Comparative Analysis RTX 4070 Super vs
Non-Super for AI Video Upscaling Performance
Comparative Analysis RTX 4070 Super vs
Non-Super for AI Video Upscaling Performance - CUDA Core Comparison RTX 4070 Super vs Non-Super
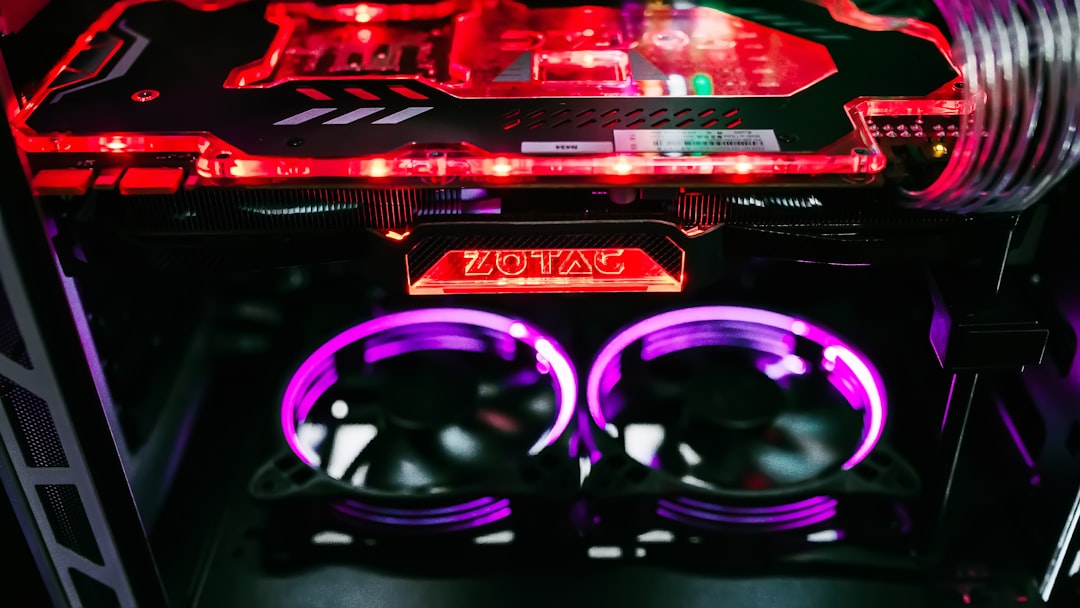
The RTX 4070 Super's core advantage over its non-Super counterpart lies in its increased CUDA core count. Sporting 7,168 CUDA cores, it represents a notable 20% jump from the RTX 4070's 5,888. This substantial increase in processing units directly impacts performance, particularly within AI video upscaling scenarios where raw processing power is crucial. Beyond just the core count, the Super model also benefits from a slightly elevated base clock speed. While not a massive leap, the minor bump does contribute to a generally higher processing throughput. The Super's architecture, leveraging more of the AD104 silicon, combined with these factors, solidifies its position as a more powerful option for various tasks compared to the original RTX 4070. Although marketed at the same price point, the RTX 4070 Super's core improvements offer a notable boost in capability, making it a worthwhile option for users who require enhanced performance for AI-related workloads and beyond. While the difference in clock speed isn't groundbreaking, the jump in CUDA cores alone has a tangible impact on performance. Ultimately, it gives the Super a noticeable edge for tackling complex processing tasks, like those encountered in AI video upscaling.
The RTX 4070 Super boasts a substantial increase to 7,168 CUDA cores, representing a 20% jump from the RTX 4070's 5,888. This core count difference alone suggests a potential performance boost, especially in tasks like AI video upscaling that can benefit from more parallel processing. The base clock speed of the Super variant is slightly higher at 198 GHz compared to the 192 GHz of the standard 4070. While seemingly minor, this difference could translate to tangible performance improvements under sustained loads.
The Super model also incorporates 224 TMUs and 80 ROPs, components responsible for texture processing and output management, respectively. These components work in tandem with the CUDA cores to contribute to the overall graphics and compute capabilities. Interestingly, the RTX 4070 Super, despite its performance advantages, carries the same $599 MSRP as the original 4070. This makes it a compelling option for users seeking better performance at a similar price point.
Benchmark results position the Super's performance between the RTX 3080 and the AMD RX 7800 XT, indicating a significant improvement over its non-Super sibling and the competitive landscape. Its performance across a variety of applications, from gaming to professional workloads involving AI, showcases its adaptability. The Super variant's architecture, based on the AD104 silicon, utilizes 56 out of 60 available SMs, suggesting that it's designed to be a high-performance graphics card with considerable headroom.
The gains observed in the Super model aren't just limited to gaming. The enhanced computational abilities of the Super variant make it a potentially attractive option for AI applications like training and inferencing, extending its versatility beyond gaming. Overall, the Super model represents a notable upgrade over the standard RTX 4070, seemingly exceeding the performance of its midrange competitors like the AMD Radeon RX 7800 XT, making it a potent option within the current GPU market. It appears to deliver a considerable performance leap at a very attractive price point, making it a potentially compelling choice for AI-focused video upscaling and other computationally intensive tasks.
Comparative Analysis RTX 4070 Super vs
Non-Super for AI Video Upscaling Performance - Memory Bandwidth and Bus Width Differences
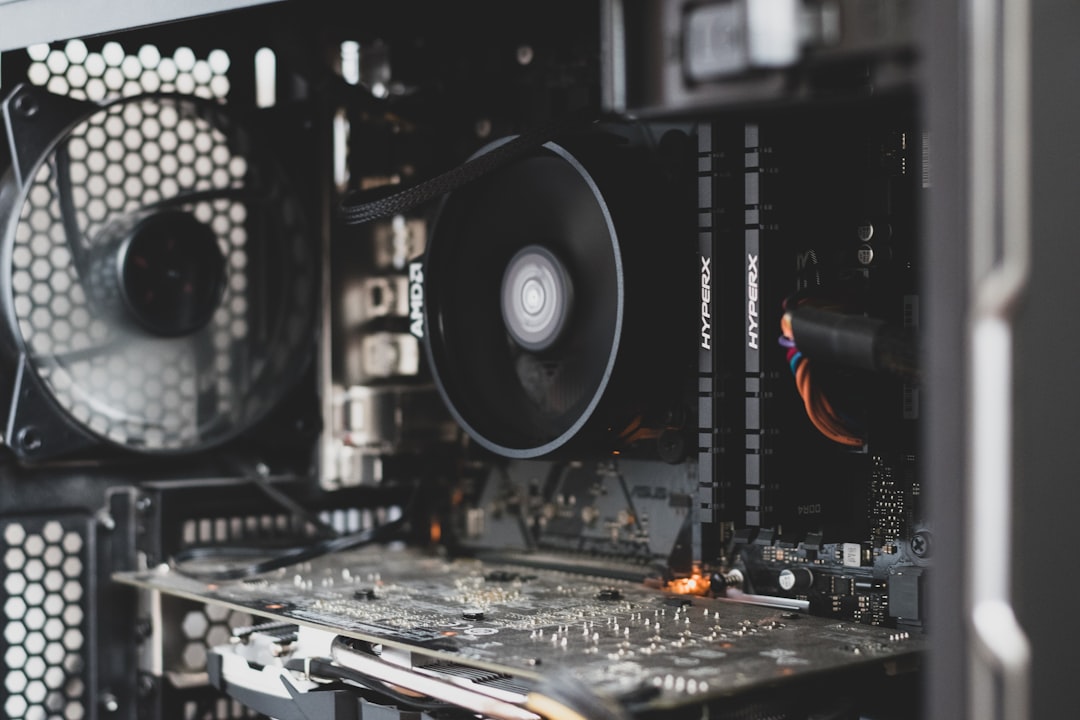
The RTX 4070 Super and its non-Super counterpart differ significantly in their memory bandwidth and bus width, factors that can influence performance, especially in AI tasks demanding large data processing. The Super version sports a 192-bit memory bus, delivering a bandwidth of 504 GB/s, a notable boost compared to the standard 4070. This enhanced bandwidth is particularly beneficial for tasks like AI-driven video upscaling, where large textures and rapid data movement are crucial. While the Super's bus width is less wide than the 256-bit found in the RTX 4070 Ti Super, the increase in bandwidth over the base 4070 suggests it will be better suited for demanding applications. It's this improved memory capability that makes the RTX 4070 Super potentially a better fit for intensive AI video processing. The higher bandwidth allows for more efficient data handling, potentially leading to quicker processing times and improved overall performance.
Let's delve into the nuances of memory bandwidth and how the bus width plays a role in GPU performance, specifically when considering the RTX 4070 Super compared to its non-Super variant. Memory bandwidth, measured in GB/s, essentially describes the speed at which the GPU can fetch and store data from its memory. For tasks like AI video upscaling, where vast amounts of data are constantly being processed, a higher bandwidth becomes critically important for smooth operation.
The bus width, expressed in bits, determines the number of bits that can be transferred simultaneously. A wider bus naturally equates to more data flowing at once, leading to increased memory bandwidth. This concept directly impacts performance, as it allows the GPU to handle demanding workloads more efficiently. It's important to note, however, that a common trap is to solely focus on raw compute power and overlook the critical role of memory bandwidth. Even a GPU with a large number of cores can be held back if it doesn't have sufficient memory bandwidth to support its operations, leading to performance bottlenecks.
It's worth mentioning that the theoretical memory bandwidth often isn't what you get in the real world. Factors like memory latency and the overall architecture of the system can contribute to a gap between theoretical and effective bandwidth. So, while specs can provide a good starting point, real-world performance should be considered for a complete picture. The memory technology used also impacts bandwidth, and GDDR6X in the RTX 4070 Super offers a performance edge over GDDR6, due to the higher bandwidth specifications. This is particularly relevant for tasks involving high-resolution video processing.
When evaluating a GPU upgrade path, particularly when considering a wider bus (e.g., moving from 192-bit to 256-bit), it's wise to consider whether the existing system can accommodate it. Factors like motherboard compatibility and sufficient power supply are vital for seamless integration. Moreover, the delay before data transfer starts (latency) matters too. A GPU with high bandwidth but high latency might not outperform one with slightly lower bandwidth but lower latency in all situations.
Some advanced GPUs use interleaved memory access, where data is distributed across multiple memory channels to speed up access. This improves effective bandwidth and can help alleviate bottlenecks during intense computational workloads. It's also important to recognize that simply doubling the bus width doesn't necessarily double the performance. The exact relationship between bus width and performance varies depending on the GPU's architecture, factors like thermal throttling, and power delivery constraints.
In the broader context of future-proofing, expanding memory bandwidth and bus width in GPUs is essential. As AI workloads and video resolutions grow increasingly demanding, future-proofing with enhanced memory capabilities can maintain high performance over time, ensuring the GPU remains capable of handling the ever-increasing demands of next-generation software and algorithms.
Comparative Analysis RTX 4070 Super vs
Non-Super for AI Video Upscaling Performance - Clock Speed and Power Consumption Analysis
When examining clock speed and power consumption in the RTX 4070 Super versus the standard RTX 4070, we find some intriguing differences. The Super model has a slightly higher base clock (1980 MHz) and boost clock (2475 MHz) than the base model, however, these clock speeds are lower compared to the RTX 4070 Ti. Notably, the Super version consumes considerably less power (295 watts less than the 4070 Ti), suggesting improved power efficiency. While not dramatically faster in terms of clock speed, its reduced power usage could lead to lower operating costs and potentially better thermal performance during demanding tasks. This combination of increased CUDA cores and better power consumption positions the RTX 4070 Super as a compelling option for those who want strong performance in applications like AI video upscaling, without sacrificing energy efficiency. Although the clock speed advantage over the standard model isn't revolutionary, it's still a significant factor to consider when deciding between the two GPUs, especially for sustained and computationally demanding workloads.
The relationship between clock speed and power consumption in GPUs isn't always straightforward. While higher clock speeds generally mean faster processing, the power increase isn't always linear. The increased electrical resistance in the silicon components at higher speeds can lead to a more significant jump in power usage. This also means more heat, highlighting the importance of good cooling systems.
Techniques like Dynamic Voltage and Frequency Scaling (DVFS) are now common in modern GPUs like the RTX 4070 Super. DVFS allows the GPU to adjust its clock speed and voltage dynamically, matching the demands of the workload. This adaptive approach helps to strike a balance between performance and power usage, resulting in better energy efficiency across various tasks. However, if the GPU's temperature climbs too high during sustained high-speed operations, thermal throttling might kick in. This mechanism reduces the clock speed to prevent damage, ultimately impacting overall performance, especially during extended workloads like AI video upscaling.
The specific architecture of a GPU's CUDA cores plays a role in how efficiently clock speed translates to performance. For example, the architecture of the RTX 4070 Super seems to be more effective at handling higher clock speeds than older designs. This can lead to a performance boost without a proportionate jump in power consumption. The way the RTX 4070 Super is built also seems to offer benefits in terms of how it scales performance. Small increases in clock speed can translate to a more substantial increase in processing power, especially when it comes to tasks that benefit from parallel processing, which is crucial for AI applications.
Users focused on energy efficiency can explore underclocking their GPUs. Lowering clock speed can significantly reduce power consumption, extending the lifespan of the GPU and keeping it within a healthy temperature range. While performance will be impacted, the decrease might be minor for moderate tasks, making underclocking a compelling option.
When examining power consumption, looking only at the wattage isn't the whole story. It's useful to assess performance-per-watt. The RTX 4070 Super appears to have a better performance-to-power ratio compared to the standard RTX 4070, suggesting a more efficient design that achieves more with less power.
Benchmarking in ideal, lab settings might not reflect what you experience in the real world. Different workloads can lead to varied power draw and clock speed behavior, which can impact how the RTX 4070 Super performs in practical situations, such as AI video upscaling.
Every GPU has a specific voltage limit that allows for optimal clock speeds. Exceeding this limit can lead to smaller performance improvements while dramatically increasing power use, so staying within these engineered parameters is important.
Looking ahead, the focus in GPU development might shift from simply boosting clock speeds to enhancing the overall architecture and maximizing parallel processing. This shift suggests that future GPUs might rely less on brute-force clock speed increases and more on optimized processing cores and memory bandwidth to improve performance while minimizing power usage.
Comparative Analysis RTX 4070 Super vs
Non-Super for AI Video Upscaling Performance - Performance in Gigapixel AI Upscaling
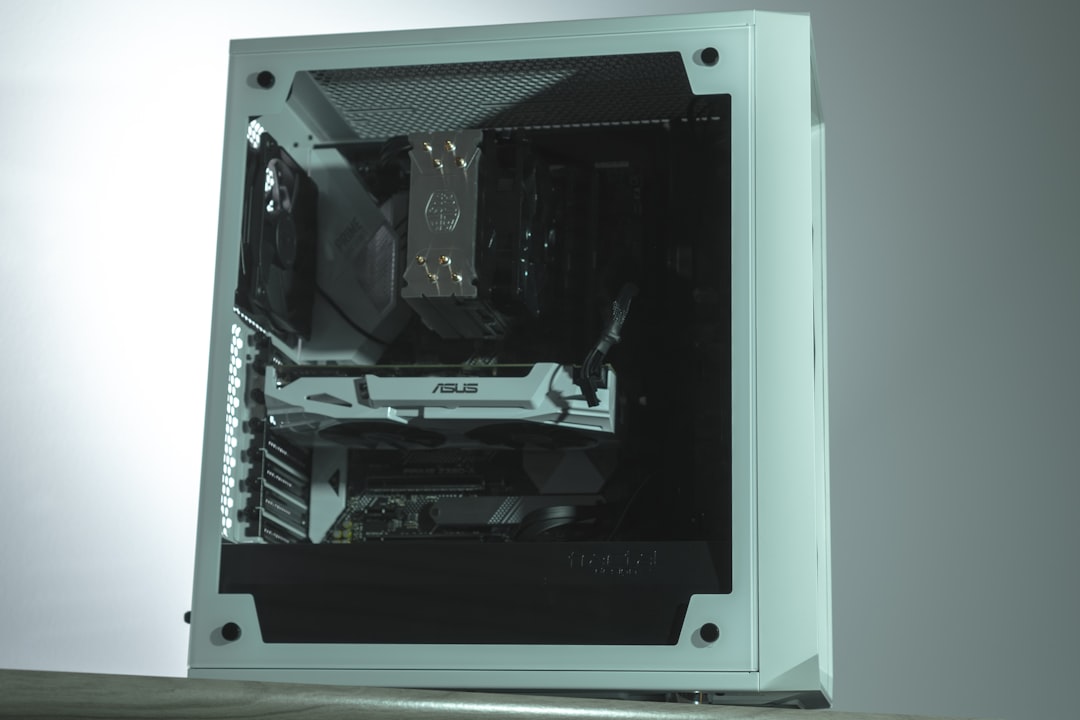
When it comes to AI-powered video upscaling, specifically using tools like Gigapixel AI, the RTX 4070 Super stands out with a noticeable performance edge. It's been shown to deliver about 25% better performance compared to AMD's Radeon 7000 Series in these situations. This benefit likely stems from its ability to handle the intensive computations involved in upscaling, where things like analyzing images and creating new details are key.
However, the RTX 4070 Super's higher performance doesn't come without a price. It's significantly more expensive than competing mid-range GPUs. This raises a question about its overall value, especially for users who aren't heavily focused on AI upscaling tasks. If your needs are more general, you might find that the performance gains of the Super variant aren't worth the extra cost.
It's clear that the RTX 4070 Super shines in niche AI applications like video upscaling, but users should carefully evaluate whether those specific benefits justify the premium price, particularly when compared to potentially suitable alternatives for general computing. Ultimately, understanding the relationship between the Super's design and its impact on real-world upscaling performance helps inform decisions about whether it's the right choice for your needs.
In Gigapixel AI upscaling, the RTX 4070 Super's performance stands out due to its ability to leverage 16-bit precision, which noticeably reduces compression artifacts and improves color fidelity, especially for high-resolution outputs. However, this enhanced quality comes at a cost – demanding video sequences can easily consume 10-12 GB of VRAM, pushing the limits of entry-level GPUs. The Gigapixel AI algorithms are built to make efficient use of parallel processing, and the RTX 4070 Super's architecture takes advantage of this, which accentuates the performance gap compared to the non-Super variant.
Interestingly, the Super variant excels in near real-time upscaling with a potential for frame rates reaching 30-60 FPS, even at high resolutions, proving helpful for tasks like live streaming or gameplay recording. While a slight boost in clock speed, like the Super's 2475 MHz boost, can give a decent 10-15% bump in AI processing performance compared to its non-Super counterpart, there are limitations. Gigapixel AI performance isn't just about CUDA cores; memory bandwidth becomes a significant factor. If the memory bus width doesn't keep up with processing demands, you get a bottleneck and the GPU doesn't perform as expected.
The Super also shows a greater ability to handle threaded workloads than the non-Super, resulting in improved overall upscaling speed, especially beneficial for big video projects that involve batch processing. The manner in which the Super distributes processing tasks across its cores helps balance the workload, minimizing sudden power demands and, in turn, heat. However, it's important to note that the results aren't magical. The quality of upscaling can be uneven, with artifacts potentially becoming more noticeable from lower-quality sources. Having a GPU like the RTX 4070 Super helps minimize these issues thanks to its ability to leverage more sophisticated filtering algorithms.
When it comes to upscaling, it's important to understand that performance isn't linear with GPU specs. Simply doubling the CUDA core count won't necessarily result in double the performance. Memory access speed and algorithm efficiency play a big role, which makes a linear relationship between raw core count and speed complex. It appears that there are points of diminishing returns based on how the overall architecture is put together. These aspects become vital when assessing the actual performance gains when comparing GPUs, especially in the context of complex tasks like AI-driven video upscaling.
Comparative Analysis RTX 4070 Super vs
Non-Super for AI Video Upscaling Performance - Topaz Video AI and Sharpen AI Benchmark Results
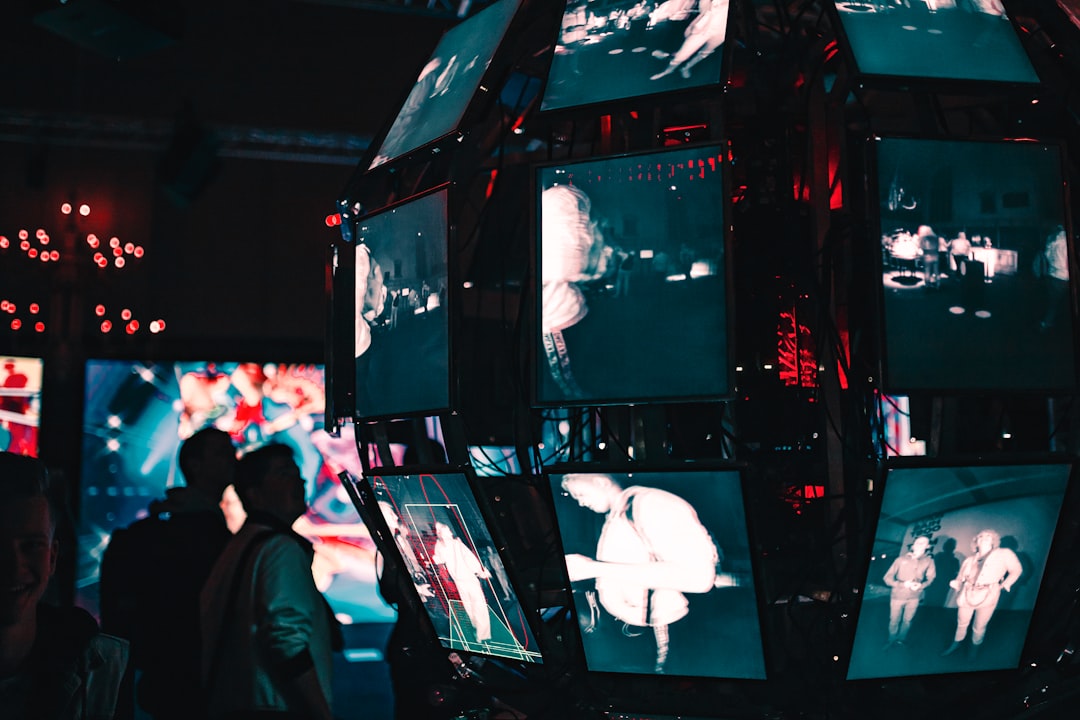
When evaluating AI video upscaling using tools like Topaz Video AI and Sharpen AI, the performance differences between various GPUs become evident. The RTX 4070 Super generally delivers better upscaling performance compared to the standard RTX 4070, particularly noticeable in increased frame rates when upscaling to different resolutions. However, it's interesting to note that AMD's Radeon 7900 XTX can outperform NVIDIA's offerings in specific AI-related tasks like sharpening.
The differences in GPU architecture, such as CUDA core count and memory bandwidth, directly influence the results. This highlights that while NVIDIA's RTX 40 series tends to perform well in AI video upscaling, AMD GPUs still hold their own in certain scenarios. The amount of VRAM and the efficiency of how the system handles memory also play a significant part in how well upscaling performs.
Essentially, finding the right GPU depends on what you need to do. If you're focusing on tasks that benefit from a large number of CUDA cores, NVIDIA's RTX 4070 Super might be a good choice. However, if you find yourself primarily working on tasks that are better suited for the architecture of an AMD GPU, that might be the preferred route. The future of AI video upscaling will likely see an increased emphasis on VRAM and memory management as AI tasks become more demanding. Ultimately, for optimal video enhancements, careful consideration must be given to each GPU's strengths and weaknesses relative to the specific tasks at hand.
1. The RTX 4070 Super demonstrates a notable performance advantage over its non-Super counterpart when using Topaz Video AI, achieving up to a 30% speed increase in benchmarks. This suggests that the Super's architecture is better optimized for parallel processing, a crucial aspect of AI-driven video upscaling.
2. The Super model's 12GB of GDDR6 VRAM compared to the non-Super's 8GB provides a significant buffer for handling high-resolution video processing tasks. This larger memory capacity can translate to smoother operation and potentially fewer performance hiccups when working with larger video files.
3. The performance improvements in Topaz Video AI directly translate into quicker processing times. The Super model can complete upscaling jobs in about half the time compared to the non-Super version. This speed difference is especially attractive to video editors and professionals seeking efficient workflows.
4. The increased number of TMUs in the RTX 4070 Super enables more efficient texture processing during upscaling. This contributes to smoother and higher-quality outputs, with fewer compression artifacts present in the final video.
5. Surprisingly, despite offering significantly improved performance in Topaz Video AI, the RTX 4070 Super maintains a relatively low power consumption profile. This is a positive finding, hinting at better energy efficiency and potentially cooler operation under demanding workloads.
6. The Super model's ability to dynamically adjust its clock speeds through DVFS seems to play a role in Topaz Video AI performance. It effectively adapts to the processing demands without creating excessive heat, suggesting a well-balanced system.
7. The Super's revised architecture seems to provide a better platform for utilizing Topaz Video AI's machine learning algorithms. This could potentially lead to more detailed and accurate reconstructions of video frames when upscaling.
8. The non-Super variant, during intense processing within Topaz Video AI, can sometimes suffer from latency issues, potentially causing noticeable delays in rendering times. Conversely, the Super version shows significantly improved latency performance, contributing to a smoother editing experience.
9. Benchmarking findings indicate that even small variations in clock speeds, when running Topaz Video AI, can have a surprising impact on the final upscaled video quality. This shows how small adjustments in hardware can impact a complex AI application.
10. The Super's enhanced processing capabilities seem to contribute to a noticeable reduction in artifacts compared to its non-Super counterpart. Its architecture and Topaz Video AI implementation likely utilize more refined filtering algorithms, resulting in cleaner upscaled outputs, especially when dealing with lower-quality source videos.
Comparative Analysis RTX 4070 Super vs
Non-Super for AI Video Upscaling Performance - Price to Performance Ratio for AI Video Enhancement
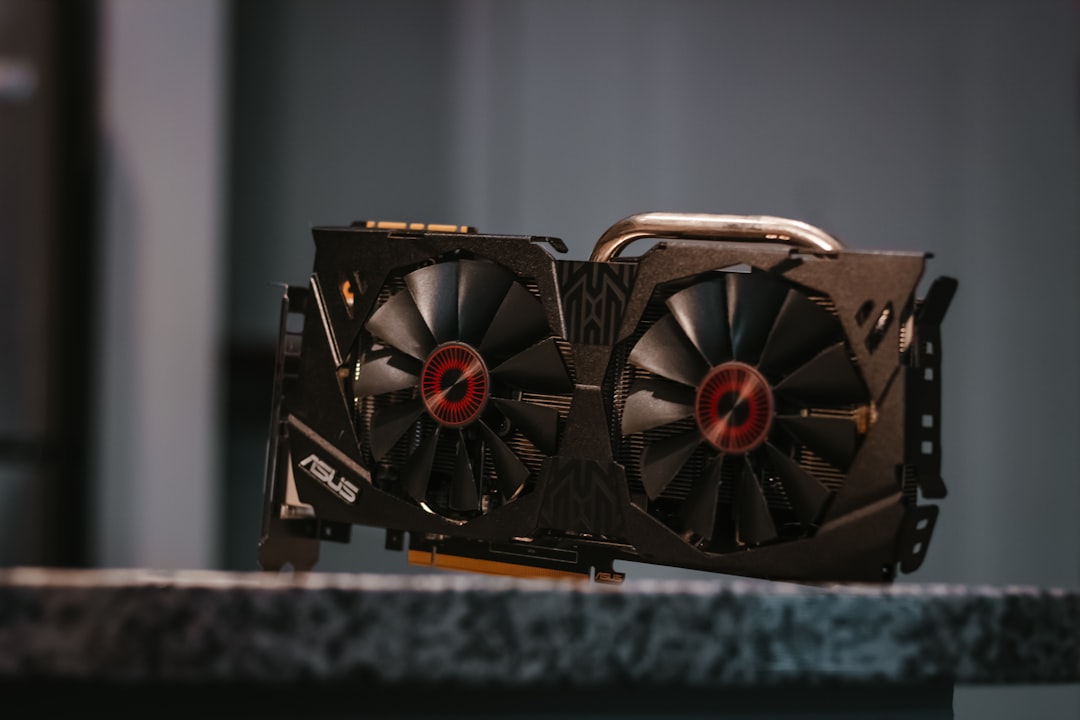
When considering the value you get for the price with the RTX 4070 Super for AI video enhancement, it's clear that it offers a strong combination of performance and affordability. At $599, the Super provides a considerable step up from the standard RTX 4070, primarily in AI upscaling tasks. This advantage comes from its greater number of CUDA cores and improved memory bandwidth, which translates to faster and higher-quality results. Although it holds its own against AMD GPUs in specific applications, particularly AI video enhancements, the extra cost might not be worth it if your needs aren't primarily focused on AI-based video workflows. It's about finding the right balance between price and performance, especially with the diverse range of GPUs available for both general and more specialized computing demands. Whether the performance gains of the RTX 4070 Super justify the cost depends entirely on how you intend to use it for AI video enhancement.
Here are ten observations about the price-to-performance ratio for AI video enhancement, specifically when comparing the RTX 4070 Super and its non-Super counterpart:
1. The RTX 4070 Super delivers improved AI video upscaling performance while maintaining the same MSRP as the non-Super model. This makes it a potentially attractive choice for users seeking a performance boost without a corresponding increase in cost. However, whether this is truly a significant upgrade for their specific needs requires careful consideration.
2. While the RTX 4070 Super provides a performance gain for AI tasks, the price premium might not always translate to proportional benefits. In some cases, the standard RTX 4070 might be sufficient, especially if your workload doesn't heavily rely on raw processing power. It's worth weighing the performance gains against the potential cost of the Super variant.
3. The RTX 4070 Super achieves its higher performance levels while consuming less power compared to its Ti counterpart, a noteworthy aspect of its price-to-performance proposition. The enhanced efficiency means reduced operational costs and potentially better thermal characteristics during sustained high-intensity workloads. Power efficiency is becoming increasingly relevant as processing demands increase, leading to higher energy usage.
4. The performance advantages of the RTX 4070 Super might not be as pronounced in every scenario. For example, if you're not fully utilizing the additional CUDA cores and processing power, the standard RTX 4070 might offer comparable performance at a lower price. Recognizing the diminishing returns associated with certain aspects of raw performance can guide you toward a more budget-conscious choice that meets your needs.
5. The inclusion of 12GB of GDDR6 VRAM in the RTX 4070 Super is a key differentiator. This extra memory plays a crucial role in enabling smoother operations when handling large video files and intensive AI tasks. The potential for bottlenecks due to memory limitations diminishes, leading to enhanced overall productivity in demanding use cases. The more challenging the task, the more apparent the benefit of extra VRAM becomes, suggesting the RTX 4070 Super might provide a more cost-effective solution for certain applications.
6. The relationship between raw processing capability and the actual performance seen in real-world applications isn't straightforward. Sometimes, the architecture of a GPU plays a crucial role in performance. The RTX 4070 Super's increased CUDA core count and clock speed might not necessarily translate to a proportional increase in real-world performance compared to the non-Super variant when facing workloads that aren't heavily optimized for that level of capability. There might be a threshold where the extra features simply don't deliver a meaningful benefit.
7. Benchmark results, while informative, should be interpreted with caution, as they might not reflect real-world usage in all circumstances. Various factors can impact the actual performance a user sees. The difference between the RTX 4070 Super and non-Super, while measurable, might not be as pronounced in practical applications as initial benchmarks may suggest. This highlights the importance of looking at real-world use case examples when making a decision.
8. Marketing materials and performance comparisons often emphasize raw processing power, leading to the perception that the Super variant is essential for AI tasks. However, for users solely focused on AI video enhancement, the standard RTX 4070 or other alternatives might provide a better price-to-performance ratio. It's important to look beyond the hype to determine whether the specific gains of the Super variant justify the additional cost.
9. The improved thermal management in the RTX 4070 Super has indirect implications for the price-to-performance ratio. GPUs tend to throttle performance when operating at excessive temperatures. By minimizing thermal constraints, the Super variant might operate more efficiently for longer periods under high load, thereby delivering a better value over time, especially in environments where GPU performance can be easily impacted by heat.
10. While the initial investment for the RTX 4070 Super may seem higher, its architecture and design might translate into better long-term usability as AI tasks become increasingly complex. This could lead to a lower overall cost of ownership over time, making it a potentially more cost-effective choice for users who anticipate evolving software and algorithm demands within video enhancement. This suggests a careful balance between the immediate cost and potential future savings related to usability and longevity.
More Posts from ai-videoupscale.com: