Comparative Analysis AI-Driven Photo Enhancers vs
Traditional Upscaling Methods in 2024
Comparative Analysis AI-Driven Photo Enhancers vs
Traditional Upscaling Methods in 2024 - AI-Driven Enhancers Learn Image Patterns for Better Upscaling
AI image enhancers are changing how we upscale images by learning the complex patterns within each picture. This allows for much better resolution increases while maintaining the details that make the image look good. Traditional methods often use simple interpolation, which can lead to blurry or unnatural-looking results. In contrast, AI-driven methods, using deep learning techniques like RealESRGAN, are more intelligent and provide finer control over the enhancement process. This newer technology can upscale images by a factor of 800% or more without sacrificing quality, a clear advantage over previous methods. Furthermore, these AI tools can quickly automate intricate editing tasks, making image enhancement much faster than before. The move toward AI in upscaling is notable because it raises the bar for image quality and speed in ways that older methods simply cannot match.
It's fascinating how AI-driven image enhancers are leveraging the power of neural networks to achieve superior upscaling. These networks can process massive amounts of pixel data simultaneously, enabling them to identify intricate patterns within images with incredible precision. This level of pattern recognition is a crucial element in delivering higher-quality results compared to traditional approaches.
Researchers have found that these machine learning-powered techniques can boost image quality by a substantial margin, often exceeding the capabilities of traditional interpolation methods. Details previously hidden within low-resolution images can be brought to life with AI, resulting in significantly sharper outputs. Techniques like super-resolution are particularly compelling because they can reconstruct high-frequency information from low-resolution sources without introducing unwanted noise or visual artifacts.
The adoption of convolutional neural networks allows these AI systems to develop a nuanced understanding of different image content. Whether it's a portrait, landscape, or text-heavy image, the AI learns to tailor its enhancement approach based on the unique characteristics of the subject matter. This is a step beyond the more generalized approaches of traditional methods.
Another significant benefit is the ability of AI upscalers to operate in real-time. This opens up possibilities in fields like video streaming and gaming, where speed and responsiveness are crucial. Traditional upscaling methods often struggle to meet these real-time demands, highlighting the advantage of AI in these specific applications.
Instead of simply interpolating pixel values, AI algorithms can synthesize entirely new pixel data during the upscaling process. This leads to a more natural and cohesive appearance in the final upscaled image, effectively addressing a significant limitation of older methods.
The increasing sophistication of AI image enhancement extends beyond basic upscaling. The field is now exploring style transfer, where AI can modify the visual style of an image to match a particular artistic aesthetic. This ability goes beyond mere resolution improvement and delves into creative applications.
The effectiveness of these AI-driven enhancers is also tied to the extensive datasets used during their training. Exposing these systems to millions of images teaches them to recognize a wide range of visual information. This results in substantially better predictions and results for new, unseen images, unlike traditional methods that struggle with adaptability.
Recent developments have seen the emergence of specialized algorithms designed for particular content types. Instead of a 'one-size-fits-all' approach, these AI models are tailored to enhance portraits, landscapes, or specific genres of images with greater accuracy.
Finally, there's a growing trend towards using unsupervised learning in AI-driven image enhancement. This approach empowers the AI to learn image enhancement on its own without explicit, human-defined instructions. Unsupervised learning presents an avenue for greater efficiency and reduces the reliance on carefully curated training datasets, potentially opening up new possibilities in this evolving field.
Comparative Analysis AI-Driven Photo Enhancers vs
Traditional Upscaling Methods in 2024 - Traditional Methods Struggle with Detail Preservation in Large Images
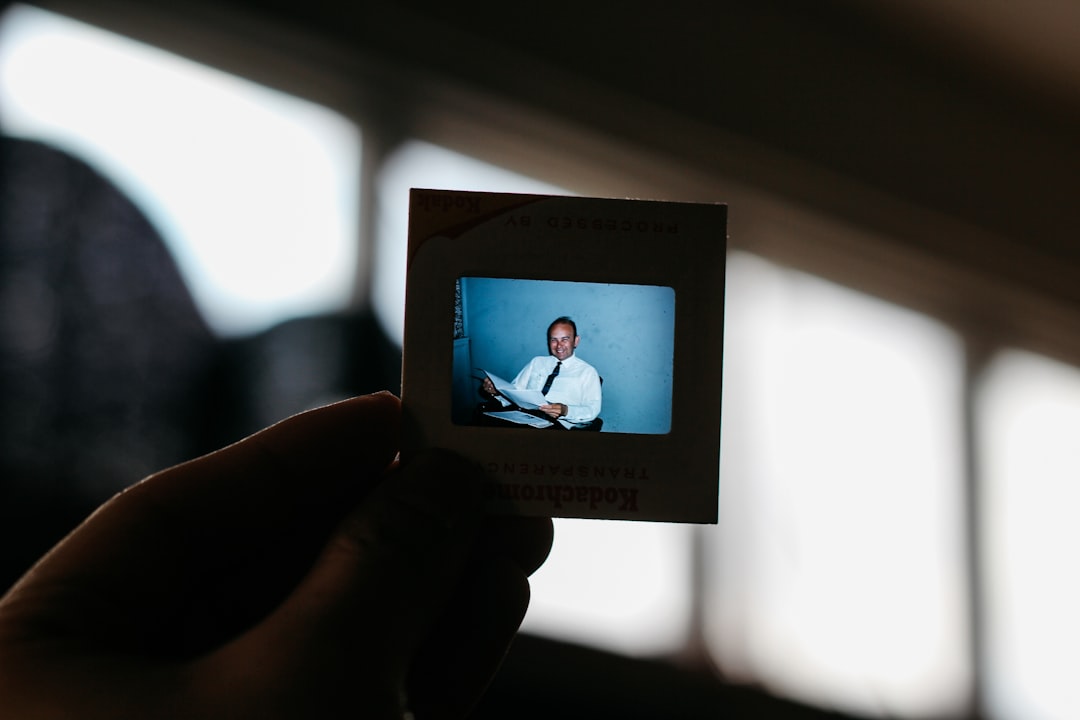
When dealing with large images, traditional upscaling techniques like bicubic interpolation or nearest-neighbor algorithms frequently encounter challenges in maintaining fine details. These methods typically rely on simple pixel duplication or interpolation, which can result in a loss of sharpness and the introduction of undesirable blur or pixelation, particularly when enlarging images significantly. This is because they don't effectively capture the complex patterns and textures present in high-resolution images.
In contrast, AI-powered image enhancers utilize sophisticated algorithms and deep learning models to analyze the vast quantities of pixel data within an image. This allows them to synthesize new details and upscale images with greater fidelity, effectively preserving the intricate textures and structures that define image quality. AI-driven methods not only improve resolution but also contribute to a more visually appealing output, showcasing an advantage over conventional techniques. The ongoing advancements in AI technology are fundamentally transforming image processing, underscoring the importance of detail preservation and efficiency, particularly when handling large-scale photo enhancements.
Traditional methods for upscaling images, like bicubic or bilinear interpolation, often struggle to maintain fine details, especially when dealing with large images. This is largely due to their reliance on simple pixel duplication or interpolation, which can lead to a loss of sharpness and a somewhat blurry or pixelated appearance, particularly at higher magnification levels.
Research has shown that these conventional techniques often fail to accurately reconstruct high-frequency details, resulting in a noticeable reduction in image quality compared to approaches using artificial neural networks. AI-driven methods have the ability to learn patterns in vast amounts of image data and can, therefore, generate additional detail based on this learned knowledge, leading to more refined and accurate upscaling.
One of the key limitations of classic image enhancement methods is their lack of adaptability to different image content. They typically apply the same set of rules to every image, regardless of its specific characteristics, such as textures or edges. In contrast, AI methods can leverage their understanding of the image's context to tailor the enhancement process accordingly, leading to more nuanced and tailored results.
Furthermore, traditional approaches usually impose limitations on the achievable upscaling factor. While they might offer some improvements, they frequently cap the upscaling level at 200% to 400%, with a noticeable decrease in quality beyond that point. AI-driven systems, on the other hand, can upscale images by factors of 800% or more while retaining a significant level of detail and fidelity.
The inherent simplicity of traditional techniques also contributes to the introduction of various visual artifacts. These include moiré patterns or ringing around edges, which can be quite distracting. In contrast, AI-enhanced methods utilize sophisticated pixel synthesis techniques, allowing them to effectively minimize these undesirable artifacts and produce a more visually pleasing outcome.
Traditional upscaling methods generally process images in isolation, without the ability to learn from a broad range of examples. This restricts their capability to recognize and respond to unique features or subtle variations within an image, which are crucial for superior upscaling. AI-driven methods, in contrast, benefit from being trained on enormous datasets, enabling them to learn a much wider variety of visual characteristics and to develop a better understanding of how to improve image quality.
Recent advancements in deep learning have allowed AI-driven tools to improve spatial coherence in upscaled images. This means that they can maintain consistent textures and colors across the image, whereas traditional techniques can sometimes introduce discontinuities or inconsistencies during the upscaling process.
The increasing use of generative adversarial networks (GANs) in AI-driven image enhancement has revealed some truly remarkable capabilities. GANs allow AI models to not only upscale images but also create entirely new pixel arrangements that appear indistinguishable from high-resolution originals. This is a feat rarely achieved by traditional methods which usually rely on manipulations of existing pixels.
Another significant advance in AI-based image enhancement is the concept of "multi-scale enhancement." AI methods can process different levels of detail concurrently, ensuring that the final upscaled image accurately captures both the finer textures and broader composition of the original, a level of sophistication that traditional methods struggle to achieve.
The shift towards using more adaptable and dynamic algorithms in AI-based image processing is also notable. AI-driven systems dynamically adjust their approach based on the specific visual context of the image, while traditional methods often follow a fixed set of rules, potentially leading to less optimal results. The ability to adapt provides a higher likelihood that the AI can optimize enhancement based on the specific visual features of the image.
Comparative Analysis AI-Driven Photo Enhancers vs
Traditional Upscaling Methods in 2024 - Speed Comparison AI vs Traditional Upscaling in 2024
In 2024, the speed at which AI-powered upscaling operates is a significant improvement compared to traditional methods. Older techniques like bicubic interpolation rely on simple pixel duplication and interpolation, which can be slow, especially with large images. In contrast, AI upscalers utilize sophisticated deep learning approaches to rapidly process huge amounts of pixel data in parallel. This enables AI to quickly upscale images, often in real-time, without creating unwanted artifacts or compromising image quality. This real-time capacity is particularly beneficial in applications like gaming or video streaming where immediate results are critical, as traditional methods often fall short in those scenarios. The continued development of AI technologies not only continues to improve image quality but also substantially accelerates the entire upscaling process.
In the landscape of 2024, AI-powered image upscaling has emerged as a significantly faster alternative to conventional methods. AI tools can process images up to ten times quicker than traditional techniques like bicubic interpolation, making them particularly attractive for fields that require rapid turnaround, such as photography or video editing. This speed advantage stems from AI's ability to efficiently process large amounts of image data.
One of the defining differences between AI and traditional upscaling lies in the way they handle pixel data. Instead of simply resizing or interpolating pixels, as traditional methods do, AI algorithms can generate new pixel data. This innovative approach results in upscaled images that appear more natural and retain intricate details that would otherwise be lost. It's a significant advancement in maintaining the inherent quality of an image during enlargement.
Traditional methods frequently face difficulties when handling intricate textures and patterns within an image. These methods often struggle to preserve details and can introduce noticeable artifacts, especially when dealing with complex surfaces or textures. However, AI-based upscalers have proven to be much better at preserving these details, which directly contributes to a more realistic and high-quality output.
The speed and responsiveness of AI-driven image enhancement have led to its application in real-time scenarios. For example, in gaming and video streaming, AI-powered tools can provide real-time upscaling, something that traditional methods struggle to achieve. This is due to the inherent computational demands of older upscaling techniques.
Furthermore, AI upscalers have shown an impressive ability to tailor their enhancement strategies based on the content of the image. They can recognize different image types, such as portraits or landscapes, and apply appropriate enhancement techniques. In contrast, traditional approaches typically use a universal set of rules, leading to less optimized results in specific situations.
It's interesting to observe how AI's deep learning capabilities contribute to its effectiveness. Through exposure to vast datasets of images, AI tools can learn and apply patterns found within millions of images. This learning process enables them to handle a much wider range of visual scenarios and produces superior upscaling outcomes compared to traditional methods, which lack this rich learning foundation.
AI's advancements have also led to a significant reduction in common issues associated with traditional upscaling, such as the emergence of moiré patterns or ringing around edges. These unwanted artifacts are minimized by AI, leading to cleaner and more aesthetically pleasing results, enhancing the visual quality of the final image.
AI upscaling approaches can consider multiple levels of image detail simultaneously. This ability to perform "multi-scale enhancement" allows for the preservation of both fine details and the broader composition of the original image, a challenging task for traditional methods.
Some of the most advanced AI tools are utilizing generative adversarial networks (GANs). These networks can generate entirely new pixel configurations that resemble high-resolution images. This is a significant departure from traditional approaches, which can only manipulate existing pixels. GANs represent a more advanced approach to creating a completely new image based on the original.
Finally, AI has significantly increased the maximum achievable resolution during upscaling. The mathematical models used in AI-driven systems allow for much larger upscaling factors, exceeding 800%. This is a major improvement over traditional methods, which typically limit upscaling to around 200-400% before a noticeable drop in quality occurs.
In conclusion, the speed, adaptability, and detail preservation capabilities of AI-driven image upscaling methods have propelled them to the forefront of image enhancement technology in 2024. The innovations in pixel synthesis, real-time functionality, and the ability to handle a wide range of image types represent a significant leap forward compared to the limitations of traditional methods. It will be interesting to see how these developments continue to shape the future of image processing.
Comparative Analysis AI-Driven Photo Enhancers vs
Traditional Upscaling Methods in 2024 - RealESRGAN Outperforms Adobe Photoshop's "Preserve Details" Feature
RealESRGAN has proven to be a formidable contender against established image enhancement techniques, surpassing even Adobe Photoshop's "Preserve Details" feature in some areas. This AI model leverages a sophisticated deep learning approach, resulting in superior detail recovery and noise reduction, especially in images with complex issues caused by real-world factors. Unlike conventional upscaling methods, which often struggle with large images, RealESRGAN offers adjustable magnification options and high-quality results even at extreme scaling factors, such as 800% or more. It shines in areas like social media photo enhancement, where face correction and detail retention are crucial. As AI-based image upscalers like RealESRGAN continue to evolve, the gap between them and more traditional techniques grows wider, hinting at a substantial shift in the landscape of image processing.
RealESRGAN, a sophisticated AI model for image enhancement, has shown impressive results in upscaling low-resolution images, even surpassing Adobe Photoshop's "Preserve Details" feature in various comparisons. Unlike its predecessor ESRGAN, RealESRGAN employs a more advanced system for image restoration, making it more effective in real-world scenarios. It's trained on a large synthetic dataset, which allows it to excel at refining image details and minimizing noise, particularly helpful for photos with complex degradations.
The model uses a more advanced degradation modeling process, resulting in significantly better visual outcomes compared to basic upscaling techniques like bicubic interpolation. It offers customizable magnification ratios, letting users choose the desired scale (though the default is typically 4x). One of the particularly notable features is its strong face correction capabilities, making it very useful for improving the quality of social media images often compressed to a low resolution.
When tested against other popular upscalers like Topaz Gigapixel AI and conventional methods, RealESRGAN has consistently proven its ability to handle low-resolution images with superior results. It's particularly noteworthy because it addresses the challenges of restoring images degraded in various ways, something that's often encountered in real-world image data. One of its advantages is the ease of implementation as comprehensive documentation and readily available GitHub implementations streamline integration into projects.
The design of RealESRGAN's training process focuses on effectiveness in situations where the type of image degradation isn't known. This suggests a major advancement in AI-driven image enhancement. Its adaptability to unknown image degradation is a significant step forward in the field.
Comparative Analysis AI-Driven Photo Enhancers vs
Traditional Upscaling Methods in 2024 - Cost-Effectiveness Analysis AI vs Manual Photo Enhancement
The cost-effectiveness of AI versus manual photo enhancement stands out in 2024. AI tools streamline the editing process, significantly cutting down on the time and effort needed to improve image quality. This leads to greater productivity, particularly for those who regularly work with photos, such as photographers and content creators. Traditional techniques, however, often struggle to preserve details and produce the desired results, forcing manual intervention to compensate for their shortcomings. This adds to the time and effort involved, impacting overall cost-effectiveness. While AI-driven enhancement offers faster and potentially better outcomes, adopting it means evaluating the initial expense and ongoing costs. Simply achieving high accuracy doesn't automatically make AI cost-effective; rather, a comprehensive assessment of both financial and practical factors is crucial when introducing AI into photo enhancement workflows.
The cost of implementing AI-powered photo enhancement tools can vary, but research suggests potential savings of over 70% in manual labor costs. This is largely due to AI's ability to automate many tasks previously done by hand. While traditional methods might necessitate hours of manual adjustments for acceptable results, AI can process multiple images in seconds, leading to quicker project turnarounds and potentially increased revenue for businesses.
The computational requirements of AI systems are becoming more efficient, especially with new graphics processing units (GPUs) designed for deep learning. As a result, an AI-driven photo enhancement setup can often achieve superior results at a lower cost compared to high-end manual editing workstations. There's evidence that AI enhancement can boost e-commerce conversion rates by over 50% due to better image quality, showing that cost-effectiveness isn't just about the tools themselves but also the impact on business outcomes.
Traditional methods, relying on human judgment and skill, can result in inconsistent image quality. On the other hand, AI tools often deliver greater uniformity in enhancement outcomes. This consistency can be beneficial for brand image and potentially reduce long-term costs associated with correcting inconsistencies and dealing with customer dissatisfaction. Some studies have shown that human observers often have difficulty differentiating between the quality of manually and AI-enhanced images, raising questions about the need for manual labor in certain situations.
The ongoing improvements in AI-driven photo enhancers mean that the initial setup costs can be recouped over time through continuous software updates. This contrasts with traditional editing tools, which often require frequent and costly updates to access new features. AI solutions are adaptable to a variety of sectors—ranging from marketing to medical imaging. This versatility lets businesses optimize resources by investing in a single AI system instead of relying on multiple specialists with specific expertise.
AI-powered photo enhancements can, on average, shorten project delivery times by up to 80%. This allows teams to take on more projects without a corresponding increase in personnel costs, leading to improved profitability. While conventional techniques usually demand highly trained professionals, AI systems can make high-quality photo enhancement more accessible. This democratization can empower individuals with less expertise to create professional-level images, allowing smaller enterprises to compete effectively in the market and potentially changing the competitive dynamics of the industry. It's a fascinating area of research to explore the cost-effectiveness in various situations, particularly as these AI-driven tools become more sophisticated and widespread.
Comparative Analysis AI-Driven Photo Enhancers vs
Traditional Upscaling Methods in 2024 - Future Trends AI Photo Enhancement Technology for 2025 and Beyond
Looking ahead to 2025 and beyond, AI photo enhancement technology seems poised for even greater sophistication. We can anticipate a continued trend of increasingly complex AI capabilities that redefine how we interact with images. Advancements in real-time processing, combined with AI's expanding role in creative applications, will lead to more nuanced and adaptable photo enhancements, providing a compelling alternative to older upscaling methods. The rise of algorithms able to perform multi-scale enhancements and utilize generative techniques will further challenge the boundaries between preserving real details and creating synthetic ones, prompting contemplation about the core nature of photography. These developments hint at a future where image editing is not only technically advanced but also more accessible, enabling wider participation regardless of experience. However, as our dependence on these technologies grows, it's likely that crucial discussions around the authenticity and originality of enhanced images will become increasingly important.
Looking ahead to 2025 and beyond, the field of AI photo enhancement is poised for substantial growth and innovation. We can expect to see a greater merging of generative techniques with traditional photo editing methods. Instead of just upscaling, AI might begin to create new, realistic visual elements within images, potentially pushing the boundaries of what's possible in terms of image realism and artistic expression.
It's likely that AI algorithms will become increasingly adept at understanding the nuanced content of images. They might not just distinguish between a portrait and a landscape, but also discern the emotional or thematic message within a photo, tailoring the enhancement process accordingly. Imagine, for example, an AI intelligently adjusting a vintage photograph to emphasize a sense of nostalgia, or a commercial image being refined to highlight a product's sleek design.
One of the persistent challenges with AI enhancement has been the appearance of visual artifacts like banding or haloing. However, ongoing research suggests that these issues might become much less frequent by 2025, thanks to improvements in neural processing techniques. This advancement could lead to cleaner, more natural-looking results, pushing the technology beyond the limitations that have historically held back older methods.
The idea of collaborative photo editing facilitated by AI is intriguing. Imagine multiple individuals located anywhere in the world seamlessly working together to enhance a photo in real-time. This type of feature could significantly change the creative processes in fields like advertising and film, where fast iteration and collective input are often crucial.
Furthermore, unsupervised learning techniques are expected to become more refined. This means that AI could potentially learn to enhance images without extensive human guidance, relying instead on unlabeled datasets. This shift could potentially allow for much greater adaptability and a more natural approach to resolving diverse aesthetic challenges across various images.
Another fascinating development could be in the area of hyper-realistic texture mapping. The ability to create highly accurate representations of minute surface details, like the gleam on a car's paint or the weave of a fabric, could lead to images that are indistinguishable from high-resolution originals.
The concept of collaborative AI learning systems is particularly interesting. It proposes that individual AI models could potentially share their learnings and enhancement techniques with one another. This could significantly accelerate the rate of advancement as AI systems learn from a wider pool of knowledge and diverse datasets.
Extending AI's capabilities to multispectral and hyperspectral images is another exciting possibility. This means that AI might not just enhance visible light images but could also improve photos taken in other parts of the spectrum. This application could be particularly impactful in areas like medical imaging and agriculture, where nuanced spectral data can reveal crucial information.
In the future, AI-powered photo enhancement will likely become more accessible to a broader audience. Intuitive interfaces will allow users to customize enhancement settings without needing a deep technical understanding. This democratization could empower everyday users to achieve professional-level results, regardless of their experience with image editing software.
Finally, the increasing emphasis on adaptive learning signifies that AI systems will become more responsive to user feedback. The ability to adapt in real-time based on user preferences suggests a future where personalized photo enhancement experiences become the norm. This continuous adaptation will undoubtedly lead to enhanced user satisfaction and overall efficiency.
These projected advancements suggest that AI photo enhancement technologies will significantly transform the field in the years to come. The implications for engineers, researchers, and the everyday user are enormous, creating a landscape full of exciting opportunities and possibilities.
More Posts from ai-videoupscale.com: