Upscale any video of any resolution to 4K with AI. (Get started for free)
7 Proven AI Techniques to Restore Resolution in Degraded Historical Photographs
7 Proven AI Techniques to Restore Resolution in Degraded Historical Photographs - Creating Missing Data Through Deep Face Recognition
Deep face recognition has become instrumental in enhancing the restoration of degraded facial images, especially by generating synthetic data to fill in missing information. This involves converting poor-quality images into higher-quality versions, addressing issues like dim lighting and parts of the face being obscured. AI, particularly through the use of generative adversarial networks (GANs) and sophisticated composite networks, has transformed the field of facial image super-resolution, leading to clearer and more detailed restored images. These methods hold potential for applications in areas like forensic science and surveillance, but require a nuanced understanding of their limitations. The current state of these AI techniques relies heavily on supervised training, which means they need pairs of high- and low-quality images. This poses a challenge because a large and varied collection of such data is often difficult to obtain. As deep face restoration technology advances, its potential applications grow, but careful consideration of ethical implications and potential drawbacks remains crucial for responsible development and usage.
1. Deep face recognition (DFR) systems are showing promise in restoring missing facial details in old photographs, using intricate pattern recognition to fill in the blanks caused by deterioration or damage. These methods essentially learn to "fill in the gaps" by recognizing the structure and common features of faces.
2. Many of these DFR techniques employ generative adversarial networks (GANs). These systems essentially pit two neural networks against each other: one creates a plausible facial reconstruction and the other judges whether it's realistic. This adversarial training process can lead to incredibly believable face restorations, even when significant parts are missing.
3. The quality and diversity of the training data significantly influences how well these systems work. Models trained on a larger and more varied collection of faces generally create more reliable reconstructions when confronted with historical images. This is because the models build a richer understanding of facial variation and can therefore adapt to different situations in degraded photographs.
4. DFR models often use facial landmarks—key points like the corners of the eyes, nose, and mouth—as guides to help them reconstruct the missing details with precision. This precise reconstruction allows for a smoother blending of the restored section with the undamaged part of the image.
5. A major concern with this technique is the potential for bias. If the training data lacks diversity in ethnicity or other characteristics, the model might struggle with accurately restoring features for underrepresented groups. This emphasizes the need for training datasets that truly reflect the range of human facial variations.
6. The ability to reconstruct facial aging has recently become possible using these methods. This means the system could restore an individual at different ages based on the remaining facial features. This can greatly enrich historical narratives by allowing a more comprehensive understanding of an individual's life stages.
7. Unlike traditional manual restoration processes, DFR automates the process of feature repair, minimizing human errors and allowing for the scalable restoration of large collections of photos. This is especially valuable when dealing with large archives of historical images.
8. However, using these techniques on contemporary images raises privacy concerns. DFR could potentially recreate and disseminate realistic images of individuals without their consent, prompting ethical questions about data usage and the boundaries of consent.
9. The incorporation of contextual information from the surrounding photograph – like clothing style and background features – can substantially enhance the realism of the restored images. The algorithms use these surrounding cues to make more informed guesses about what the missing facial details might look like.
10. The evolution of deep learning architectures continues to refine DFR methods. It's likely that future systems will be capable of learning from a smaller amount of training data, thereby potentially accelerating the image restoration process and making it more accessible for different applications.
7 Proven AI Techniques to Restore Resolution in Degraded Historical Photographs - Noise Removal with Generative Adversarial Networks
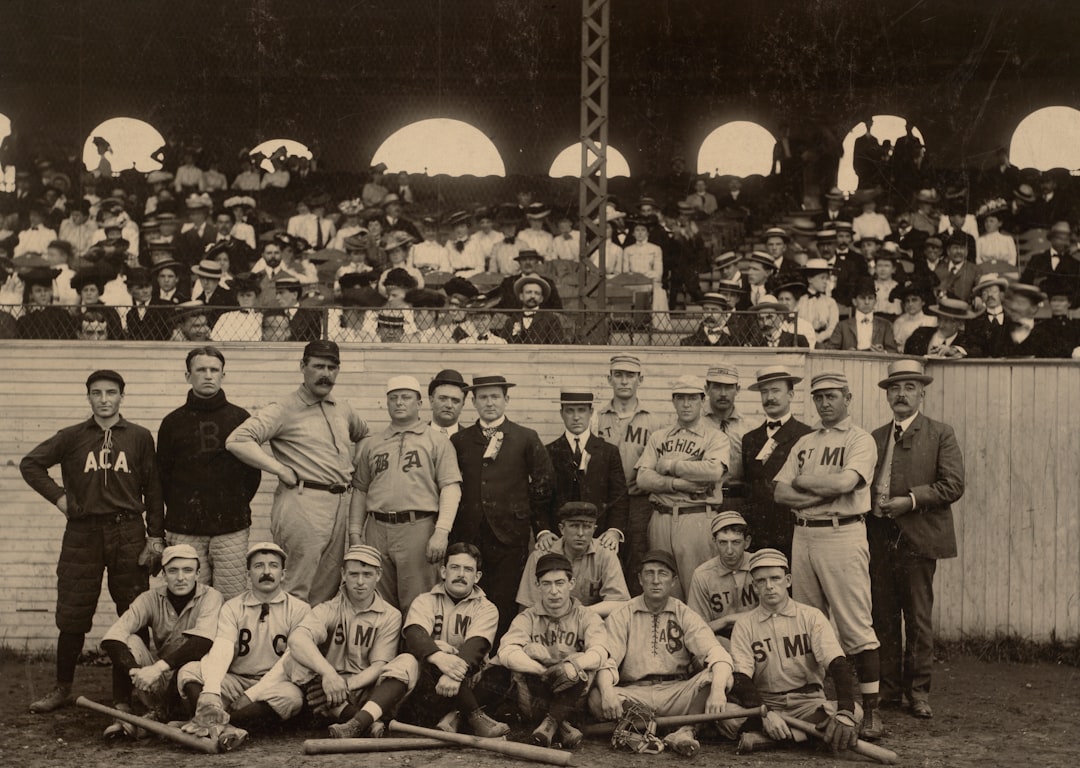
In the quest to revitalize degraded historical photographs, Generative Adversarial Networks (GANs) have emerged as a powerful tool for noise removal. GANs, with their ability to synthesize new data, are particularly adept at tackling the challenge of removing mixed noise, like the combination of Gaussian and impulse noise. The DeGAN model is a prime example of this, using a "generator vs. discriminator" framework to refine image quality. A notable contribution of DeGAN is its incorporation of a loss function that considers how humans perceive images, improving the noise reduction process while retaining essential features like edges and textures. This is vital in historical photograph restoration, as maintaining the integrity of the original artwork is critical.
Despite their effectiveness, GAN-based noise removal methods are not without limitations. The quality of restorations depends heavily on the diversity of the training data. If the training data doesn't include a wide range of photographic styles and noise types, the resulting models may struggle to accurately remove noise in certain historical photos, potentially introducing unintended artifacts or biases. This emphasizes the importance of carefully considering the training data used in these restoration processes. While GANs are a significant step forward in noise reduction for historical images, ensuring robust and unbiased results requires ongoing development and thoughtful dataset creation.
Generative adversarial networks (GANs) present a promising approach for noise removal in old photographs, leveraging their ability to learn complex noise patterns. They can intelligently distinguish between noise and actual image features, enabling them to remove artifacts while preserving the integrity of facial details, ultimately enhancing the overall fidelity of restored images. However, training GANs for this purpose is computationally demanding. They often require extensive iterations over massive datasets to fine-tune their ability to differentiate between noise and essential details, potentially impacting the time and resources needed for effective restoration.
Despite the computational demands, GANs can achieve remarkable results, often recovering details even in severely degraded images, essentially bringing lost artifacts back to life. This ability surpasses the capabilities of conventional noise reduction filters which rely on simpler statistical methods. GANs, in contrast, learn from the complex patterns within the data, allowing them to dynamically adjust their noise removal strategies based on the image's unique characteristics, leading to superior outcomes.
A common training approach involves a two-stage process: initially training the GAN with synthetic data, then fine-tuning it on genuine historical datasets. This strategy helps address the scarcity of suitable training data, a major challenge in this domain. GANs don't just remove noise; they can transfer learned features from high-quality images to enhance the clarity and even the color palette of degraded images. This means that even faded or monochrome historical photos can be enhanced with more vibrant and realistic colors, enriching the restoration process.
Interestingly, the GAN architecture itself can be tweaked to focus on specific image components, such as facial expressions, while simultaneously managing noise removal. This targeted approach ensures the preservation of important emotional cues present in historical figures' portraits. Yet, achieving consistently high performance with GANs can be challenging due to the variety of noise characteristics found in historical photos. Scratches, fading, and color distortions require tailored approaches for optimal restoration, highlighting the difficulty in developing universal solutions for diverse types of degradation.
By using visualization techniques, we can gain insights into how GANs identify noise versus real details. This interpretability helps us understand and improve the algorithms, leading to even better noise removal methods. It's crucial to note, however, that GANs can introduce their own artifacts if inadequately trained or supervised. This risk highlights the necessity for meticulous model evaluation before applying GANs in real-world restoration projects to ensure that the pursuit of noise removal doesn't inadvertently erase crucial details in the process.
7 Proven AI Techniques to Restore Resolution in Degraded Historical Photographs - Upscaling Resolution Through Neural Super Resolution
Neural Super Resolution (NSR) signifies a major leap in image enhancement, particularly valuable for restoring historical photographs. These techniques use advanced deep learning models, such as Convolutional Neural Networks (CNNs), to transform low-resolution images into higher-resolution versions while safeguarding fine details. Often focused on single images through Single Image Super Resolution (SISR), NSR offers a more sophisticated approach to image enhancement than traditional methods. The quality of the results is directly tied to the quality and diversity of the training datasets used to develop the neural networks. This is a crucial factor when considering the application of NSR to historical photographs, where relevant training data might be limited. Although the ability to quickly and meticulously restore details is appealing, it's vital to acknowledge potential biases and shortcomings that might arise in real-world applications.
Neural Super Resolution (NSR) uses a process called "upscaling" to boost the resolution of an image, essentially turning low-resolution historical photographs into higher-resolution versions without losing significant details. This is different from traditional methods that just fill in pixel gaps based on surrounding pixels. Instead, NSR uses deep learning to understand the overall context of the image, allowing it to predict missing high-frequency details and create a more realistic and coherent restoration.
The training of NSR models often involves large datasets of diverse images. This helps the models learn a variety of textures, patterns, and details, allowing them to be applied to various types of historical photographs more effectively. The power of NSR comes from its ability to model the complex relationships between pixels in an image. This allows neural networks to predict the appearance of missing details while also preserving the overall natural look of the photograph by considering how things like lighting and perspective interact.
However, NSR faces challenges with heavily degraded images. If a photograph is too damaged or lacks enough context and recognizable features, even advanced neural networks may generate unnatural enhancements or fail to produce satisfactory results. Research is revealing that NSR can be adapted to handle specific types of images or subjects. For example, you could potentially enhance landscapes differently than urban settings or treat portraits distinct from abstract art, suggesting versatility in restoration applications.
Transparency in how the model works is increasingly important. Some NSR architectures now provide methods to see how the model processes images, helping researchers better understand the model's choices. This deeper understanding allows for more informed adjustments and improvements. Applying NSR to sequences of images adds another dimension. It enables us to create a smoother visual experience when viewing the series, improving the overall narrative of historical events by considering the images together.
One drawback of NSR is the possibility of introducing artifacts—unwanted distortions—during the upscaling process. It is crucial to carefully test and validate NSR models to prevent these artifacts and ensure that restorations don't compromise the original historical value of the images. As neural network technology continues to improve, researchers are focusing on decreasing training time and increasing restoration quality. This includes techniques that allow models to perform well even with limited training data (few-shot learning), which can potentially make NSR more accessible for a broader range of historical preservation projects.
7 Proven AI Techniques to Restore Resolution in Degraded Historical Photographs - Smart Colorization with DeOldify Architecture
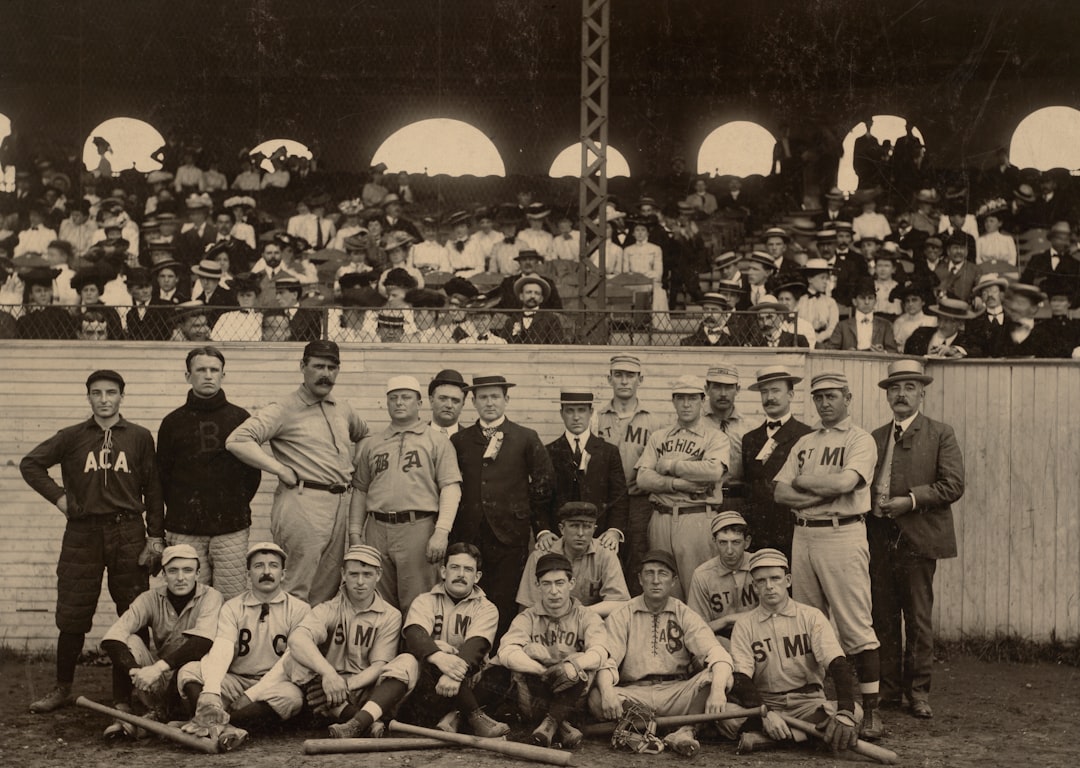
"Smart Colorization with DeOldify Architecture" introduces a compelling approach to breathing life into old black-and-white photographs by intelligently adding color. DeOldify, an open-source project spearheaded by Jason Antic, utilizes a deep learning model built upon a ResNet34 backbone within a U-Net structure. This model, refined through a method called NoGAN, aims to produce high-quality colorizations. The technology can be used on both still images and videos, where individual video frames are colorized separately before being reassembled into a coherent sequence.
While the technology can generate vibrantly colored and detailed images, it relies on user adjustments of settings like rendering resolution and various parameters. This flexibility allows for optimization but also introduces a level of uncertainty regarding the consistency and accuracy of the colorization across different images or segments of a video.
Despite these potential inconsistencies, DeOldify remains a prominent tool in the field of image restoration. It exemplifies the capabilities of AI-driven approaches in bringing historical images to life through intelligent colorization. However, the technology also serves as a reminder of the ongoing challenges and nuances within the field of image restoration as researchers continue to refine and optimize the colorization process.
DeOldify is a fascinating AI project, spearheaded by Jason Antic, that's designed to breathe color back into old black-and-white images and film footage. It's open-source, allowing anyone to explore and modify its inner workings. The core of DeOldify's capabilities is its clever blend of deep learning techniques. It utilizes a ResNet34 architecture, a type of convolutional neural network, embedded within a U-Net framework. This combination helps DeOldify effectively learn the intricate patterns and structures within images. During the training process, it leverages a variation of GANs called NoGAN, repeating the training cycle five times to achieve higher colorization quality.
It's notable that DeOldify isn't limited to single images – it can also tackle videos. It essentially processes each frame individually before stitching them back together, resulting in a colorized video. Users have a degree of control over the output through adjustable parameters and render resolution, allowing for some fine-tuning of the restoration process. The results can be quite impressive, with DeOldify generating vivid colors and a high level of detail, earning it the distinction of being a cutting-edge approach to image restoration. The project has also evolved to enhance the stability of colorized videos, mitigating potential motion artifacts.
Considering that color photography only became widely available after the 1960s despite its invention much earlier, initiatives like DeOldify take on significant relevance. It allows us to visualize and appreciate the world as it was through a different lens. At its core, DeOldify's approach relies on image-to-image translation – a key area of image processing research – to transform grayscale into colored representations. However, one aspect I find quite intriguing is how the quality of the results depends heavily on the training data. The model needs access to a diverse range of images and color palettes to make accurate and realistic colorizations, which can be a challenge when working with historical images. If the training data isn't diverse or lacks the proper contextual nuances, the colorization can fall short.
Furthermore, DeOldify, like many AI models, faces some limitations. For example, extremely complex scenes, with multiple subjects or intricate backgrounds, can cause the model to struggle. It occasionally introduces what we might call "pseudo-colorization," where colors appear unrealistic or exaggerated. While these limitations are not unusual, they highlight the ongoing development needed to enhance its capabilities. Interestingly, DeOldify is implementing approaches to generate synthetic data, which helps address biases in training datasets and can improve equity in the restoration of images from diverse cultural backgrounds. This innovative approach allows for more accurate and historically responsible colorizations. Overall, DeOldify offers a powerful example of how AI can be utilized to restore and enhance our understanding of the past, but it also serves as a reminder of the importance of high-quality training data and ongoing research in this field.
7 Proven AI Techniques to Restore Resolution in Degraded Historical Photographs - Texture Enhancement via Adaptive Sharpening Models
AI-powered restoration of old photographs often involves enhancing textures that might be lost due to degradation. Adaptive sharpening models provide a way to improve these textures and make the overall image look better. These models aim to enhance the visual quality of photos by focusing on textures that might be faint or missing in degraded images. Some new approaches, like the EvTexture model, incorporate event signals to better restore intricate textures. By focusing on high-frequency details, these methods show promise in exceeding the limitations of older texture restoration techniques.
However, even with the promise of these techniques, problems arise when dealing with images with very complicated textures. This reveals that the field of AI-driven image restoration still needs work to truly solve these challenges and ensure a better quality of restoration. A crucial aspect of enhancing these old images is the ability to rebuild natural-looking textures so they appear as close to the original as possible. These methods contribute to the greater goal of reviving the original quality and authenticity of these historical visual records.
1. Adaptive sharpening methods focus on improving image detail while carefully avoiding the introduction of unwanted artifacts. This balance is crucial, as excessive sharpening can distort textures, creating an unrealistic and undesirable outcome.
2. A promising approach involves analyzing images at multiple scales, adjusting the level of sharpening based on the content within each section. For instance, facial features might require a different approach than background elements to ensure a natural and consistent look.
3. Surprisingly, the historical context of an image can influence how effective adaptive sharpening is. If a model understands typical stylistic elements from a particular period, it can enhance textures in a way that's faithful to the original aesthetic of the photograph.
4. Maintaining the integrity of edges is a critical aspect of adaptive sharpening. Edge preservation algorithms help ensure that sharp transitions in an image aren't amplified or lost, contributing to a photograph's authenticity.
5. Adaptive sharpening models can dynamically adjust parameters in real-time based on the image content, allowing for more subtle and accurate restorations. This adaptability is particularly beneficial for historical photographs, which often exhibit inconsistencies in quality.
6. Some adaptive sharpening models use frequency domain analysis to selectively enhance textures based on their spatial characteristics. This advanced method can dramatically improve the perception of detail in degraded photographs.
7. Research suggests that machine learning-based adaptive sharpening models can learn user preferences over time. This means that the model can adapt its output to match specific stylistic choices made during previous restoration projects.
8. The effectiveness of texture enhancement can be significantly impacted by the initial resolution of a photograph. Low-resolution images might respond differently to sharpening algorithms compared to higher-resolution images, influencing the overall restoration process and time required.
9. Several advanced techniques integrate deep learning principles, training neural networks on extensive image datasets to improve their sharpening abilities. This leads to greater adaptability and more refined control during restoration.
10. A potential drawback of texture enhancement models is the risk of creating "ringing" artifacts – unwanted halos that appear around sharp edges. Carefully adjusting model settings is essential to prevent these artifacts and preserve the original integrity of the photograph.
7 Proven AI Techniques to Restore Resolution in Degraded Historical Photographs - Damage Repair Through Image Inpainting Networks
Image Inpainting networks use sophisticated techniques to automatically rebuild damaged or missing parts of photographs, mostly relying on deep learning approaches. Recent advancements in this field have led to improved performance using specialized architectures like VResNet and the application of Generative Adversarial Networks (GANs). GANs use a competitive learning framework to create highly realistic repairs. However, there are still challenges, particularly when it comes to accurately understanding the extent of the damage within an image and maintaining accurate colors and textures during the restoration process. Some of the limitations are also seen in traditional convolutional neural networks that sometimes create color inconsistencies and lose image texture. The ongoing development and research in image inpainting technology show potential for creating more accurate and sophisticated methods of restoring historical images, all contributing to the ongoing goal of preserving historical visual resources.
Image inpainting networks use convolutional neural networks (CNNs) to fill in missing or damaged parts of images by considering the surrounding pixels. They learn to intelligently complete these gaps, often creating very convincing restorations. The effectiveness of inpainting depends heavily on the specific network architecture. Techniques like U-Net and GANs have gained popularity because of their ability to capture both local and global features, leading to higher-quality results.
One of the more unexpected uses of inpainting networks is restoring not just photos, but also historical art. They can help reconstruct damaged paintings or sculptures where complete records are missing, highlighting the versatile nature of inpainting across different visual mediums. To avoid generating unrealistic results, inpainting networks often use loss functions that incorporate visual and contextual cues. These help gauge the quality of the repaired sections better than the traditional pixel-by-pixel methods.
Current research shows that advanced inpainting can handle many kinds of degradation – from scratches and missing pixels to seamlessly blending altered colors back into images. This adaptability widens the range of damaged historical materials that inpainting can be applied to. It seems the iterative process, where initial estimations are refined step-by-step, can lead to significantly improved results. This process involves making preliminary inpaintings and then adjusting them based on feedback from deeper parts of the neural network, which adds to the realism.
The success of inpainting depends not just on the algorithms, but also on the quality of the training data. Using a wide variety of representative samples during training can lead to models that generalize well across different styles and historical periods, improving their usefulness in real restorations. It's intriguing that real-time inpainting is becoming feasible with improved computational speed, which has implications for video restoration and dynamic media. This is a significant step forward, as it allows for seamless replacement of damaged sections in videos frame-by-frame, showcasing the potential of inpainting in interactive media.
Some researchers are exploring the idea of including user input into the process, allowing people to manually indicate areas that need restoration. This interactive approach not only customizes the results but also helps contextualize the restoration process based on historical importance or aesthetic preferences. The field of image inpainting continues to advance, aiming not just for visual restoration but also historical accuracy, which raises questions about authenticity. As these models get more complex, it could become increasingly difficult to tell the difference between original artifacts and AI-generated completions, emphasizing the need for transparent methods throughout the restoration process.
7 Proven AI Techniques to Restore Resolution in Degraded Historical Photographs - Edge Preservation with Convolutional Neural Networks
When restoring old photographs using artificial intelligence, particularly with Convolutional Neural Networks (CNNs), preserving edges is extremely important. CNNs are now being used in new ways to keep important image edges while also doing things like removing noise and increasing resolution. One technique involves using clean images to generate edge maps, which are then used to train CNNs. This helps improve the quality of restored photos without losing key details. Some CNN designs, like DnCNN, are built specifically to tackle the challenge of edge preservation during image restoration. This focus on edges has become increasingly vital in computer vision, highlighting the significance of edge preservation when restoring historical photos. While these improvements are promising, some researchers are critical of the over-reliance on deep learning solutions for edge preservation, especially in historically significant images. The challenge remains in balancing the use of advanced techniques with the need to maintain the original character of the photos.
Convolutional Neural Networks (CNNs) have become increasingly adept at preserving the edges of objects in images, which is especially crucial when restoring the details of historical photographs. Maintaining these edges is essential because they contribute to the overall authenticity and historical context within the image. Techniques like anisotropic diffusion and gradient preservation help CNNs differentiate between important image features and noise, which reduces the possibility of undesirable artifacts during enhancement.
It's surprising how effectively edge preservation can improve the restoration of not just low-resolution images, but also those that are significantly degraded. This is important for recovering both readily identifiable features and the valuable historical context embedded within the image. The interplay between edge detection and upscaling highlights the benefit of using multi-layered CNN architectures. By employing deeper networks, these models become better at extracting and representing edge features at different levels of detail, ultimately improving their capacity to restore intricate images.
However, there's a key challenge with edge preservation: overemphasizing edges can lead to unnaturally sharp transitions, potentially harming the cohesiveness of a restored photograph. Finding the right balance between enhancing details and maintaining a natural look remains an area of active research. Utilizing specialized edge detection filters within CNNs, like Sobel or Canny filters, helps ensure the retention of critical boundaries throughout the restoration process. These filters are often incorporated into the CNN's training process to systematically improve edge clarity.
Interestingly, CNNs are becoming increasingly adept at adapting their edge preservation strategies to specific content. This means edge detection can be optimized based on the characteristics of the photos being restored, whether a portrait, landscape, or abstract artwork. This advanced capability makes modern CNNs far superior to previous generation methods. The choice of activation functions within CNN architectures also affects edge preservation capabilities. Functions like Leaky ReLU or PReLU have proven particularly effective at maintaining edge integrity. Research has revealed that these functions can help reduce artifacts typically found with standard convolution operations.
The quality of the initial training data significantly impacts the effectiveness of edge preservation techniques within CNNs. A lack of diversity in the training data can lead to models that perform well on familiar image types, but struggle with unique or complex historical photographs. Therefore, diverse and extensive training data is essential. Researchers are exploring the concept of dynamic edge preservation, where CNNs can adapt in real-time to the specific qualities of images being restored. This adaptability is particularly valuable for applications involving live feeds or interactive historical image displays, enabling more engaging ways to connect with historical artifacts.
Upscale any video of any resolution to 4K with AI. (Get started for free)
More Posts from ai-videoupscale.com: