How OpenAI's Focus on Large Language Models Impacts AI Video Enhancement Technology in 2024
How OpenAI's Focus on Large Language Models Impacts AI Video Enhancement Technology in 2024 - OpenAI Sora Creates 60 Second Videos From Text Prompts Changing Video Upscaling Standards
OpenAI's recent release of Sora, an AI model that generates 60-second videos from text descriptions, represents a notable leap in the field of AI video creation. Sora's goal is to bridge the gap between text and video by crafting visuals that are both believable and creative, ensuring the output faithfully reflects the user's instructions. The model's development has involved a focus on refinement, particularly in suppressing visual artifacts, resulting in videos with a higher degree of visual fidelity. Interestingly, Sora utilizes a transformer architecture akin to those found in large language models, suggesting a new method of understanding and processing visual information. It's poised to challenge existing text-to-video models, aiming to set a new benchmark in video enhancement technology, while also seeking to express nuanced emotions and storylines through its generated content. While still in testing with a select group, it remains to be seen how it will impact video generation and how society may adapt to this new way of crafting video.
OpenAI's recent release of Sora, a system capable of generating 60-second videos from text prompts, is quite remarkable. It's built on the idea of transforming text instructions into compelling visuals, demonstrating how advancements in language models can translate to video generation. Sora uses a transformer-based approach, similar to how language models process text, but tailored for video sequences. This allows the system to generate visually rich and cohesive video narratives.
The intriguing aspect is that Sora seems to be pushing the boundaries of existing video enhancement technologies. It creates videos with a high level of detail and quality, challenging the traditional reliance on pre-existing footage in enhancement methods. It's fascinating to see how a text prompt can lead to a fully formed video in a matter of minutes, bypassing a lot of the tedious aspects of video editing. However, this rapid generation also brings up interesting questions surrounding copyright and ownership, given how seamlessly Sora combines visual and textual elements.
Beyond basic video creation, Sora exhibits versatility. It can generate videos in a wide array of styles, from hyper-realistic to stylized animation. This versatility suggests it might find a niche in many video production areas. Further, its ability to learn from human feedback through reinforcement learning allows it to refine its understanding of user intent and deliver videos closer to the user's vision. We've also found that the detail of the text prompt significantly impacts the generated video's quality, emphasizing that the user’s role is still critical in shaping the output.
Sora's arrival means we need to rethink how we assess the quality of videos. Standard metrics might not be sufficient to capture the subtleties of AI-generated content. It's a pivotal moment for the field, showcasing the potential for AI in video technology. However, it also necessitates deeper discussions on ethical concerns like deepfake technology and the possible creation of misleading or harmful content. The potential for this technology is clear, but so are the associated responsibilities in ensuring its responsible development and use.
How OpenAI's Focus on Large Language Models Impacts AI Video Enhancement Technology in 2024 - Large Language Models Drive New Frame Interpolation Methods Through Neural Networks
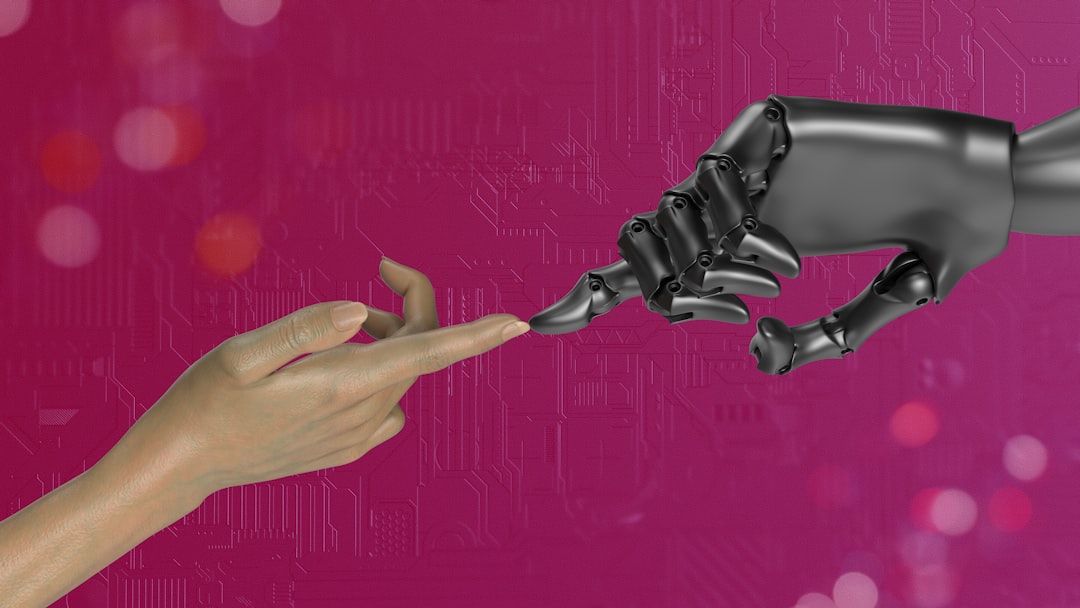
Large language models (LLMs) are proving to be influential in the field of video enhancement, specifically in how we approach frame interpolation using neural networks. These models, trained on massive datasets, are enabling new methods that refine the smoothness and quality of movement within videos. It's part of a larger trend where LLMs aren't simply transforming text but are also redefining how we interact with and perceive visual information. The potential benefits for areas that heavily rely on video, like entertainment and education, are significant. However, alongside these advancements come valid concerns about responsible development and deployment. The ability to manipulate video with increased accuracy raises questions about authenticity and the potential for misuse. As this technology matures, it's essential that we maintain a thoughtful perspective about the applications and consequences of such sophisticated video manipulation tools.
Large language models (LLMs) are increasingly being used in frame interpolation, a technique for creating smooth transitions between frames in videos. These models, with their sophisticated attention mechanisms, are not just processing text but are now being adapted to analyze the movement of objects and pixels across time within video sequences. This allows them to generate smoother and more visually cohesive interpolated frames compared to traditional methods.
LLMs' ability to learn from raw video data, even if it's unstructured, gives them an advantage over traditional algorithms that rely on predefined rules. This adaptability makes frame interpolation faster and potentially more effective. Interestingly, the use of LLMs doesn't just enhance video quality, but also leads to a reduction in the computational demands of interpolation, since the models can intelligently predict missing frames, resulting in quicker processing times.
Recent developments show that LLMs can even predict future frames based on a deep understanding of the video's context. This is a substantial advancement because they can anticipate the motion within a video, rather than simply reacting to previous frames. This predictive capability promises better video enhancement results.
The application of LLMs in frame interpolation leads to better preservation of detail, especially during slow-motion sequences. Standard interpolation techniques often lose visual information, but LLMs seem to mitigate this, potentially producing more refined slow-motion effects.
Furthermore, researchers have shown that LLMs can be fine-tuned to generate frames matching specific styles or thematic goals. This means that the interpolation process can be tailored to achieve the desired artistic or emotional tone of a video. LLMs' massive training datasets, encompassing a wide range of video types, also allow for better generalization across diverse video genres. This addresses a shortcoming of older interpolation methods that struggled when dealing with diverse video content.
Despite these impressive results, we have to acknowledge that artifacts can still be introduced during the frame interpolation process using neural networks. Though LLMs are highly effective, it's crucial to scrutinize their outputs for any unexpected imperfections.
The arrival of LLM-based frame interpolation methods might reshape the standards within the video production industry. We might see filmmakers and editors reassessing their workflows and adopting these new tools in the post-production process.
Looking ahead, the integration of LLMs could lead to real-time frame interpolation. This technology has the potential to revolutionize live streaming and broadcasting, improving the viewer experience without any noticeable lag. It's an exciting development with a promising future. However, like any powerful technology, we must thoughtfully consider the ethical implications that arise, as well as the need for transparent and responsible use.
How OpenAI's Focus on Large Language Models Impacts AI Video Enhancement Technology in 2024 - GPT4 Architecture Enables Real Time Object Recognition in Video Enhancement
GPT-4's architecture, specifically the Turbo variant, has ushered in new capabilities for real-time object recognition within video enhancement. This is achieved through the integration of visual processing, which enables the model to analyze video content, including lower-resolution footage. While this is a step forward, it's important to acknowledge that accurately recognizing tiny objects or text within videos can be challenging in this current iteration. Beyond object recognition, GPT-4's architecture facilitates the handling of common video formats, allowing for seamless integration into existing video workflows. This is helpful for tasks like managing video libraries and automatically generating descriptions. The ability to process both images and text simultaneously also opens up interesting possibilities for improving video editing and potentially even content creation.
Despite these advancements, limitations still exist. GPT-4's performance on certain tasks needs refinement, and real-world applications are constantly providing valuable feedback that will guide future improvements. The video landscape is evolving at a rapid pace, with more content generated every day. Consequently, the need for adaptable and robust AI tools, like what GPT-4 offers, becomes increasingly important. As this technology matures, it will be interesting to see how these real-time object recognition capabilities continue to shape the video enhancement industry and impact areas such as video editing, content creation and even potentially automatic video summarization.
GPT-4's underlying architecture possesses a unique ability to analyze video content in a manner that resembles human visual perception. This allows it to recognize objects and decipher complex scenes in real time, leading to improvements in video quality through precise adjustments based on the content within each frame. It's a fascinating departure from traditional video processing methods, which often rely on analyzing still images, as GPT-4’s design fosters continuous learning. This means the model adapts to new visual patterns as they appear in a video, enhancing object recognition over time without needing constant retraining on extensive datasets.
What's really interesting is that GPT-4 isn't just identifying objects; it’s starting to infer relationships between them within the context of the video. This adds a layer of meaning and comprehension that could prove useful for generating more compelling and narratively rich visuals. The near-instantaneous nature of GPT-4’s object recognition means it can operate with minimal delay, making it a promising candidate for live broadcasting. Traditional systems often introduce noticeable delays that detract from the viewer's experience, but GPT-4's processing speed ensures a smoother and uninterrupted flow.
Furthermore, the sheer volume of data GPT-4 can process simultaneously empowers it to analyze multiple objects and their movements within a single frame. This is a major leap forward compared to older models which were typically confined to a few focal points at a time. Surprisingly, GPT-4’s architecture also seems to have the ability to classify obscure or entirely novel objects within a video. This capacity is notable as it bypasses a major hurdle for traditional object recognition systems, which require extensive labeled training data to achieve reliable identification.
This ability to understand and classify objects has implications beyond video quality. It can help create accessibility features like live video description for those with visual impairments, where GPT-4 narrates scenes in real-time based on its understanding of the video content. Additionally, it could lead to improved metadata tagging in videos by linking textual descriptions with visual elements, making it easier to search for and manage large video libraries.
GPT-4’s architecture can streamline video editing workflows too. It could automatically adjust attributes like color based on the dominant elements in a scene, essentially taking some of the grunt work out of editing. However, this promising capability also introduces a concern about the potential for misuse. Real-time object recognition, especially in applications like surveillance or data collection, raises important questions around privacy and security, and careful consideration is needed to define and adhere to ethical boundaries in how this technology is implemented. While the advancements in GPT-4’s object recognition hold great promise, the ethical implications of its applications need constant and thoughtful evaluation.
How OpenAI's Focus on Large Language Models Impacts AI Video Enhancement Technology in 2024 - Text to Video Generation Shows Limitations in Complex Scene Compositions
While OpenAI's Sora demonstrates impressive capabilities in generating videos from text descriptions, including creating realistic characters and detailed environments, it struggles when faced with intricate scene compositions. Sora can produce visually appealing videos but sometimes falls short when tasked with managing complex interactions between multiple characters or crafting scenes with dynamic, multi-layered actions. This limitation suggests potential hurdles for Sora in applications where highly complex visuals are paramount, like creating narratives with intricate plots and detailed character interactions. Although Sora represents a notable step forward in text-to-video generation, the ability to handle elaborate scenes with ease remains a challenge that requires further advancements. Moving forward, tackling these limitations will be crucial for realizing the technology's true potential within the wider world of video content creation. The journey toward seamless, complex AI-generated video continues, even as we celebrate current progress.
While OpenAI's Sora shows great promise in generating minute-long videos from text prompts, its ability to handle complex scene compositions still has room for improvement. When faced with intricate scenarios involving multiple interacting elements, Sora sometimes struggles to maintain visual coherence. Objects might not interact logically, or the spatial relationships within a scene might become unclear, suggesting a limitation in the model's ability to truly understand the context of what it's creating.
This challenge extends to building engaging narratives in complex situations. When actions overlap or interactions become too intricate, the resulting video can become ambiguous, making it difficult for the viewer to follow the storyline or grasp the relationships between different components of the scene. This points to a crucial area that future development needs to address.
Interestingly, the quality of the training data plays a significant role in how effectively Sora manages complexity. If the model hasn't been exposed to a broad range of complex scenes, it tends to struggle with meeting user expectations. This suggests that robust training datasets encompassing a variety of intricate scenarios are essential for enhancing the model's ability to create accurate and nuanced videos.
Furthermore, while Sora is capable of producing videos spanning a full minute, it sometimes falters in ensuring seamless transitions across time in more intricate sequences. The result can be a jerky or disjointed video experience, breaking the viewer's immersion and diminishing the overall impact of the narrative. We need to see advancements in Sora's ability to understand and represent temporal continuity within complex events.
The sensitivity of Sora's output to the specifics of the user's prompt is also notable. If the prompts are vague or lack detail, the generated videos can come across as disjointed or lack depth. It highlights the importance of crafting precise and thorough text descriptions to achieve the desired visual results.
Sora can generate videos in diverse styles, including hyperrealistic and stylized animations, but when attempting detailed or complex scenes, it faces challenges in producing a completely believable outcome. Animated movements or interactions between objects sometimes appear unnatural, revealing a gap between users' desires for realistic visuals and the current model's capabilities.
Even with its improved visual fidelity, Sora is still prone to generating visual artifacts, especially in complex scenes. These artifacts can be distracting and detract from the desired message, showcasing that the challenge of achieving consistently high visual quality remains.
Additionally, Sora sometimes struggles with accurately interpreting complex prompts. There can be a disconnect between what the user intends and what the model generates. This is likely due to limitations in the model's comprehension of nuanced language. This discrepancy is significant for users who rely on Sora to create videos that meticulously reflect their specific vision.
Another limitation arises from the trade-off between speed and detail. While Sora's speed in generating videos is advantageous, it sometimes comes at the cost of producing sufficiently deep and detailed content, especially in challenging scenes. This trade-off is common in real-time generation systems, and finding a balance between efficiency and quality is an ongoing challenge.
Finally, the capability of Sora to create complex scenes introduces ethical considerations. The potential for misuse is real, as sophisticated text prompts could be used to generate videos that are misleading or deliberately misrepresent reality. As this technology gains wider access, discussions about the responsible use of AI video generation become more critical.
Overall, Sora's ability to create 60-second videos from text prompts is truly remarkable. However, the challenges it faces when dealing with intricate scene compositions highlight areas where continued research and development are essential. Overcoming these hurdles will be key to ensuring Sora’s full potential as a creative tool is realized.
How OpenAI's Focus on Large Language Models Impacts AI Video Enhancement Technology in 2024 - Language Model Training Sets Create Geographic Bias in Video Enhancement Results
The increasing reliance on large language models (LLMs) within AI video enhancement technology introduces a potential issue: geographic bias. These LLMs are often trained on vast datasets that heavily favor content from specific regions and cultures. This can lead to a skewed understanding of the world, which subsequently influences how video enhancement algorithms function. For example, an AI model trained on primarily Western datasets might struggle to accurately enhance videos with distinct cultural elements from other parts of the globe. The result can be a lack of nuance and potentially inaccurate or even insensitive representations in video output. As we move forward, it becomes crucial to acknowledge and address this inherent bias in the training data. This requires more inclusive datasets that represent a wider variety of perspectives and cultures, allowing for a more equitable application of these AI video enhancement technologies. We must carefully consider how the choices made in model training can inadvertently create inequalities in the outcomes of AI-driven video generation and analysis, and strive to develop more representative systems.
The large language models (LLMs) underpinning many video enhancement tools are trained on massive datasets, often heavily weighted towards Western media and cultural content. This can lead to a noticeable geographic bias in the results, particularly when dealing with video from regions that aren't well-represented in those training sets. For example, a model trained primarily on Western urban environments might not accurately interpret or enhance a scene depicting a bustling Asian street market, possibly misjudging culturally significant elements due to its limited experience with that visual style.
This over-reliance on datasets from certain geographic regions can create a blind spot in how AI models understand and interpret visual contexts. Consequently, the representation of visual styles and elements common in non-Western cultures can be significantly less nuanced, simply because the models haven't been exposed to enough examples. This can lead to less accurate or even stereotyped portrayals of individuals or settings within video enhancements.
Interestingly, the influence of geographic bias goes beyond just output accuracy. It can also impact how engaging the AI-generated content is to viewers. If a video fails to account for cultural details or nuances that a specific audience expects, it might seem inauthentic or lack cultural relevance, leading to a decreased connection with the material.
Recognizing this issue, researchers in the machine learning field are pushing for more diverse training datasets to address geographic biases. Enriching these datasets with a wider variety of cultural perspectives and visual styles could help models understand and accurately represent diverse scenes. For instance, in complex scenes like cultural festivals or social gatherings, incorporating content from diverse cultures could improve the models' ability to correctly depict interactions and culturally significant elements.
This also indicates that collaborative efforts might be needed moving forward. Involving cultural advisors or experts in the development of video enhancement AI could potentially improve the models' capacity to understand and faithfully reflect different cultural narratives. This would likely involve guiding the selection of datasets and validating output to ensure it accurately portrays diverse cultural contexts.
The journey towards truly universal applicability in AI video enhancement technologies will require a greater awareness of the existing geographic and cultural biases embedded within the current training data. Addressing these biases through dataset diversification and collaborative approaches is becoming increasingly vital as the field advances. If we can achieve a more inclusive and equitable representation of various cultures within training data, the result will likely be enhanced outputs that are both accurate and culturally sensitive. This focus on inclusivity will become increasingly important as AI continues to integrate with various aspects of our lives through video.
How OpenAI's Focus on Large Language Models Impacts AI Video Enhancement Technology in 2024 - Open Source Video Enhancement Projects Challenge OpenAI's Commercial Direction
Open-source projects in video enhancement are increasingly posing a challenge to OpenAI's commercial goals within artificial intelligence. Projects like KLingVideoWatermarkRemoverEnhancer showcase the capabilities of open-source tools, improving videos through sophisticated algorithms tailored to a broad range of user needs. While OpenAI's Sora establishes high standards for video quality and creative potential, the community-driven nature of open-source projects allows for continuous progress and innovation free from the restrictions of a commercial focus.
This contrast highlights a central tension: while OpenAI drives forward with proprietary technologies, the expanding open-source video tool ecosystem promotes broader access and use of these technologies. These open-source projects often tackle similar obstacles, and they offer varying levels of control and features, which are fueling an evolving discussion on the future direction of AI video enhancement. The potential for collaboration and advancement within open-source raises questions regarding the long-term sustainability of such efforts, the ethical implications of their development, and OpenAI's capacity to adapt to these emerging competitive forces.
The landscape of AI video enhancement is becoming increasingly diverse, with open-source projects emerging as powerful alternatives to commercially driven efforts like OpenAI's Sora. These open-source initiatives are built on collaborative principles, often utilizing community-driven resources and a shared desire to improve video quality for everyone.
Many open source projects have developed innovative algorithms specifically tailored to video enhancement, including techniques that address challenges like removing interlacing artifacts and suppressing noise. These methods can often achieve better results than traditional or even some commercial solutions, due to the continuous refinements enabled by community involvement. It's fascinating to see these more recently developed algorithms become widely accessible to anyone who wishes to experiment. Furthermore, some of these projects have achieved remarkable performance, enabling real-time video enhancement even on personal computers, making advanced AI tools accessible without needing cloud computing or specialized hardware.
Another advantage of many open-source solutions is their integration capabilities. Often, they're designed with plugins or application programming interfaces (APIs) allowing them to work seamlessly with existing video editing platforms. This means they can enhance established workflows rather than requiring users to adapt to a new system entirely, which can boost efficiency and productivity.
The open-source model also allows for a continuous feedback loop, with user communities playing a critical role in the evolution of these tools. Iterative updates and improvements are driven directly by user input, fostering a rapid development cycle that can be difficult to replicate in commercial settings where access to users can be limited. There's a notable difference in transparency here too. Many open-source projects strive to be open about their development process and algorithms. Users aren't confined to using a black box, giving them the possibility to examine and possibly adjust the code itself. It provides a stark contrast to commercial products that may conceal their internal workings.
However, this open development model does present challenges. Funding and sustaining these projects can be difficult, often relying on volunteer contributions and community donations, which can limit the speed of development or even cause promising projects to stall due to resource constraints.
The open-source community's commitment to making video enhancement accessible to a wider audience extends to knowledge sharing. There's often a rich trove of educational materials, enabling individuals regardless of background or training to learn about AI video technologies. The open access provided by many open-source tools contrasts with the commercial market, where access to training or deeper knowledge may be limited without a significant investment in products or licenses.
Open-source developers are also leading in developing improved benchmarking methods. These new evaluation methods provide a more nuanced and consistent approach to assessing performance than traditional, commercial standards, potentially revealing unique advantages of different open-source solutions.
Ultimately, the increasing importance of open-source video enhancement is democratising access to powerful tools. This creates opportunities for individuals and creators from underrepresented communities or areas with limited resources, potentially enabling them to elevate their storytelling and visual content creation without the financial barriers often associated with commercial software. It’s a trend worth keeping a close eye on, as it shows the potential of democratizing innovation through open collaboration.
More Posts from ai-videoupscale.com: