Converting MP4 to M4V Technical Impact on Video Quality and File Compatibility in AI Upscaling Workflows
Converting MP4 to M4V Technical Impact on Video Quality and File Compatibility in AI Upscaling Workflows - MP4 vs M4V Container Format Impact on Video Codec Retention During AI Processing
When preparing video for AI processing, the choice between MP4 and M4V container formats can significantly influence how the video's codec is handled. M4V, being more restrictive, is limited to the H.264 codec, while MP4 offers flexibility by supporting multiple codecs like H.264 and the more efficient HEVC. This codec diversity with MP4 is crucial for AI upscaling, where maintaining the codec during processing can impact quality and efficiency. Furthermore, HEVC's capability to achieve comparable video quality at half the bit rate of H.264, translates to significantly smaller file sizes when using MP4. MP4 also boasts better compatibility across devices and platforms, which can be important for processing and sharing workflows. Conversely, M4V's association with Apple products potentially limits its usability outside of their ecosystem. Ultimately, the decision between the two formats can impact how easily your video is processed by AI algorithms and, subsequently, the quality of the final upscaled video output.
1. While MP4 and M4V appear similar in their role as containers for video and audio, M4V is essentially a tweaked version of MP4. This suggests that M4V might carry metadata specific to Apple's devices, possibly affecting compatibility outside of their ecosystem.
2. M4V files can sometimes be associated with Apple's DRM (Digital Rights Management), which can limit playback on non-Apple devices. This restriction can present issues in AI pipelines that are meant to be flexible and work with various formats.
3. AI algorithms often depend on the codecs inside the container format. Since MP4 supports a wider variety of codecs compared to M4V, this could potentially influence the final video quality after conversion between the two.
4. When scaling video with AI, compression levels are crucial for detail preservation. Starting with an MP4 source might deliver a superior initial quality level compared to an M4V, especially if the M4V has undergone heavy compression.
5. Some M4V streams may use Apple's proprietary codecs. AI tools might not always fully support these codecs, raising concerns regarding output quality and processing speed.
6. MP4 files often bundle video, audio, and subtitle streams within one file without significant quality loss. M4V primarily focuses on video and audio, with less emphasis on subtitles, which could impact AI workflows that require subtitle data.
7. The specific error correction methods found in each format are worth considering. MP4's support for codecs like H.264 and H.265, which are known for their reliability, may be preferable compared to the H.264 found in most M4V files.
8. The visual impact of compression artifacts during AI scaling can differ depending on the encoding settings of MP4 versus M4V. Potentially, MP4 could be more suitable for delivering visually sharp outputs in high resolution.
9. The choice of MP4 or M4V as a source file can influence AI processing times. The efficiency of decoding can vary between formats, with MP4's wider compatibility often allowing faster access for AI algorithms.
10. Switching from M4V to MP4 in certain AI upscaling scenarios might result in improved quality. This is because of the increased compatibility with a wider range of tools, which could lead to a cleaner, more detailed final video.
Converting MP4 to M4V Technical Impact on Video Quality and File Compatibility in AI Upscaling Workflows - Technical Analysis of Color Space Changes in MP4 to M4V Conversion
When converting MP4 to M4V, a key technical aspect to understand is how color space changes might affect the final video output. While both formats generally use the same MPEG-4 compression, ensuring video quality isn't compromised during playback, the color representation methods used—like RGB, YUV 444, and others—can make a difference. M4V often relies on the H.264 codec, and sometimes includes DRM (digital rights management), potentially altering file sizes and device compatibility in comparison to MP4 which supports a wider range of codecs and is more widely accepted. How colors are handled in this conversion process is crucial because our eyes perceive colors differently depending on how they're represented, potentially impacting the quality, especially within AI upscaling workflows. Balancing file size and video quality is always a challenge, and understanding the subtle effects of color space changes is necessary for getting the best outcome during conversion.
1. Converting from MP4 to M4V can potentially change how color information is handled. M4V often uses a more limited set of color representations, which might not accurately capture the original video's colors. This could lead to subtle shifts in how the colors are displayed.
2. Many M4V files tend to rely on the YUV color space. While this is a common standard, it might not be ideal for advanced AI upscaling techniques. In comparison, MP4 can utilize color spaces like XYZ, which offer potentially richer color details for AI processing. It is still unclear if this is a meaningful difference in many practical workflows.
3. During conversion, the color profile details within the video can get lost or altered. This means the color information the AI system uses might not be fully representative of the original MP4 file. This metadata loss could lead to unpredictable color changes or errors when the AI process scales the video.
4. The way M4V files compress video data can reduce color accuracy. Color information gets simplified during this process, potentially leading to a less vivid or less detailed representation compared to the original MP4. This could be a factor in why AI upscaling might deliver better results when starting with an MP4, especially if the M4V was heavily compressed.
5. Compressed M4V often displays visible color banding, which is like noticeable steps in color transitions. This can negatively impact AI algorithms meant to refine details and restore smooth gradients, because they might have a harder time correcting for these jumps in color.
6. M4V's default gamma settings can influence the perceived brightness and contrast of the video after conversion. This means AI algorithms that try to improve color might need to adjust more if starting with an M4V, adding a layer of complexity to the color correction process.
7. MP4 generally handles HDR (High Dynamic Range) video better, which is crucial for maintaining vivid and accurate colors. If your original video contains HDR information, converting it to M4V might cause this information to be discarded or less accurately represented. This detail loss can impact AI upscaling processes aiming to maximize the visual quality of the video.
8. M4V files frequently use 4:2:0 chroma subsampling. This means some color information is reduced, which can be important when AI systems aim to improve image clarity and resolution by using detailed color information. This reduction may hinder the effectiveness of the AI upscaling process for color related enhancements.
9. Since M4V files tend to prioritize audio, the color spaces they use might not be as precisely controlled as in MP4. This lack of focus on color might result in missing or limited information that is important for achieving optimal AI-based upscaling results. It's debatable whether this is practically relevant.
10. When precision and visual fidelity are paramount, M4V's limited color capabilities can pose some challenges. AI tools that expect a wider range of colors might have issues reaching their full potential with an M4V input. It is unclear how significant this limitation is in the context of most AI upscaling techniques.
Converting MP4 to M4V Technical Impact on Video Quality and File Compatibility in AI Upscaling Workflows - Frame Rate Consistency Measurements Between MP4 and M4V During AI Upscaling
When evaluating the consistency of frame rates between MP4 and M4V files during AI upscaling, it's important to recognize that both formats, in theory, can handle similar frame rates based on the encoding settings used. However, practical performance can differ due to compatibility differences and how each format interacts with different AI tools. MP4, being more widely compatible across devices and platforms, generally offers a more seamless experience. On the other hand, M4V's connection to Apple devices and its potentially restrictive nature might lead to playback issues outside of that ecosystem. This, in turn, can affect not only the upscaled video's quality but also its smoothness and the way individual frames align during playback, which is especially notable in fast-paced scenes. As AI upscaling continues to improve, a deeper understanding of how different container formats fit into these workflows will become critical for optimizing video quality and ensuring that frame rates remain stable and consistent throughout the process.
Frame rate consistency between MP4 and M4V can be a significant concern during AI upscaling. M4V, often limited to the H.264 codec, might show more frame rate inconsistencies, particularly in scenes with rapid motion or demanding high bitrates. This could be due to the compression limitations of H.264.
On the other hand, MP4, with its wider codec support, including potentially more efficient ones like HEVC, can lead to a smoother upscaled output. This broader codec choice allows for more tailored handling of frame data during the upscaling process.
Converting between MP4 and M4V can introduce temporal artifacts, such as stuttering or blurring in fast-paced content. These artifacts stem from potential frame rate inconsistencies during the conversion, impacting the overall video quality after AI enhancement.
MP4 inherently manages timestamp data more effectively than M4V. This attribute helps AI upscaling processes, like interframe interpolation—which generates new frames to enhance smoothness—operate more efficiently and effectively.
Compression levels significantly impact frame rate. M4V, often employing higher compression levels, can suffer more frame inconsistencies compared to MP4. This difference can lead to visible discrepancies in video quality after AI upscaling.
Some AI upscaling algorithms rely on the assumption of consistent frame rates. Using M4V could lead to decreased performance as these algorithms might struggle to handle the frame inconsistencies that are more likely to occur with M4V files.
M4V's adherence to Apple's encoding guidelines could also influence frame rate measurements. These specific guidelines might cause unexpected frame timing issues not commonly found in MP4 due to its more flexible nature.
Various playback devices respond differently to the two formats. MP4's greater compatibility usually translates to better frame rate consistency across diverse devices compared to M4V, which has a stronger association with Apple products.
The character of AI upscaling workflows can change depending on frame rate inconsistencies. When processing M4V, AI systems might require extra adjustments to address these discrepancies, increasing processing time and reducing overall efficiency.
Ultimately, while M4V can offer advantages in areas like audio quality, its potential frame rate issues might not be ideal for all AI workflows, especially those where stable, consistent playback is crucial. This highlights the need for users to carefully consider the potential impact on frame rate when choosing between MP4 and M4V for AI upscaling tasks.
Converting MP4 to M4V Technical Impact on Video Quality and File Compatibility in AI Upscaling Workflows - Bitrate Management Strategies for M4V Output in Neural Network Processing
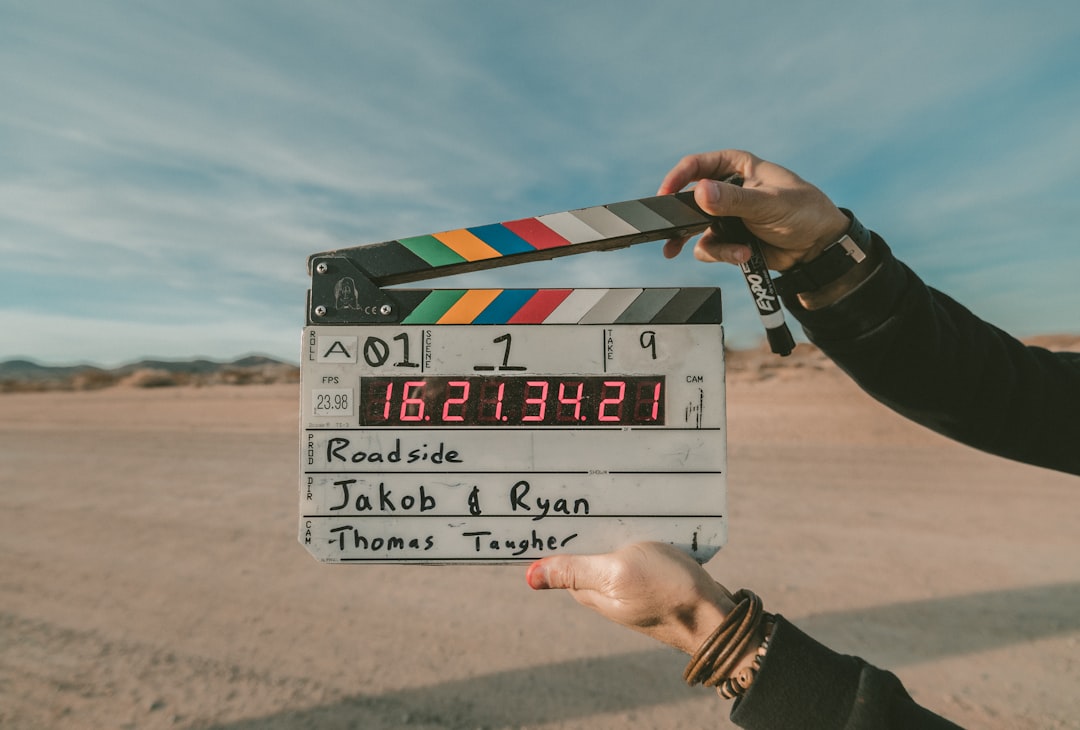
When employing neural networks for video processing, particularly in AI-driven upscaling, managing the bitrate of the M4V output becomes essential for a good outcome. The ability to dynamically adapt the bitrate, using techniques like those found in CNNs and RNNs, is important. This dynamic adaptation allows the system to respond to changes in video content and the network's capacity, which helps ensure the video quality remains high and the user experience is smooth. This kind of dynamic approach is better than the traditional methods that use fixed bitrate control rules, which can be inflexible and not as responsive to real-world conditions. Furthermore, some new compression methods, including DeepCABAC in Neural Network Coding, offer potential for improving the efficiency of bitrate management. These newer techniques aim to compress the file size without significant performance loss, so the end result for the user is a video that plays well and has a good quality level. In the complex landscape of AI video processing, carefully considering and managing the bitrate in the final M4V output is key to making the most of AI upscaling.
1. How we manage the bitrate when outputting to M4V format within neural network processing directly impacts the quality of the AI-enhanced video. A higher bitrate can preserve more details, especially important in scenes with a lot of complexity. However, higher bitrates also demand more storage and computing power, potentially slowing down our workflows.
2. The way we choose a bitrate can cause unusual artifacts that appear differently in M4V files. These artifacts make it harder for the neural network to accurately fill in or create new frames, leading to a loss of quality that might be hard to recover during upscaling.
3. M4V files often end up with more noticeable compression artifacts because of how the codec is designed. These artifacts can interfere with AI upscaling because the algorithms might confuse compression noise with actual details in the video.
4. The bitrate settings we use for MP4 and M4V processing can be different, and this affects the output quality. Neural networks often work best with higher bitrates because they have more detailed data to work with when making predictions.
5. A video's timing—how frames are shown over time—is influenced by the bitrate. Lower bitrates can cause frames to be skipped or make playback jerky, especially in scenes with a lot of motion. This makes it even harder for AI systems to correctly analyze movement.
6. There isn't a simple relationship between bitrate and how detailed the colors are. In M4V, a higher bitrate can improve color accuracy, but it might not get rid of all the errors from quantization, which can still cause banding when AI tries to enhance the video.
7. Sometimes the audio setup of a M4V file can unintentionally affect how the video bitrate is managed. This can lead to decisions that favor audio over video quality, which is a frequent subject of debate for engineers working on processing both audio and video.
8. Sophisticated bitrate management within AI workflows often needs a careful analysis of the data during the encoding process. The limitations of M4V can make it less flexible, making it harder to find the best encoding settings for AI tasks.
9. Different encoding standards within the M4V format can change how efficiently we use the bitrate. For example, using the "Main Profile" versus the "High Profile" encoding can significantly affect video quality and how fast neural networks can process it. This creates a direct link between bitrate management and AI performance.
10. Using variable bitrate (VBR) settings for M4V might theoretically improve quality, but it can lead to unpredictable outcomes in AI workflows. Sudden bitrate changes can disrupt how algorithms function smoothly, particularly when trying to process things in real time.
Converting MP4 to M4V Technical Impact on Video Quality and File Compatibility in AI Upscaling Workflows - Audio Codec Compatibility Assessment for Cross Format Conversion
When converting between MP4 and M4V formats, especially within AI upscaling pipelines, evaluating audio codec compatibility becomes vital. Both containers often utilize the AAC codec, but MP4's wider support for various audio codecs, including MP3 and others, offers more flexibility in preserving audio quality during conversions. However, transitioning audio streams can pose challenges. For instance, AAC typically delivers better audio than MP3, so selecting the right codec during the conversion is important to avoid quality losses. Furthermore, device compatibility can vary greatly depending on the chosen audio codec, which can impact successful cross-format conversions. Understanding these intricacies is crucial for ensuring both the best audio quality and smooth integration with AI-driven video processing. It is unclear if these details meaningfully affect the outcome of most AI upscaling algorithms in practice.
1. The compatibility of different audio codecs can have a big impact on how well AI upscaling works. For example, while M4V files usually use AAC for audio, MP4 files can handle a wider range of codecs like LPCM and Dolby Digital, giving AI systems more flexibility.
2. The limitations of M4V in terms of supported audio codecs can lead to audio quality getting worse during conversion. This might force AI tools to do more work, which could slow down the whole video enhancement process.
3. Differences in audio sample rates between MP4 and M4V can cause problems for AI systems. MP4 often allows for higher sample rates, but some M4V settings might use lower ones, leading to inconsistencies that make synchronization difficult during upscaling.
4. M4V's reliance on specific audio codecs often used by Apple products could make it hard to use with general-purpose AI tools, potentially leading to unexpected issues with audio-video syncing or a decrease in quality during upscaling.
5. The way video and audio codecs are related can be difficult for AI systems to handle, especially if the AI model isn't designed to deal with proprietary audio formats often found in M4V. This could limit the quality of the audio and video.
6. Features like spatial audio, which are often found in newer formats, might not be as easily available in M4V. This can restrict the AI's ability to optimize the audio when upscaling, leading to a less impressive sound experience.
7. When carefully managing the bitrate is important, MP4 can allow for more precise adjustments to balance audio and video needs, while M4V might not offer the same level of control.
8. The way audio is encoded in M4V can make it more dependent on specific codecs, leading to potential bottlenecks when using AI applications that need quick access to a variety of audio formats for effective upscaling.
9. Getting perfectly synchronized audio and video can be more challenging with M4V files because of potential delays associated with certain audio codecs. This makes it harder for the AI system to accurately analyze and enhance the video.
10. Finally, a sudden switch between M4V and an AI processing system could cause unexpected issues. This is because the AI system's expectations for how the audio should be processed might not match the more flexible audio layer often found in MP4. This can lead to inconsistencies in the final output.
Converting MP4 to M4V Technical Impact on Video Quality and File Compatibility in AI Upscaling Workflows - Metadata Preservation Methods Between Container Format Switches
When converting between video container formats, like from MP4 to M4V, preserving the associated metadata becomes a crucial aspect. Video files carry crucial information, including codecs, audio/video settings, subtitles, and other playback attributes. If this data isn't handled carefully during the format switch, it can cause problems. Different container formats have varying ways of storing and presenting metadata, which can affect how media players and software interpret the video. This means a video might play back differently on different devices or programs. It's important to consider how metadata is transferred during these container format changes, as losing or misinterpreting this data can negatively impact the video's usability and overall user experience. Furthermore, as AI upscaling gets more advanced, carefully handling this metadata is even more critical for maximizing video quality and making sure that the AI processing is efficient and produces reliable results. In short, metadata preservation is important when changing container formats, and getting it right is especially vital as AI plays a bigger role in video workflows.
1. Maintaining metadata when switching between container formats like MP4 and M4V can be tricky. M4V might not keep all the metadata that MP4 does, which could mean losing details like titles or chapter markers. This could make it harder for AI systems to organize and find the videos efficiently.
2. MP4's metadata structure lets you have multiple types of streams—like subtitles, video, and audio—all in one header. M4V, however, usually focuses on just video and audio metadata, limiting its use in applications that need to handle a wider range of metadata.
3. The limitations of M4V's metadata format can cause problems when AI systems try to process the video. The restricted metadata might lead to AI systems misunderstanding or ignoring important information that could improve the quality of the final video, especially when syncing audio and video precisely.
4. The different ways that MP4 and M4V handle metadata can lead to changes in data quality after the conversion. AI tools might have a harder time working well with M4V due to possible issues in understanding the metadata during conversion, which can throw off the workflow.
5. Some AI programs rely on specific metadata formats to work properly. When converting to M4V, if some of the metadata fields are missing, the files might not work with those AI algorithms, which reduces the effectiveness of the entire AI processing process.
6. Losing codec-specific metadata during conversion can make it harder for AI systems to take advantage of advanced features in MP4 files, like playback optimization and error correction. This can affect the quality of the final AI-enhanced output.
7. MP4 lets you add lots of descriptive tags to video content, while M4V tends to be more limited in this area. This difference might affect how AI systems assess and improve the content based on the information from the tags in MP4.
8. Bitrate and quality metadata in MP4 is usually structured in a way that makes it easier for AI systems to change how they process the video. M4V, however, has a more rigid metadata structure that might require more processing, slowing down the AI workflow unnecessarily.
9. M4V doesn't support some advanced metadata features, like segment-based indexing. This limits the efficiency of AI upscaling because the algorithms can't easily find and process parts of the video that need extra attention due to their complexity.
10. Checking the integrity of metadata after conversion is crucial. Without preserving the original attributes, the risk of errors goes up. AI systems could make incorrect assumptions about the structure of the content, leading to suboptimal enhancements and potentially underestimating the capabilities of M4V during processing.
More Posts from ai-videoupscale.com: