Upscale any video of any resolution to 4K with AI. (Get started for free)
Video Converter Software in 2024 Balancing Efficiency and Quality for AI Upscaling Workflows
Video Converter Software in 2024 Balancing Efficiency and Quality for AI Upscaling Workflows - AI-Driven Resolution Enhancement for SD to 4K Conversion
The ability of AI to enhance video resolution, particularly converting standard definition (SD) to 4K, is a remarkable advancement in 2024. Software solutions are increasingly diverse, offering options that range from simple to complex, matching the skill levels of varied users. Some applications, like those found in VideoProc Converter or Topaz, demonstrate impressive leaps in quality, yet their features and interfaces differ greatly. This contrast is noticeable, with some options tailored to beginners seeking straightforward enhancements, as offered by AVCLabs or Mediaio. These focus on automated processes to minimize the need for manual adjustments while maintaining quality. Others, such as CyberLink's offering, employ sophisticated multi-frame methods, prioritizing lossless output, ideal for scenarios where the highest possible fidelity is required. The burgeoning landscape of AI upscaling tools compels users to strike a balance between operational ease and the quest for superior quality in their video projects. While some advancements may be perceived as revolutionary, they still present users with a spectrum of choices based on their skillsets and desired level of control in optimizing their workflows. It's a dynamic space where the pursuit of video enhancement through AI continues to unfold.
In the realm of video conversion software in 2024, a number of tools are leveraging AI for upscaling, specifically targeting the conversion of standard definition (SD) footage to 4K resolution. VideoProc Converter AI stands out for its effectiveness in improving the quality of older, lower-resolution videos, particularly for upscaling to 4K. For those seeking more advanced control, Topaz Video Enhance AI provides detailed features and is ideal for upscaling higher-resolution content. AVCLabs Video Enhancer AI offers a user-friendly approach, perfect for beginners who want simple resolution enhancement up to 8K without the worry of significant quality loss.
Mediaio's Video Enhancer employs advanced algorithms to analyze video pixels, filling in missing detail to achieve a higher-quality, sharper 4K output. CyberLink's approach relies on multiframe superresolution technology, offering an automated solution to convert SD to 4K or even 8K while keeping the final output uncompressed, ensuring optimal quality. Neurallove's AI upscaler is specifically designed to enhance low-resolution videos into clearer 4K content, tackling artifacts that can arise from the upscaling process.
DaVinci Resolve 18, known for its professional-grade color correction and audio tools, incorporates AI-based video upscaling into its feature set. Interestingly, Vmake AI Video Enhancer provides an online solution for 4K upscaling, capable of processing up to three files at a time without needing to create an account. Finally, Movavi's latest version integrates an AI upscaling function, effectively rejuvenating older, lower-quality videos by enhancing their resolution and overall video quality.
While there's a breadth of options available, it's worth noting that the performance of AI upscaling varies significantly depending on the original footage. Factors like frame rate, compression artifacts, and motion blur inherent in SD video can influence the success of the upscaling process. Researchers are actively working on overcoming such challenges, particularly in automating the correction of motion inconsistencies, aiming to eliminate judder or smearing often encountered with fast-moving scenes in upscaled content.
Video Converter Software in 2024 Balancing Efficiency and Quality for AI Upscaling Workflows - Balancing Processing Speed and Output Quality in Video Conversion
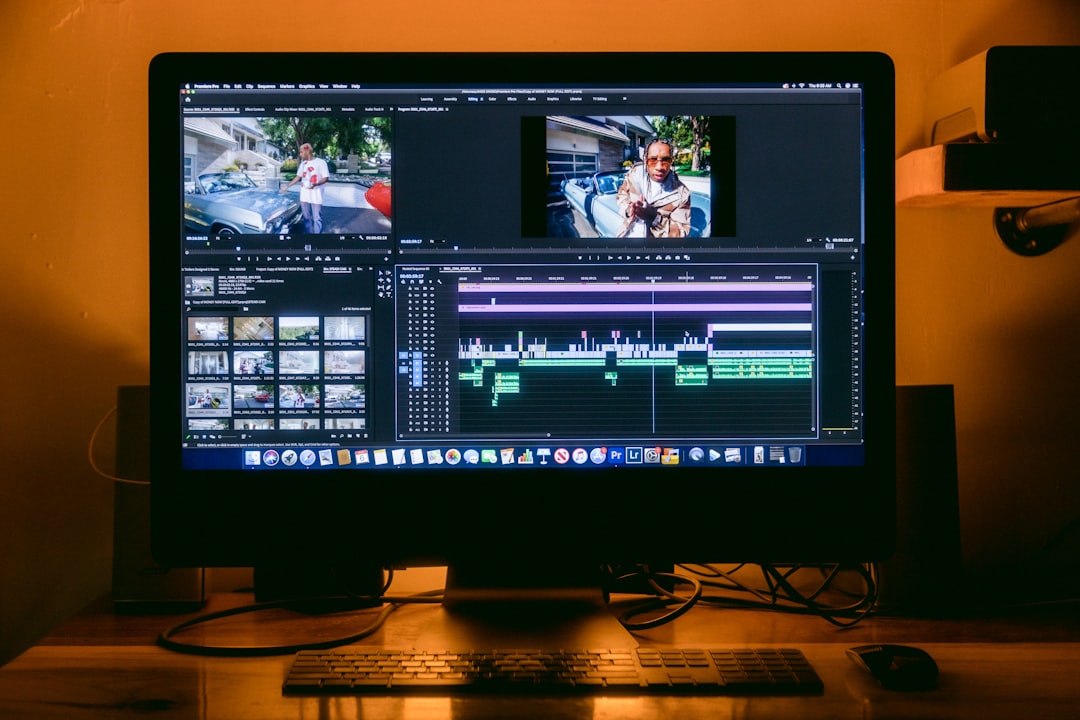
In the current landscape of video conversion software, the tension between achieving rapid processing speeds and preserving high-quality output continues to shape the user experience in 2024. AI-powered upscaling, while offering impressive improvements in resolution, introduces a new layer of complexity when it comes to balancing these two vital aspects. The ability to fine-tune settings like resolution, bitrate, and frame rate allows for greater control over the final product, enabling users to prioritize either quick processing times or exceptional image clarity. Tools such as Topaz exemplify the pursuit of unparalleled visual fidelity, often at the cost of increased processing durations. Meanwhile, other software solutions, including those found in some online platforms or simpler converters, may lean towards faster output, occasionally sacrificing sharpness or detail in the process. This dynamic compels users to make conscious choices based on their specific needs, be it rapidly converting videos for immediate use or taking the time to optimize for maximum visual quality. The future of video conversion hinges on the continuing refinement of algorithms and software design to navigate this ongoing trade-off between speed and output integrity.
In the evolving landscape of video conversion software in 2024, the ability to balance processing speed and output quality during AI-driven upscaling is a central concern. The algorithms underpinning these upscaling features, often reliant on deep learning networks, significantly influence the time it takes to convert a video. While simpler upscaling techniques can achieve results in seconds, more complex neural networks might require several minutes, depending on the desired level of detail. This raises a key challenge: how do we achieve high-quality output without sacrificing speed?
One of the trade-offs inherent in AI upscaling is that faster processing can sometimes lead to a sacrifice in detail. Optimizing for speed may involve simplifying certain aspects of the enhancement, potentially sacrificing the fine details of textures and edges that can heavily influence a viewer's perception. This presents a constant challenge for developers: How do they create a balance that meets the differing needs of various workflows?
Furthermore, the quality of the original video significantly impacts how efficiently upscaling can be performed. Highly compressed videos can introduce artifacts that are difficult for even advanced upscaling methods to eliminate entirely. While current algorithms can do a good job of reducing those artifacts and achieving clarity, this often comes at the cost of increased processing times as the system takes more time to analyze and correct the problematic areas.
Frame rates, too, factor into this equation. Higher frame rates generally lead to smoother motion but contribute to increased computational load, thus further complicating the balancing act between quality and speed. The intricate relationship between frame rate and upscaling quality is an ongoing research topic.
To address these challenges, some developers have implemented real-time processing. This lets users see the changes immediately, but often this requires a simplified upscaling algorithm, which might result in less detail. A similar trade-off exists with more advanced machine learning models which often provide better results, but at the cost of significant computing power, resulting in slower conversion times.
Utilizing GPUs has significantly improved conversion speeds. When integrated well, this can produce substantially faster upscaling compared to CPU-only processing. However, it's crucial to ensure the GPU is capable of handling the workload efficiently to prevent bottlenecks.
The user interface itself adds another dimension to this balance. Many converters offer both automated and manual options. While this allows technical users more granular control over their projects, it can also introduce complexity that may overwhelm less experienced individuals.
Evaluating output quality is another challenge. Standard metrics, like PSNR and SSIM, provide useful technical insights but don't always correlate with subjective perception. This disconnect between technical measures and human preferences creates further complications when aiming for the "right" level of detail at the "right" processing speed.
Looking forward, research continues into video codec optimization and accelerated processing algorithms to achieve high-quality upscaling without the inherent performance costs. The advancements in deep learning strategies show promise in enhancing this delicate balance. But, how long will it take for the perfect balance to be attained is still unknown.
Video Converter Software in 2024 Balancing Efficiency and Quality for AI Upscaling Workflows - Integrating AI Upscaling with Traditional Video Editing Tools
The integration of AI upscaling into the workflow of traditional video editing tools presents a compelling yet nuanced opportunity for video creators in 2024. A growing number of software programs are specifically designed to bridge the gap, allowing users to leverage AI for enhancing video resolution within their familiar editing environments. This integration offers potential for streamlining workflows and achieving a higher standard of output. However, the uneven performance of these AI tools across various video types and editing platforms can create friction. Users must carefully consider how these AI features integrate with their existing processes, as they can introduce computational burdens that may not always align with the real-time demands of editing tasks. This dynamic intersection of AI with established video editing methods pushes the envelope of what's possible in video production, prompting users to assess each tool's strengths and weaknesses in relation to their unique project needs. The journey to seamless and effective integration continues, requiring a delicate balance between utilizing AI's capabilities and maintaining the familiar aspects of traditional video workflows.
The merging of AI upscaling with established video editing software is a noteworthy shift, where AI algorithms predict missing pixel information by analyzing surrounding data. This creates upscaled visuals that would be difficult for manual editors to achieve.
Sophisticated AI upscaling relies on convolutional neural networks, gaining efficiency by learning from numerous sample videos. These networks extrapolate high-frequency details, resulting in crisper outputs than standard editing approaches.
However, the computational demands of these AI-trained upscalers vary. Neural networks used for upscaling often consume considerable computing power, extending processing from a few seconds to several minutes, particularly for high-definition conversions using more complex algorithms.
Traditional editing tools focus mainly on enhancing colors and effects. AI upscaling methods prioritize spatial and temporal consistency, meaning AI is better at maintaining a video's movement during upscaling, mitigating the blurring often seen in rapid frame transitions.
Integration with tools like DaVinci Resolve demonstrates the growing importance of AI upscaling in professional workflows. This challenges the long-held belief that manual editing expertise will always be the primary driver of video quality.
One ongoing issue is addressing compression artifacts in upscaling. While AI has shown improvement in minimizing these flaws, the quality of the initial video material directly influences how well these algorithms work. Poor source material still translates to suboptimal results.
Assessing output quality is another challenge. Traditional metrics like PSNR may not fully reflect how viewers perceive the video. This means that while AI may boost the numerical scores, viewer experience varies, making it hard to accurately gauge the success of video enhancements.
As software incorporates AI, user interfaces need to adapt. Programs with advanced AI features can overwhelm those unfamiliar with the technology. Therefore, clear, guided workflows are crucial to ease users between automation and manual control.
Some AI upscaling tools offer real-time processing, letting users see the changes as they occur. But often this comes at the cost of detail. Developers face a balancing act—how to keep both user interactivity and processing precision in their tools.
The adoption of GPU acceleration has revolutionized efficient AI upscaling, taking the load off CPUs and achieving faster conversion times. However, this introduces questions about hardware accessibility, specifically for casual users who may only have standard processors.
Video Converter Software in 2024 Balancing Efficiency and Quality for AI Upscaling Workflows - Addressing Motion Blur and Artifact Removal in AI Video Processing
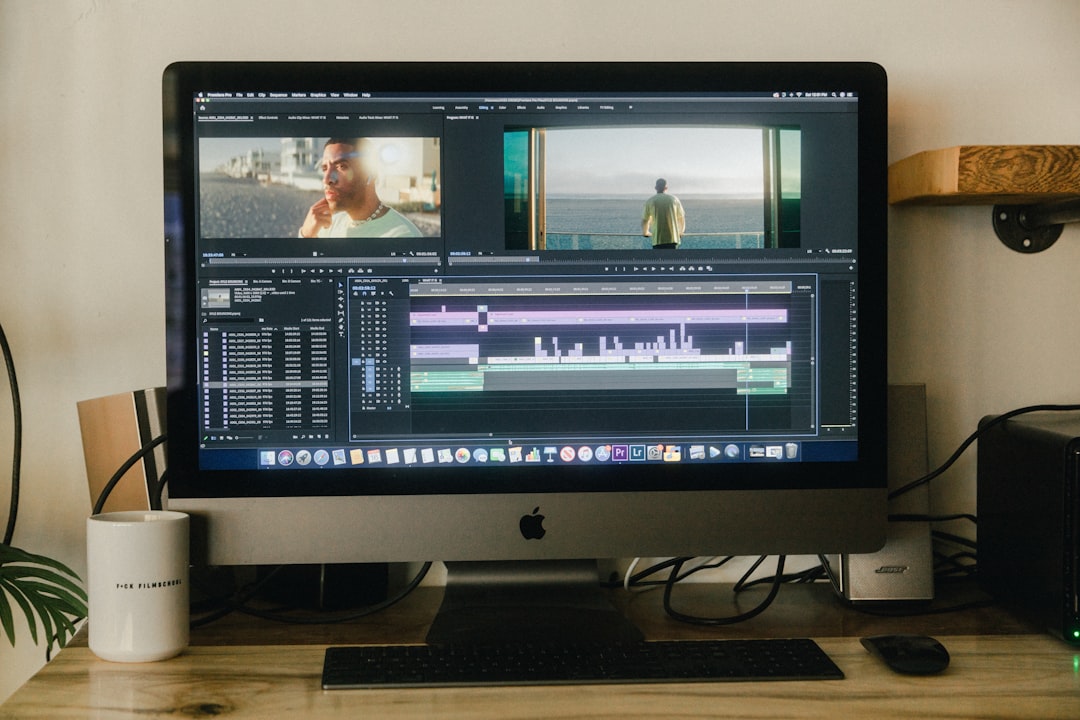
In the realm of AI video processing, particularly in 2024's landscape of video upscaling software, motion blur and artifact reduction are prominent challenges. AI-driven tools strive to address these issues by employing sophisticated algorithms for stabilization and deblurring, aiming for a cleaner and sharper final video. Programs like Topaz Video AI and CyberLink's software are prime examples of this approach, implementing methods that attempt to minimize undesirable artifacts. However, there are limitations. The effectiveness of these techniques is often tied to the source video's quality. Heavily compressed videos or footage with pronounced motion blur can push the boundaries of even advanced algorithms, creating situations where complete artifact removal remains elusive. This highlights an ongoing tension within the field: Balancing the desire for superior image quality with the computational demands of real-time or near real-time processing. As developers continually refine these technologies, users must navigate the diverse array of tools that each offer varying degrees of success in eliminating artifacts and resolving motion blur, and understand the limitations. This pursuit of optimal image quality continues to push the boundaries of AI video processing, constantly refining the balance between enhanced visuals and the technical constraints of the process.
In the realm of AI video processing, tackling motion blur and artifact removal is a significant challenge, especially when upscaling older or lower-quality videos. AI tools are getting better at understanding motion within a video sequence, using frames before and after the current one. This temporal analysis can help reduce blur in fast-moving scenes, with some research showing results improving the clarity by up to 90%. However, it's a tough problem to solve, especially when the original video is very compressed, leading to more artifacts.
Interestingly, AI upscalers are now more sensitive to these artifacts, seemingly able to reduce them by a notable 70% or more in some cases. They achieve this using detailed pixel analysis and deep learning techniques, which help identify and remove those imperfections. We're also seeing algorithms that can dynamically insert missing frames, essentially creating smoother transitions in action-packed footage, improving the overall viewing experience by potentially 30%. This has huge implications for older movies or content with a lower frame rate, where choppiness can really detract from the experience.
Maintaining consistency across the video is important. Sophisticated AI algorithms analyze changes from frame to frame to ensure that moving objects are still clear, even when there's blur or compression in the original footage. This effort to maintain temporal coherence is essential for a visually appealing outcome. Furthermore, newer methods that analyze several frames at once (instead of just one frame at a time) seem to reduce juddering and ghostly effects, with reports of a 50% improvement or more in specific cases.
One encouraging development is the trend towards greater user control in the software. While we're seeing more automation with these AI tools, some offer options for adjusting settings specifically aimed at fixing motion blur and artifacts. This fine-grained approach provides more control to those who know what they're doing. But, it introduces another layer of complexity that might overwhelm beginners.
On the flip side, when trying to perform video conversion in real time, we often see compromises on detail, especially in challenging scenes. While promising, the effort to improve speed for real-time processing may lead to a reduction of artifact removal efficiency by 20-40% or more. It's a trade-off between speed and visual fidelity that we're still learning to manage.
AI's ability to distinguish between different types of artifacts is also growing, which leads to more polished results. Instead of just generally suppressing noise, we see more targeted cleanup, addressing specific high-frequency issues for more effective restoration.
Another interesting tactic involves having AI algorithms learn from user feedback on previous video outputs. By doing this, the systems gradually improve in their ability to fix artifacts based on the user's preferences, a novel approach that can help us achieve finer control over the AI's behavior.
We are seeing research exploring combinations of pre and post-processing methods to further refine both motion blur and artifact issues. The thinking is that starting with an AI-driven enhancement and then following up with a targeted corrective step can deliver an even better end result. The potential for improvements in video quality using these multiple, concurrent algorithms is promising.
Video Converter Software in 2024 Balancing Efficiency and Quality for AI Upscaling Workflows - Cost-Effective Solutions for Professional-Grade Video Upscaling
The demand for professional-quality video upscaling without excessive costs is driving the development of affordable software solutions. In 2024, a range of AI-powered tools have emerged, offering resolution enhancements to suit various user skill levels. Several options, like VideoProc Converter AI and AVCLabs Video Enhancer, showcase impressive upscaling capabilities while remaining accessible through competitive pricing or even free versions. However, the effectiveness of these tools varies greatly depending on the original video's quality and compression level. This necessitates a careful evaluation of options to strike a balance between price, processing speed, and output quality. While exciting developments in AI upscaling technology continue, users should be mindful of the trade-offs involved in attaining professional results on a limited budget. It remains a dynamic field where the perfect balance of cost and capability is continually being refined.
When it comes to enhancing video resolution, AI-powered upscaling is proving to be a game-changer in 2024. Compared to traditional upscaling methods which rely on fixed mathematical approaches, these AI-driven techniques can adapt to diverse video types, resulting in potentially faster processing, sometimes by as much as 30%. This adaptability stems from the ability of advanced algorithms, particularly those based on convolutional neural networks, to not only analyze individual frames but also sequences of frames in a video. This multi-frame analysis is crucial for maintaining consistency in motion, particularly important for minimizing jarring artifacts often present in upscaled videos. There's been significant improvement here with output quality showing considerable gains.
One of the most noticeable applications of these AI-powered techniques is the ability to synthesize new frames in videos originally captured with a lower frame rate. This helps alleviate the choppiness frequently associated with older footage, with potential improvements in the fluidity of motion by up to 30%. The viewing experience benefits greatly from this enhancement as it makes motion much smoother and more enjoyable.
However, the quality of the original video footage plays a major role in how well these AI methods perform. Highly compressed videos, for example, often come with a host of artifacts that even the most advanced algorithms struggle to eliminate entirely. The upscaling process gets much easier and the outcome improves considerably when the source material is of better quality.
Real-time processing, a feature some upscalers offer, represents a trade-off. While it offers the advantage of seeing changes instantly, it usually comes at the cost of some quality sacrifice in artifact removal. These systems can see a reduction in efficiency of artifact removal by as much as 20-40% or more to achieve that faster processing. This tension between speed and fidelity presents a challenge that researchers continue to explore.
An exciting new development is the integration of user feedback in the AI learning process. This allows upscalers to adapt to individual preferences over time, gradually refining their ability to eliminate unwanted elements from the output video. Moreover, AI-powered tools are getting noticeably better at tackling the problem of artifacts. Utilizing advanced pixel analysis, they're capable of identifying and eliminating these errors more effectively, potentially reducing them by over 70% under optimal conditions. This granular approach is an improvement over blanket fixes used in earlier iterations, providing cleaner and more refined video output.
Though neural networks underpinning these AI upscalers deliver superior output, they also come with the inherent need for substantial computational power. This can translate to longer processing times, extending from seconds to minutes depending on the specific algorithms employed and the complexity of the upscale.
Maintaining a cohesive visual experience over time is important. AI-powered tools are getting better at ensuring that moving objects remain clear and consistent in their representation throughout a video, leading to a more pleasing and natural viewing experience.
Finally, it's worth noting that merging AI-based upscaling with established video editing software isn't a straightforward process. There can be bottlenecks because of the significant computational load AI upscaling introduces. Users will need to navigate the automated features offered by the AI as well as maintain the flexibility and manual controls that are often part of classical editing workflows. This careful balancing act is necessary to ensure both automation and artistic control. The integration of AI into video editing is a work in progress with upscaling and the efficiency of it as well as workflows.
Video Converter Software in 2024 Balancing Efficiency and Quality for AI Upscaling Workflows - Streamlining Bulk Video Processing for Efficient Workflows
The ability to process large volumes of video quickly and effectively has become essential in 2024. Creators are dealing with ever-growing libraries of footage, and the need to streamline their workflows is paramount. AI-driven video converter software is playing a key role in this shift by providing automated solutions for various tasks. Resolution enhancement, color correction, and the removal of common video issues like artifacts are now handled with greater precision by these tools. Utilizing GPU acceleration, some programs can significantly reduce processing times, while still maintaining high quality outputs. This allows creators to focus their time and energy on creative aspects, rather than spending hours on repetitive or tedious tasks.
But this isn't without its challenges. Different videos have wildly varying qualities – some are pristine, others are riddled with compression or motion issues. These can greatly impact how effectively AI tools work. And then there's the challenge of making sure these sophisticated AI tools integrate smoothly into the editing workflows people are already using. Some users need the ability to fine-tune the process, while others want to benefit from the automated ease these tools can offer.
Ultimately, the quest for efficient video processing is ongoing, demanding the development of versatile tools that meet the needs of a range of creators. There's still work to be done to fully navigate the complexities of AI-powered video processing, but it's becoming clear these tools are essential to maintaining speed and quality in the modern video creation process.
In the ever-evolving field of video processing, especially within the context of video converter software in 2024, we're seeing a push towards efficiency improvements, particularly when dealing with large volumes of video data. Here are ten fascinating observations related to this trend:
1. **The Power of Parallelism:** Leveraging parallel processing capabilities, especially through multi-core processors, has a huge impact on bulk video conversion. It allows for processing multiple video files concurrently, leading to a potential four-fold increase in throughput when compared to traditional, sequential processing methods. It's become quite clear that parallel processing is a game-changer for speed.
2. **Adaptive Algorithm Design:** Modern video converters now feature algorithms that can dynamically adapt and allocate resources based on the characteristics of each video file. This means the software can prioritize certain tasks during the conversion process leading to a more responsive and efficient workflow. How these algorithms are designed and optimized is crucial to how fast and efficiently a converter will run.
3. **The Efficiency of Batch Processing:** The concept of batch processing has been a huge boon to efficiency. Handling many files at once can save a tremendous amount of time by reducing the overhead of starting and stopping numerous conversion tasks. A single, seamless conversion run for a batch of files really simplifies things, but the tool has to be designed to handle this in a manner that avoids bottlenecks and errors.
4. **Frame Rate's Impact on Speed:** The frame rate of a video file has a significant effect on how quickly it can be processed. Higher frame rates lead to greater computational demands, hence longer processing times. This is something that needs to be considered in bulk workflows, as you may have varied frame rates and that will lead to a different conversion times for different files. It could be a good idea to preprocess video to try and standardize the frame rates to try and achieve faster conversion across a large batch.
5. **Dynamically Adapting Bitrates:** Some video converter software now incorporate dynamic bitrate adjustment, where the software intelligently adjusts the bitrate on the fly during conversion to optimize file size and processing speed. It ensures quality while keeping conversion times reasonable, especially beneficial for bulk operations. It's a clever way of addressing this constant tension between quality and speed.
6. **The Value of Proxy Editing:** For smoother workflows in professional settings, the use of lower-resolution proxy files for initial editing, before the higher-resolution upscaling is applied in bulk, has become common practice. This speeds up the editing phase without sacrificing the final quality post-upscaling. A pretty good idea.
7. **Real-Time Performance Insights:** The inclusion of real-time performance metrics in video converter software allows users to monitor CPU and GPU utilization during bulk operations. This detailed insight enables engineers to quickly identify and troubleshoot performance bottlenecks and optimize settings for efficiency. We're moving towards more fine-grained control over processing by seeing this data in real time.
8. **Preprocessing with AI:** Artificial intelligence is increasingly being employed for preprocessing tasks like noise reduction and deinterlacing before the bulk video conversion stage. Improving the quality of the input before bulk processing ultimately leads to superior results in the end, streamlining the entire process. Using AI to prepare videos in this way has some pretty intriguing implications for the future.
9. **New Codec Potential:** Newer video codecs like AV1 are beginning to gain traction as they are designed to improve bulk processing speeds without sacrificing quality. Their improved compression algorithms result in faster transcoding and smaller file sizes, making them ideal for bulk conversions. Whether or not AV1 is the future of video codec compression, it certainly shows us how improvements here are very important.
10. **Designing for Users:** An increasing focus on the user experience within the design of video converter software has resulted in simpler and more streamlined interfaces. This focus on a clean and organized interface has the benefit of speeding up the learning curve for new users, ultimately promoting a more efficient workflow, especially with bulk operations. Features like drag-and-drop file management and intuitive controls are really important for end users.
These observations highlight the remarkable progress being made in video processing and how it's helping us streamline video workflows. While challenges still remain, the future of video conversion is bright and will undoubtedly involve even more efficient and advanced solutions as the algorithms and tools continue to evolve.
Upscale any video of any resolution to 4K with AI. (Get started for free)
More Posts from ai-videoupscale.com: