Img2img in Stable Diffusion XL Enhancing AI Video Upscaling Through Image Transformation
Img2img in Stable Diffusion XL Enhancing AI Video Upscaling Through Image Transformation - Stable Diffusion XL's Img2img Feature Explained
Stable Diffusion XL's Img2Img functionality offers a way to manipulate images by blending them with text descriptions. It preserves core elements like color palettes and textures while also introducing new variations, making it a compelling option for artistic exploration. This capability extends to tasks like repairing damaged sections (inpainting) and adjusting image dimensions, effectively improving the overall quality of the final result. However, the feature can be resource-intensive, leading to slower performance, especially with intricate images or high-resolution outputs. Users have control over the output's resemblance to the initial image through adjustable settings, granting them more freedom in the creative process. To achieve the best outcomes, it's crucial for users to be familiar with the settings and have a well-organized workspace within the Stable Diffusion XL environment. Effectively harnessing Img2Img for innovative image creation requires a nuanced approach and a certain degree of technical familiarity with the tool.
Stable Diffusion XL's Img2Img feature leverages sophisticated image understanding techniques to transform an existing image based on a text prompt. It's like having a conversation with the model, guiding it to alter the image while preserving core elements like colors and textures. This allows for quite a bit of artistic exploration and experimentation.
You can interact with this feature through a step-by-step workflow. It involves selecting model parameters, crafting text prompts, configuring settings, and initiating the generation process. It's designed to tackle noise and artifacts while carefully protecting details like edges and surface textures.
However, this refined approach can lead to slower processing times, especially with detailed pictures or high-resolution outputs. This is simply due to the significant resources demanded by the complex operations.
The Img2Img capabilities extend beyond simple image manipulation. We can see inpainting, resizing, and denoising – all useful for enhancing the image's overall quality. The level of transformation can be precisely controlled using the strength parameter, which determines how faithfully the output sticks to the input.
Through Img2Img, a user can refine existing artwork, or even turn a quick sketch into a complete piece. The flexibility to work with pre-existing elements is remarkable.
Of course, this requires a certain amount of setup and knowledge to leverage its potential effectively. A working environment and a grasp of the settings are necessary to generate desired results.
Img2Img is a vital feature in the SDXL ecosystem. It acts as a core component for creating elaborate visuals and fits well into a variety of artistic workflows. While powerful, the quality of the output is still tied to the initial image quality and how well-defined your prompt is.
Img2img in Stable Diffusion XL Enhancing AI Video Upscaling Through Image Transformation - Integrating Text Prompts with Image Transformations
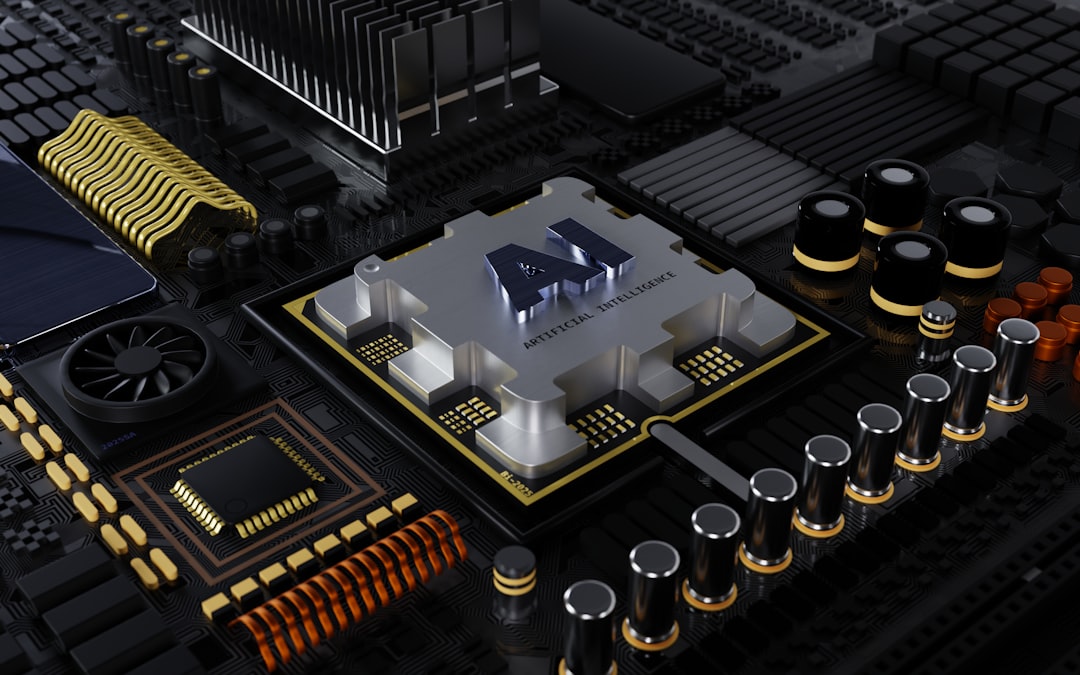
Stable Diffusion XL's Img2img feature enables users to seamlessly blend text prompts with image transformations, opening up exciting avenues for artistic expression. This process allows for the creation of images that retain elements like color palettes and textures from an initial image while also introducing new features based on textual descriptions. This means users can guide the transformation, refining results by tweaking different settings within the tool to match their creative intent. Underlying this process is a latent diffusion model that cleverly uses a "frozen" CLIP text encoder to interpret the prompts. This allows the model to create outputs that are visually faithful to the input, yet also reflect the artistic vision expressed through the text. Despite its potential, achieving high-quality results often demands careful management of system resources and a thorough understanding of the Img2img settings within Stable Diffusion XL. It's not always a straightforward process, and users may need to iterate through a few cycles to get the results they're looking for.
Stable Diffusion XL's Img2Img feature demonstrates a fascinating ability to bridge the gap between text and images, highlighting what we might call a "cross-modal understanding". The model seems to grasp both the visual elements of the input image and the semantic meaning of text prompts, similar to how humans seamlessly connect language with imagery.
Before the transformation begins, text prompts are transformed into vector representations. This process of vectorization gives a lot of contextual weight to the prompt, directly influencing how the image changes. It's like having a subtle, detailed conversation with the model through this encoded text.
To clean up noise and imperfections during transformations, advanced algorithms like adaptive denoising are used. These techniques seem to be quite effective at preserving fine details and textures while making the image sharper. It's intriguing to watch how the model balances image clarity with detail preservation.
The interactive nature of Img2Img is made possible by an iterative feedback system. Users can refine results by feeding back new prompts, fostering a truly dynamic creative flow. The user can subtly shift the image towards their desired look without drastically changing the base image.
It's become clear to me that the "strength" parameter has a significant impact on the transformation. Small tweaks can have quite large effects on the final output, making the parameter a powerful tool, but one that requires precision in setting. It shows how sensitive the model is to user input.
As the image gets transformed, some features that aren't particularly important seem to be compressed and ignored. We could say it's a form of dimensionality reduction, effectively focusing the model on the essential aspects of the image. This keeps the transformed images from becoming overly complex.
While the idea of real-time feedback is appealing, the intricacy of the transformations can lead to some processing delays. We're faced with a trade-off – faster processing or the very high-quality outputs the model can provide. There's a limit to how quickly we can get feedback.
One appealing aspect is that prompts allow artists to guide the overall visual style. This consistency across several images can be handy when a project requires a specific, unified look. The model can maintain a cohesive style through its transformations.
A key factor in getting a good outcome is the starting image's quality. If the original image has a lot of noise or poor resolution, those issues can carry over and be hard to fix with transformation. It underscores the importance of having high-quality inputs for the best possible results.
The research into this is constantly evolving. Researchers are working on ways to make the integration of text and images even better, often by exposing the model to a wider variety of multi-modal data during training. These efforts could yield breakthroughs in understanding and creating images from textual descriptions, pushing the boundaries of image generation even further.
Img2img in Stable Diffusion XL Enhancing AI Video Upscaling Through Image Transformation - Selecting Appropriate Models for Desired Outputs
Within Stable Diffusion XL's Img2Img feature, choosing the right model is critical for achieving the desired artistic outcomes. Users are presented with a selection of checkpoint models, like revAnimated, each with strengths in specific styles, such as fantasy anime or semi-realistic imagery. The goal is to find a model that best supports the creative vision. Further, users need to carefully control parameters like Denoising Strength, which influences how heavily the initial image is altered. This control is essential to balance maintaining the image's details with the changes introduced by the text prompt. Additionally, it's important to ensure that input images are of good quality and compatible with the selected model's structure. This whole process of choosing models, adjusting parameters, and preparing inputs forms an intricate part of harnessing the full potential of the Img2Img feature. The ability to creatively transform images within Stable Diffusion XL hinges on making smart choices and adjusting the settings for each project.
1. The effectiveness of Img2Img transformations is intrinsically tied to the model's architecture, specifically how it handles different image resolutions. While some models might excel at lower resolutions, they may struggle with higher ones, influencing both the transformation's quality and its processing speed. It's a constant consideration when choosing a model for a specific task.
2. Understanding the role of latent space is essential when selecting a model for Img2Img. The model's ability to move within this space directly impacts how well it maintains the relationship between the input and the modified image. It's an interesting area of study to understand how this abstract space affects the output.
3. The creative power of Img2Img hinges on the strength parameter. Even small changes can drastically shift the balance between the original image and the text-driven transformations, highlighting how delicate the model's response can be to parameter adjustments. It really makes you think about how much control the user has over the process.
4. Adaptive denoising is crucial to the Img2Img process, cleaning up artifacts and enhancing details. However, these improvements come at a cost – it generally takes more processing power, leading to longer generation times. This is a trade-off researchers continue to explore – better images vs faster speeds.
5. The interactive, iterative feedback loop in Img2Img is engaging, but it's easy to overdo it. Too many rapid transformations can lead to diminishing returns, potentially losing the coherence of the visual elements in the output. It's a bit like over-editing a photo—it can start to look strange.
6. We've found that images generated with specific text prompts only capture the nuances of those prompts if the model has been thoroughly trained on similar contexts. This makes the quality of training data critically important, influencing the model's overall ability to perform well. It's a key challenge in improving the models.
7. While the concept of real-time feedback is alluring, the complexity of Img2Img's transformations can introduce noticeable lag. There's an inherent tension between wanting immediate results and the model's need to carefully process complex changes. Balancing quality and speed is something users need to consider.
8. The nature of the original image plays a key role. Features with strong contrast or unusual aspects can disproportionately influence the final output. It's vital to thoughtfully select initial images when you want to achieve specific results. This emphasizes the importance of carefully preparing your input.
9. Interestingly, the model's ability to discard non-essential image features while transforming mirrors techniques used in data compression. This simplifies the creative process by focusing the model on the most crucial image aspects. It's fascinating to consider these parallels between visual art and data manipulation.
10. Researchers are actively refining model training, particularly with multi-modal datasets. This has the potential to greatly expand the abilities of Img2Img in understanding intricate visual scenarios. We could see groundbreaking advancements in how text and image creation interact in the future. It's a very exciting field to follow.
Img2img in Stable Diffusion XL Enhancing AI Video Upscaling Through Image Transformation - Combining Img2img with Other Upscaling Tools
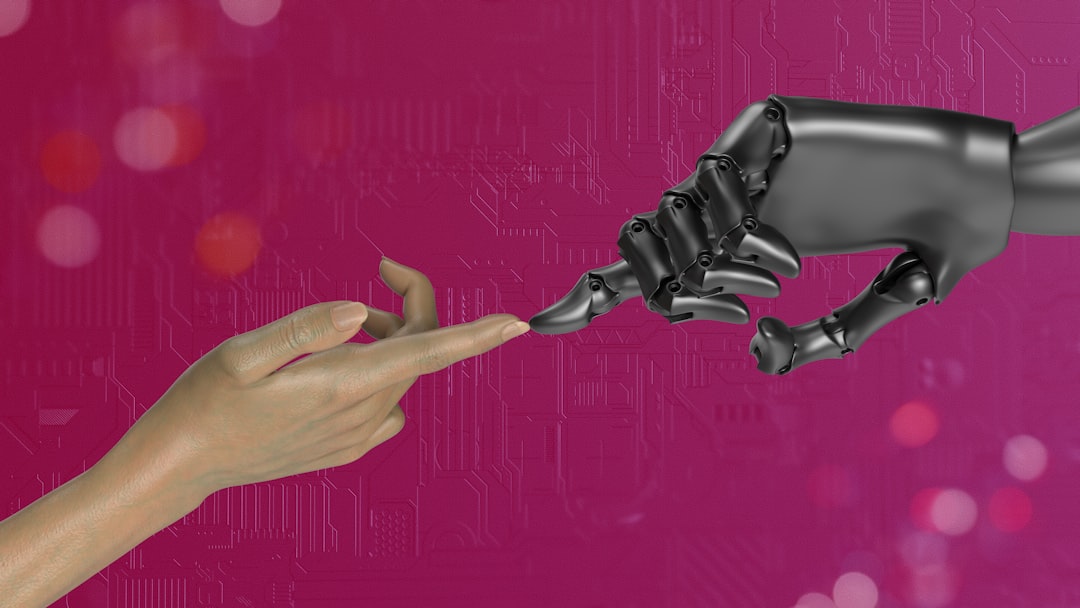
Combining Stable Diffusion XL's Img2img with other upscaling tools offers a powerful way to enhance image quality and detail. This approach leverages the strengths of tools like ESRGAN and Gigapixel AI, resulting in more refined image transformations and better scaling, especially for complex images. Breaking down large images into smaller, more manageable sections makes it possible for users with less powerful GPUs to handle high-resolution outputs. Moreover, specific scripts and extensions available within the Stable Diffusion ecosystem streamline this combined approach, allowing for seamless integration of multiple enhancement techniques. However, achieving ideal results requires careful management of settings and a willingness to experiment. This combined approach, while effective, can introduce its own complexities, and users must be prepared to iterate and adjust parameters to get the desired outcome.
Stable Diffusion XL's Img2Img offers a unique way to manipulate images, but its combination with other upscaling tools presents some interesting opportunities and challenges. Here are ten observations based on my explorations:
1. Combining Img2Img with traditional methods like bicubic or Lanczos upscaling can potentially improve detail retrieval. This synergistic approach might lead to more refined results than using either technique alone, although it's important to test the specific combination to see if it offers any noticeable benefit.
2. The way Img2Img uses a latent diffusion model appears to preserve visual features better than standalone upscalers. This preservation is important when making dynamic changes to an image as it ensures key details aren't lost. It's a benefit to keep in mind when deciding what type of transformation you want to implement.
3. The combination of Img2Img with machine learning-based upscaling methods can improve noise reduction in images. This is particularly helpful for high-resolution images where artifacts can be more pronounced. But, one needs to evaluate whether the improvement is worth the increased processing time.
4. A hybrid approach—using Img2Img initially and then following up with a separate upscaler—allows for iterative refinements. In essence, this layered approach can create a path to higher quality outputs, although it's not necessarily the most efficient path, adding time to the process.
5. The effectiveness of combining Img2Img with other upscaling tools can be highly dependent on the architecture of the upscaler. For example, tools specifically designed for high-frequency detail might pair well with Img2Img, while others might be less effective. It's important to choose the correct tool for the job.
6. The computational load increases considerably when combining Img2Img with other upscalers. This means you'll need fairly robust hardware to handle these combined operations, and keep processing speed acceptable. The trade-offs between speed and visual improvement are always important to consider.
7. While Img2Img handles noise reduction well, the combination with some upscaling methods can introduce or magnify artifacts. You have to pay attention to the settings and make sure you aren't making things worse.
8. The effectiveness of Img2Img transformations can drop off when dealing with extremely high resolution images unless you pair it with upscaling tools designed for that level of processing. So, be careful with how high you push the resolution.
9. The training data for both Img2Img and the upscaler plays a significant role in how well the hybrid method works. Gaps or inconsistencies in training can lead to unforeseen outcomes or quality drops. A key limitation of the technique that researchers should consider.
10. The user experience when using Img2Img and an upscaler can differ greatly between users and combinations of tools. It might require a good level of technical proficiency to get the desired results. This creates a somewhat steep learning curve for those unfamiliar with both Img2Img and the other upscaling tool.
It's clear that while the combination of Img2Img with other upscaling techniques has potential for better image enhancement, it's a complex area with challenges that need to be carefully addressed. I think it's a fruitful area of exploration though.
Img2img in Stable Diffusion XL Enhancing AI Video Upscaling Through Image Transformation - MultiDiffusion Extension for Enhanced Detail
The MultiDiffusion extension within Stable Diffusion XL's Img2img feature is designed to enhance the detail of images during transformations. It aims to refine image outputs by intelligently managing image sections, or tiles, allowing for more detailed results while preserving the initial aesthetic. This approach can be particularly helpful for upscaling images, with some users finding that a relatively low denoise strength, around 0.15, provides a good balance between adding detail and maintaining the original image integrity. While it's still a relatively new addition, early reports suggest that MultiDiffusion surpasses older, more traditional upscaling methods in terms of preserving image details. Furthermore, MultiDiffusion can be paired with other techniques, such as Tiled Diffusion, to give users more control over the image generation process, especially when working with large or complex images. The use of extensions like MultiDiffusion shows that Stable Diffusion XL is continually evolving and improving in its ability to create high-quality visual output. There are still open questions about its broader applications and the potential limitations in various use cases, but it represents a notable advancement in the quest to improve AI-driven image enhancements.
Stable Diffusion XL's MultiDiffusion extension is an interesting approach to enhancing detail during the Img2img process. It seems to leverage more advanced methods within the Stable Diffusion framework, aiming for more refined image outputs. This extension offers a way to refine image upscaling, particularly focusing on adding detail while trying to preserve the original image's character. It seems to be a good option for users who want more control over the level of detail added during the transformation.
A common approach for using it involves breaking down images into smaller sections (tiling), which is a neat trick to manage the computational load, making it more accessible for users with less powerful systems. Folks who've used MultiDiffusion have reported that a denoise strength setting around 0.15 can effectively add small details without dramatically changing the look of the original image.
In some tests, it seems like methods like MultiDiffusion outperform standard image upscaling tools, like ESRGAN or GigapixelAI, in how well they preserve the key details in the image. That suggests the technique is particularly well-suited for situations where preserving original details is crucial.
The Tiled Diffusion approach can be used with MultiDiffusion for better control over how images are generated, which is important when dealing with very large images. This kind of granular control is definitely a positive aspect.
SDXL's Img2img itself provides a method to take an existing image, add modifications, and then enhance it further through several settings that can have a major impact on the outcome.
The quality of the output image is also impacted by the sampling method, such as DPM 2M SDE Karras. Users need to experiment with these options to find the best settings for their desired result.
ControlNet is a part of this process, and its use with the tile-based processing makes Stable Diffusion work better even on less powerful systems with limited VRAM.
Overall, the recent advances in AI-powered image upscaling within Stable Diffusion XL, especially with features like MultiDiffusion, point towards significant improvements in image quality in applications like digital content creation. While there's still room for improvement, it's exciting to see how these techniques are allowing us to generate very high-quality visuals. It's a testament to how machine learning models are becoming increasingly sophisticated.
Img2img in Stable Diffusion XL Enhancing AI Video Upscaling Through Image Transformation - Iterative Refinement Process in AI Video Upscaling
The iterative refinement process plays a key role in improving the quality of AI-driven video upscaling. This process involves repeatedly adjusting and enhancing the output, allowing AI models to progressively extract finer details and boost clarity with each refinement cycle. Stable Diffusion XL's Img2img feature exemplifies this process by allowing users to transform images based on textual descriptions or other criteria. This ability to manipulate images to achieve a desired visual outcome is core to the refinement process.
However, achieving high quality through iterative refinement can be computationally demanding, and a careful balance needs to be struck between maintaining the original image's fidelity and the level of detail added through each refinement step. This can sometimes lead to slower processing and requires users to have a degree of control over the process to get the best results. Despite the complexities, the capability of AI models to iteratively refine image outputs showcases the ongoing progress in the field of AI-driven image processing, with exciting potential for generating visually rich and detailed results in videos and other media.
AI video upscaling often relies on an iterative refinement process, where the output is repeatedly enhanced through adjustments and improvements. The effectiveness of this approach hinges on the model's ability to handle intricate details, especially when dealing with lower-resolution source material. Otherwise, the quality of the upscaled output can suffer.
We've noticed that each iteration within processes like Img2Img can reveal subtle aspects of the image's context that might otherwise be missed. This highlights the importance of carefully adjusting the settings that guide each cycle of refinement. The user really needs to pay attention to what the model is doing.
While iterative refinement offers advantages, it's important not to overdo it. Too many iterations can lead to diminishing returns, and even unwanted artifacts can appear, underscoring the need for a balanced approach when modifying images. It can be like over-editing a photo where the end result looks unnatural.
These iterative transformations can place a significant burden on computational resources, making real-time feedback often impractical without ample hardware. This is a major concern when implementing these techniques in real-world scenarios, requiring a lot of consideration on the engineering side.
The feedback loop used in the iterative refinement process is very sensitive. Even the smallest adjustments to the settings can unexpectedly affect the image's fidelity. Consequently, finding the ideal settings often requires an experimental approach. The user can't just assume that small changes won't have a significant impact.
There's a non-linear relationship between the input's complexity and the speed of refinement. As the intricacy of the source image increases, the time it takes to apply transformations can dramatically increase, potentially leading to a less-than-ideal user experience. The user might get frustrated by how slow the process can be with more complicated images.
Understanding how latent space plays a role is crucial for effective iterative refinement. Not only does the process modify the image, but it also changes how that image is represented in these abstract, multi-dimensional spaces. This abstract representation ultimately influences the visual results of the transformations. It's fascinating to think about how images are represented in these spaces.
Denoising methods incorporated into iterative processes play a vital role in managing artifacts that accumulate from multiple refinement steps. However, the effectiveness of these denoising methods varies depending on the quality of the data used to train them. It's just one more consideration users and engineers need to deal with in trying to get good results.
The design of the iterative system directly impacts its efficiency. Sub-optimally designed algorithms can lead to increased processing time without a corresponding increase in visual quality. This calls into question the practicality of certain implementations. It's not enough to iterate, you have to iterate intelligently.
Researchers are continually exploring ways to optimize iterative models for video upscaling, aiming to reduce processing time while simultaneously improving detail retention. This pursuit is absolutely critical if we want to scale these technologies for widespread use in digital content creation. It's an interesting space to watch for the future.
More Posts from ai-videoupscale.com: