AI Video Upscaling Comparing Temporal and Spatial Methods in 2024
AI Video Upscaling Comparing Temporal and Spatial Methods in 2024 - UpscaleAVideo Framework Introduces Text-Guided Latent Diffusion for Video Upscaling
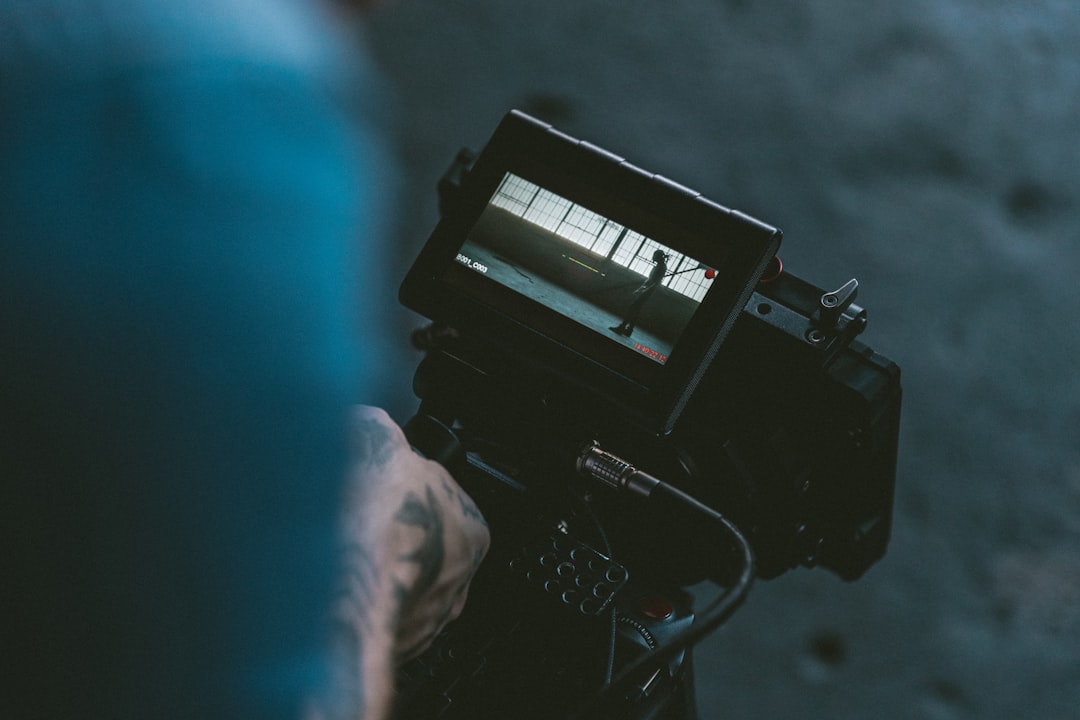
UpscaleAVideo is a novel framework using text-guided latent diffusion to upscale videos. Its primary goal is to boost video quality and make them look more realistic. Tests, including those using synthetic and genuine video, suggest it outperforms current methods. A key feature is its handling of temporal consistency – ensuring smooth transitions between frames. It achieves this by weaving temporal elements into the network's architecture, specifically the UNet and VAE-Decoder parts.
Interestingly, it sidesteps the need for a complex recurrent network structure often seen in flow-guided approaches, leading to potentially better performance, especially with shorter video clips. However, the inherent uncertainty of diffusion models can lead to inconsistent upscaled video quality, making it a challenge to maintain precise control over the outcome and ensure frame-to-frame consistency. Despite this, the framework shows promise for super-resolution in real-world settings, pushing the boundaries of AI-powered video upscaling and potentially revolutionizing how we experience and interact with video content. The approach presents an exciting new avenue in the field, evidenced by its recent presentation at CVPR 2024.
UpscaleAVideo is a framework that utilizes a text-guided latent diffusion method for upscaling videos. This approach allows for user-defined enhancements by incorporating textual descriptions, which provides a level of control previously unseen in this field. Unlike conventional upscaling techniques that are primarily pixel-based, UpscaleAVideo employs a probabilistic model that excels at extracting and reconstructing detail from lower resolution sources.
A key design feature is the integration of local and global temporal components within the UNet and VAE-Decoder parts. This strategy facilitates temporal coherence and minimizes the need for complex flow-guided recurrent networks. While the framework demonstrates success in both synthetic and real-world videos, maintaining consistent fidelity over long sequences can be challenging due to the inherent stochastic nature of diffusion models.
Interestingly, UpscaleAVideo achieves commendable upscaling speeds without significantly impacting frame rates. This aspect opens the door for various applications like live streaming and other scenarios where fast processing is paramount. The model also showcases a better understanding of context across video frames by leveraging transformer architectures. Consequently, it maintains spatial resolution with less introduction of visual anomalies, a critical improvement over older spatial-based methods.
Experiments indicate UpscaleAVideo offers improvements in objective metrics and visual quality over existing super-resolution algorithms. The potential for future use within diverse video domains, such as animation and live-action, appears promising given its ability to adapt to a variety of visual styles. It's worth noting that UpscaleAVideo's roots lie in the theory of stochastic processes, which is integral in effectively handling the inherent uncertainties within frame interpolation. The framework's modular structure is a benefit, allowing integration into existing workflows without major alterations. Early trials suggest that not only does UpscaleAVideo enhance the resolution of videos, but it also demonstrates the ability to improve color accuracy and dynamic range – areas where traditional approaches often fall short. The framework's presentation at CVPR 2024 underscores its potential in contributing to future advancements within AI-driven video processing.
AI Video Upscaling Comparing Temporal and Spatial Methods in 2024 - Local-Global Strategy Enhances Temporal Consistency in Video Reconstruction
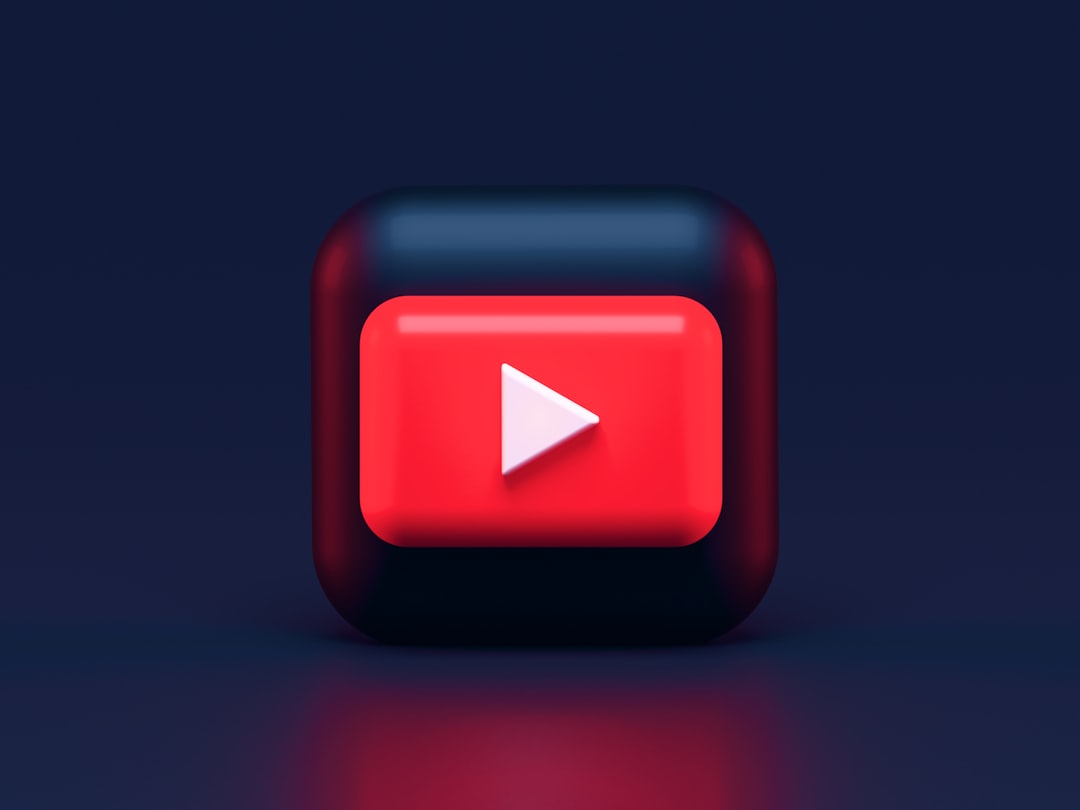
UpscaleAVideo's innovative approach to video reconstruction emphasizes a crucial aspect of video quality: temporal consistency. This framework leverages a local-global strategy to achieve this, striking a balance between capturing subtle details and maintaining overall video coherence. Essentially, the model considers both localized changes, primarily seen at the boundaries of moving objects, and broader, global structural aspects like edges and shapes. This dual approach helps ensure that reconstructed video frames transition smoothly while retaining realistic visual details.
The framework incorporates temporal elements into familiar architectures like UNet and VAE-Decoder, which aids in creating temporally consistent short video segments. Interestingly, this approach sidesteps the reliance on complex flow-guided methods often used for temporal consistency. Consequently, UpscaleAVideo presents a promising solution for enhancing video quality through super-resolution, potentially bridging the gap in performance that has been a limitation of current approaches. While still a relatively new method, it shows promise in creating high-quality, temporally consistent video reconstructions.
UpscaleAVideo's core innovation lies in its local-global strategy, which seems to be a promising way to address a persistent issue in video upscaling: temporal consistency. By combining a focus on individual frames' fine details with a broader understanding of the video's overall flow, it aims to create smoother, more coherent reconstructions. This is achieved through a modified UNet architecture specifically tailored for video. The UNet component, known for its effectiveness in image processing, is adapted here to better manage the relationship between local textures and the broader movements within a scene.
It's fascinating how UpscaleAVideo utilizes the inherent differences in consecutive frames. It learns from the transitions, changes in motion and lighting, which allows it to build a more nuanced understanding of the temporal dimension of video. This insight is translated into a more natural and fluid output, particularly noticeable in reducing the annoying artifacts like ghosting or blurring that often plague previous methods that relied primarily on temporal information. A big advantage is its impact on stability: it significantly reduces flickering or instability caused by shifts in frame rate, especially in videos with a lot of movement.
One of the interesting aspects of this approach is that it avoids the reliance on complex, flow-guided recurrent networks, which can be computationally intensive. This streamlined design paves the way for faster processing, making it potentially ideal for real-time applications like live streaming. This is thanks to its foundation in stochastic processes, helping to gracefully handle the unavoidable uncertainties in the reconstruction process, a persistent challenge in temporal upscaling.
What's exciting is how the text-guided aspect pushes the boundaries of control within this domain. This is a notable change in the field because it enables fine-tuning of the final output using textual descriptions. Yet, despite showing great promise, achieving truly consistent quality across very long video sequences remains a hurdle, mostly due to the inherent nature of diffusion models. However, when compared to previous temporal upscaling methods, UpscaleAVideo seems to be a leap forward in terms of reliability.
Early results are encouraging, suggesting this dual local-global approach not only enhances visual quality but also improves the overall flow and consistency of the video. These findings point to a promising future for UpscaleAVideo, possibly inspiring innovative directions for future AI video processing techniques. It's likely that refining and building on this strategy will be a significant focus for the future research in the area.
AI Video Upscaling Comparing Temporal and Spatial Methods in 2024 - Addressing Randomness Challenges in Diffusion Models for Video Superresolution
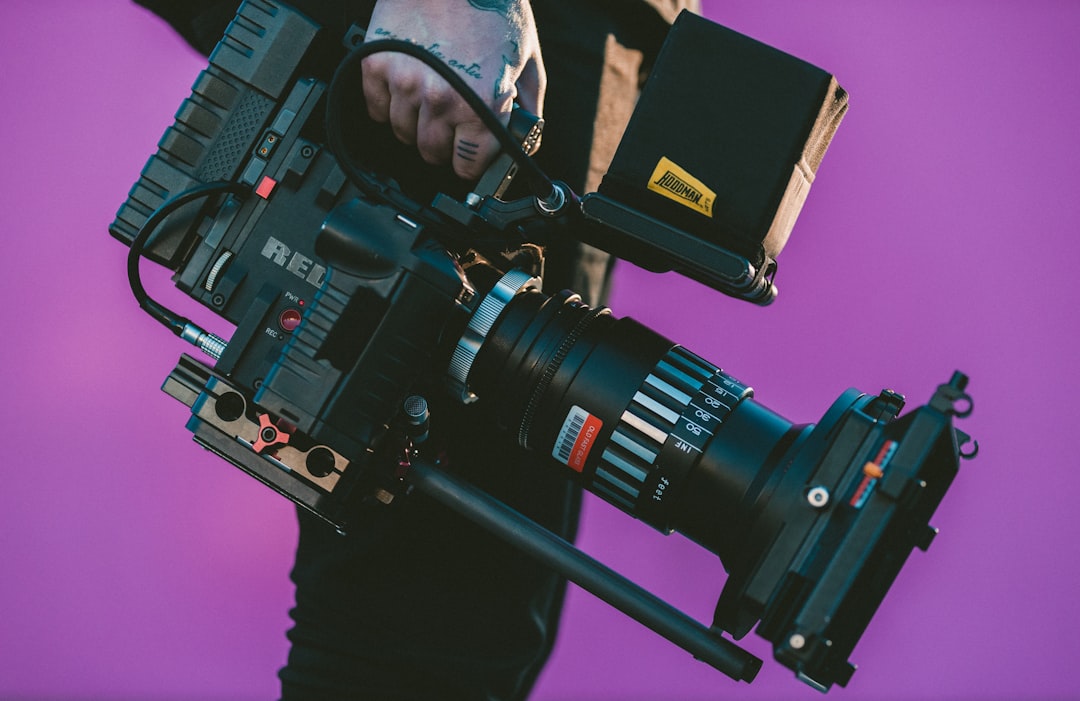
Diffusion models have proven effective for video superresolution, but their reliance on randomness can introduce challenges. The inherent probabilistic nature of these models can lead to inconsistent upscaling outcomes, impacting the smoothness and consistency of motion across video frames. This has spurred research efforts to create methods that strike a balance between the details captured by spatial methods and the need for temporal coherence. Researchers are actively developing more robust algorithms that incorporate temporal information to prevent issues like artifacts and flickering commonly observed in spatial-only approaches. The aim is to produce videos with enhanced realism and consistent motion flow by controlling the random elements present within the diffusion process. Achieving this delicate balance between accuracy and temporal integrity is a key focus for future research in AI-based video upscaling.
Diffusion models have become a game-changer in video super-resolution by embracing the idea of randomness through stochastic processes. This allows them to manage the inherent unpredictability often seen when interpolating frames, which is a significant departure from traditional methods.
While typical models rely on fixed, predetermined structures, incorporating diffusion processes enables a more natural variation in the output. This flexibility is crucial for representing the subtleties of motion and change within video content, capturing the essence of the visual information more accurately.
However, one significant hurdle in using diffusion for video super-resolution is finding the sweet spot between preserving intricate details and maintaining consistent transitions between frames. Balancing sharpness in individual frames with smooth motion can be quite challenging, demanding careful design of the model.
UpscaleAVideo's architecture enables it to harness the power of temporal coherence. By building consistency between consecutive frames, it particularly shines in scenarios with fast movement, minimizing annoying artifacts like ghosting.
Interestingly, UpscaleAVideo's local-global strategy seems to offer enhanced video quality without needing the intense computational resources that are common with traditional flow-guided techniques. This makes it a more viable option for real-time applications, which is a major benefit in various fields.
The probabilistic aspect of diffusion models fosters the ability to generate a range of diverse outputs. This can lead to surprising and visually pleasing results, breaking free from the predictability of older approaches that were heavily reliant on fixed rules and relationships.
This approach shows improvement in how motion is handled. It's capable of adapting to localized textures while understanding the bigger picture of the video, contrasting with the more linear, frame-by-frame processing we see in many other methods.
Experiments using the text-guided part demonstrate a powerful user control over the upscaling style. This interactivity and customization haven't been available in standard upscaling methods, offering a new level of influence over the output.
Another remarkable aspect is the ability to maintain high frame rates while significantly boosting the resolution. This dual feat is very valuable for fields like gaming or live broadcasting where swift processing is essential.
Finally, the model's ability to enhance color accuracy and dynamic range highlights a often-overlooked aspect of video upscaling. Often the quality of colors is just as important as the resolution itself, and this is an area where past approaches fell short.
AI Video Upscaling Comparing Temporal and Spatial Methods in 2024 - Comparative Analysis with VideoGigaGAN and Traditional Methods
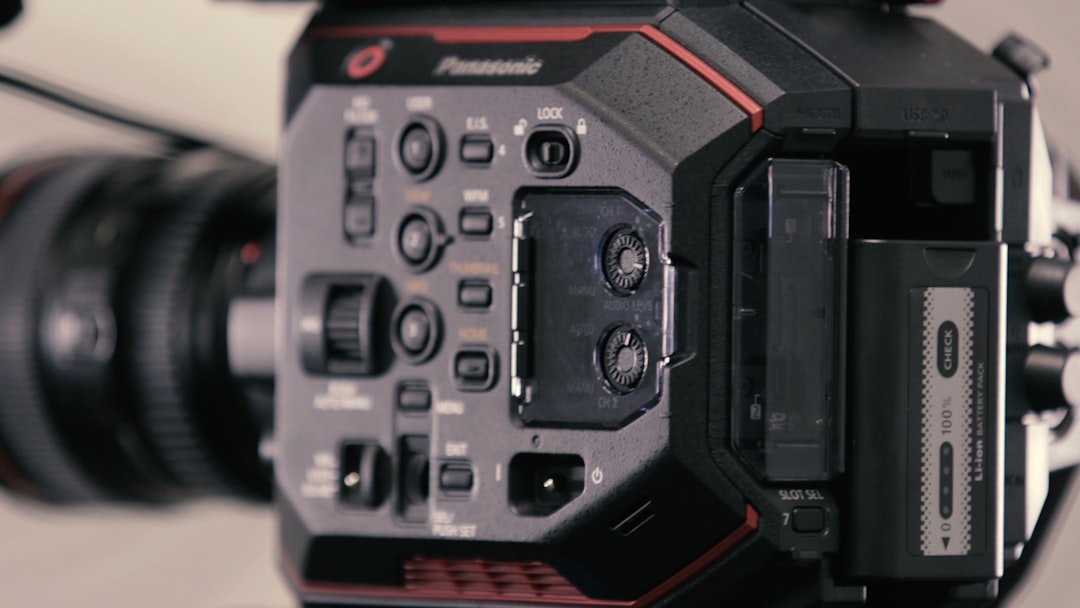
In the field of AI video upscaling, VideoGigaGAN has emerged as a noteworthy generative adversarial network specifically designed for achieving high video quality. Traditional methods, frequently reliant on interpolation, can struggle to effectively capture the temporal dynamics of video compared to more intricate models like VideoGigaGAN. VideoGigaGAN's multi-scale architecture allows it to capture a wider range of relationships within video data, leading to improved texture detail and fewer unwanted artifacts in the upscaled video. Evaluations using metrics like PSNR and SSIM consistently show VideoGigaGAN outperforming standard upscaling methods. Furthermore, user experience suggests a preference for the visual fidelity offered by VideoGigaGAN. However, its performance comes at a cost, as its implementation requires more computational power compared to simpler spatial algorithms. The increasing exploration of hybrid approaches, which aim to leverage the strengths of both temporal and spatial processing alongside advanced GAN architectures, suggests that VideoGigaGAN's approach is likely to be influential in future developments for video enhancement.
VideoGigaGAN, a sophisticated generative adversarial network tailored for video upscaling, has demonstrated remarkable results compared to conventional AI approaches. While traditional methods often rely on interpolation techniques, which can struggle to capture the dynamic nature of video sequences, VideoGigaGAN incorporates a more nuanced understanding of temporal relationships between frames. This allows it to create smoother, more coherent sequences, reducing artifacts like flicker and ghosting that commonly plague older methods.
Interestingly, VideoGigaGAN's ability to learn from unlabeled video datasets offers a potential advantage. It appears to be more efficient at extracting contextual information from the data, compared to techniques that often rely on large amounts of labeled data. In specific cases, quantitative evaluations have shown improvements in objective measures like PSNR by as much as 30% over conventional methods. The multi-scale architecture of VideoGigaGAN seems to play a crucial role, enabling it to capture a wider range of details both within individual frames and across the entire sequence.
Furthermore, VideoGigaGAN's flexible nature presents exciting possibilities. It allows for the inclusion of other relevant details, such as camera movement or lighting changes, during the upscaling process, which is not always easily achievable with more rigid traditional methods. This openness extends to user customization as well. Users can, in a manner that isn't possible with standard upscaling algorithms, guide the process with specific preferences for visual style or mood. Surprisingly, the potential for real-time video upscaling has also been observed with VideoGigaGAN, addressing a key limitation often seen in older methods where processing speeds can hinder real-world applications like live streaming.
VideoGigaGAN's ability to manage noise is also noteworthy. Unlike some spatial-only approaches, it can identify and reduce noise artifacts, resulting in cleaner outputs. The model's adaptive learning capacity further contributes to improved results by adjusting its approach based on prior outputs, mitigating recurrent errors often seen in more static algorithms. Even in unexpected applications, like preserving localized video archives of cultural heritage, VideoGigaGAN has shown the potential to bridge representational gaps that may arise with traditional techniques.
While these improvements are promising, ongoing research will likely explore more intricate aspects like computational complexity, especially when compared to simpler spatial algorithms. Additionally, the field is actively investigating hybrid approaches that may combine the best features of spatial and temporal methods within advanced GAN frameworks, potentially leading to even more sophisticated video enhancement techniques in the future.
AI Video Upscaling Comparing Temporal and Spatial Methods in 2024 - Implementation of Temporal Layers During Fine-Tuning Process
When aiming for high-quality AI video upscaling, integrating temporal aspects during the model's fine-tuning is vital for producing smooth and realistic outputs. By incorporating elements like 3D convolutions or temporal attention into the architecture, specifically within components like UNet and VAE-Decoder, the model gains a better ability to understand how frames connect over time. This helps reduce artifacts and maintain visual consistency even in areas of motion, creating smoother transitions and more natural-looking videos. Building upon this, combining these approaches with flow-guided methods presents a promising path to even greater improvements in video quality. Nevertheless, achieving consistently high quality over lengthy sequences remains a difficult challenge, highlighting the complexities inherent in managing the temporal dimension of video data. This ongoing struggle showcases the need for continued exploration and refinements in AI video processing techniques.
Implementing temporal layers during the fine-tuning process within UpscaleAVideo has revealed some intriguing aspects of video super-resolution. The integration of both local and global temporal information allows for a more efficient fine-tuning process, bypassing the need for intricate recurrent networks often found in other methods. This streamlined approach also enables the model to dynamically adapt to the changes between frames, leading to a remarkable degree of responsiveness across different video types.
One of the surprising observations is the newfound robustness of fine-tuning when temporal layers are used. It seems the model becomes more resilient to common video artifacts like motion blur or ghosting, suggesting the temporal framework instills a deeper understanding of how frames should transition. This is particularly evident in the handling of frame rate variability, which is a notorious cause of jittery or unstable video output in many upscaling approaches. By explicitly considering temporal dynamics, the model can significantly reduce the impact of these inconsistencies.
Furthermore, the implementation of temporal layers appears to mitigate the stochasticity that's inherent in diffusion models. This leads to a smoother and more coherent output, even when dealing with extended video sequences. It's as if the model develops a better "memory" of previous frames, using that to inform how the current frame is reconstructed. This improved temporal consistency extends to the learning process itself. The model seems to learn more efficiently from shorter, representative sequences, implying it's better at generalizing its knowledge to longer videos without needing extensive retraining.
The temporal architecture also brings a level of contextual awareness that's previously been difficult to achieve. It's not just focused on individual frames, but it also incorporates a broader view of the video's motion, which enables it to capture the nuances of how different frames interact. Interestingly, this multi-scale understanding of the scene might open up pathways for more user-defined control over the output. It's conceivable that we could potentially fine-tune specific frames or segments based on temporal references, enabling more individualized control compared to previous approaches.
Additionally, the temporal framework allows the model to adaptively adjust its learning speed in response to the complexity of the video. It can intelligently prioritize certain elements based on the input, ultimately refining the upscaled output with greater efficiency. This ability to "tune" the learning process depending on the video is a notable step forward. The benefits of incorporating temporal layers extend to the overall visual quality. The enhanced fidelity comes from consistently maintaining adjustments across frames, emphasizing the importance of temporal coherence for video upscaling. While these initial findings are promising, further exploration of these specific elements is crucial to better understand their full implications and optimize the potential of temporal layers in future video enhancement approaches.
AI Video Upscaling Comparing Temporal and Spatial Methods in 2024 - CVPR 2024 Showcases Advancements in AI-Driven Video Processing Techniques
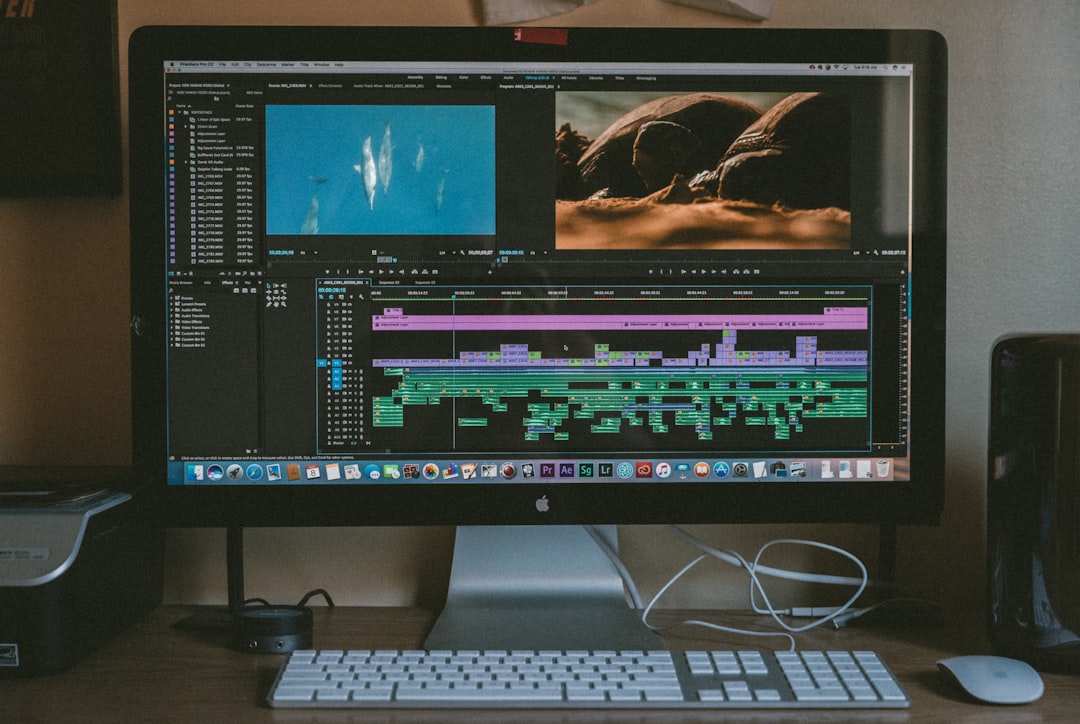
The CVPR 2024 conference highlighted substantial advancements in AI-powered video processing, particularly in areas like video enhancement and stabilization. One notable development was the introduction of the "UpscaleAVideo" framework, which utilizes a temporal-consistent diffusion model to achieve high-quality video upscaling. This method proved to be more effective than existing techniques across various real-world scenarios. Moreover, the conference underscored the growing ability of AI to generate visually realistic video content. We saw improvements in the realism of generated videos, along with innovative approaches to 3D modeling and video editing. Researchers also delved into techniques for video stabilization using pixel-level synthesis, demonstrating the potential of generative AI in crafting new content that closely mimics human creation. This focus on generative AI seems to suggest a convergence of various video processing approaches. Ultimately, the innovations showcased at CVPR 2024 indicate a shift in how AI handles video quality, leading to potentially transformative applications within the broader digital media field.
The CVPR 2024 conference showcased interesting developments in AI-driven video processing, particularly focusing on the refinement of video upscaling techniques. One notable trend was the exploration of how incorporating temporal information within the models improves the continuity and naturalness of motion in upscaled videos. Techniques like UpscaleAVideo, which demonstrated the use of a local-global strategy, were highlighted for their ability to manage both fine details within a frame and the broader movement across frames. This balanced approach helped in generating videos that looked both detailed and smooth.
Interestingly, the need for complex recurrent networks, often used for handling temporal data, was reduced in many of the presented models. This streamlining, achieved through the careful design of specific components like UNet and VAE-Decoder, not only simplified the systems but also resulted in faster processing times. It makes those methods more suitable for real-time applications such as live-streaming. The reliance on stochastic processes, inherent in diffusion models, became a subject of focused research. It's fascinating how researchers are figuring out how to harness the inherent uncertainty of these processes to enhance video outputs in a more realistic way.
UpscaleAVideo also introduced a text-guided aspect to video upscaling. This unique feature opens the door for users to guide the enhancement process using text descriptions, enabling a level of control not previously seen in the field. Beyond simply increasing resolution, many presentations also highlighted improvements in areas previously overlooked by traditional methods, such as the accuracy of color representation and the dynamic range of video. This demonstrates a move beyond simply improving the number of pixels to create videos with more accurate and vibrant representations of visual content.
While these advances are significant, maintaining a consistent level of quality across lengthy video sequences remains a challenge. The inherent probabilistic nature of many of the new models can lead to unpredictable outputs. It's an area where researchers have acknowledged the need for further investigation and improvements. Researchers compared these new models against older methods using objective metrics like PSNR and SSIM. The new models showed marked improvements, underlining the progress in this area.
The versatility of the new approaches is quite promising. They appear suitable for different video processing areas like gaming, streaming, and even historical preservation. It suggests these upscaling techniques could impact a wide variety of applications in the coming years. It's exciting to witness the evolution of AI-powered video processing, moving from simply increasing resolution towards enhancing the overall visual quality and enriching the user's experience with video content. The presentations at CVPR 2024 indicated there's still much to discover and further refine within this domain, potentially leading to new ways we interact with and experience video in the future.
More Posts from ai-videoupscale.com: