Upscale any video of any resolution to 4K with AI. (Get started for free)
Exploring Topaz Video Enhance AI's 24 Temporally Aware Models for Video Upscaling
Exploring Topaz Video Enhance AI's 24 Temporally Aware Models for Video Upscaling - 24 AI Models for Video Enhancement Explained
Topaz Video Enhance AI offers a diverse toolkit of 24 distinct AI models, each tailored for specific video enhancement scenarios. This array of models allows users to upscale video resolutions with impressive versatility, handling the complexities of different source materials. For example, the software intelligently differentiates between interlaced and progressive video formats, selecting the appropriate model for optimal results.
The introduction of models like Chronos Fast demonstrates a significant advancement in processing speed, especially for high-resolution footage with rapid motion. This model, among others, significantly accelerates processing times compared to older iterations, making the entire enhancement process much faster. Conversely, the Nyx model is focused on tackling challenging footage, namely video shot in low-light conditions. This model excels at restoring clarity and reducing noise commonly associated with low-light captures, alongside addressing the effects of compression.
Users aren't confined to a single choice but can freely explore and compare the various model outputs directly within the Topaz Video Enhance AI interface. This hands-on approach empowers users to select the model that aligns best with the precise enhancements they're seeking for their project. Moreover, the software's continuous development ensures the benefits of ongoing optimizations extend to users, including notable performance gains for those using Apple's M1 chip-based systems.
Topaz Video Enhance AI offers a diverse set of 24 AI models, each tailored to specific video characteristics and enhancement goals. The software aims to cater to a wide range of upscaling needs, handling everything from SD to 8K resolution. The model selection process allows users to account for video format differences, such as progressive or interlaced footage. Recent additions like Chronos Fast are specifically geared towards high-resolution, fast-paced content, demonstrating substantial performance gains compared to previous iterations.
The Nyx model stands out with its emphasis on handling low-light conditions, demonstrating proficiency in managing noise stemming from high ISO settings or compression artifacts. Users are presented with an intuitive interface that enables direct comparison of various model outputs, facilitating informed decision-making for specific video enhancement scenarios.
Performance has also been a focus, particularly on Apple Silicon hardware. The current version leverages the M1 chip to deliver a notable speed boost. While marketed as a tool for professionals, the automatic updates, covering both major and minor improvements, suggests a focus on user convenience and ongoing development. These models collectively cover the most common video enhancement tasks, encompassing stabilization, smoothing, detail enhancement, and noise reduction, giving the software a degree of versatility. However, it is important to remember that each model's effectiveness can vary depending on factors like the input footage quality, content type, and movement in the scene. There might be cases where the results do not meet the high expectations of specific video enhancement tasks.
Exploring Topaz Video Enhance AI's 24 Temporally Aware Models for Video Upscaling - Temporal Awareness in Upscaling Technology
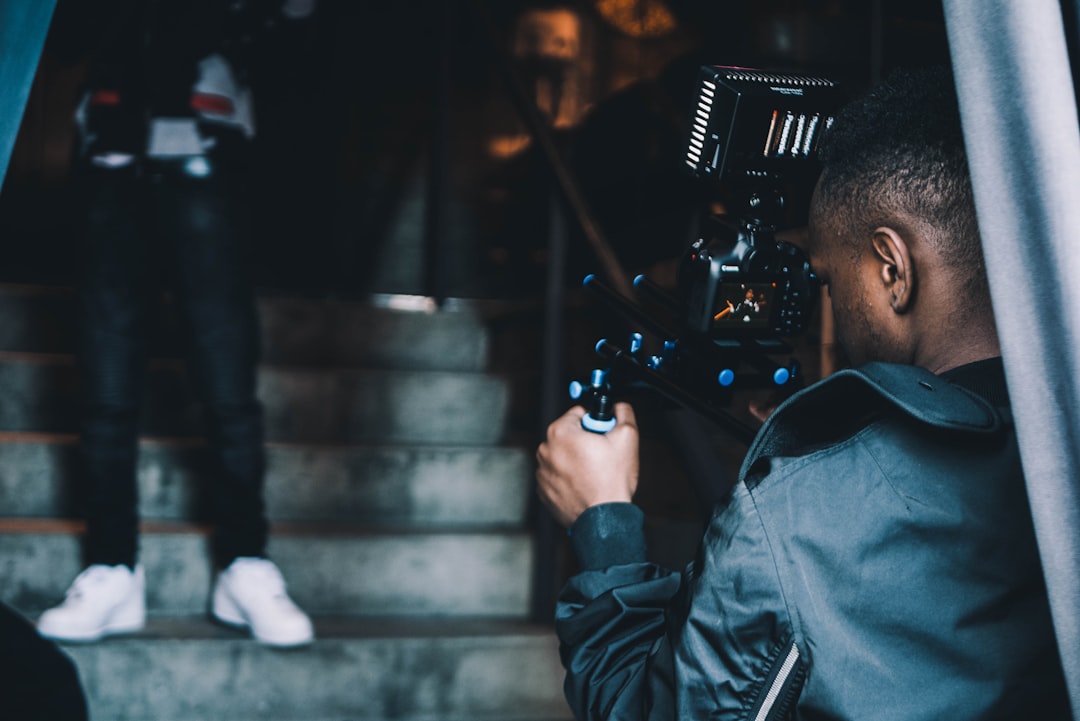
Upscaling technology's effectiveness hinges on its ability to understand the temporal aspects of video—the changes and movement across frames. Traditional methods often struggle to seamlessly handle these transitions, sometimes leading to artifacts or inconsistencies that disrupt the natural flow of motion. However, "temporal awareness" in upscaling algorithms specifically tackles this challenge. These algorithms are designed to recognize and maintain consistency across the time dimension, ensuring smooth transitions and minimizing blurring or ghosting, especially in high-resolution or fast-paced footage.
The importance of temporal awareness is growing as upscaling capabilities increase. It's no longer enough to simply increase resolution; the output also needs to be visually coherent over time. Achieving this fidelity, especially in demanding situations, necessitates a deeper understanding of how motion impacts the video's appearance. This sophistication in temporal awareness signifies a major advancement in video enhancement, making it possible to tackle even complex scenarios with more effective results. The ultimate goal is not only improved resolution, but a seamlessly integrated visual experience that preserves the integrity of the original content across every frame.
Maintaining a sense of time, or temporal awareness, in upscaling technology is crucial for generating believable and smooth results, especially in dynamic scenes. This awareness relies on sophisticated motion estimation algorithms that dissect individual frames to anticipate the movement of objects within the video. This predictive capability is vital for producing seamless transitions and minimizing blurriness or jagged edges, particularly when dealing with fast-paced content.
The advent of temporally-aware models has fundamentally changed how artifacts are managed during frame interpolation. Traditional upscaling often resulted in visual anomalies like ghosting or blurring, but these newer approaches mitigate these problems considerably. By cleverly incorporating temporal information into the interpolation process, AI-driven upscaling generates more natural and accurate frame sequences.
Interestingly, different models within Topaz Video Enhance AI employ various temporal window sizes. Some models analyze a wider range of surrounding frames to grasp the broader context of movement, while others may focus on the immediately preceding frame for quicker processing and a more localized analysis of detail reconstruction. This variation in approach is designed to optimize for different kinds of video content.
This temporal optimization is not limited to live-action footage. Animated scenes also benefit from accurate motion predictions and upscaling capabilities, showing how the technology can intelligently adapt to diverse video characteristics. The fidelity achieved with animation upscaling can be surprisingly detailed and effective, highlighting the flexibility of the underlying AI.
Beyond improving visual quality, maintaining temporal consistency is fundamental for preserving the authenticity of a video. The movement captured within the original footage contributes significantly to the way we experience the video, and disruptions to this inherent motion can harm the overall impact. So, temporal awareness isn't just about sharpening things up, but also keeping the inherent 'feel' of the video intact.
Some models within this AI software are specifically crafted for variable frame rate (VFR) footage. This ability to handle different types of input sources is important as videos shot at inconsistent frame rates are common in various content creation workflows. By dynamically adapting to these changes in frame timing, these models produce consistent results without sacrificing visual quality or introducing artifacts.
The integration of temporal awareness comes at a computational cost. Efficient implementation often leans heavily on advanced hardware capabilities like high-powered GPUs for real-time or near-real-time processing. This hardware dependency can potentially limit access to the best features for some users, depending on their computing resources.
While impressive, there is a cautionary note with temporal upscaling. Overly aggressive application of temporal smoothing can lead to a loss of intricate details, potentially making static elements of the video look overly blurry. Carefully selecting the model and refining settings is critical for achieving the desired balance of smooth motion and fine detail.
Improvements to AI-driven temporal models usually rely on massive datasets of video sequences. These datasets expose algorithms to intricate patterns of movement across diverse scenes and genres. Through exposure to such a massive range of data, the AI can develop the ability to generate remarkably coherent and visually appealing frame sequences.
Furthermore, the user interface plays a crucial role in accessing these capabilities. Interactive previews allow for real-time comparisons, helping users understand the impact of temporal adjustments on the overall video quality. This interactive evaluation allows users to make nuanced choices in finding the right model and fine-tuning parameters for achieving desired outcomes.
Exploring Topaz Video Enhance AI's 24 Temporally Aware Models for Video Upscaling - Comparative Analysis of Interlaced vs Progressive Footage Processing
Video content can be processed in two main ways: interlaced and progressive. Interlaced video, a legacy method, combines two separate fields, or sets of lines, to form a complete frame. While efficient for its time, this approach often results in lower image quality and the potential for artifacts such as flickering. Progressive video, on the other hand, displays each frame in its entirety, producing a smoother, more natural viewing experience without the need for combining fields. This method is generally preferred in modern video content due to its superior visual quality. AI-powered video enhancement tools like Topaz Video Enhance AI are equipped to handle the nuances of these differing video formats. They incorporate specialized models that cater to each type, striving to maximize video quality in diverse scenarios. With the increase in access to older video formats, understanding the distinction between interlaced and progressive processing is increasingly important for those seeking to restore or enhance video quality, ensuring that the original content is preserved and presented in the best possible light.
Interlaced video, which captures odd and even lines of a frame separately, can pose challenges for upscaling algorithms. If not carefully managed, it can lead to visible artifacts like combing or flickering in the upscaled output, potentially degrading the visual experience. In contrast, progressive video, capturing all lines simultaneously, provides greater temporal consistency, generally yielding smoother results during upscaling since interlacing artifacts aren't introduced.
While interlaced video might appear sharper on standard displays due to how it's presented, advanced AI upscaling often reveals limitations in the detailed information embedded in the video. This can highlight a disparity between perceived quality and the underlying data available, especially compared to the benefits of progressive formats.
The temporal awareness employed in AI upscaling is vital for interpolating frames smoothly, but the effectiveness of this process can be hindered by the nature of interlaced video. This begs the question of whether interlaced formats will remain relevant in video production and consumption workflows.
The chosen AI model can significantly affect the quality of upscaled video depending on whether the source footage is interlaced or progressive. AI models with varied processing techniques might produce distinct results, so understanding and adapting to these differences is essential for optimal outputs.
Some AI approaches use deinterlacing algorithms to convert interlaced footage into progressive before upscaling. However, this conversion process can also introduce its own set of artifacts, making the choice of a processing approach crucial to preserving visual integrity.
The computational load can differ considerably between processing interlaced and progressive formats. Interlaced video demands additional computations for correction and interpolation, which can potentially impact processing speeds.
Intriguingly, even models trained primarily on progressive footage can encounter difficulties when processing interlaced sources because they might not be adequately prepared for the unique characteristics and artifacts present in interlaced formats. This underscores the need for distinct processing pathways designed specifically for each type.
The focus on addressing the disparities between interlaced and progressive formats reflects a broader trend in video technology. It's a testament to a drive towards achieving the highest visual fidelity from any source material, regardless of its original quality.
Perhaps a promising route would be to explore hybrid approaches. Combining models specialized in handling both interlaced and progressive video might lead to superior results. This could offer a powerful solution by merging the strengths of both approaches into a more holistic video enhancement strategy.
The exploration of these techniques represents a fascinating intersection of AI and video processing. Continued research into overcoming the challenges posed by interlaced content will likely play a vital role in shaping the future landscape of video upscaling.
Exploring Topaz Video Enhance AI's 24 Temporally Aware Models for Video Upscaling - Integration with DaVinci Resolve via OpenFX Plugin
Topaz Video Enhance AI's integration into DaVinci Resolve via an OpenFX plugin represents a notable step forward for video editors. Now, users on both Windows and macOS can readily access Topaz's array of AI-powered enhancement tools directly within Resolve. This includes all the currently available models, even advanced ones like Motion Deblur.
The plugin seamlessly integrates into Resolve's environment, creating a dedicated "Topaz Video AI" folder within the Effects & Presets panel. This provides a streamlined experience for applying and adjusting the AI effects. It also seems Topaz Labs sees this partnership as a way to improve the overall post-production process, especially benefiting DaVinci Resolve users who can take advantage of its node-based setup for visual effects and grading.
While the direct access to a plethora of AI enhancement tools is convenient, it's crucial to understand that these tools aren't a magic bullet. Their efficacy heavily depends on the initial video quality and the specific scene. Furthermore, future updates are expected to introduce further enhancements, such as advanced frame interpolation and upscaling options. However, the onus remains on the user to employ these capabilities judiciously to avoid introducing undesired alterations to their videos. The goal remains to improve the video, not necessarily create something entirely different.
Topaz Video Enhance AI's integration with DaVinci Resolve through an OpenFX plugin offers a compelling path for video enhancement directly within the editing environment. It's now possible to apply the full range of Topaz's enhancement models, including the Motion Deblur tool, directly within Resolve on both Windows and macOS. Interestingly, the plugin is automatically included during Windows installations, while macOS users have to manually enable it through a checkbox. After installation, a dedicated "Topaz Video AI" folder appears in Resolve's Effects & Presets panel, making it easy to access.
Applying the plugin is as straightforward as selecting a layer and then choosing a model from the panel. This then unlocks controls for that specific AI model, including keyframing capabilities. However, it's noteworthy that currently, all models in this implementation operate at a 1x scale, which could limit upscaling options for some users.
Looking ahead, Topaz Labs plans to include their upcoming Aion Frame Interpolation model in version 4.2. This feature promises more accurate motion estimation, which could be a valuable addition to Resolve workflows. Further down the line, the next version of Topaz Video Enhance AI will likely introduce enhanced upscaling, 3D LUT export support, and further improvements to slow-motion and motion deblurring features within the plugin framework.
The plugin integration essentially provides access to a subset of Topaz's features via the OpenFX section within Resolve's effects tab. The Topaz Labs team prioritizes tight integration with editing software, and it’s clear that they're strategically leveraging DaVinci Resolve's node-based approach for both visual effects and color grading, which aligns with the current trend towards hybrid workflows in video production. This tight coupling could potentially offer workflows that are hard to beat but does require careful monitoring of resource usage since applying multiple complex AI models on demanding video can significantly impact rendering performance. It’ll be interesting to see how these workflows are embraced by the community in the coming months, as it's still quite early in the integration lifecycle.
Exploring Topaz Video Enhance AI's 24 Temporally Aware Models for Video Upscaling - Real-world Applications in Legacy Video Restoration
Legacy video restoration often faces the challenge of degraded quality inherent in older formats. AI-powered tools like Topaz Video Enhance AI are increasingly valuable in this context, offering the ability to upscale video resolution while preserving fine details and minimizing unwanted artifacts. The 24 unique models within the software are particularly beneficial, allowing users to tailor their restoration efforts to the specifics of different video types, such as interlaced or progressive formats. These models are crucial for achieving optimal results and enhancing visual clarity. However, there's a need for caution in utilizing these powerful tools, as excessive processing can lead to an unintended loss of the original footage's character. Striking a balance between quality improvement and preserving the authentic qualities of the source material is critical when restoring historical video content. This delicate balance is becoming increasingly important as creators seek to restore and share valuable, aged video archives.
The realm of legacy video restoration has historically relied on manual methods, but the advent of AI-powered upscaling models, like those found in Topaz Video Enhance AI, marks a substantial shift. These models automate processes that once demanded specialized expertise and considerable time, promising a more streamlined approach to restoring old footage.
Restoring vintage films often involves grappling with worn, degraded sources. AI models, particularly those employing machine learning, show promise in these situations. Their ability to predict missing information allows them to reconstruct details that might be lost due to damage or deterioration, but the quality of this reconstruction can depend heavily on how well the model's training data matches the characteristics of the footage being restored.
The concept of temporal awareness in restoration tools significantly impacts the processing of older video formats, such as 8mm or VHS tapes. Through clever interpolation techniques, temporal awareness helps maintain consistency across frames, reducing some of the distortion and flicker common in older recordings.
Video formats like interlaced content, where odd and even lines are captured separately, pose challenges for restoration. Advanced models can not only improve image quality but also employ deinterlacing techniques to convert these formats to progressive, simplifying the restoration pipeline.
However, the integration of correction algorithms within restoration software can sometimes introduce artifacts during upscaling of older footage. While these algorithms strive to enhance visual fidelity, misjudging the amount of correction applied can lead to an overly sharp appearance that may not accurately reflect the original material.
Legacy media often features lower resolutions and frame rates, but AI-driven techniques can upscale this footage to higher resolutions and smoother frame sequences. Yet, a key challenge remains in preserving the original aesthetic of the video, which might necessitate fine-tuning parameters to avoid creating an artificial or overly processed look.
An intriguing area of restoration work involves the effective upscaling of animated footage from earlier eras, where AI models can improve character definitions and backgrounds. This task contrasts with live-action restoration, highlighting the need for adaptable algorithms that are suited to a range of source materials.
Training video enhancement models typically relies on large datasets that expose the algorithms to complex movement patterns and common artifacts found in historical footage. However, if these datasets are primarily composed of modern video, the restoration of older content may not receive optimal results.
AI-enhanced video restoration, particularly at high resolutions, can be computationally demanding. This often necessitates powerful hardware, potentially creating barriers for smaller organizations or independent restorers who may rely on more affordable technology.
Finally, collaborative integrations, such as the combination of Topaz's AI software and editing suites like DaVinci Resolve, foster sophisticated workflows for video restoration. However, these integrations also require users to carefully manage system resource allocation. Failure to do so can impede productivity, especially when applying multiple restoration effects and processing complex video sequences.
Exploring Topaz Video Enhance AI's 24 Temporally Aware Models for Video Upscaling - Future Developments in AI-driven Video Upscaling
The future of AI-driven video upscaling points towards increasingly sophisticated capabilities. With ongoing advancements in processing power, we can expect a greater emphasis on real-time upscaling that preserves intricate details while minimizing unwanted artifacts. A key area of development will likely be improving temporal awareness, enabling algorithms to better understand and manage motion across frames. This could lead to smoother and more consistent video outputs, particularly in scenes with complex or rapid movement. Furthermore, as AI models are trained on increasingly diverse datasets, they will likely become better at handling a broader spectrum of video types, encompassing everything from vintage footage to fast-action sequences. This expanded adaptability will make them more useful for a wide array of restoration and enhancement projects. However, alongside these exciting advancements, it's crucial to be mindful of the potential for over-processing, which can unintentionally diminish the original character of the video. Striking a balance between enhanced quality and preserving authenticity will continue to be a challenge as AI upscaling technology matures.
1. **AI Upscaling and Legacy Film**: The development of AI-driven video upscaling, particularly models with temporal awareness, holds exciting possibilities for restoring older film formats. Their ability to reconstruct lost detail in degraded footage could help revitalize older movies and videos. However, we need to consider how well the model's training data aligns with the unique characteristics of vintage films.
2. **Adapting to Variable Frame Rates**: AI upscaling is increasingly adept at handling video with variable frame rates (VFR). This is significant because it addresses a growing trend of mixing frame rates within projects, allowing for smoother editing and better compatibility without sacrificing the visual quality.
3. **Computational Demands of Temporal Enhancement**: While effective, the integration of temporal awareness into AI upscaling leads to increased computational demands. This reliance on powerful hardware, especially GPUs, for real-time processing could be a barrier for individuals with less robust systems. We're likely to see a widening gap between those who can readily leverage these tools and those who cannot.
4. **Training Data Diversity**: The effectiveness of AI upscaling models hinges on the breadth and variety of data used for training. If training data lacks sufficient representation of older formats, the upscaling might not perform optimally when applied to legacy video content. This highlights the need for developing training methods tailored to specific historical formats.
5. **Adapting to Diverse Video Types**: A crucial direction in AI upscaling development is creating models capable of dynamically adapting to various source materials. This flexibility is key for minimizing artifacts and maximizing output quality, irrespective of whether the source is interlaced or progressive. We need to explore algorithms that learn to recognize these characteristics.
6. **Tackling Compression Artifacts**: The latest upscaling techniques are starting to directly address the artifacts often associated with video compression, like blocking and banding. This means we can expect cleaner and more detailed outputs, particularly for videos originally captured or stored in compressed formats.
7. **User Control: Still Vital**: While AI automates a lot of upscaling work, human input remains important. Users will need to continue to fine-tune settings based on the specific features of their video to achieve a balance between enhanced resolution and a natural aesthetic. We are likely to see the development of more intuitive controls for adjusting these aspects.
8. **Real-time Feedback through Interfaces**: The inclusion of interactive previews in the AI software allows for real-time feedback, empowering users to iteratively refine the upscaling process. This is a boon for professionals working in editing environments where quick and accurate adjustments are vital.
9. **Over-Processing Concerns**: There's an inherent danger in over-applying AI upscaling. Overly aggressive enhancement can distort the original visual character of a video, making the output appear artificial. It's crucial to develop algorithms that understand a more subtle approach and can prevent this undesired outcome.
10. **Advanced Motion Estimation**: Recent advancements in AI-driven upscaling models leverage more sophisticated motion estimation techniques. These algorithms better predict and incorporate movement across frames, greatly improving the fluidity of fast-moving scenes, a long-standing hurdle for upscaling methods. This represents a leap forward in capturing the subtleties of motion.
Upscale any video of any resolution to 4K with AI. (Get started for free)
More Posts from ai-videoupscale.com: