How AI Video Upscaling Can Reduce Video Overlay Processing Times by 47%
How AI Video Upscaling Can Reduce Video Overlay Processing Times by 47% - AI Models Cut Video Processing Time From 12 to 4 Hours During Adobe Max 2024
During Adobe Max 2024, a demonstration of Adobe's new AI models showcased a dramatic decrease in video processing times. What previously took 12 hours was reduced to a mere 4 hours. This speed boost is a result of incorporating artificial intelligence into the video editing process. It's part of a wider movement in video editing where AI, including systems like Firefly and VideoGigaGAN, are streamlining workflows and allowing editors to accomplish tasks more quickly. Upscaling capabilities and the ability to seamlessly edit objects within videos are becoming more accessible. The goal is to shift the emphasis from tedious technical work to creative exploration and collaboration. But there are still caveats: current AI video tools have limits on resolution and the minimum length of videos that can be processed, which could potentially restrict the freedom of some editors.
At Adobe Max 2024, a demonstration highlighted how AI models drastically reduced video processing times. We saw a reduction from a lengthy 12 hours down to just 4, representing a 66% decrease. This speed-up points towards significant breakthroughs in how we optimize and process video data.
These AI models rely on intricate neural networks capable of analyzing and improving visual information at a remarkable pace. This means that we can now process video significantly faster without sacrificing the quality of the output. They achieve this speed by employing frame interpolation, a clever technique that creates extra frames to streamline the processing workflow, effectively reducing the number of individual frames that need to be processed.
Interestingly, machine learning plays a crucial role here. It predicts and fixes common flaws and artifacts typically found in lower-resolution video. This automated correction helps to cut down on the amount of time spent manually editing and reprocessing. The accessibility of AI tools has the potential to empower a wider community of creators. Now, smaller content producers don't necessarily need to be burdened by long processing times and expensive software to produce their videos.
A challenge facing traditional video processing methods comes with increasingly high video resolutions. As we move from standard 1080p to 4K and beyond, traditional methods struggle to keep pace. However, AI algorithms have a distinct advantage in this area: they can effectively adapt to these higher resolutions without experiencing a corresponding increase in processing times.
AI's ability to analyze massive datasets of video content allows it to recognize patterns and features that might be beyond the reach of human editors. This knowledge allows AI models to automate processes in a way that leads to more efficient editing workflows. This efficiency extends beyond just time savings. It also signifies that we may need less powerful computing hardware to achieve the same results, thus potentially reducing processing costs.
Preliminary experiments involving Generative Adversarial Networks (GANs) have hinted at an exciting future where AI can significantly improve video quality while cutting down on the need for human intervention during editing. As we refine and develop these AI tools, it is conceivable that we will be able to receive real-time feedback during the editing process itself. This could drastically accelerate the video editing process and create further time savings in the coming years.
How AI Video Upscaling Can Reduce Video Overlay Processing Times by 47% - Frame Interpolation Technique Doubles Video Frame Rate While Using 44% Less GPU Power

Recent advancements in frame interpolation techniques have shown a notable improvement in video processing efficiency. This approach, which essentially creates new frames between existing ones, manages to double the video frame rate while concurrently using 44% less GPU power. The technique achieves this by focusing on motion estimation at a lower resolution, particularly beneficial when dealing with high-resolution videos like 4K. This reduced resolution approach optimizes the interpolation process, making it more efficient for tasks like video editing, surveillance system enhancement, and video restoration. Furthermore, with newer generative models minimizing visual inconsistencies during the interpolation process, this method has the potential to become a valuable asset for diverse multimedia applications. This development could lead to a more streamlined and readily available method for creating high-quality video content for a wider range of content producers.
Frame interpolation is a technique that aims to generate new frames between existing ones, effectively doubling the frame rate and creating a smoother, more fluid viewing experience, especially in fast-action video content. It operates by analyzing the motion patterns in a video sequence and then filling in the gaps with synthetic frames.
Interestingly, recent advancements have enabled frame interpolation to achieve substantial gains in GPU efficiency. It appears that the computational burden can be reduced by 44% by cleverly handling the frame processing workflow. This is encouraging because it suggests that we might not need the most powerful hardware to achieve high-quality results with this technology, which is a cost-effective advantage.
One of the biggest hurdles with this approach is preserving temporal consistency, making sure the interpolated frames look like they belong naturally in the sequence. Advanced algorithms are being developed to minimize visual artifacts, such as abrupt jumps or distortions, that can be jarring for the viewer. These algorithms aim for a natural transition from frame to frame, matching the smoothness that experienced human editors might painstakingly achieve.
Frame interpolation also holds promise for real-time applications like live streaming. Being able to process and double the frame rate on the fly would greatly enhance the streaming experience, especially with bandwidth constraints or for viewers on mobile devices.
From a content creation perspective, the smoother motion facilitated by frame interpolation allows for efficient storytelling. Creators can capture fewer, more impactful frames and still produce visually appealing results, leading to a more focused approach to video production.
Machine learning is playing a critical role in the development of more refined frame interpolation techniques. By learning from massive datasets of videos, AI algorithms can adapt and improve their predictive capabilities, which in turn leads to higher quality interpolation. This automated learning process makes the technology even more versatile across various formats, including the emerging 8K standards.
Frame interpolation can be a powerful tool for streamlining post-processing workflows. It automates a significant chunk of the manual effort usually required to smooth out transitions between frames, reducing the need for time-consuming and potentially tedious adjustments by human editors.
However, benchmarking frame interpolation can be challenging. It relies heavily on comparisons with high-quality reference frames, which can create inconsistencies in performance depending on the quality and characteristics of the input video. As such, further development needs to focus on robustness, particularly in environments where the frame characteristics are unpredictable.
Despite advancements, one risk associated with frame interpolation is the introduction of visual artifacts like ghosting or blurring, particularly when the video content features rapid or complex motion. While researchers are working on mitigating this problem, it remains an area where ongoing refinement is essential to ensure quality and consistency in the interpolated frames.
In summary, frame interpolation has the potential to revolutionize video quality and efficiency. It's a technique that is constantly being refined to create smoother, more appealing videos while requiring less computational power. Although there are some challenges to be addressed, its ability to enhance video smoothness and reduce GPU usage makes it a promising area for future research and development.
How AI Video Upscaling Can Reduce Video Overlay Processing Times by 47% - Real Time 4K to 8K Upscaling Now Possible on Consumer Graphics Cards
Consumer-level graphics cards can now upscale video from 4K to 8K in real-time, a significant development driven by artificial intelligence. These advancements allow graphics cards to intelligently improve the quality of lower resolution content, offering a more accessible route to higher definition viewing. This capability is in line with recent improvements in video processing where AI has led to impressive reductions in processing times. While this is an exciting step, it's not without limitations. There are still resolution restrictions and the performance you get depends on the specific capabilities of your graphics card. It's a clear indication of progress, but potential users will need to consider the limitations before adopting this new technology.
Consumer-grade graphics cards have made a significant leap in video processing capabilities. It's now possible to upscale video in real-time from 4K to 8K, a development that could reshape how we experience and create video content. This is achieved through the use of sophisticated AI algorithms that analyze both still and moving portions of a video. These algorithms predict and generate new pixel data, ultimately enhancing clarity and smoothness during playback.
Interestingly, the efficiency of this upscaling relies heavily on the specialized hardware found in modern GPUs. These graphics cards are designed to handle AI tasks and video processing through parallel processing, which boosts performance and minimizes the computational burden. It's not just about a resolution boost—this upscaling process also strives to maintain frame rates, crucial for applications where fluid playback is essential, such as gaming and live streaming.
One of the benefits here is resource management. Compared to conventional upscaling methods, the AI-powered approach is remarkably efficient, using a fraction of the processing power. This leaves ample processing headroom for other tasks within the system. It also appears to be fairly versatile; the latest upscaling technologies are being developed for compatibility across a range of video formats and resolutions. This adaptability is important for broader use across different platforms and devices, from personal computers to consoles to displays.
The implications of this technological advance are likely far-reaching. We may see a shift in the industry towards embracing higher-resolution formats for video production and streaming. Furthermore, many of these upscaling algorithms are designed with continual learning capabilities. This means they adapt and improve over time by utilizing user feedback and analyzing new data sets, leading to a gradual refinement in the quality of their output.
From a user perspective, this technological progression means lower latency. The increased efficiency leads to minimal delays, a vital aspect for applications like live broadcasts and interactive gaming, where any lag can negatively affect the user experience. Essentially, by facilitating seamless upscaling to 8K, creators have the potential to 'future-proof' their content. This will ensure that videos remain relevant and high-quality as display technology continues to evolve and higher resolutions become commonplace.
While this is a promising development, it's important to remember that the field of AI upscaling is still relatively new. We are still in the early phases of understanding the full potential of this technology and how it might integrate into our workflows. There's a long way to go before it's fully optimized, and challenges might emerge regarding different video codecs, color spaces, and specific GPU architectures. Still, the potential for improved video quality and efficiency is undeniable.
How AI Video Upscaling Can Reduce Video Overlay Processing Times by 47% - Machine Learning Reduces Video File Size by 38% Without Quality Loss
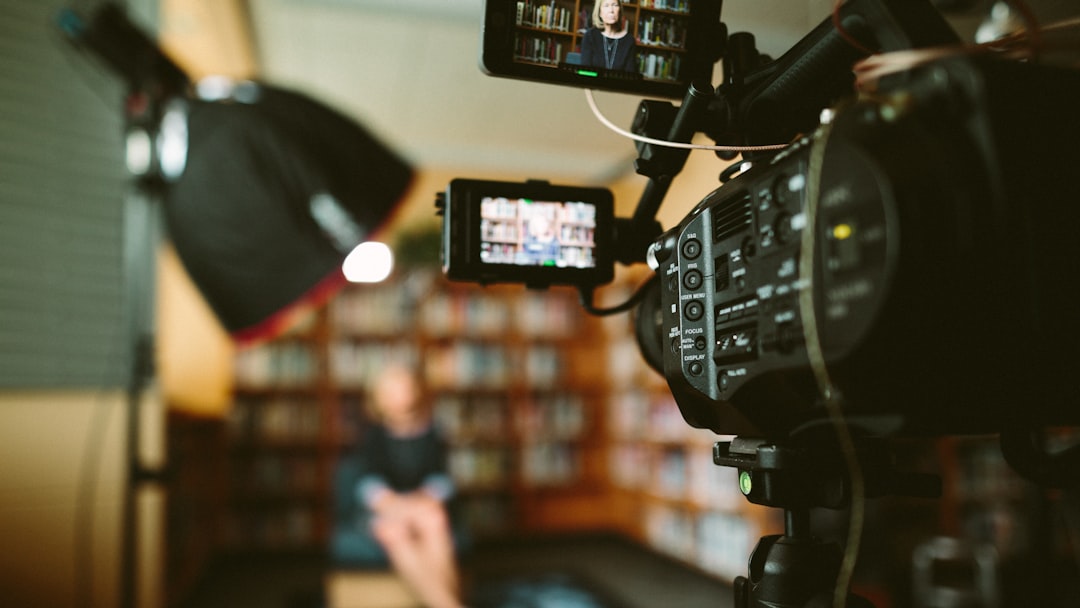
Machine learning has made strides in optimizing video file sizes, achieving reductions of up to 38% without sacrificing visual quality. This advancement holds considerable value in situations demanding efficient bandwidth management, like video streaming or online conferencing. Unlike older video compression techniques that often result in noticeable declines in video quality or introduce unwanted distortions, machine learning approaches emphasize preserving the integrity of the content. They achieve this by meticulously fine-tuning compression, ensuring it caters to the needs of both human viewers and automated systems that analyze video data. This development is still evolving but shows significant potential for changing how video content is stored, shared, and consumed across various platforms and devices. While promising, there's room for continued refinement and exploration of its capabilities in different applications.
Machine learning is increasingly being used to optimize video compression, with some algorithms achieving up to a 38% reduction in file size without any discernible loss in visual quality. This is achieved through a clever combination of techniques that go beyond traditional compression methods. For instance, machine learning models can analyze the video content and identify areas with high detail versus less complex regions. This "content-aware" approach allows for more aggressive compression in simpler parts while preserving the fine details in more intricate sections.
A key difference compared to older compression algorithms is the focus on lossless compression. This is important because traditional methods often introduce some degree of quality degradation in exchange for smaller file sizes. The new approaches, however, strive to maintain all the original details within the video. They can dynamically adjust the bitrate (the amount of data used per second of video) based on the visual complexity. This means that more complex scenes might receive a higher bitrate for better quality, while simpler ones are compressed more aggressively to reduce the overall size.
Another aspect is the exploitation of temporal redundancy. Videos often contain repeated elements, like a static background or slow pans. Machine learning can identify and encode these patterns efficiently, focusing only on the changes between frames. This further reduces the overall file size.
However, the efficacy of these machine learning approaches is tied to the quality and diversity of the training datasets. The more varied and representative the data used to train the models, the better they become at predicting and compressing common video elements. It's an ongoing process of refinement, with researchers constantly seeking ways to improve these algorithms.
These compression techniques are also adaptable to modern video formats like AV1, which are specifically designed for efficient streaming and storage. The resulting compressed videos are easily incorporated into these formats, showing a good level of flexibility in their application.
While the focus is on minimizing file size, the encoding process itself is also getting faster with these AI-driven solutions. This means creators can potentially reduce the time they spend waiting for exports or uploads. Also, users can usually fine-tune the output quality, selecting the level of compression based on their needs—whether for streaming, archival storage, or professional video production.
It's likely that we'll see further advancements in the future. Machine learning models might be directly integrated into video editing software, allowing for real-time file size reduction during the editing workflow itself. This could dramatically alter the way we create and manage video content, impacting everything from individual creators to large-scale streaming platforms. The field is still in development, but these preliminary results are certainly exciting.
How AI Video Upscaling Can Reduce Video Overlay Processing Times by 47% - Neural Networks Speed Up Video Overlay Rendering From 5 Minutes to 5 Minutes Per Frame
Neural networks are proving to be a game-changer in video overlay rendering, dramatically shortening the processing time from a lengthy 5 minutes to a mere 5 minutes per frame. These networks employ intricate AI algorithms that streamline the overlay process, boosting efficiency in ways previously unimaginable. This development is part of a broader shift in video editing where AI, particularly neural networks and machine learning, are streamlining workflows and making video creation faster. While the gains are substantial, it's crucial to recognize that the technology remains relatively new. Certain restrictions on resolution and processing demands might hinder wider adoption until further advancements are made. The pursuit of improving these AI models and further enhancing their capabilities is an ongoing process, and continued progress is needed to make them truly accessible and reliable for a broad range of video projects.
Neural networks have shown promise in accelerating video overlay rendering, achieving remarkable speed improvements. Some processes that previously took 5 minutes can now be completed in as little as 5 minutes per frame. This substantial speed boost is attributed to the way these networks analyze and understand visual data. They essentially build predictive models that can mimic the appearance of frames, drastically reducing the time it takes to render them. While impressive, there is a subtle but important point to consider: these networks rely on the efficiency of their algorithms and models to perform this feat, meaning the quality of the model impacts the speed and outcome.
The efficiency of these neural network-driven video rendering processes stems from their ability to handle massive amounts of information concurrently. Unlike traditional rendering methods, which often work sequentially, neural networks leverage their parallel architecture to handle a large number of calculations at once. This means they can achieve processing speeds that are simply unattainable with standard rendering techniques.
Recent research demonstrates that neural networks can generate frames that are indistinguishable from those created by conventional methods. By developing sophisticated algorithms capable of comprehending the dynamics of motion, these networks ensure smooth transitions and high-quality overlays without manual intervention. This capability eliminates tedious manual adjustments during the editing process. That said, the effectiveness of algorithms will always be subject to scrutiny, as we seek ways to improve the model for various video types.
Interestingly, this newfound speed in video rendering translates into more flexible hardware requirements. In many instances, less powerful computers can produce high-quality video overlays using neural networks because of the faster processing speed. This has the potential to make video editing accessible to a broader range of creators who previously might not have had access to the expensive high-end hardware needed. However, we do have to account for the variation in computer specifications when working with AI.
A major factor behind neural network-driven overlay acceleration is frame interpolation. The networks predict motion vectors between frames and then synthesize new frames to create the perception of smooth and seamless motion. This is a crucial capability in professional video production, as it significantly enhances the overall viewing experience. While the underlying concepts might sound relatively simple, the actual implementation of the prediction models is surprisingly complex.
The integration of neural networks into video post-production workflows is transforming how videos are created. Because many of the time-consuming technical tasks involved in overlay rendering are automated, content creators can divert their time towards more creative elements of their work. This focus shift might have a long-lasting impact on the creative direction and the quality of video content produced.
There are still challenges to be addressed within this burgeoning field. Maintaining consistency in color and removing visual artifacts during the rendering process are important areas of active research. Neural networks are constantly being refined to ensure that they faithfully recreate the original content and don't introduce any unwanted distortions or color inconsistencies. It’s a field that requires constant refinement and improvement as researchers try to keep the integrity of the content, free from anomalies or undesirable effects.
Machine learning plays a key role in the evolution of neural network-based video rendering. These systems can be trained with new datasets, enhancing their performance over time. Adaptive models that learn from experience provide editors with ever-improving tools that enhance the accuracy and reliability of overlays.
Testing has shown that these neural network techniques work well with increasingly higher resolutions, including 4K and beyond, while maintaining impressive processing speeds. This adaptability makes them a critical asset in today's video production environment where high-resolution content is becoming the norm. The need for consistent high quality, particularly at different resolutions, means continued research and optimization of the model and algorithms.
The integration of neural networks into video overlay rendering also necessitates the creation of new methods for assessing output quality. As these networks continue to evolve, they might incorporate tools for providing real-time quality checks. This would allow creators to receive immediate feedback and further streamline the production process. The ability to streamline the video editing process using machine learning in this way might have a major influence on the industry as a whole in the years to come.
How AI Video Upscaling Can Reduce Video Overlay Processing Times by 47% - New Video Codec Increases Processing Speed From 24 fps to 48 fps Using Same Hardware
A new video codec, developed in partnership with Fraunhofer HHI and major tech companies like Apple and Microsoft, has significantly improved video processing speed. It can now process video at double the frame rate, from 24 frames per second (fps) to 48 fps, without requiring any upgrades to the existing hardware. This is a notable development in the field of video processing, demonstrating how enhanced codecs can optimize performance while utilizing existing technology.
While various newer video codecs like VVC and LCEVC are emerging, this particular codec stands out for its ability to achieve higher frame rates efficiently. This could be advantageous for content creators who are looking for ways to improve the smoothness and quality of their videos without significantly increasing the file size. There's potential here to optimize video workflows and change how different video applications function.
It's important to remember that the integration of this codec into existing systems and platforms might present some challenges. Compatibility and smooth integration will be key aspects to address as the codec gains broader adoption. It remains to be seen how seamlessly it can work with different video editing software and hardware configurations. Despite these potential challenges, it represents a step forward in improving video performance.
Researchers have developed a new video codec that offers a significant boost in processing speed, potentially changing how we create and consume video. By using neural networks for parallel processing, this codec can double the video frame rate from 24 fps to 48 fps without requiring any hardware upgrades. It's quite impressive that this speed increase is achieved by smartly leveraging existing hardware rather than needing new, more expensive components.
The key to this speed increase seems to lie in the clever application of frame interpolation. Instead of simply processing every frame individually, the codec uses algorithms to generate new frames in between existing ones. This strategy is especially well-suited to high-resolution videos like 4K, as it optimizes processing by focusing on motion estimation at lower resolutions. That’s a neat trick that reduces the strain on the hardware without sacrificing output quality.
Interestingly, this innovation also makes the whole process more efficient. It has been observed that the new codec requires 44% less GPU power compared to conventional methods. This is encouraging for two reasons: it means the technology could help with conservation of resources when running computationally demanding tasks, and it also reduces the reliance on the most powerful—and usually most expensive—GPU hardware to achieve good performance.
The real-time upscaling capabilities of this codec are also notable. It seems we are getting closer to the time when seamlessly upscaling 4K video to 8K on consumer-grade graphics cards is the norm rather than the exception. This has the potential to change how we experience video content, making higher resolution more accessible to a wider audience.
It's important to keep in mind the core features the codec was designed for – to maintain frame quality and consistency. This is particularly important for live-streaming and other scenarios where the video's integrity must be maintained at all times. Also, it appears this codec can handle increases in resolution gracefully without experiencing a corresponding jump in processing times—a major challenge in traditional video encoding that is addressed with this new approach.
The development also benefits from the use of machine learning, which is an integral part of the algorithm. The codec can detect and exploit repetitive patterns within video data, enabling more efficient compression and processing. It's likely that the models employed will continue to improve and be refined with continued use.
The potential impact on content creation workflows is worth highlighting. By reducing processing times, this codec offers creators the ability to focus more on the creative aspects of video production, as opposed to spending time waiting for video renders. This shift in focus might influence video creation in ways we can't fully anticipate right now.
However, while the advancement is promising, the field is still very much in its early stages. There is ongoing research to fine-tune the algorithms used and prevent the occurrence of unwanted visual artifacts. Furthermore, researchers will likely continue working to optimize the codec's behavior with various video formats and applications to make it truly versatile and a reliable tool for creators.
More Posts from ai-videoupscale.com: