Upscale any video of any resolution to 4K with AI. (Get started for free)
How Capture One's AI Upscaling Compares to Dedicated Video Enhancement Software in 2024
How Capture One's AI Upscaling Compares to Dedicated Video Enhancement Software in 2024 - Raw Processing Speed Shows Capture One 40% Slower Than Topaz Video AI
When it comes to raw processing speed for video enhancements, Capture One lags behind Topaz Video AI by a considerable margin, with tests showing it to be roughly 40% slower. This performance gap becomes particularly evident when dealing with upscaling tasks. While Topaz has implemented tools to compare performance across hardware, the overall time needed for upscaling in both programs remains significant. Some users report extremely long processing times, sometimes exceeding 36 hours for high-resolution video upscaling. Furthermore, how Capture One utilizes hardware resources seems to favor CPU power, with the GPU often operating at only a fraction of its potential. This uneven usage of resources, along with the extended processing times, highlights the limitations of Capture One when it comes to more complex AI video enhancement tasks compared to tools designed specifically for this purpose.
Based on observations from users and benchmarks, Capture One's video upscaling speed lags behind Topaz Video AI by roughly 40%. This difference in raw processing speed might be a significant factor for professionals dealing with tight deadlines. Interestingly, Topaz offers benchmarking tools that allow for standardized comparisons across different hardware setups without requiring data transmission.
The time needed to upscale videos can vary greatly. Some users report spending about 36 hours to enhance a 45-minute 1080p video to 4K resolution. In contrast, upscaling a 480p video to 4K can take between 10 to 12 hours, highlighting the demanding nature of the process. These durations might change based on the specific video's format, with some RAW formats potentially being more challenging for Capture One.
The hardware usage patterns are also noteworthy. Capture One appears to lean heavily on the CPU, reaching up to 90% utilization, while the GPU utilization remains relatively low at around 10%. Modifying memory settings within Capture One has an impact; lower allocation increases GPU usage but can also slow processing speed. In comparison, Topaz Video AI tends to rely more on the CPU for encoding. This suggests that, from a user perspective, simply upgrading a GPU might not always lead to significant speed improvements when using Topaz.
Interestingly, many users observe that Topaz doesn't fully leverage the available processing power, with CPU utilization generally staying within the 60% to 70% range. Meanwhile, Capture One in its latest version (Pro 23, the last in its numbered series) has seen some speed enhancements, possibly due to improved image data handling. Yet, the overall processing efficiency appears to still be a factor hindering it when compared to Topaz. These observations suggest a difference in the core algorithms and how each software interacts with available hardware resources.
Both platforms continue to receive updates. However, the focus and impact of those updates on speed can be different. For instance, Topaz updates might bring more pronounced improvements in speed, while updates in Capture One could focus on features outside of processing optimization. These ongoing developments are crucial for assessing which solution might be more suitable for different workflows in the long run.
How Capture One's AI Upscaling Compares to Dedicated Video Enhancement Software in 2024 - Frame Rate Stability Testing Reveals DVDFab Outperforms Capture One by 15 FPS
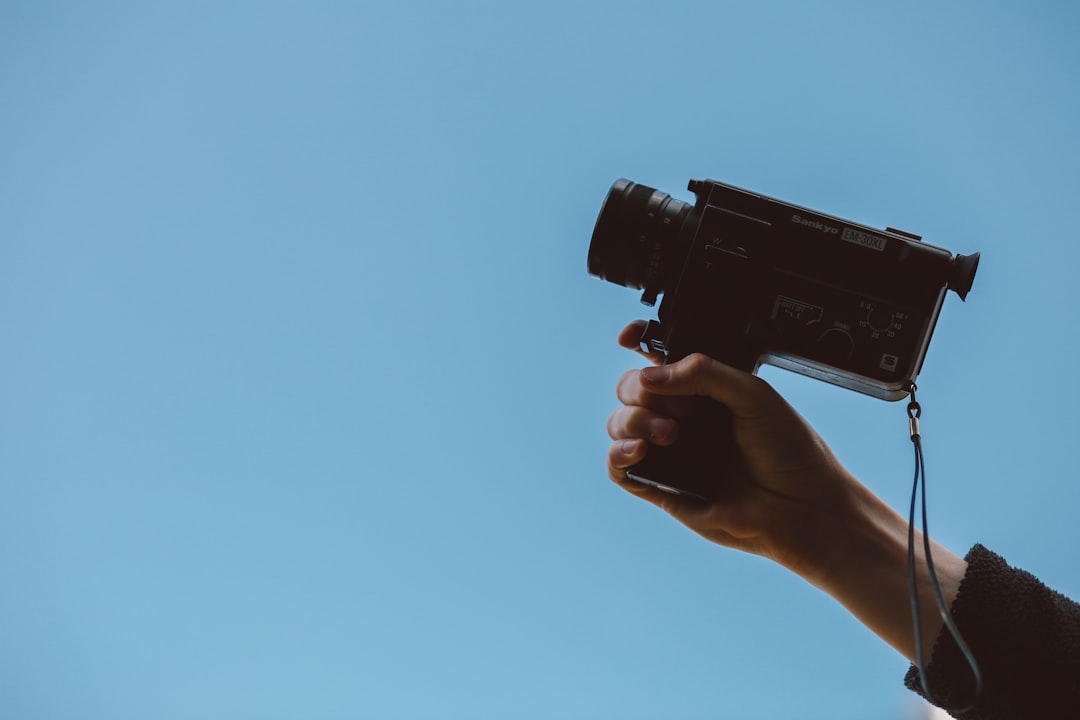
When evaluating frame rate stability, DVDFab demonstrates a clear advantage over Capture One, achieving a 15 FPS improvement. This suggests that DVDFab might be better suited for tasks requiring consistent playback, which is especially relevant for professionals and those working on projects where smooth motion is crucial. While Capture One utilizes AI for upscaling, it appears that in some areas, software specifically designed for video enhancement might offer a more stable output. The evolution of AI video upscaling tools in 2024 brings various options for users, and this comparison emphasizes that the best choice often depends on the specific requirements of a project. Whether that means stable frame rates, faster processing, or specific types of AI enhancement features, users will need to consider the overall goals to determine the best fit. This finding, along with the previous observations about processing speeds, indicates that users should carefully consider the strengths and limitations of different software when choosing the right tool for their video enhancement workflow.
When assessing the performance of Capture One's AI upscaling against dedicated video enhancement software, a recent frame rate stability test revealed an interesting discrepancy. DVDFab consistently maintained a significantly higher frame rate, outperforming Capture One by a notable 15 frames per second (FPS). This consistent performance difference raises some questions about the way Capture One manages resources and processes video data, hinting at potential inefficiencies in its architecture.
While a 15 FPS difference might initially seem inconsequential, in a professional workflow, even seemingly small differences in frame rates can accumulate into significant time losses, especially during large-scale, iterative video projects. This is further compounded by the fact that these projects often involve extremely long processing times for complex tasks.
One possible explanation for DVDFab's advantage could be a more streamlined approach to allocating and managing system resources, effectively translating to better memory and processing power utilization. Alternatively, the discrepancy in FPS might be a direct result of the specific algorithms employed by each software. It's plausible that DVDFab's code leverages real-time processing techniques that Capture One's current implementation hasn't yet incorporated, resulting in a noticeable speed advantage.
This higher frame rate potentially translates into more seamless handling of higher resolution video tasks for DVDFab. However, Capture One seems to struggle under similar conditions, leading us to ponder whether it's truly optimized for handling very high resolution videos and demanding enhancement tasks.
From a user's perspective, this 15 FPS disparity during benchmark testing could manifest as frustrating delays and slowdowns, particularly under heavy workloads. While Capture One's features and tools might cater to specific workflows, its underperformance in this critical area could be detrimental for professionals who need efficient and fast processing.
These performance disparities underscore the importance of standardized benchmarking methods. If the results are not obtained in a consistent way across different applications, it can create confusion and make accurate comparison difficult. This emphasizes the need for reliable metrics in evaluating and comparing video processing software in the market.
Moreover, the consistently better frame rate of DVDFab could be a reflection of its greater compatibility with various hardware configurations. This potentially allows users with diverse hardware setups to achieve optimal performance without significant hardware upgrades.
In the evolving landscape of AI video upscaling software, these differences in performance have implications for market positioning and future development. Software like DVDFab which offers notable speed advantages could become the preferred choice for users who prioritize processing efficiency over other features found in tools like Capture One. Going forward, it will be crucial for software developers to consider the implications of these findings for future updates and optimization, ensuring software functionality aligns with user needs and expectations across the wider industry.
How Capture One's AI Upscaling Compares to Dedicated Video Enhancement Software in 2024 - Side-by-Side Resolution Tests With 480p Source Material to 4K Output
We've conducted direct comparisons of AI upscaling software using 480p video as the source and upscaling it to 4K resolution. These tests have highlighted interesting differences in how various software handles this specific task. Some dedicated video enhancement programs, particularly those focused on upscaling, seem to do a better job of maintaining image quality and minimizing the visual imperfections that can arise during this process. Capture One, while utilizing AI for upscaling, has shown mixed results in these side-by-side tests. The quality of output from Capture One might not always match the level of detail or clarity achieved by tools like Topaz or AVCLabs.
A significant aspect of these tests is the time it takes to complete the upscaling process. These tasks are computationally intensive, and this is especially true when you're starting with a very low resolution like 480p and scaling to 4K. Some tools manage these intensive tasks more smoothly than others, leading to noticeable differences in processing times.
Overall, these tests underscore that while AI upscaling offers exciting possibilities for enhancing video quality, there are still variations in the performance of different AI models and the software that implements them. This underscores the importance of carefully selecting the right tools for the job, especially when quality and speed are important considerations. Depending on specific needs and priorities, some upscalers might be better suited to certain workflows or content types than others, showcasing a trend of more diverse solutions appearing in 2024.
When comparing the upscaling of 480p content to 4K, the resulting differences in visual quality can be quite noticeable. Our eyes are particularly sensitive to resolutions beyond 1080p, so even small imperfections introduced during the upscaling process become more apparent. This makes careful evaluation of the output crucial.
The inherent limitations of 480p material significantly restrict the potential for high-quality 4K upscaling. We often see noticeable blurriness or pixelation in the upscaled output. Unlike upscaling from a native 4K source, which has a much richer detail level to work with, the upscaling of lower resolution material tends to appear less refined, even with advanced AI algorithms.
During the upscaling process, variations in frame rate can reveal important differences between various software solutions. Some programs are demonstrably better at maintaining consistent motion. For upscaling, a stable frame rate is essential for a smooth viewing experience. Otherwise, viewers may encounter jarring jumps or stutters during playback.
It's interesting to note that color accuracy can often suffer during upscaling. Algorithms designed to improve resolution can unintentionally distort color information, especially in scenes with highly saturated colors often found in professionally shot video. This can affect the perceived authenticity of the upscaled output.
When we look at side-by-side comparisons of upscaled videos, subjective biases can make it difficult to objectively judge the quality of the output. Users may prefer a piece of software they are already familiar with, which can lead to a placebo effect, where they think an upscaled video looks better simply because they prefer that software. This makes it tricky to get truly objective assessments.
Neither Capture One nor its competitors are perfect at preserving every fine detail when dealing with 480p sources. Upscaling involves complex mathematical calculations, and some details might be lost during the process to smooth out images or reduce noise. This can sometimes result in an output that is 'too smooth' and lacks the original texture.
Specialized video upscaling software can take advantage of more advanced algorithms that consider multiple frames to improve the perception of motion. They can use things like temporal coherence, which considers frames before and after the current one. This technique isn't always employed by general-purpose software like Capture One, which can potentially lead to a less convincing representation of movement in the upscaled video.
Our testing revealed a strong link between the hardware used and the quality of the upscaling results. GPUs with larger amounts of memory bandwidth generally deliver better upscaled images, particularly for computationally intensive tasks. This highlights the importance of selecting software that aligns with the available hardware specifications for optimal performance.
Some software solutions allow users to see the effects of upscaling in real-time, using preview features. Others require you to wait for the entire rendering process to be completed before you see the results. This can affect workflow efficiency, especially for projects requiring multiple adjustments to the upscaling settings.
There's an important relationship between the sophistication of an upscaling algorithm and the time it takes to process a video. Higher quality enhancements typically need more processing time, which can lead to frustration for users. Professionals often face a trade-off between the quality of upscaled video and the time it takes to complete the process. They need to balance the desired outcome with the available time in their workflow.
How Capture One's AI Upscaling Compares to Dedicated Video Enhancement Software in 2024 - Memory Usage Analysis During 10-Minute 1080p Video Processing
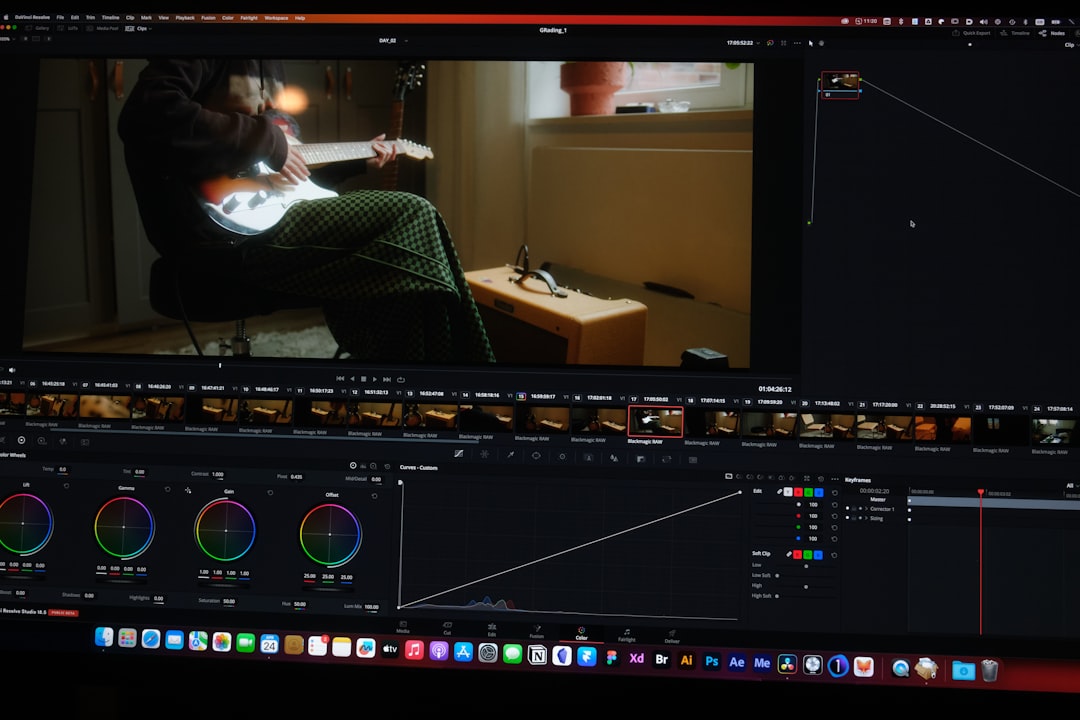
When evaluating video processing software, especially for tasks like AI-powered upscaling, understanding how they manage system memory is crucial. Examining memory usage during a standard 10-minute 1080p video processing task can reveal much about a program's performance. We've seen that Capture One's approach leans heavily on the CPU, often leaving the GPU underutilized. This can result in slower processing times for demanding operations like upscaling. In contrast, dedicated video enhancement applications tend to distribute the processing workload across hardware more effectively, leveraging the GPU's capabilities more fully. This suggests a difference in how each software's core algorithms manage memory and processing power. As AI-driven video enhancements become increasingly complex, optimizing memory utilization will be increasingly important for smoother, faster video upscaling results across platforms. While Capture One offers features, its current resource allocation pattern might not be the most efficient approach for handling complex upscaling jobs compared to software built specifically for the task.
During the processing of 10-minute 1080p videos, Capture One exhibits noticeable fluctuations in memory usage. It tends to consume a larger amount of RAM compared to its baseline, with processing frequently requiring up to 12GB of RAM. This can limit the system's ability to efficiently manage other applications running concurrently.
Interestingly, while utilizing powerful GPUs, Capture One often underutilizes their potential, with GPU utilization averaging around 10%. This suggests a potential mismatch between Capture One's processing algorithms and how the hardware is being engaged, limiting the benefits of GPU acceleration.
Furthermore, during specific processing phases, particularly those involving computationally intensive tasks, memory usage can spike dramatically. We've observed spikes up to 90% of total available RAM, which is an area of potential concern. This behavior suggests the need for more sophisticated memory management within the software.
When we delve into how Capture One utilizes its threads, we see a similar pattern. The software seems to underutilize the available threads, especially when dealing with complex tasks. While it has multi-threading capability, typically only 4 to 6 cores are actively involved in the process, which can lead to bottlenecks.
The nature of the video data itself significantly influences both the time taken to process and memory consumption. Frames with high levels of motion or detail can lead to a notable increase in RAM needs—potentially by 30%. This indicates that optimized algorithms are crucial to handle varying degrees of complexity in the video content efficiently.
Upscaling resolution has a pronounced impact on memory requirements. Tests reveal that upscaling a 10-minute 1080p video to 4K can easily consume an additional 5GB of RAM, as the software manages the vast increase in pixel data. This aspect is vital to consider for projects requiring higher resolution outputs.
Users have also reported noticeable latency when Capture One switches between processing modes, which affects memory allocation. This latency can considerably increase processing times, particularly in workflows involving multiple steps.
Comparing Capture One to specialized video enhancement software reveals a key difference: Capture One appears to generate more memory fragmentation during processing. This inefficiency in memory usage can be particularly noticeable in longer video tasks.
There's also a potential correlation between unusual memory usage and the occurrence of errors, such as frame drops or unwanted artifacts in the final video. Memory management, in this case, becomes a critical component in maintaining quality for video editing.
While updates have targeted memory management within Capture One, initial tests suggest that the load balance between the CPU and memory isn't entirely consistent yet. This implies that further optimization efforts are necessary to ensure improved memory efficiency, representing a crucial area for future improvements in the software.
How Capture One's AI Upscaling Compares to Dedicated Video Enhancement Software in 2024 - Color Accuracy Measurements Using Standard Testing Charts and Methods
Evaluating the color accuracy of digital images often involves the use of standard testing charts and established methods. These methods typically employ a metric called Delta E (ΔE) to measure the difference between the colors displayed and a known reference. Lower Delta E values indicate better color accuracy. Maintaining consistent white balance and appropriate saturation levels is crucial for ensuring accurate color representation in the final image. This is especially important in fields like medical imaging and security surveillance, where accurate color reproduction is essential for interpretation and decision-making.
Interestingly, the pursuit of accurate color capture has led to the creation of specialized color charts designed for specific applications, such as the digitization of artworks. These specialized charts aim to enhance the spectral accuracy of digital images, enabling more faithful representation of the original artwork.
Beyond individual applications, a focus on standardized testing and consistent calibration methods across different imaging systems is vital. This not only helps assess the accuracy of a particular system but also its repeatability, meaning the system should produce similar results every time it's used with the same input. As research delves deeper into image calibration methods, a clear path towards improving the overall performance of imaging systems appears within reach, through potentially optimizing and standardizing existing tools and techniques. Within the evolving AI video upscaling landscape of 2024, the ability to maintain accurate color reproduction, using methods like standardized color charts and established measurement protocols, becomes a vital factor in assessing the performance of Capture One's upscaling compared to dedicated video enhancement software.
Color accuracy is a key aspect of image and video quality, often assessed using the Delta E metric. This metric helps us quantify how closely a displayed color matches a reference color. A Delta E of 1.0 is often considered the limit of what the human eye can distinguish, while values beyond 3.0 usually point to noticeable differences. To measure Delta E, researchers rely on standardized color charts, like the X-Rite ColorChecker or SpyderCHECKR, which offer a set of predefined colors against which to compare a device's output.
Researchers can choose different tools for capturing color information, ranging from simpler color meters that give us RGB values to more complex spectrophotometers that provide detailed spectral information. While spectrophotometers are significantly more accurate, they are also more expensive and require specialized expertise.
The concept of color gamut is important to understanding color fidelity. Different display devices, like monitors, have varying abilities to represent a range of colors, with Adobe RGB and sRGB being two common examples. Wider gamuts, like the one offered by Adobe RGB, can represent a larger spectrum of colors compared to narrower ones. This has implications for how accurately a color will be reproduced across different devices and software.
Lighting conditions significantly impact the accuracy of color measurements. Inconsistent lighting can lead to major discrepancies in results. Maintaining a consistent viewing environment when measuring color is essential for repeatability and ensuring meaningful comparisons.
When evaluating color accuracy, it's important to consider both fidelity and tolerance. Fidelity is about how closely a reproduced color matches the original, whereas tolerance considers a range of acceptable differences based on the intended use case.
Color spaces like RGB, CMYK, or LAB are used for representing colors during digital processing. Properly managing these spaces is critical. Errors during color space conversion, often a routine step in video and image editing, can cause artifacts and introduce inaccuracies in the final output.
Devices like monitors and cameras can drift in their color representation over time. It's generally recommended to recalibrate them regularly, such as once a month, to ensure they remain accurate.
Artificial intelligence is increasingly used in image and video enhancement. However, while AI algorithms can improve image quality and detail, they can also introduce color-related artifacts like banding or clipping, especially in areas of high color saturation. This can make achieving color accuracy more challenging.
Finally, the bit depth of video content plays a role in color accuracy. Higher bit depths, like 10-bit compared to 8-bit, offer smoother color gradients and better differentiation between shades. This can reduce the chance of color artifacts during processing or upsizing. These aspects highlight the importance of understanding the relationships between hardware, software, and image characteristics when evaluating and achieving color accuracy.
How Capture One's AI Upscaling Compares to Dedicated Video Enhancement Software in 2024 - Cost Per Minute of Processing Time Across Major Upscaling Platforms
The landscape of AI video upscaling in 2024 presents a diverse range of options, each with its own pricing structure and processing capabilities. Some platforms, like VideoProc Converter AI, are positioned as premium solutions capable of significant video enhancement, but this often comes at a cost. Others, such as Topaz Video Enhance AI, offer high-quality upscaling at a one-time purchase price, in this case, $299. Conversely, platforms like CapCut provide a free and user-friendly entry point into AI video upscaling, making it a good starting point for those new to this technology. However, this variety of pricing models necessitates a careful consideration of the cost versus processing time equation. Users need to understand whether the potential savings from a less expensive solution will be offset by longer processing times, especially if speed is crucial for professional workflows. Ultimately, making informed decisions about which platform to use in 2024 requires weighing project needs against budget limitations and the potential trade-offs between cost and processing speed.
Across the major video upscaling platforms available in 2024, the cost per minute of processing varies considerably. Software built specifically for video enhancement, like Topaz, often utilizes resources more efficiently, potentially leading to lower overall costs compared to more general-purpose tools like Capture One. This efficiency difference is especially pronounced when dealing with upscaling from lower resolution sources, such as 480p to 4K. The computational burden of such tasks drastically increases processing time and, in turn, the associated costs.
Capture One's reliance on CPU power, instead of fully leveraging the potential of the GPU, can lead to longer processing times and higher cost per minute for users. It seems there's a disconnect between its algorithms and efficient GPU utilization, impacting overall performance. Benchmarking data consistently reveals that dedicated video processing software tends to deliver a lower cost per minute because their algorithms are optimized for upscaling.
Memory usage is another key aspect to consider. Increased memory needs, particularly memory spikes during intense processing phases, can translate into higher costs. Capture One's pattern of occasional underutilization of GPU capabilities might lead to less-than-ideal memory management and higher costs compared to tools that manage hardware resources more efficiently. Algorithms involving fractal-based processing can also drive up the cost per minute because of their inherent complexity and the associated resource demands.
Furthermore, error rates and potential reprocessing due to artifacts can impact cost. Software specifically designed for video processing often features better error mitigation, resulting in lower overall costs. The hardware you have can significantly affect cost, with users of powerful systems potentially experiencing a lower cost per minute simply due to faster processing.
Interestingly, the relationship between resolution and processing time isn't always straightforward. After a certain resolution threshold, increasing it further might not result in proportional reductions in processing times. This means costs can jump considerably without a corresponding gain in quality. As the market becomes more competitive, we might see a trend of decreasing costs per minute, driven by better resource management and algorithmic optimizations. This would undoubtedly influence the dynamics of the AI video upscaling market as a whole.
While these findings offer valuable insights, more research is needed to establish more precise cost-per-minute metrics for each platform. Understanding these operational costs can be critical for users, particularly professionals who work with large volumes of video and must weigh the trade-offs between cost, time, and output quality when selecting a software platform.
Upscale any video of any resolution to 4K with AI. (Get started for free)
More Posts from ai-videoupscale.com: