Enhancing Image Quality Exploring the First Cell Phones Through AI Upscaling
Enhancing Image Quality Exploring the First Cell Phones Through AI Upscaling - AI Upscaling Techniques for Enhancing Early Cell Phone Photos
AI upscaling has become a valuable tool for enhancing the often-poor quality of early cell phone photos. These techniques utilize sophisticated machine learning, specifically neural networks, to analyze existing pixels and generate new ones, effectively increasing the image's resolution. This process goes beyond simple interpolation, a common older method that often leads to a loss of sharpness and detail. Instead, AI upscaling aims to maintain, and even improve, the visual quality of the original low-resolution image by intelligently filling in missing information and reducing blur.
The ability to choose various upscaling levels, like 2x or 4x, allows for a degree of customization. While many upscaling services are available online, some platforms also offer downloadable software, which can be beneficial for users who prefer offline processing. The incorporation of preview features further empowers users to review the enhanced image before committing to any changes, ensuring the final result aligns with their expectations. Though there's a tendency to hype up the 'stunning' results achievable with AI upscaling, it's important to remember that these tools still have limitations in completely reconstructing lost details from extremely low-resolution images. However, they offer a remarkable opportunity to preserve and enhance precious moments captured on the earliest cell phones, bridging the gap between past and present in a visually compelling way.
AI upscaling leverages machine learning to boost the resolution of images by analyzing existing pixels and filling in the gaps with newly generated ones. These techniques, powered by neural networks, can effectively predict and recreate missing details, significantly improving image clarity and quality without sacrificing sharpness. In contrast, traditional methods often introduce blurriness, whereas AI methods maintain visual integrity by injecting supplemental information into the original photo.
Users commonly find options for 2x or 4x upscaling when using AI tools. The core difference between AI upscaling and conventional interpolation methods lies in the sophistication of the algorithms; AI minimizes blurring and better preserves details. Various online services, such as Upscalemedia or Pixelcut, offer AI upscaling for image files in a range of formats. Vanceai stands out as it provides a downloadable program for upscaling offline, a feature useful for users who prefer not to use internet-based services.
The upscaling process often incorporates a preview feature, letting users examine the enlarged image before accepting the changes. Many of these AI tools work by automatically enhancing the image quality—sharpening and refining—while diligently managing pixel values for optimal results. The level of image enhancement that's achievable has led many of these AI platforms to boast about their "stunning" results, reflecting the highly refined processing techniques embedded within their software. There's a lot to be explored still when it comes to AI upscaling. The interplay between pixel generation and authenticity in image manipulation is a fascinating field of research in the digital realm.
Enhancing Image Quality Exploring the First Cell Phones Through AI Upscaling - Reconstructing Low-Resolution Images from the First Mobile Devices
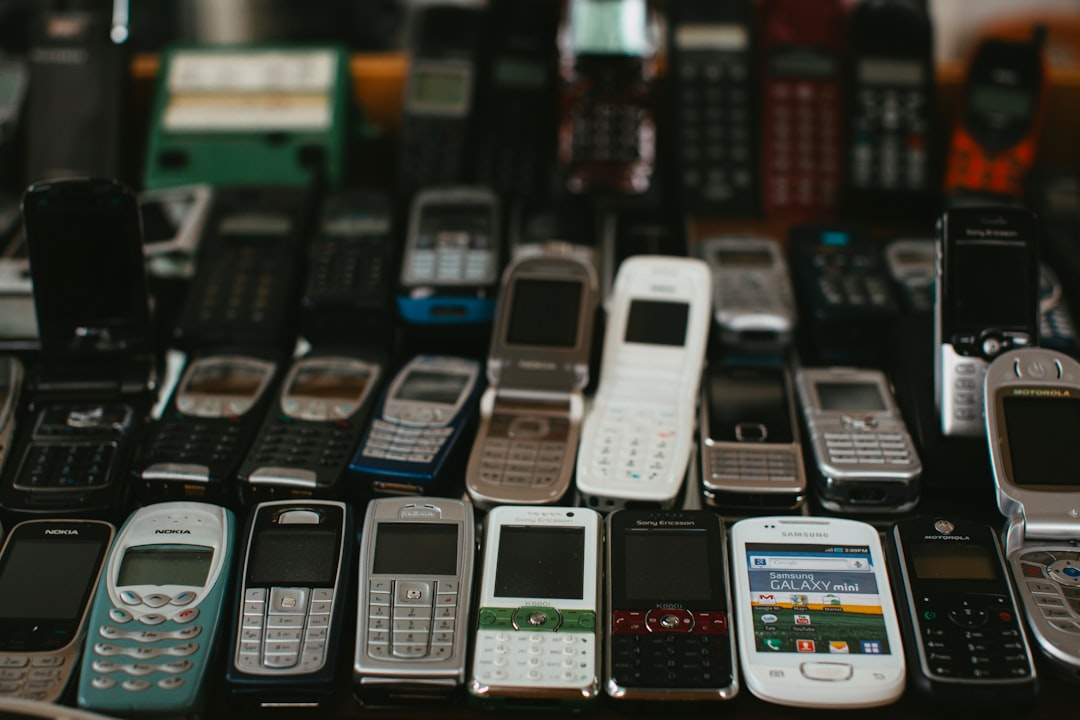
The early days of mobile photography presented a unique challenge: capturing images with severely limited resolution. Reconstructing these low-resolution images from the first mobile devices is a fascinating pursuit, made increasingly achievable by recent innovations in image enhancement. Lightweight neural networks designed for this purpose can now leverage self-extracted features from the image itself to intelligently improve quality while retaining a sense of authenticity. This approach subtly adjusts the image's characteristics using parameters derived from within the image, rather than relying on generic filters. Furthermore, hybrid models specifically tailored for low-light situations have emerged, addressing a common problem faced by these early devices. Such models employ advanced techniques within deep learning to effectively upscale the image and restore clarity lost in poor lighting conditions. The promise of these techniques is notable, though it's important to remain mindful of the intrinsic limitations of the original image data. Successfully reconstructing these early images involves finding a delicate balance between enhancing visual quality and respecting the inherent constraints of the captured moment, ensuring the end result preserves a sense of historical authenticity rather than creating a modern-looking image that may not be truthful to the original.
Reconstructing images from the earliest mobile devices presents unique challenges due to the extremely low resolutions they captured, often under 1 megapixel compared to today's hundreds of megapixel cameras. This scarcity of information makes accurately reconstructing details a complex task for even the most advanced AI upscaling techniques. It's fascinating how these AI systems can, in some cases, learn from older photographic techniques, like those employed in traditional film, to potentially replicate textures and color palettes that might be absent in the original, low-quality image.
Early image compression methods, such as JPEG, while useful for reducing file sizes, also resulted in the loss of image data. AI upscaling must navigate this loss intelligently to recover as much detail as possible. Furthermore, the training data used to teach these AI models is crucial. Often, it involves combining high-resolution images with artificially downscaled versions, effectively teaching the system how to predict and replace missing information.
Another hurdle is the inherently two-dimensional nature of these photos. Low-resolution images lack the depth information we see in more advanced cameras, which can make it harder for AI to infer attributes like shadows and highlights. This is where the clever use of 3D learning models can play a role. In the process of restoring these low-resolution images, AI often utilizes techniques such as deconvolution to combat the blurring that might stem from the limitations of the early camera sensors and lenses. This attempt to recapture clarity, lost at the moment the initial photo was taken, underscores the complexity of image reconstruction.
Many AI upscaling tools provide users with some control over the process. Options for adjusting sharpness, noise reduction, and other parameters allow individual preferences to influence the final image. It's a reminder that image quality perception remains somewhat subjective, influenced by individual tastes and expectations. A significant challenge for AI involves addressing compression artifacts like blocking and ringing, common in low-resolution images. These artifacts can be partially addressed by AI through analysis and correction, but the level of success can vary significantly based on the complexity of the artifact itself and the extent to which the AI has been trained to handle such situations.
The intricate nature of the neural networks used for AI upscaling, with their millions of parameters capable of deciphering complex image patterns, is impressive. However, this complexity does come at a cost. It raises concerns about computational load and the processing time needed for effective upscaling. We're witnessing how AI image enhancement is a truly interdisciplinary field, drawing expertise from disciplines such as computer vision, statistics, and even elements of cognitive science. The evolution of AI upscaling relies on collaborative efforts across these varied areas of research and development.
Enhancing Image Quality Exploring the First Cell Phones Through AI Upscaling - Challenges in Preserving Historical Details During AI Enhancement
### Challenges in Preserving Historical Details During AI Enhancement
When using AI to enhance old images, particularly those from the earliest cell phones, we encounter challenges in preserving their historical context. While AI can significantly improve image quality, there's a risk of losing the original nuances that make them historically significant. The potential to unintentionally alter the artistic intent of the photographer or the inherent aesthetic of the time period is a concern. Striking a balance between enhancing visual appeal and respecting the original capture is crucial. It's important to acknowledge the potential for AI to inadvertently impose a modern sensibility that might not align with the past. To truly benefit from AI enhancement while also protecting the integrity of these historical moments, a collaborative approach between the technologists developing the AI and the historians and cultural experts who understand the historical context is vital. This collaboration helps ensure that the enhancements serve to preserve and better understand the past, not create a potentially misleading interpretation of history through a contemporary lens.
When we delve into enhancing the image quality of early cell phone photos using AI, we encounter a fascinating set of challenges in preserving the original historical details. Let's explore ten intriguing aspects of this intricate process:
First, the inherent limitations of early mobile cameras, often capturing images at resolutions below 1 megapixel, present a significant hurdle. The scarcity of original data makes it difficult for AI to accurately reconstruct missing details. Essentially, we are trying to build a detailed picture with very few original pieces.
Second, early image compression methods, like JPEG, inevitably led to a loss of information. This results in compression artifacts like blocking and ringing, making it difficult for AI to separate the original intent from the compression side-effects. AI has to learn to filter out the noise from the actual photo, which isn't always easy.
Third, AI models are trained using datasets of high-resolution images paired with artificially downscaled ones. The accuracy of the training data profoundly impacts the quality of the AI's enhancements. It's like teaching a student with a limited set of examples—if the examples aren't diverse or comprehensive, the student might not learn to perform the task correctly across all situations.
Fourth, the early cell phone images lack depth information, common in more modern cameras, which makes it hard for AI to recreate subtle depth cues such as shadows and highlights. Essentially, we're working with a 2D representation of a 3D scene, making it harder to restore details that were naturally present in the original setting.
Fifth, while we strive for clarity, AI enhancements may unintentionally remove the contextual historical details that give vintage images their unique character. For instance, film grain or minor imperfections can disappear, potentially leading to a more modern aesthetic, that might misrepresent the original image's historical origins. It's a delicate balance between cleaning up and preserving history.
Sixth, images captured in low light often suffer from blurriness. AI utilizes deconvolution, a technique that attempts to reconstruct clarity, but may struggle to replicate the characteristic soft focus often present in these older photographic styles. We're trying to reconstruct a blurry photo, making sure the clarity restoration doesn't erase the artistic choice of a soft focus originally captured.
Seventh, the intricate internal workings of AI models—with millions of parameters—are impressive, but this complexity can also lead to issues. The models might overfit to the historical images, potentially distorting their authenticity during enhancement. It's like having a tool that's too specialized, it might work perfectly in one setting but not in another.
Eighth, AI upscaling depends on prediction—it fills in the missing information. This predictive approach can unintentionally introduce elements not originally present, like textures or colors that didn't exist in the original image. It's a bit like guessing the missing details, which can lead to inaccuracies.
Ninth, AI tools frequently offer users adjustable parameters for things like sharpness and noise reduction. While this customization can be useful, it introduces subjectivity into the enhancement process. Balancing user preferences with the authenticity of the historical image is a challenge—making sure that we don't lose track of the historical relevance of the image.
Finally, preserving historical details during AI image enhancement requires a collaborative effort. It necessitates expertise across different fields, like computer vision and cognitive science. This interdisciplinary approach will be crucial in refining the accuracy of AI in handling historical image processing, ensuring that the past is represented with sensitivity and care. This cross-collaboration helps ensure that technological enhancements don't replace or misrepresent historical artifacts.
Enhancing Image Quality Exploring the First Cell Phones Through AI Upscaling - Comparing Manual Restoration vs AI-Powered Upscaling Methods
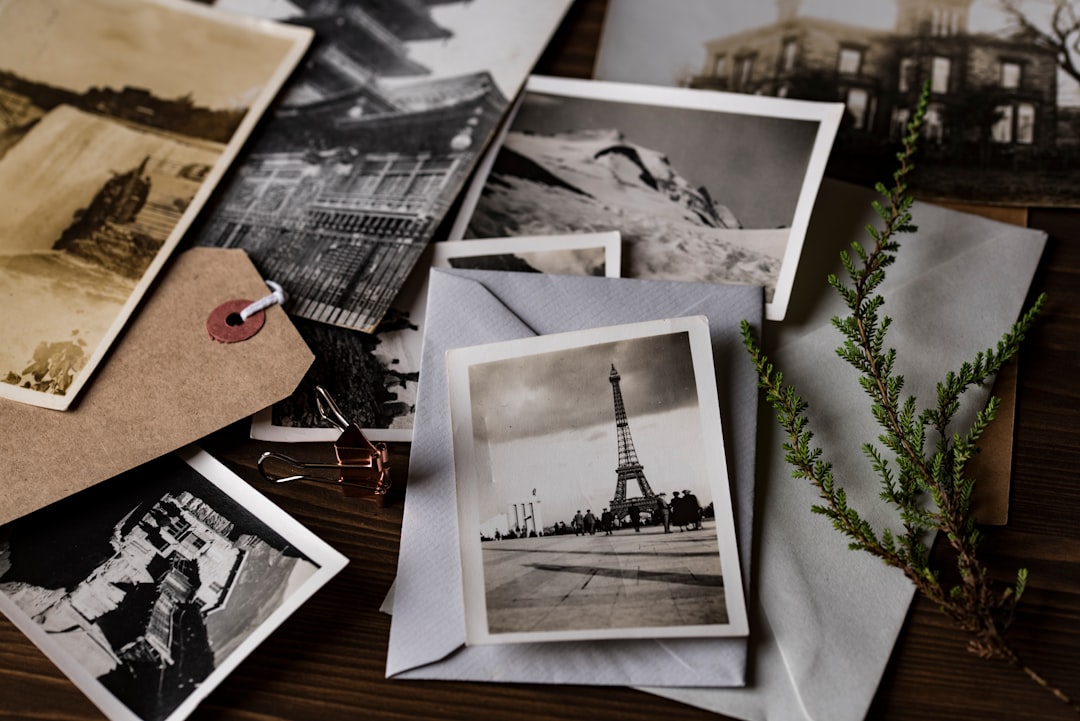
When comparing manual image restoration to AI-powered upscaling techniques, a key difference lies in the approach. Manual restoration typically involves a human expert who carefully examines and adjusts an image, prioritizing the preservation of its original qualities. This method demands a deep understanding of image editing and can be time-consuming, making it potentially costly for large-scale image enhancement projects. AI upscaling, on the other hand, utilizes complex algorithms to analyze image patterns and synthesize new pixels. This automated process can be significantly faster and more economical, often producing impressive results, especially in areas like enhancing sharpness and clarity. However, AI's reliance on predictions to fill in missing information can lead to a slight disconnect from the original image, potentially introducing textures or elements that weren't initially present. The challenge lies in finding a point where AI enhances images effectively without straying too far from the authentic look and feel of the original capture, a challenge that becomes especially important when working with historical images where maintaining the original context is vital. As AI technology continues to progress, finding this balance between image improvement and historical preservation will be an area of ongoing exploration and development.
When comparing manual image restoration with AI-powered upscaling techniques, several key distinctions emerge. Human restoration often relies on a person's skill and intuition, allowing for a more personalized touch to the enhancement process. AI methods, on the other hand, try to standardize improvements based on learned patterns, sometimes delivering impressive results but potentially oversimplifying unique details that give images their character.
While AI models can learn from huge collections of images, they may lack the understanding of artistic intent that’s crucial for historical images. This can result in misinterpretations during upscaling, where algorithms may enhance features that aren't genuinely present or historically relevant in the original photo.
AI upscaling frequently presents a vast array of options, which can confuse users. Manual restoration offers a clearer, albeit slower, path to enhancements, where skilled individuals can focus on specific details instead of broadly applied algorithms.
Interestingly, some methods blend manual and AI approaches. AI can provide a preliminary upscaled version, which is then further refined by human restoration experts. This hybrid method retains benefits from both techniques, marrying efficient AI enhancements with skilled craftsmanship.
Early cell phone photos were often plagued by compression artifacts, which reduce quality. Manual restoration has specific approaches to fix these issues, while AI might struggle if it hasn't been trained to deal with similar degradations.
Keeping the historical integrity of an image is a greater challenge with AI. Algorithms can inadvertently add modern aesthetics, which might remove culturally significant elements that define vintage photos. Manual methods generally emphasize maintaining these elements as a primary goal.
One downside to AI upscaling is that the algorithms can unintentionally introduce features that weren't originally present. This predictive nature can add textures or details that never existed, which might distort the original. In contrast, manual restoration usually focuses on improving what already exists and preserving the photo's inherent character.
The effectiveness of AI restoration is influenced by the quality and scope of the training dataset. If the training data lacks variety, the AI may mistakenly replicate flaws or patterns, leading to inaccuracies that a person with experience might avoid.
Although AI can deliver results rapidly, the intricate nature of its algorithms often requires powerful hardware for processing. Manual restoration, while slower, doesn’t require high-end computers, making it accessible to a broader range of users.
Ultimately, achieving successful image enhancement, particularly in the historical realm, hinges on collaboration between engineers, historians, and artists. This combined effort makes sure that the use of technology respects historical accuracy while utilizing state-of-the-art restoration techniques. This joint venture helps ensure that technological enhancements don’t replace or distort historical materials.
Enhancing Image Quality Exploring the First Cell Phones Through AI Upscaling - Ethical Considerations in Altering Original Cell Phone Images
When using AI to enhance old cell phone photos, we must consider the ethical implications of altering the original images. While AI upscaling can significantly improve their quality, there's a risk of misrepresenting the image's original appearance and historical context. The line between artistic license and factual accuracy becomes blurry, especially when the goal is to create visually impressive results. This is particularly relevant with historically important photos, where preserving the original image is vital.
Further complicating this, what's considered ethical image manipulation can differ across individuals and cultures. This subjectivity adds another layer of complexity when deciding what level of enhancement is appropriate. It's crucial to recognize the possibility of imposing a modern aesthetic onto images from a different era, potentially leading to a misinterpretation of history. To effectively use AI enhancement while preserving the integrity of these images, it's essential to have a collaborative process that combines technological innovation with careful consideration of the ethical implications. Only through this balance can we ensure our interactions with these historical visual records remain respectful and truthful.
When enhancing early cell phone images, we encounter a range of ethical considerations that are crucial to address. One major concern revolves around maintaining the authenticity of the original images. Improving the quality with AI could inadvertently distort the historical record, making it difficult to distinguish between the captured moment and the digitally enhanced version. This raises questions about the true nature of the image and the potential for misrepresentation.
Furthermore, there's a risk of altering the original artistic intent of the photographer. Enhancing old images might inadvertently shift the aesthetic qualities of a particular era, potentially diminishing the cultural significance of the original image. We must be careful not to impose a modern aesthetic on images from a bygone era.
The inherently low resolution of early cell phone images poses challenges for AI. These photos often have less than 1 megapixel of detail, making it hard for AI algorithms to accurately fill in the missing information. It's similar to trying to reconstruct a map with large portions missing, where details can be lost or misconstrued.
Early image compression methods, like JPEG, introduced artifacts that can further cloud the original details. AI must delicately balance restoring the image while navigating these artifacts and preventing the introduction of new flaws or alterations.
AI enhancement heavily relies on prediction, filling in missing information based on learned patterns. This predictive nature introduces a possibility of errors, such as creating textures or colors that weren't originally present in the image. This raises concerns about unintentionally distorting historical truth and the fidelity of the restored images.
The effectiveness of AI image enhancement heavily depends on the quality and breadth of its training data. If the training data is skewed towards particular styles or artifacts, it may not handle the unique characteristics of historical images effectively. A training dataset lacking in diversity can lead to unpredictable and potentially inaccurate results.
Manual restoration, though often time-consuming, offers a more personalized approach compared to AI's automation. It allows for a skilled individual to retain the subtle details that are critical for historical preservation. This highlights the importance of human judgment and expertise in this type of image enhancement.
Successfully restoring historical images relies on cross-disciplinary collaboration between engineers, historians, and art experts. This collaborative approach ensures that technological advancements are coupled with historical sensitivity, preserving the image's context and pushing the boundaries of restoration techniques.
It's crucial to acknowledge the subjectivity inherent in judging image quality. An enhancement perceived as an improvement by one person might be considered a loss of original charm by another. This subjective element complicates the process of enhancing these images and achieving universally agreeable outcomes.
Techniques like deconvolution, often used for enhancing clarity, can potentially clash with the subtle aesthetic qualities of vintage photographs. This risk exists as we try to restore clarity while preserving the original feel and intent of the image, ensuring that the restored image remains historically truthful.
Enhancing Image Quality Exploring the First Cell Phones Through AI Upscaling - Future Applications of AI Upscaling in Mobile Technology History
The future of AI upscaling within mobile technology promises a significant shift in how we interact with and understand images from our mobile history. As AI model development continues, we can anticipate even more refined techniques that not only improve image resolution but also retain the distinctive characteristics of historical photographs. This is particularly vital when working with the first cell phone images, where preserving historical authenticity while enhancing image quality is a significant concern. Future AI applications could integrate directly into mobile devices, offering a user-friendly way to restore and enhance older photos while upholding ethical considerations. However, as the boundaries between technical innovation and historical accuracy become less distinct, the requirement for collaborative efforts between different fields of study will be crucial in shaping the responsible use of AI in image enhancement. This collaborative approach will ensure technology serves to preserve the past rather than accidentally distorting it.
Early mobile camera sensors often captured images with limited depth information, a consequence of their inherently 2D nature. This poses a challenge for AI systems as they attempt to accurately recreate the spatial relationships and details originally present in a scene. It's as if the AI is trying to build a 3D puzzle with only a 2D image to guide it, leading to complexities in the upscaling process.
Early image formats like JPEG, while useful for compressing data and reducing file size, also resulted in a loss of fine detail and introduced artifacts like blocking and ringing. AI upscaling models have to intelligently navigate this loss during image restoration, attempting to differentiate genuine image features from these imposed artifacts. It's a bit like a detective trying to find the true image amidst a collection of clues and distractions.
Interestingly, AI upscaling techniques can draw upon concepts from traditional photography, enabling neural networks to reproduce the unique textures and color palettes often associated with vintage photographs. This interdisciplinary approach highlights the evolving nature of AI image processing and its ability to leverage established methods from other fields.
While the complexity of AI upscaling algorithms is impressive, it can lead to computational bottlenecks. As these algorithms become more sophisticated, processing times for image enhancement can increase, requiring hardware with significantly more processing power than is typically found in a standard mobile phone. This reality can make AI upscaling on mobile devices a challenge, highlighting the need for optimization and efficiency improvements in AI software.
It's also counterintuitive that the quality of AI-generated enhancements is heavily dependent on the breadth and variety of its training data. If the dataset used to train the AI doesn't accurately reflect the diversity of historical photographic styles and imperfections, the system might generate inaccurate or unrealistic results. Effectively, the AI learns from the information it's given, and if that information isn't representative of the historical context, it's likely to produce errors.
Deconvolution, a common technique employed in AI upscaling to enhance sharpness, can sometimes be at odds with the soft focus aesthetic often found in older photographs. This conflict between clarity and artistic intent can result in the AI inadvertently altering the original photographer's vision. It's a tightrope walk between restoring sharpness and respecting the original feel of a vintage image.
The use of hybrid approaches—combining AI-powered upscaling with human-driven refinement—is becoming increasingly prevalent. This collaborative method leverages the efficiency of AI algorithms with the artistry and intuition of skilled restorers. This allows for image enhancement that combines speed and accuracy with a strong emphasis on preserving historical authenticity.
The very low resolution of early mobile images, often less than 1 megapixel, presents a unique challenge for AI upscaling algorithms. Restoring these low-detail images requires advanced techniques to effectively fill in significant information gaps while preserving a sense of historical accuracy. The challenge is akin to filling in the missing sections of a jigsaw puzzle with very few original pieces.
AI upscaling tools also exhibit variable response times depending on the complexity of the image and chosen enhancement parameters. This fluctuation can lead to unexpected delays, especially frustrating when trying to share images quickly through social media platforms. The speed of upscaling can vary widely, creating situations where a user may be waiting an extended period of time for a result.
The field of AI upscaling is showing promise through the use of 3D learning models, which aim to address the inherent two-dimensionality of early photographs. These models potentially enhance the restoration process by enabling the AI to infer depth and dimensionality, ultimately producing more comprehensive and realistic-looking enhanced images. It's still an evolving area of research, but it shows that the quest to improve AI upscaling continues, with new ideas and methods continually being explored.
More Posts from ai-videoupscale.com: