video synthesis and modification. Tools like OpenAI's Sora, which can generate detailed, high-definition videos from simple text prompts, are lowering the barrier to entry for video creation. It's quite remarkable that complex scenes can be rendered from just a few sentences, something previously limited to professionals with specialized software and expertise. Similarly, Google DeepMind's Veo model demonstrates the growing ability to produce longer video content from text instructions, suggesting further advancements in this relatively new area.
The fusion of generative AI with large language models (LLMs) is transforming the landscape. It’s becoming more apparent that AI isn't just about generating new content, but is also enhancing our understanding and interaction with existing video content. This means a future where video can be more personalized to viewers, based on their preferences or viewing patterns. For instance, an AI system could dynamically adjust the video's quality based on network conditions, or it could modify aspects of a video to evoke specific emotions in the viewer. This could have a profound impact on the future of storytelling.
One of the most interesting aspects of this evolution is the increased focus on accessibility. By providing easier pathways for creating and modifying video content, generative AI is empowering a wider range of individuals to participate in the field. This aligns with broader efforts to increase inclusivity within digital media, allowing more diverse voices and perspectives to be shared. We’re also seeing innovative applications in areas like education, where generative models can be used to create customized learning materials, a notable application given the increasing use of video for learning across many disciplines.
However, the rapid advancement of generative AI in video production raises a number of important considerations. As these tools become more powerful, it becomes crucial to examine the ethical implications of their use. How do we ensure that this technology is used responsibly, and that its outputs don't perpetuate harmful biases or stereotypes? These are important questions, and will likely become more crucial as the capabilities of generative AI expand. There's also the question of copyright and ownership of content created using these tools.
Overall, the current trend indicates a growing interest in developing general-purpose AI tools that simulate the physical world with remarkable realism. These models can generate a variety of video content, from realistic simulations of natural events to fantastical imagery. Further, this evolution towards realism is apparent in the development of technologies like EMU VIDEO and Genie, which show the power of merging cutting-edge technology with the creative process. It remains to be seen how these capabilities will shape the future of video production and storytelling, but the advancements are quite remarkable.
There are also open questions on how this increased level of realism will affect the perception of authenticity and truth. As the boundaries between AI-generated and real-world content blur, it is becoming increasingly important to establish ways for audiences to distinguish between them. Developing effective strategies for identifying and labeling AI-generated content will become crucial to maintaining trust in online information.
It’s clear that the potential of generative AI in video is enormous, but it's a technology that requires thoughtful exploration and development. The ongoing advancements in generative video technology are shaping the future of video production and consumption. Balancing innovation with responsibility will be key to realizing the full potential of this fascinating technology, while mitigating any potential drawbacks.
Upscale any video of any resolution to 4K with AI. (Get started for free)
More Posts from ai-videoupscale.com: