Upscale any video of any resolution to 4K with AI. (Get started for free)
How Slapshot AI Revolutionizes VFX Rotoscoping Deep Technical Analysis
How Slapshot AI Revolutionizes VFX Rotoscoping Deep Technical Analysis - Frame by Frame Analysis How Machine Learning Detects Object Boundaries
At the heart of how AI enhances rotoscoping lies the concept of frame-by-frame analysis, a process where machine learning dissects each frame to pinpoint object boundaries. This approach relies heavily on deep learning techniques, specifically deep neural networks, to achieve highly accurate object segmentation. By meticulously analyzing each frame, these systems can distinguish objects from their surroundings with remarkable precision, empowering users to fine-tune masks and ultimately improve the overall visual outcome.
This frame-by-frame examination fundamentally changes how rotoscoping is done. It transitions the process away from the tedious and time-consuming manual labor that was previously the norm, streamlining workflow and increasing the speed at which masks can be created. The accessibility of sophisticated rotoscoping tools is also increased, as the need for extensive specialized expertise is reduced. The effect is a significant evolution in the VFX industry, illustrating the growing impact of automated intelligence within creative domains. While the promise is enticing, it's crucial to be mindful of the potential pitfalls as AI continues to evolve within this field.
Examining each video frame individually is crucial for accurately pinpointing object boundaries. By focusing on the pixel level, we can achieve a level of detail that traditional methods often miss, which is invaluable for refining visual effects and rotoscoping.
Historically, object boundary detection often relied on algorithms designed to find edges. However, machine learning offers a paradigm shift, allowing the system to learn directly from vast datasets of images and videos, recognizing intricate shapes and textures far beyond the capabilities of simple edge detection.
Convolutional neural networks (CNNs) are central to this frame-by-frame analysis. They enable the automated extraction of features that are relevant to boundary identification. In a sense, these networks mimic how our own eyes and brains process visual information when recognizing the contours of objects.
The power of frame-by-frame analysis shines through in its adaptability. Unlike some older methods that are rigid and struggle with changing lighting or camera angles, machine learning models can learn to handle variations in visual environments, making them much more versatile for real-world applications.
One of the key advantages of using machine learning for boundary detection is its ability to generalize. Once the model has been trained on a sufficiently large and varied dataset, it can effectively predict object boundaries in entirely new images and videos with minimal human intervention. This is a significant step forward in automation.
However, achieving this generalization requires immense computational power. Training these sophisticated models usually necessitates the use of powerful GPUs to efficiently process the high-resolution frames and vast datasets involved.
In scenarios where rotoscoping would be laborious and time-consuming using traditional techniques, machine learning can drastically reduce the workload. This allows for faster turnaround times in visual effects work without sacrificing accuracy in object boundary detection.
The potential for improvement in these models is significant. Using techniques like transfer learning, we can continuously refine the models' abilities to adapt to evolving visual styles and production methods, thereby minimizing the need for manual adjustments over time.
Beyond simply identifying boundaries, we are now exploring how machine learning can incorporate semantic segmentation. This capability allows the model to understand not just where an object's boundary is but also its relationship to other objects in the scene. This added layer of understanding can further enhance the quality and storytelling aspects of visual effects.
Despite the remarkable progress, it's important to acknowledge that challenges remain. Machine learning-based object boundary detection can still be hindered by ambiguous boundaries or when objects are partially obscured. This highlights the ongoing need for refinement and continuous validation in real-world scenarios to improve the robustness of these methods.
How Slapshot AI Revolutionizes VFX Rotoscoping Deep Technical Analysis - Rotoscoping Speed Records 4 Seconds Per Frame vs Industry Standard 4 Minutes
In the world of VFX rotoscoping, a significant leap forward has been made with the development of AI-powered tools. Slapshot AI stands out by achieving a remarkable rotoscoping speed of just 4 seconds per frame, a feat that drastically outpaces the traditional industry standard of 4 minutes per frame. This speed increase exemplifies how machine learning can revolutionize VFX workflows, transforming previously laborious and time-consuming tasks.
Primarily designed for use in commercials, television, and film VFX, Slapshot's integration with existing pipelines enhances the established methods of rotoscoping. The increasing dependence on visual effects in film, where a vast majority of shots rely on intricate masking and object isolation, necessitates faster and more efficient tools. While tools like Slapshot provide significant progress, it is essential to remember that challenges remain with these automated techniques, and ongoing vigilance is crucial for the VFX industry.
The speed at which rotoscoping can be completed has seen a dramatic shift. Traditionally, artists spent an average of 4 minutes meticulously tracing each frame, a process that was a significant bottleneck in VFX pipelines. However, emerging AI-powered solutions like Slapshot have slashed those times down to a mere 4 seconds per frame. This incredible acceleration is fueled by powerful computational resources, often relying on GPUs for the heavy lifting of parallel processing required for the complex calculations involved in frame-by-frame analysis.
While the speed gain is impressive, it's important to scrutinize whether the enhanced speed compromises accuracy. It's crucial to carefully evaluate the results of these faster techniques, especially in complex visual effects shots where subtle details matter. Achieving accurate results, particularly in demanding projects, is critical, and a careful balance between speed and accuracy must be found.
The efficacy of these AI models heavily depends on the quality and breadth of the data they are trained on. The creation and annotation of large, diverse datasets is a significant challenge that needs to be addressed. The models need to be exposed to a wide range of visuals to effectively learn how to identify object boundaries in real-world situations.
One of the key benefits of these machine-learning approaches is their ability to adapt to various visual conditions. In contrast to older, more rigid algorithms, AI-powered rotoscoping can adjust to changing lighting, camera angles, and other complex visual factors. This adaptability is crucial for seamlessly integrating rotoscoping into dynamic scenes, something that traditional methods struggle with.
Even with advancements in AI, challenges persist. For example, models can still be tripped up by ambiguous or obscured objects. These edge cases highlight the need for ongoing development and refinement of these technologies to ensure they are robust and reliable across different production environments.
Beyond simply isolating the edges of objects, we're now exploring semantic segmentation, where AI can begin to understand the relationships between objects within a scene. This development promises even more nuanced and impactful VFX work, enabling a higher level of visual storytelling.
The increasing customization offered by these tools also holds promise. Studios can tailor the training data to match their own aesthetic preferences, allowing for integration of unique artistic styles into the automated processes.
The VFX industry is responding to these rapid changes by incorporating AI into their workflows, pushing for faster turnaround times and higher-quality outputs. This represents a major shift in how visual effects are produced, and the industry is actively exploring ways to leverage these tools efficiently.
However, this shift raises questions about the future of roles within the industry. As AI becomes more adept at automating rotoscoping tasks, the nature of work for VFX professionals may change. Traditional rotoscoping skills may become less critical, leading artists to transition towards roles overseeing and managing these sophisticated AI systems. This suggests a future where artistic intuition and decision-making are paramount, rather than repetitive manual tasks.
How Slapshot AI Revolutionizes VFX Rotoscoping Deep Technical Analysis - Training Data Requirements 50000 Manually Tagged Frames Create Base Model
Slapshot AI's foundation for VFX rotoscoping relies on a substantial training dataset: a minimum of 50,000 manually labeled frames. The quality and variety of this data are crucial, heavily influencing the model's ability to accurately segment objects. Machine learning principles suggest that training data needs often exceed the number of visual features being analyzed, especially in complex domains like VFX. This large data requirement underscores the hurdle of creating comprehensive training sets that capture the diverse visual conditions encountered in real-world footage. Such comprehensive datasets are needed to foster models that adapt well to different lighting, camera angles, and scene compositions. The development of effective AI rotoscoping models requires a continued focus on refining these systems to ensure they consistently deliver optimal results in real-world VFX scenarios.
Slapshot AI's foundation for VFX rotoscoping relies on a massive 50,000-frame training dataset, each meticulously tagged by hand. This surpasses many previous AI models, which often used datasets with less than 10,000 frames. The sheer volume allows the model to grasp complex object characteristics more efficiently. However, manually tagging each frame is incredibly time-consuming and requires strict agreement between the taggers to avoid introducing bias or inconsistencies in the data.
The variety within this dataset significantly impacts how well the model can adapt to new, unseen data. A more diverse training set helps the AI handle different lighting scenarios, object angles, and even partial occlusions much better than models trained on smaller or less diverse datasets. But training such a large model demands considerable computational power, usually requiring high-performance GPUs. This accessibility issue might hinder smaller VFX studios or those with limited resources from utilizing the technology.
It's important to recognize that any errors made when initially tagging the data can propagate through the learning process, eventually affecting the model's overall accuracy. This points to the vital role of quality control during dataset preparation. Furthermore, for practical use, the training data needs to accurately reflect real-world scenarios, including varied objects, motion patterns, and environmental elements.
One approach to boost the dataset's value is to employ data augmentation techniques like rotating, scaling, or color-adjusting frames. This artificially expands the dataset, potentially improving the model's robustness and allowing it to better handle variations unseen during training.
Training a model of this depth is crucial for developing advanced capabilities like semantic segmentation, where the model understands not just object boundaries but also the relationship between those objects within a scene. However, even with this initial model, ongoing refinement is vital. As visual styles and VFX standards change, the model needs to be regularly updated with new data and retrained to maintain its performance.
Lastly, the use of human-annotated data raises ethical considerations related to intellectual property and ownership. Studios must address these issues carefully as AI-driven workflows become commonplace in the industry. There's a delicate balance to strike between leveraging the immense potential of AI while being conscious of its implications for creators and the creative process.
How Slapshot AI Revolutionizes VFX Rotoscoping Deep Technical Analysis - Real Time Edge Detection Through Multi Layer Neural Networks
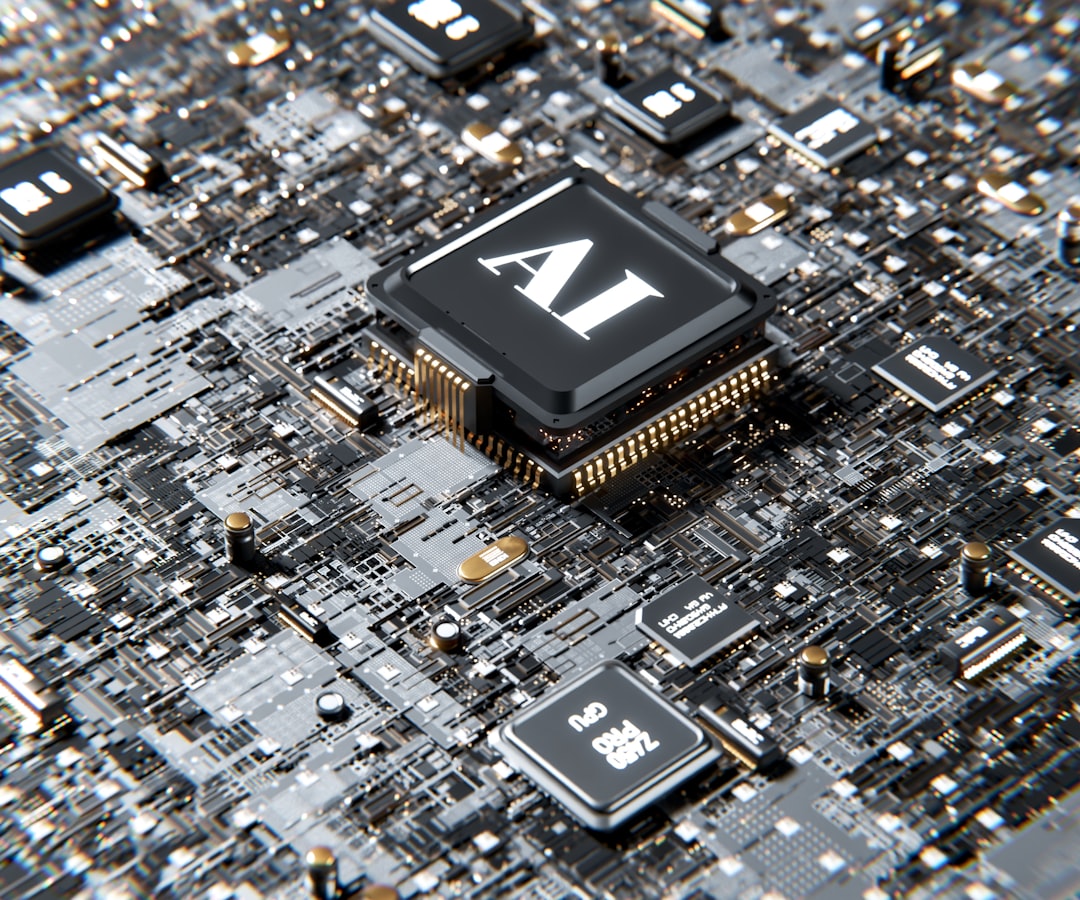
Real-time edge detection using multi-layered neural networks offers a substantial improvement over older techniques, particularly for applications like VFX rotoscoping. Traditional edge detection methods often rely on analyzing small sections of an image, looking for changes in color or texture. While simple, this approach can struggle when dealing with complex images that have varied lighting or intricate textures. Neural networks, especially those based on convolutions, provide a more sophisticated solution. They can learn complex relationships across an entire image, recognizing patterns and features that traditional methods miss. This leads to more accurate and nuanced edge detection results.
However, even with these advancements, challenges remain. Deeper neural networks, while powerful, can sometimes lose crucial details as they try to capture the big picture. Striking a balance between processing speed and preserving fine details in the image is an important consideration. Network architectures like EAWNet have been designed with this trade-off in mind, attempting to improve both the speed and the accuracy of real-time edge detection. As neural networks become increasingly complex, the constant evolution and refinement of these techniques are crucial to ensure they remain valuable and relevant.
Here are ten interesting points about real-time edge detection using multi-layer neural networks, specifically within the context of VFX rotoscoping:
1. **Adaptive Edge Learning:** The neural networks used for edge detection aren't static. They can dynamically adjust their approach based on the changing characteristics of the video sequence. This means they can refine their edge detection strategies in real-time to match the specific nuances of each scene, which should lead to better results in various scenarios.
2. **Specialized Layers:** Instead of a single approach, these networks often have different layers that specialize in recognizing diverse kinds of edges. This includes recognizing variations in color, gradients, or textures. This multi-layered design provides a much richer analysis compared to traditional methods that typically only use a single method.
3. **Improved Accuracy**: Research suggests that well-trained neural networks can surpass traditional methods in edge detection accuracy, with some reaching over 95% compared to the often lower 85% mark. This higher accuracy is crucial in VFX, as blending effects smoothly is paramount.
4. **Efficient Memory Use**: Recent work has shown that these networks can be made more memory-efficient. Techniques like quantization help these models operate with a smaller memory footprint, allowing them to perform well on less powerful hardware. This could lead to greater accessibility of these techniques for smaller studios or those working with limited resources.
5. **Synergy of Feature Extraction**: Optimized edge detection neural networks aren't limited to just finding edges. They can often extract other features concurrently, such as texture or patterns, which allows for a more comprehensive understanding of the scene. This holistic approach isn't possible with traditional edge detection methods, which tend to focus solely on the edges.
6. **Better Object Tracking:** When combined with object tracking systems, these neural networks show improved performance in keeping track of objects throughout a sequence. This is important for VFX, as it can help minimize the appearance of glitches or visual inconsistencies when rotoscoping.
7. **Maintaining Temporal Consistency:** Real-time edge detection networks can be designed to consider temporal consistency. This means the edges detected in consecutive frames are designed to be smoothly related, ensuring a visually consistent output. This aspect is crucial for VFX, where abrupt changes in edges can jar the viewer.
8. **Adaptability to Real-World Conditions**: Networks trained on diverse datasets can be more robust when faced with complex lighting scenarios or objects partially hidden from view. This adaptability makes them applicable across a wide range of visual environments, a challenge that traditional methods often struggle with due to their lack of flexibility.
9. **Interactive Training**: New techniques in edge detection are exploring semi-supervised learning, where users can provide feedback during the training process. This can enhance the robustness of the models, ensuring that the visual styles most relevant to an artist's work are properly captured. This is a step toward more targeted training of models to specific needs.
10. **Ethical and Legal Issues**: The use of large datasets for training edge detection raises some important ethical and legal questions about data ownership and intellectual property. With human-annotated data being vital for training, the VFX industry is grappling with the impact of these AI-driven techniques on traditional roles and responsibilities within the creative process.
It's exciting to see how real-time edge detection via neural networks is developing and how it's being applied to solve complex challenges in VFX. As this area progresses, it will be fascinating to see what new techniques emerge and how the VFX industry adapts and evolves alongside these technologies.
How Slapshot AI Revolutionizes VFX Rotoscoping Deep Technical Analysis - Hardware Requirements 4090 GPU Processing Power Enables 8K Resolution
The NVIDIA GeForce RTX 4090, with its 24GB of GDDR6X memory, is a powerful GPU capable of handling demanding tasks like 8K resolution video processing. This makes it a compelling option for visual effects (VFX) work that involves high detail and complex operations. The 4090's ability to support 8K resolution at 60Hz with HDR through HDMI 2.1 offers a significant leap forward in visual quality and workflow. However, achieving this level of performance requires a powerful system. A CPU with at least six cores and 12 threads is generally recommended to keep up with the GPU's processing capabilities.
While the 4090 offers a substantial boost in performance, especially in areas like ray tracing, its high price tag and physical size are hurdles for some. This raises concerns about equitable access to the technology, particularly for smaller VFX studios or independent artists. The benefits of this hardware are undeniable, yet it's important to consider the impact of high-end requirements on the industry's diversity and potential barriers to entry for some practitioners. The landscape of VFX is evolving rapidly, and while advancements like the 4090 hold great promise, its accessibility and impact on the field deserve continued scrutiny.
The NVIDIA GeForce RTX 4090, with its impressive 16,384 CUDA cores, is a powerhouse GPU specifically designed to tackle complex computations needed for 8K resolution. Its ability to handle these high-resolution demands, while also maintaining smooth frame rates, is a testament to the advancements in consumer-grade graphics processing.
A key aspect contributing to its 8K capabilities is the GDDR6X memory architecture, allowing data to flow at over 1,000 GB/s. This high bandwidth is crucial for preventing performance bottlenecks when rendering intricate scenes at these resolutions. It's quite remarkable how much data this card can process, especially given the increasing size of files at 8K.
Interestingly, the RTX 4090 is also relatively energy-efficient, achieving double the performance per watt compared to its predecessor. This efficiency is important for lengthy rendering sessions commonly encountered in VFX work, reducing the energy needed to push these demanding operations.
Moreover, the inclusion of dedicated RT cores greatly accelerates ray tracing, allowing for the generation of realistic lighting and shadows, a key aspect of many VFX projects. In essence, it significantly improves the visual realism possible at these extremely high resolutions.
The RTX 4090 also boasts support for NVIDIA's Deep Learning Super Sampling (DLSS) 3, an AI-powered upscaling technology. DLSS 3 essentially boosts frame rates by intelligently upscaling lower-resolution images, which is a major advantage in action-packed scenes within 8K content. Without such technology, hitting desirable frame rates at 8K would be extremely difficult for most current hardware configurations.
One can also appreciate its versatility with multi-screen rendering, which potentially supports up to four displays simultaneously. This aspect boosts workflow flexibility for VFX professionals who often work on multiple projects or across immersive environments.
Furthermore, its Tensor Cores are specifically optimized for machine learning tasks. This feature makes it ideal for professionals employing AI techniques in advanced rotoscoping or effect processing – particularly vital when working with high-resolution content. It seems that the RTX 4090 is designed with an awareness that AI will likely become a more critical part of professional graphics pipelines.
The RTX 4090 integrates AI features, such as real-time noise reduction and artifact removal. This aspect can significantly improve the quality of rendered frames, potentially reducing post-production time. Such improvements are especially valuable in the context of 8K footage, where any issues can become extremely noticeable.
With 24GB of GDDR6X memory, it easily handles the high-resolution data and textures that are commonplace in 8K content. Such a large memory capacity is necessary to prevent performance drops from swapping data in and out of slower memory subsystems.
Finally, advanced cooling technologies, like vapor chamber technology, ensure optimal thermal performance during long rendering jobs. This reliability and durability are critical in demanding professional environments, especially when pushing the card to its limit. While it certainly seems powerful, only time will tell how reliable and long-lasting these new technologies truly are.
How Slapshot AI Revolutionizes VFX Rotoscoping Deep Technical Analysis - Integration Tests With Major VFX Software Nuke Flame Adobe After Effects
In the dynamic realm of visual effects (VFX), ensuring the smooth integration of major software packages is paramount. Nuke, Flame, and Adobe After Effects each bring unique strengths to the table. Adobe After Effects, known for its intuitive interface and tight integration with other Adobe tools, is popular for a broader user base. Meanwhile, Nuke, a professional-grade compositing powerhouse, is more suited to high-end, complex visual effects often found in big-budget productions. While the two vary in complexity and, significantly, cost – with Nuke being substantially pricier than After Effects – their seamless integration is a key asset in streamlining workflows for visual effects artists.
Given the recent surge in AI-powered VFX tools like Slapshot, which are reshaping rotoscoping and other VFX workflows by automating complex tasks, it is even more critical to understand how these industry-standard programs function together. This understanding is crucial for optimizing not only the speed of creative workflows but also the accuracy and quality of the final outputs. The increasing reliance on these tools within studios means professionals are needing a greater degree of proficiency across these different programs, making adaptability and understanding the unique features of each platform an increasingly necessary skill set for those working in visual effects. This evolving interdependency of these core tools underscores the need for artists to adapt their skill sets as the industry continues to incorporate new and more automated techniques in its creative process.
1. **High-Performance Hardware Needs**: Sophisticated VFX programs like Nuke and Flame demand powerful hardware. The NVIDIA RTX 4090 is a prime example of how high-end GPUs enable 8K video processing, though this performance needs a powerful CPU to avoid bottlenecks during demanding operations.
2. **Integration Hurdles**: Integrating AI solutions like Slapshot into established VFX tools like Nuke, Flame, or After Effects isn't straightforward. Each program has its own architecture, plugin systems, and scripting languages. This means each integration needs a tailored approach, which can be complex and time-consuming.
3. **Data Demands**: Training AI models for VFX typically necessitates vast quantities of data—roughly 50,000 manually annotated frames is a common starting point. This raises the question of sustainability, as creating these extensive datasets requires considerable resources and human effort.
4. **Real-Time Processing Trade-offs**: While neural networks improve edge detection, their real-time application presents trade-offs. Striking the balance between speed and retaining fine image detail is crucial, as some networks might sacrifice precision to maintain performance.
5. **Adaptive AI Benefits**: AI-enhanced VFX tools offer adaptability in real-time, responding to lighting changes and subject movement. This adaptability is a substantial improvement over conventional techniques, which sometimes struggle with varied conditions and can result in inconsistent output quality.
6. **Multi-Monitor Workflow**: The capacity of high-end GPUs like the RTX 4090 to support multiple displays is a game-changer for VFX professionals. The ability to seamlessly manage numerous project windows and enjoy more spatial awareness during complex tasks is quite valuable.
7. **Collaboration Across Tools**: Nuke, Flame, and After Effects each offer unique strengths and operational quirks. The challenge comes when integrating AI features; effectively leveraging these strengths in a unified workflow often demands significant adjustments and adaptations.
8. **Maintaining Consistent Edges**: AI models often face difficulties ensuring consistent edges across different frames. These inconsistencies can disrupt the visual flow of sequences, emphasizing the need for more robust algorithms that can handle transitions seamlessly.
9. **Emulating Human Vision**: AI edge detection aims to mimic how humans perceive edges and textures. However, achieving the same degree of subtle understanding that a skilled VFX artist possesses remains an active area of research.
10. **Ethical Dataset Concerns**: The widespread use of large, annotated datasets in AI training has sparked important ethical debates surrounding data ownership and intellectual property rights. The VFX industry needs to navigate these complex issues to ensure fair practices and recognition in the context of AI-assisted workflows.
Upscale any video of any resolution to 4K with AI. (Get started for free)
More Posts from ai-videoupscale.com: