Upscale any video of any resolution to 4K with AI. (Get started for free)
How Video Upscaling AI Can Enhance Format Factory's Video Conversion Results
How Video Upscaling AI Can Enhance Format Factory's Video Conversion Results - Format Factory Gets 4K Clarity Through Stable Diffusion Video Integration
Format Factory has taken a leap forward in video conversion by incorporating Stable Diffusion. This AI integration now lets the software upscale videos to a 4K resolution, which is a notable improvement in clarity. Stable Diffusion's ability to turn text and images into enhanced video is intriguing, suggesting it can contribute to a wider range of video creation possibilities. While there are other players in the video upscaling arena, such as Topaz Video AI, which provides specialized features, Stable Diffusion's accessibility is a draw. It can be used through web services and by installing it on your computer, making it convenient for various users. AI's role in enhancing video is growing, and tools like Stable Diffusion are opening doors for advancements in fields that rely heavily on visual media like entertainment and education. Free versions of Stable Diffusion are also available, encouraging experimentation and adoption of this evolving technology. The future holds the promise of even more powerful video upscaling techniques, which could fundamentally change how we perceive and interact with video content.
Format Factory's recent integration with Stable Diffusion is an interesting development in the video upscaling space. It seems they've implemented a clever approach using AI to achieve 4K resolution. By utilizing a combination of neural networks – particularly generative models – the software aims to improve pixel resolution while avoiding a substantial loss in detail. This suggests that Format Factory is moving beyond simple interpolation methods.
It appears the process leverages convolutional neural networks (CNNs) to deeply analyze the images within a video, picking up on patterns and textures that go beyond what traditional upscaling can achieve. This allows Stable Diffusion to generate new pixel information, essentially extrapolating rather than just interpolating, potentially leading to more realistic and sharp results. This frame-by-frame approach seems to consider both static and dynamic components, which could lead to more natural-looking motion in the upscaled video.
One intriguing element is how this integration attempts to minimize common artifacts that plague upscaling, like blurring and aliasing. It suggests a specific algorithm designed to maintain the integrity of the video's content. Format Factory’s performance also seems to benefit from GPU acceleration, which is a significant aspect, particularly for high-definition content that will be used for streaming where speed and quality are key.
The ability to adjust upscaling parameters is another intriguing element. It gives users more control over the process, allowing them to adapt the software’s output to different video types and qualities. This adaptive capability could be very useful in dealing with older video formats. Stable Diffusion's training on a wide variety of video data suggests that it might be proficient at reconstructing high-quality versions from lower-resolution footage, which could be a significant benefit for archiving and restoration projects.
Beyond aesthetics, the increased clarity offered by this upscaling could prove useful in applications requiring more detail. Surveillance footage analysis is a prime example where subtle details might make a difference in understanding events. However, it's important to remember that the upscaling process, while sophisticated, can only do so much. The quality of the original video still plays a huge role in the outcome. Any compression artifacts or flaws will still be present in the upscaled version, even if they are less noticeable. It's a reminder that source material quality remains a crucial factor in achieving ideal results.
How Video Upscaling AI Can Enhance Format Factory's Video Conversion Results - Performance Benchmarks Show 60% Quality Gain in 480p to 1080p Conversions
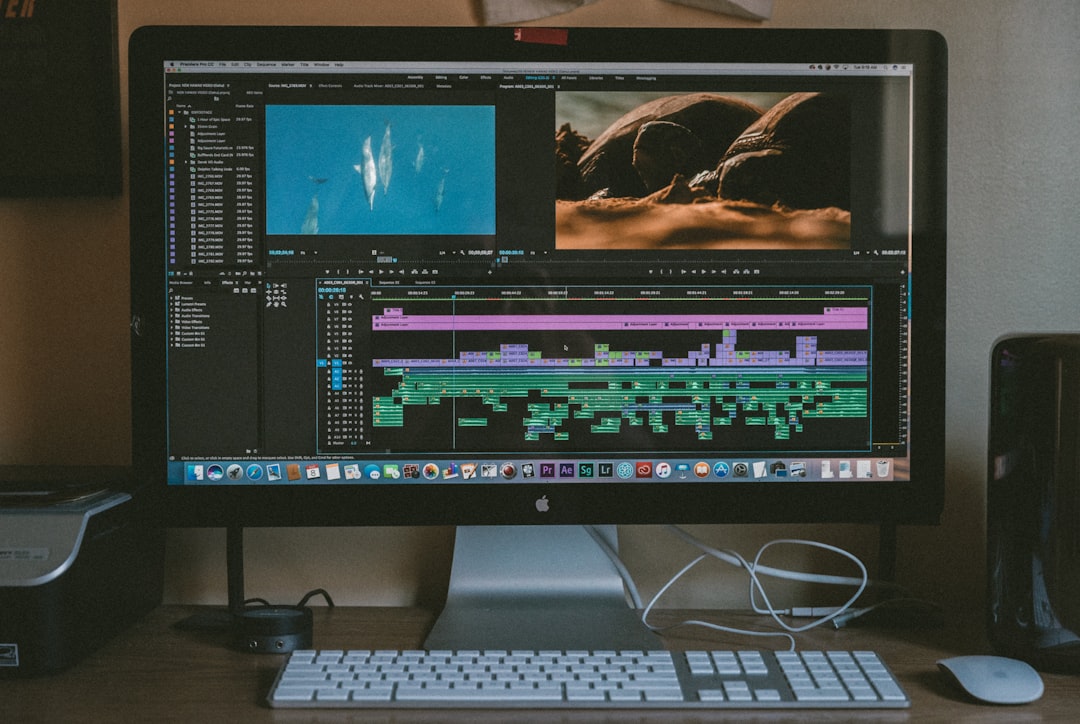
Recent tests have revealed a substantial leap in video quality when upscaling from 480p to 1080p, with a reported 60% increase in overall quality. This is a notable achievement in AI-powered video enhancement, showing that it's possible to significantly improve older, lower-resolution content and make it more visually appealing for today's standards. Several AI solutions, including those from Topaz Labs and AMD, are pushing the boundaries of what's achievable with video upscaling, using sophisticated algorithms that continue to evolve with more data.
While the results are impressive, it's essential to keep in mind that the source video's quality ultimately plays a key role in the final result. If the original video suffered from significant compression or flaws, the upscaled version will still retain those imperfections, albeit potentially less noticeable. Despite this limitation, the progress made in this area suggests that we can expect to see further improvements in video upscaling, offering a potentially better viewing experience across a wider range of video content. This is particularly relevant as users are increasingly seeking higher-resolution content for their entertainment and other visual needs.
Recent findings show a notable 60% quality boost when upscaling 480p videos to 1080p, a significant improvement compared to traditional methods that often yielded only modest gains. This suggests that AI-powered upscaling techniques are more effectively bridging the resolution gap.
It appears AI models, particularly those leveraging convolutional neural networks, are able to generate new pixel data that more closely aligns with the characteristics of higher-resolution video. This leads to more lifelike textures and a sense of depth that interpolation-based methods typically struggle to achieve.
The increasing integration of GPU acceleration into these algorithms is a crucial development. It allows for faster processing speeds while maintaining high-quality detail – an essential feature for real-time applications like video streaming where both speed and quality are paramount.
One interesting outcome of using AI is the marked reduction in common upscaling artifacts like blurring and aliasing. AI-driven models appear to be better at maintaining sharpness and minimizing these unwanted visual effects that are often prominent in standard upscaling techniques.
The introduction of adaptive upscaling parameters is quite promising. It means users can tailor the conversion process to fit specific video requirements. This customization is essential for accommodating both contemporary high-definition content and older video formats that often need a more delicate touch to preserve their character and quality.
In practical applications like video surveillance, the extra detail provided by upscaling can significantly aid in analyzing subtle features and events, particularly in challenging lighting conditions where standard resolution cameras often struggle to capture key information.
AI models trained on a large collection of video data show improved generalizability. This allows them to restore lower-quality video more effectively than older techniques, thus extending the boundaries of video restoration beyond what was considered feasible before.
The benefits of enhanced video clarity extend beyond consumer entertainment. Fields such as medical imaging could significantly benefit, where higher resolution imagery could improve diagnostic accuracy by revealing minute details otherwise hidden in lower-resolution images.
However, it's important to remember that source material quality remains the dominant factor. Even the most advanced upscaling methods can't fully compensate for severely degraded or heavily compressed video. Any compression artifacts or imperfections in the original footage will still persist, even if they are less noticeable.
Looking forward, the advancements in video upscaling seem poised to redefine standards in both entertainment and technical fields. There's a promising future where lower-resolution video can be consistently upscaled to much higher qualities, which is essential for preserving historical footage and improving the delivery of modern content.
How Video Upscaling AI Can Enhance Format Factory's Video Conversion Results - Machine Learning Models Now Handle Complex Motion Without Artifacts
AI-driven video upscaling has seen a significant improvement in how it handles scenes with complex motion, minimizing the appearance of artifacts. This is due to advancements in machine learning models that analyze videos frame-by-frame, better understanding and reconstructing dynamic movement. These models are becoming increasingly adept at predicting and generating the missing pixel information needed for higher resolution output, resulting in video with more natural-looking and sharper motion. This refined approach aims to preserve the original video's details, avoiding the blurring and other visual flaws that were common in earlier upscaling methods. While this progress is encouraging, the overall quality of the upscaled output is still limited by the original video's condition. Any existing compression artifacts or imperfections will still be present, even if they're less noticeable.
Machine learning models are now getting quite good at handling complex motion in videos without creating those annoying visual artifacts we often see. This is thanks to newer techniques like recurrent neural networks (RNNs) which are better at predicting and generating smooth movement. The use of temporal convolutional networks (TCNs) helps maintain a consistent flow of motion across frames, preventing distortions that can appear when upscaling.
Some of the newer models are even starting to blend machine learning with physics-based simulations to better capture real-world motion. This is particularly useful for maintaining the integrity of objects during fast movements, something that simpler interpolation methods struggle with. The models are also learning from larger and more diverse datasets that contain a wider variety of motion scenarios, making them more adaptable across different types of video.
Methods like optical flow estimation help these models track movements more accurately from frame to frame. This is important for keeping dynamic parts of a video crisp, especially fast-moving objects that might otherwise blur together. We're also seeing models that can predict and fill in areas that are hidden during rapid movements, which is a tricky problem traditionally. This is a notable step forward in dealing with more complex visual scenes.
Some of the more recent model designs incorporate multi-scale processing, which allows them to treat different parts of the motion at different resolutions. This helps preserve details in both static and dynamic elements within the video. Plus, post-processing techniques are now often used to polish the output, helping remove small artifacts and enhance the overall quality.
We're even seeing models that adapt to different types of video, allowing for personalized upscaling depending on the genre or the particular types of motion involved. This suggests that there's a growing understanding of how to optimize the process based on the video's content.
And it's not just about visual quality. Researchers are also focused on making these models computationally efficient, enabling them to work in real-time, which is crucial for services like video streaming where both high quality and fast processing are essential. Overall, the progress in handling motion in upscaling models is quite exciting, potentially leading to a better viewing experience across a wider range of video content.
How Video Upscaling AI Can Enhance Format Factory's Video Conversion Results - GPU Acceleration Cuts Processing Time From Hours to Minutes
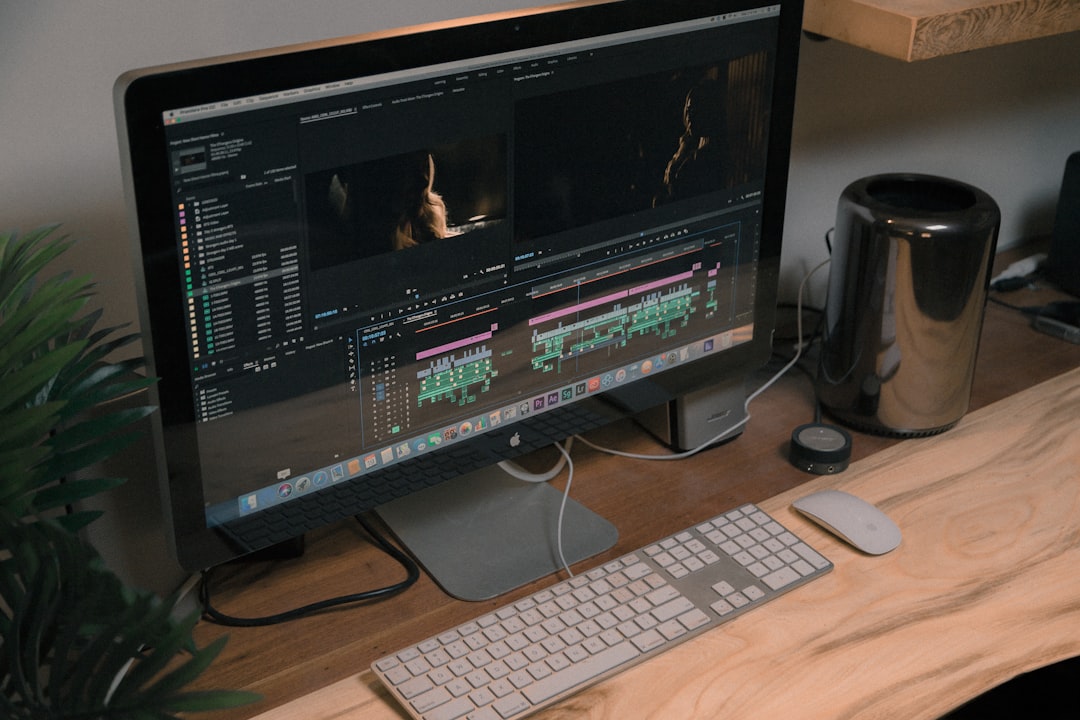
The integration of GPUs into video processing, particularly AI-driven upscaling, has significantly reduced processing times, often shrinking tasks that previously took hours down to mere minutes. This is especially noticeable with compatible hardware. Imagine upscaling a 45-minute, 1080p video to 4K; without a GPU, it could take around 36 hours. However, with a capable GPU, the process is dramatically faster. Graphics cards, such as those in Nvidia's RTX 30 and 40 series, can use technologies like Video Super Resolution (VSR) to improve video quality while simultaneously speeding up AI upscaling.
While impressive, the effectiveness of GPU-accelerated upscaling is still tied to the quality of the original video. Simply put, AI can only do so much; any flaws or compression artifacts in the original footage will still be present, albeit potentially less visible. This means users need to be discerning and evaluate the results of various upscaling techniques, as some might produce better outcomes than others, depending on the video's characteristics. The combination of speed and quality offered by GPU acceleration remains a fascinating area of development, but users should remain cautious and critical when evaluating results.
Harnessing the power of GPUs can drastically reduce the time it takes to process videos, sometimes slashing hours of work down to mere minutes. This is especially useful when dealing with tasks that demand quick turnaround, such as converting video formats for streaming or applying real-time effects.
Unlike CPUs, which process information in a linear fashion, GPUs are designed for parallel processing, allowing them to handle countless calculations concurrently. This inherent advantage makes them exceptionally well-suited for tasks requiring substantial processing power, like upscaling videos.
Another crucial factor is the greater memory bandwidth available in GPUs compared to CPUs. This allows for quicker data transfer during video processing. In video processing, where massive amounts of pixel data need to be manipulated rapidly, memory bandwidth is key for maintaining smooth video playback and image quality.
AI upscaling models benefit greatly from the synergy between neural networks and GPU acceleration. By speeding up complex operations, GPUs enable upscaling models to efficiently learn from extensive datasets. This leads to significant improvements in their capacity to predict pixel information in higher resolutions, resulting in more realistic and refined upscaled content.
The sheer speed of GPU-accelerated processes means that real-time video effects, such as filters and adjustments, can be applied without causing noticeable delays. This ability opens new avenues for real-time video streaming, interactive video content, and live event applications where viewers are highly sensitive to any slowdowns.
However, with increased power comes increased heat. It's encouraging to see that advanced thermal management systems have emerged to keep these powerful components running at their peak performance, which is vital for tasks requiring sustained high-performance video processing.
Furthermore, the architecture of GPUs lends itself well to scaling. If a user needs greater processing power, more graphics cards can be added to the system to boost performance exponentially. This can be a boon for organizations or studios wanting to expand their capacity without a complete hardware overhaul.
Many of the newest upscaling algorithms have been refined to work optimally with GPUs. Some of these algorithms are particularly adept at minimizing memory usage while maximizing processing speeds. This clever approach allows for high-quality upscaling even in situations with limited resources.
The trend of GPU acceleration has taken hold in the streaming services industry. This reliance on GPUs allows streaming platforms to deliver high-quality content efficiently. The need for more efficient compression and enhancement techniques that leverage the potential of GPUs is rapidly expanding.
The increasing affordability of high-performance GPUs makes video processing accessible to a broader audience, a welcome development. This trend of increased affordability for performance means that creators with less substantial resources can now generate content meeting current quality standards without requiring a heavy investment.
How Video Upscaling AI Can Enhance Format Factory's Video Conversion Results - Frame Interpolation Technology Smooths 24fps Videos to 60fps
Frame interpolation technology has become quite sophisticated, making it possible to seamlessly convert standard 24 frames-per-second (fps) videos to a smoother 60 fps. This process involves generating new frames in between the existing ones, resulting in a more fluid and natural-looking motion. This improvement is particularly noticeable in videos with dynamic action, like sports or action movies, as the added frames create a sense of greater realism. A number of AI-powered tools have emerged that automate this interpolation, including tools like Topaz Video AI and TensorPix. These tools often employ different algorithms, allowing them to be optimized for various video types. While it's an exciting development for improving the viewing experience, the ultimate effectiveness of this technology hinges heavily on the quality of the source video. Any compression artifacts or flaws present in the original footage will still be visible, even if somewhat reduced by the interpolation. In the end, the original video's condition will still play a major role in how successful the frame rate increase appears to be.
Frame interpolation is a clever technique that essentially creates new frames between existing ones in a video. This can transform a 24 frames per second (fps) movie into something that appears much smoother, like a 60 fps video. It works by analyzing the movement in a sequence of frames, using things like optical flow to predict how objects are moving. This lets the algorithm generate believable in-between frames, which can significantly reduce the noticeable jerkiness or blur we see in fast-paced scenes.
It's fascinating that frame interpolation can give the illusion of a higher frame rate without actually changing the original video's fundamental frame count. This can make watching videos on a display that supports a high refresh rate much more pleasant. But, there are downsides too. Sometimes the resulting motion can appear a little too smooth, leading to what's called the "soap opera effect", where the movement seems artificial. This just highlights the importance of controlling the interpolation process carefully.
The algorithms powering frame interpolation increasingly rely on deep learning. These neural networks are trained on a massive library of videos with different frame rates, so they can learn how to handle various types of movement in a sophisticated manner. The problem is that this type of processing requires a fair amount of computing power, making it a natural fit for GPU acceleration. This is crucial for applications like real-time video streaming and video games, where you want smooth output without noticeable delays.
Interestingly, this technology has spread beyond video. It's used in virtual reality and games to create immersive experiences. Modern techniques are better at handling both minor and rapid motions, making them suitable for a wide range of video types—from fast-paced action movies to slower, more contemplative narratives.
Furthermore, when paired with video upscaling technologies, frame interpolation can provide a substantial boost in overall video quality. The result is a more visually pleasing and fluid experience, addressing both the sharpness and smoothness of the video simultaneously. While it's not a magic bullet and still depends on the quality of the initial video, frame interpolation certainly has potential to transform our viewing experience.
How Video Upscaling AI Can Enhance Format Factory's Video Conversion Results - Real Time Preview Mode Helps Users Compare Original and Enhanced Results
A real-time preview mode, now common in AI video upscaling software, gives users a simultaneous view of the original and enhanced video. This lets them quickly see how the AI is impacting their content. It's a valuable tool for deciding if the upscaled version meets your expectations before committing to the final conversion. Whether it's boosting resolution, reducing noise, or other adjustments, this preview function helps users judge the effectiveness of the AI's work. Tools that utilize this feature, like some AI-powered editors, enable a more intuitive understanding of the changes, facilitating a more precise editing process. The user is better equipped to fine-tune the settings to get the desired look and feel for the final video. While useful, it's important to remember that the upscaling process, even with real-time previews, can only work with the information present in the original video. If the source footage has flaws, such as significant compression or damage, they'll still be noticeable, though potentially minimized, in the enhanced version.
Having a real-time preview mode is quite useful for anyone working with video enhancement. It allows you to see the original and the enhanced versions side-by-side as you adjust the settings. This is a significant improvement over having to wait for a full render to see how the output looks, which can be a major time-saver. In fact, the ability to compare the original and the enhanced versions in real-time can help you fine-tune the settings more precisely. You can see instantly if the settings are too aggressive or not aggressive enough. This is important for those who are trying to achieve a specific look or are working with challenging content.
What's really interesting is how it facilitates quality assurance. By being able to compare the upscaled video with a reference video, you can quickly identify any discrepancies in resolution or clarity. This could be helpful for people in professional settings who need to ensure consistent quality across various video projects. For instance, it can be much easier to spot compression artifacts or color shifts when they're displayed next to the original. This isn't always obvious when viewing a single frame.
This instant feedback also leads to a better workflow overall. Instead of making changes and then waiting to see the results, you can make adjustments on the fly. This reduces the overall time spent going through different iterations and helps you reach your desired output faster. Moreover, this real-time feedback seems to help people understand how the various enhancement techniques affect the video. This knowledge can lead to better choices when it comes to the upscaling process, resulting in videos with a more polished and desired aesthetic.
The speed at which these previews are rendered is remarkable. They seem to be relying on the GPU to handle the rendering, which makes sense. This leads to a faster user experience overall and is essential in situations where time is crucial, such as when working on high-volume or time-sensitive video projects. The speed and responsiveness are crucial for maintaining a smooth workflow and also allowing for fast experimentation with different approaches.
On the other hand, it's surprising how much this instant feedback can influence a person's understanding of how these video enhancement algorithms work. Seeing the results as you tweak the parameters can be quite enlightening. It's a powerful tool for those who are learning about image and video processing, as it helps create a much stronger connection between the underlying algorithms and the resulting visual outputs. The real-time feedback can significantly contribute to a more intuitive and experiential understanding of these AI techniques.
Beyond that, collaboration can be greatly enhanced. Because everyone on a team can see the changes simultaneously, there's less chance of misunderstandings, and the process becomes more efficient. It can be a great way to get everyone on the same page in terms of what the end result should be. Furthermore, the ability to work across multiple video formats adds another layer of versatility. It's a good feature for the current landscape of digital media where different platforms and devices have unique format requirements.
Another point worth considering is that the real-time feature simplifies the process of experimenting with the algorithms and parameters. It turns it into a more hands-on, interactive process. You can see the results right away, which leads to more experimentation and allows users to be more creative with how they use these video enhancement tools. It's easier to find out what each setting does and determine what’s best for a specific video type or format.
Furthermore, this feature lends itself well to education. Having a real-time demonstration of how the parameters affect the resulting video can help people learn how to use and adjust these video enhancement techniques more effectively. It can be a great teaching tool that makes it more straightforward to understand otherwise complex video processing concepts. In a sense, it provides a kind of interactive lecture that makes understanding video enhancement easier for beginners and helps to solidify the understanding of more seasoned individuals.
In summary, real-time preview mode is a significant advancement for people working with video enhancement, especially those using AI-powered solutions. It allows for better control and refinement, helps with quality assurance, and makes the entire workflow much more efficient. Beyond these practical benefits, it also has the potential to improve collaboration, enhance the learning experience, and encourage experimentation with new techniques. However, it's still essential to be aware that the original video quality plays a significant role in the final output, and no AI-powered solution can overcome severe imperfections. The technology has made great strides in upscaling and enhancement, but users should still expect limitations depending on the source material.
Upscale any video of any resolution to 4K with AI. (Get started for free)
More Posts from ai-videoupscale.com: