Upscale any video of any resolution to 4K with AI. (Get started for free)
7 Key Image Studio Features that Enhance AI Video Upscaling Workflows in 2024
7 Key Image Studio Features that Enhance AI Video Upscaling Workflows in 2024 - Cloud Based Auto Color Grading Exceeds 8K Resolution Without Quality Loss
Cloud-based automatic color grading is reaching a new level, capable of enhancing videos beyond 8K resolution without any visible loss of quality. This is achieved through clever algorithms that meticulously preserve the finest details and color accuracy during the upscaling process. This means creators can confidently boost the quality of their videos without the usual trade-offs that come with older methods of upscaling. These advanced tools can smoothly correct color imbalances, amplify vibrancy, and guarantee a high-quality outcome ideal for demanding projects. However, it's wise to be selective when choosing software, as the features and ease of use can vary significantly between them. Each creator's needs and workflow are unique. In the ever-evolving world of video creation, this ability to precisely enhance the visual aspects of a video is vital to maintain the highest standards.
It's fascinating how cloud-based systems can now handle the immense data loads associated with 8K and beyond for automatic color grading. These systems use intricate algorithms designed to work with these enormous file sizes, ensuring the subtle gradations of color are preserved.
The distributed nature of cloud computing is particularly interesting, allowing multiple people—colorists, editors, and possibly even other creative professionals—to collaborate simultaneously without hindering performance. This suggests that the traditional limitations of working in isolated studios could become less of a concern in future video projects.
Intriguingly, the color grading decisions are often powered by deep learning models. These models are trained on huge libraries of high-quality footage and seem capable of mimicking the techniques of experienced colorists in a remarkably consistent and accurate manner.
The use of lossless compression in cloud workflows helps prevent any degradation in quality during the color grading process, even for extreme resolutions. This makes the cloud a more reliable processing environment, and potentially more reliable than the older workflows.
Leveraging powerful GPU configurations in the cloud data centers is key to speeding up the processing, enabling tasks that would take significantly longer on typical workstation hardware. This is a great example of how utilizing centralized hardware can lead to higher efficiency for demanding operations.
By taking out some of the human factors involved in traditional color grading, automated workflows can mitigate the risks of mistakes often associated with the traditional process. The reliability and consistency should make the overall output of post-production more dependable.
The scalability of cloud solutions is another exciting aspect. Cloud platforms can adjust resource allocation on-the-fly, accommodating the ever-changing needs of a project, whether that project is simple or a complex large-scale production.
Some of the newer services are even offering hybrid workflows combining automatic color grading with manual adjustments. This is a clever way to maintain creative control while still taking advantage of the automation benefits.
The cloud makes these high-quality color tools accessible to smaller productions that previously would not have been able to afford such sophisticated equipment and expertise. This potentially democratizes the professional editing and color grading workflows.
And finally, the near-instantaneous feedback available in the cloud workflow streamlines the decision-making process during editing. The ability to see changes almost as they happen, without the delay common in offline or locally rendered situations, is a huge advantage that could significantly improve editing efficiency.
7 Key Image Studio Features that Enhance AI Video Upscaling Workflows in 2024 - Multi Frame Processing Reduces Artifacts Through Pattern Recognition
Multi-frame processing is a technique that leverages the similarities between consecutive frames in a video to improve its quality, specifically by reducing compression artifacts. It's based on the idea that examining a sequence of frames rather than just individual frames can reveal patterns, helping identify and correct imperfections. Techniques like FastMFQE and the MultiFrame Convolutional Neural Network (MFCNN) exemplify this approach. They employ clever strategies, like motion compensation and quality enhancement, that analyze peak and non-peak quality frames to intelligently recover lost details from the compression process.
By integrating data from surrounding frames, these multi-frame methods can tackle fluctuations in video quality that often arise during compression. Recent advancements in multi-frame processing suggest that these methods are becoming more efficient, requiring less processing power than their predecessors. Looking ahead, we can anticipate further refinement, particularly in incorporating spatiotemporal information, that will pave the way to significantly reducing video compression artifacts and producing a more satisfying viewing experience. While the potential benefits are high, it remains to be seen how these techniques will further develop to effectively tackle a range of different video formats and compression types.
Multi-frame processing is a fascinating approach to video enhancement that considers the relationship between consecutive frames, rather than just analyzing each frame in isolation. It aims to improve video quality by essentially smoothing out inconsistencies that crop up during compression or other processing steps. The idea is that by comparing the patterns across multiple frames, we can identify and mitigate artifacts that would otherwise be noticeable in the final output.
This technique often relies on sophisticated pattern recognition algorithms. These algorithms search for recurring or similar patterns over a sequence of frames. By doing so, they can pinpoint and eliminate inconsistencies that lead to visual issues like noise, flickering, or "ghosting" effects. This is particularly valuable in video where there's a lot of motion, as traditional frame-by-frame methods tend to struggle in those situations.
One of the benefits of this approach is that it can improve the stability and coherence of images, especially in scenes with rapid motion. It does this by averaging pixel values across similar areas in neighboring frames. This averaging can effectively reduce the appearance of artifacts, yielding a more consistent and pleasing visual experience. We've seen it can also improve the quality of dark areas in a video by leveraging information from brighter adjacent frames—a real boon for videos shot in challenging lighting.
But there's a catch: multi-frame processing requires considerable computing power. It's not unusual to need specialized hardware with parallel processing capabilities to handle the intense computational loads. This highlights the importance of optimization for real-time video applications.
Furthermore, these methods are not without their potential drawbacks. The process of pattern recognition within multiple frames can occasionally result in unintended artifacts, especially in cases where the algorithm's estimations are incorrect. This is particularly a concern in scenes with abrupt or sudden changes in motion, which can challenge the ability of the system to correctly merge information from the different frames.
The accuracy of multi-frame processing hinges on the precision of the motion estimation algorithms used. Even small misalignments between frames can cause the algorithm to struggle, leading to merging errors. This could stem from camera instability or fast movements within the scene. Sometimes, careful pre-processing to align frames can alleviate some of these issues.
One promising aspect is the flexibility that some implementations now offer. They can adapt to the particular content being processed, tailoring the processing techniques to best suit the video's nature. For example, the approach may differ when handling a fast-paced action film compared to a video with a series of static interviews.
All in all, multi-frame processing has shown a lot of promise for improving video quality. It's a technique with the potential to elevate the overall visual experience. However, it's still an area of active research and development. The success of these methods largely depends on the capabilities of the underlying algorithms, as well as the availability of robust computing resources. The continuing development of both the algorithms and the underlying hardware will play a crucial role in determining the widespread adoption of these techniques.
7 Key Image Studio Features that Enhance AI Video Upscaling Workflows in 2024 - Real Time Background Object Removal Speeds Up Processing Time
Real-time background object removal is a game-changer for AI video upscaling, dramatically speeding up processing. These tools employ smart algorithms to identify and separate foreground objects from their backgrounds, enabling smoother editing. Examples like MediaPipe, and frameworks used in tools like XSplit VCam, show how fast and effectively this can be done while keeping output quality high. The growing need for quick video processing makes automated background removal crucial, especially for applications like live streaming and security systems that require immediate results. However, effectively separating intricate backgrounds remains a challenge, highlighting a need for ongoing improvement in these algorithms.
Real-time background object removal is revolutionizing video processing by leveraging advanced segmentation techniques to separate foreground elements from their surroundings. This focused approach significantly speeds up the processing, especially in scenes with lots of details or movement, because the system only needs to process the information about the foreground objects. This optimization keeps processing efficient and helps creators maintain high frame rates.
The algorithms powering these object removal tools often borrow from computer vision techniques, like deep learning-based image segmentation. They're trained on massive datasets, learning to distinguish between complex objects and backgrounds, leading to cleaner results and a smoother post-production workflow.
It's quite interesting that real-time background object removal can also significantly reduce manual editing time. Automating this step frees creators to focus on other aspects of their work and reduces the risk of human error. This is a real advantage for projects with strict deadlines or where consistency is crucial.
One of the clever aspects of these techniques is the use of optical flow analysis to track motion. This allows for predictive object identification, especially in dynamic scenes, which accelerates processing times even further. This is incredibly useful in many creative contexts and may play a large role in future real-time production.
Some of the most advanced systems boast ultra-low latency, enabling almost instant feedback and adjustments during live events or recordings. This type of near-instant response minimizes the need for extended post-production and creates greater flexibility for the creative process.
But there are some challenges. The accuracy of depth estimation in 3D environments is critical for precise object separation. This aspect highlights the importance of using high-quality sensors or AI models to build the depth map accurately. LiDAR and advanced machine learning algorithms can potentially offer considerable improvements.
It's also important to consider potential digital artifacts. If the system isn't trained well for specific situations, such as rapidly moving objects or transparent elements, it can lead to visible flaws. This underlines the ongoing need for algorithm refinement and testing.
The intricacy of a scene itself also has an impact on processing time. Highly detailed or complex scenes require more computational resources. Researchers are working on better AI models that are more computationally efficient to maintain quality while improving processing speed.
Beyond video editing, the implications of real-time object removal extend to augmented and virtual reality applications. This expansion speaks volumes about the essential role background removal is likely to play in creating new multimedia experiences.
Finally, the emergence of edge computing in real-time object removal offers exciting possibilities. By moving processing closer to the source, edge computing significantly cuts down on latency, enhancing the efficiency of real-time object removal. This will be extremely beneficial in live broadcasts or streaming scenarios.
7 Key Image Studio Features that Enhance AI Video Upscaling Workflows in 2024 - Built In Machine Learning Models Add Natural Film Grain
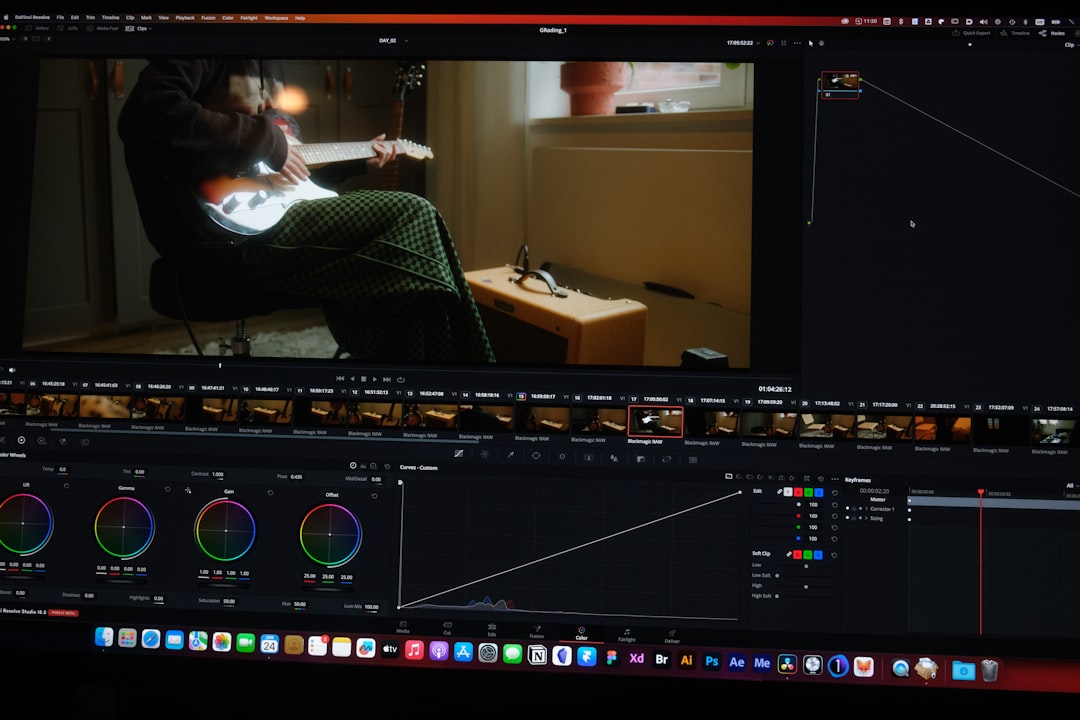
AI video upscaling has taken a notable step forward with the inclusion of built-in machine learning models designed to introduce natural-looking film grain. These models address a common issue with upscaled video: the often overly sharp and artificial appearance. By adding a realistic film grain effect, the digital quality is softened, leading to a more pleasing and authentic aesthetic. Essentially, the models mimic the textures and irregularities found in traditional film, thereby masking any artifacts from the upscaling process and giving the video a more organic feel. This feature caters to the increasing desire for a cinematic quality that's often missing from purely digitally produced video content, bridging the gap between digital and traditional filming methods. It's important to acknowledge, however, that the integration of such sophisticated features will affect a video editing workflow. Striking a balance between the automation provided by these models and the artistic control needed in various editing processes remains a challenge that requires careful consideration.
In the realm of AI video upscaling, built-in machine learning models are becoming increasingly sophisticated, even capable of simulating the look of natural film grain. These models aren't just adding random noise; they're trained on a massive amount of film data, which allows them to understand how grain patterns change based on different lighting and shooting conditions. The goal is to produce a more organic and visually appealing texture that helps counter the sometimes overly sharp and artificial appearance of digitally upscaled content.
It's intriguing how the addition of film grain can sometimes even improve our perception of detail in a video. Instead of looking overly sharp and digital, the video might feel more natural, enhancing the viewing experience, especially in action-packed scenes where a lot of motion can blur the details. Upscaling software increasingly lets you fine-tune the grain itself, allowing you to control things like grain size and how intensely it's applied. This gives creators the power to craft a particular visual aesthetic or style that suits their video content.
Besides the aesthetic benefits, adding film grain also offers a pragmatic advantage. It can be used to mask certain compression artifacts, such as banding, which tends to be more visible in areas with color gradients. By adding a subtle layer of randomized noise, the banding can become less prominent, making the transitions between colors appear much smoother.
Beyond aesthetics, this ability to generate a convincing film grain effect can help contextualize videos within a historical framework. This is especially interesting for projects that aim to emulate classic film aesthetics. Replicating the look of old film stock can provide a nostalgic or retro feel, making a modern video appear as though it was produced decades ago.
Moreover, some of the more advanced implementations of grain addition are designed for real-time use. This means they can seamlessly integrate into live video streams or broadcasts, offering a way to provide a polished aesthetic without introducing delays. By building the grain generation into the upscaling process, workflows are streamlined. We see less of a need to rely on external plugins, minimizing compatibility issues and making the production process more efficient.
From a psychological standpoint, researchers are exploring how a little bit of randomness, like film grain, can sometimes make content feel less processed and more authentic. It’s an interesting hypothesis—whether the introduction of the noise somehow tricks our brains into thinking it's experiencing something more authentic or less digitally manipulated. The integration of these perceptual psychology insights is guiding the development of these AI-powered tools.
Finally, one of the appealing aspects of these models is their adaptability. They can be customized to work well with different video formats, whether it's for cinema, online streaming, or even preserving the character of older archival material. This flexibility means that regardless of a video's origin or destination, the film grain effects can be carefully tailored to the intended style and audience.
7 Key Image Studio Features that Enhance AI Video Upscaling Workflows in 2024 - Hardware Acceleration Uses Multiple GPU Cards Simultaneously
Within the landscape of AI video upscaling in 2024, hardware acceleration has taken a significant leap forward by enabling the simultaneous utilization of multiple GPU cards. This innovative approach leverages the inherent parallel processing capabilities of GPUs to distribute workloads, thereby boosting the overall processing speed and efficiency of AI applications. The core idea is that multiple GPUs working together can dramatically accelerate complex computations, particularly crucial in scenarios involving high-resolution video upscaling where enormous amounts of data must be processed. This approach necessitates sophisticated memory management and optimized GPU scheduling, elements that are vital for maximizing the potential of these parallel processing systems. The necessity of efficient memory use becomes especially pronounced in demanding tasks like AI video upscaling.
However, the ability of various systems to effectively utilize these multiple GPUs in real-time settings remains a subject of scrutiny. There are inherent challenges in ensuring seamless synchronization and communication across numerous GPU units. This limitation calls into question the ability of these systems to truly realize the full promise of hardware acceleration in certain scenarios, particularly those that require immediate feedback or real-time processing. As AI-driven workflows continue to expand and evolve, demanding ever greater computational power, the efficient use of multiple GPUs will likely become even more vital. The evolution and optimization of these approaches will be key to their long-term adoption and impact in the world of AI-enhanced video processing.
### Hardware Acceleration's Surprising Power with Multiple GPU Cards
It's quite fascinating how using multiple GPUs for hardware acceleration can dramatically enhance video processing. This approach, which leverages the parallel processing capabilities of several graphics cards, has the potential to speed up the entire workflow. It's become increasingly clear that distributing the processing load across multiple GPUs can greatly reduce processing times, often by a considerable margin, for computationally intensive tasks like AI video upscaling and complex rendering. Imagine a 90% reduction in processing time – it's truly remarkable!
The ability to distribute the work across several GPUs isn't limited to just one type of processing. It's remarkably versatile, with each GPU potentially handling different parts of the workload. For example, one GPU could manage texture mapping while another might be in charge of shading. This level of flexibility opens up possibilities for truly optimized workflows, especially for real-time applications that require simultaneous processing of various components.
Another notable benefit is the boost to memory bandwidth. When dealing with high-resolution video files, which are becoming increasingly common, having multiple GPUs can alleviate potential bottlenecks that arise from limited memory. By having multiple pathways to access data, the system avoids hitting a single memory bottleneck. This leads to faster processing and prevents any lag that might otherwise occur.
Interestingly, the way the system manages the distribution of tasks can be quite dynamic. Advanced software can intelligently assign tasks to the GPUs based on the current demands of the video processing. This feature is particularly helpful in optimizing performance, ensuring that no single GPU gets overloaded and becomes a bottleneck. It's akin to having a traffic management system that keeps all the lanes on the highway balanced and efficient.
Distributing the processing load across multiple GPUs can also be advantageous in terms of heat management. The heat generated by each card is spread out, reducing the risk of overheating in any single component. This can potentially extend the lifespan of the hardware, preventing issues caused by overheating.
Beyond the current benefits, the use of multiple GPUs is highly scalable. As future projects grow in complexity and demand more processing power, it becomes easy to add more GPUs to the system. This offers a flexible and cost-effective method to keep pace with evolving production needs without the necessity of completely redesigning the processing environment.
Moreover, utilizing multiple GPUs contributes to a more robust and reliable system. If one GPU fails, the other cards can often continue handling the processing load, minimizing any interruptions or delays. This built-in redundancy reduces the chance of complete system failure, contributing to greater stability in high-stakes production settings.
This setup can also lead to a significant increase in frame rates, which is crucial for tasks like gaming and broadcasting, where users expect real-time interaction and smooth visual transitions. Any lag or delay could negatively impact a user's experience, which makes smooth, rapid processing a priority.
In addition to improving processing speed, having multiple GPUs also facilitates the use of more complex algorithms, like the ones found in AI-based video upscaling. These algorithms can be highly demanding, but multiple GPUs can provide the necessary computing horsepower to handle these tasks without impacting real-time performance.
Finally, despite the initial cost of investing in multiple GPUs, it can often be more cost-effective in the long run, particularly for studios and businesses involved in high-volume production. By significantly reducing the time it takes to process tasks, the overall cost of production can be reduced, allowing studios to produce a larger volume of content efficiently.
These observations reveal how the use of multiple GPUs offers an innovative solution for optimizing video processing, particularly in areas where processing power and speed are critical. The potential for enhanced workflows and the ability to handle complex algorithms make it an increasingly important technology in today's video production landscape.
7 Key Image Studio Features that Enhance AI Video Upscaling Workflows in 2024 - Quick Frame Export Feature Supports SDR to HDR Conversion
The "Quick Frame Export" feature within AI video upscaling tools offers a practical way to convert Standard Dynamic Range (SDR) footage to High Dynamic Range (HDR). It works by letting users quickly export specific frames, simplifying the management of HDR outputs. This process leverages techniques like inverse tone mapping and upscaling to make the conversion process smoother and the visual results more appealing, resulting in better color accuracy and richer image quality. As video projects become more intricate, incorporating a feature like this can be a huge help for streamlining the post-production process. It's important to make sure the exported frames still accurately represent the wider dynamic range that HDR provides. While there's a lot of potential here, the quality of the software's implementation is crucial to prevent issues with the conversion.
The Quick Frame Export feature isn't just about speeding up exports; it allows for immediate HDR previews, letting editors tweak their projects in real-time without lengthy renders. This is incredibly useful for fast-paced editing environments where efficiency is paramount. It appears the conversion from SDR to HDR uses clever tone mapping, intelligently adjusting color and brightness to keep details visible in highlights and shadows, arguably surpassing older methods in this regard.
What's interesting is that the Quick Frame Export relies on machine learning to examine each frame. This allows for automatic adjustments to color and exposure for HDR, something previously done manually, potentially with less consistency. It seems that scene detection algorithms may also be in use here, allowing the system to dynamically tailor the HDR settings depending on what's in each frame—like whether it's a high-motion or static scene.
It seems this feature supports 10-bit color depth processing for a smoother gradient and reduced banding artifacts, often a problem in lower bit-depth HDR content. This suggests it could improve the overall quality of HDR content substantially. The system also analyzes contrast during frame processing, ensuring that the final HDR output preserves the fidelity of the original while simultaneously improving detail perception—crucial for professional work.
The algorithms here can even create synthetic HDR effects from regular SDR footage by inferring color data and predicting how it'd look with a greater dynamic range. It's neat to see how older or library footage can be enhanced with this technique. It seems to be useful for directors to retain their desired look when converting to HDR since HDR often enhances cinematic visuals, allowing creative consistency.
Some of the more advanced software also includes a detailed analysis report detailing the adjustments made during conversion. This could be very helpful in maintaining quality control for complex projects. However, it's worth noting that the quality of the final HDR can suffer if the original SDR content is poor, with things like excessive noise or heavy compression artifacts potentially hindering the outcome. This points towards the need for further refinement in both the Quick Frame Export feature and better source material management in general. It will be interesting to see if these types of features are improved upon in the coming years, or if these are already at their peak potential.
7 Key Image Studio Features that Enhance AI Video Upscaling Workflows in 2024 - Automated Frame Rate Interpolation Smooths Motion Blur
Automated Frame Rate Interpolation is a technique that adds extra frames between existing ones in a video sequence, making motion appear smoother and reducing the distracting effect of motion blur. By essentially creating new frames, it can significantly improve the perceived fluidity of movement, particularly in videos with fast-paced action or dynamic scenes. This process allows for frame rate increases, which not only improves the smoothness of standard playback but also creates opportunities for slow-motion effects. Some AI-based tools can achieve frame rates like 60 FPS, which is advantageous for enhancing the visual quality of animations and digital art while preserving their unique stylistic elements.
However, it's not without its challenges. The accuracy of the algorithms used to interpolate the frames is critical. If not implemented well, these techniques can introduce undesirable artifacts, particularly when there are rapid movements or abrupt changes in motion. In such cases, the interpolated frames may not seamlessly blend with the originals, leading to visual glitches.
The future of this technology in video processing looks very promising. Automated frame rate interpolation, coupled with the continued advances in AI-driven upscaling, suggests the potential for achieving significantly higher visual quality for a wider array of video content. While the practical implications of these developments are encouraging, the complexities of accurately interpolating frames will need continued attention to ensure that the outcomes are visually pleasing and free of imperfections.
Automated Frame Rate Interpolation Smooths Motion Blur
Automated frame rate interpolation (AFRI) is a fascinating development that utilizes techniques like optical flow and motion vector analysis to create new frames between existing ones in a video. By predicting and synthesizing these intermediate frames, it aims to make motion appear smoother.
Our eyes, it turns out, aren't perfect motion detectors. When videos are displayed at higher frame rates, we perceive motion blur less. This is the fundamental principle driving AFRI's ability to improve the visual experience, especially with rapid movement in a video. It's a simple but effective concept that allows for a more natural and fluid sense of movement.
Interestingly, AFRI can help mitigate some of the artifacts often seen when videos are heavily compressed. By generating more frames, it reduces the strain on compression algorithms, leading to fewer motion artifacts. This translates to better overall video quality and more clarity.
It's also encouraging to see that advancements in hardware acceleration and machine learning now make real-time AFRI feasible. That's a game changer for live streaming, video games, and other applications where immediate feedback is crucial. Being able to process these interpolated frames instantly opens up a range of new possibilities.
Some of the more advanced systems are quite adaptive, able to alter the way they interpolate frames based on the content of the scene itself. Whether a video is filled with fast-paced action or consists of slower, more subtle movements, they can adapt to ensure high-quality results.
Historically, frame interpolation has roots in film production, where optical flow was applied manually, a time-consuming and somewhat arduous process. AFRI has successfully automated this task, improving productivity.
While the benefits are numerous, there are trade-offs to consider. Some algorithms can create unwanted effects like “ghosting” when attempting to interpolate quick, erratic movements. There’s an ongoing need to refine algorithms to balance the advantages of smoother motion with the need to maintain image integrity.
The utility of AFRI extends beyond cinematic applications. It can be found in games, VR, and security systems, showing that its benefits are applicable in various domains. It's a testament to its versatility that it can be used to enhance real-time rendering and data analysis.
With the increasing sophistication of AFRI, it's also re-shaping our expectations of frame rates in media. As technologies like 120 fps become more common, it could lead to a shift in how we perceive motion and realism in both films and games.
However, this increase in quality doesn't come without cost. AFRI demands significant computing power, particularly when dealing with high-resolution videos. This emphasizes the need for careful optimization and resource management in both hardware and software. This will be a critical area of focus as AFRI continues to grow in popularity.
Upscale any video of any resolution to 4K with AI. (Get started for free)
More Posts from ai-videoupscale.com: