Maximizing Color Information Retention A Guide to High-Fidelity Video Export in 2024
Maximizing Color Information Retention A Guide to High-Fidelity Video Export in 2024 - Understanding Color Spaces for Enhanced Video Export
The way a device handles color, its ability to capture, display, and reproduce it, is governed by a concept called a color space. These spaces are essentially mathematical definitions outlining the boundaries of color a system can work with. Video production utilizes various color models, such as the common RGB and different YUV formats (like YUV 444, 422, and 420). Choosing the right color space is paramount for maintaining color accuracy across every stage of a video's life – from capture to export and eventual display.
In professional video workflows, color spaces like sRGB, Adobe RGB, DCI-P3, and Rec.2020 are frequently used, each tailored for specific needs and representing a unique range of colors. Understanding the nuances of a color space goes beyond just a label. It involves grasping the interplay of its components, such as the way its primaries are defined, its gamma curve (which impacts the relationship between light intensity and color), and the color of its designated white point.
Software solutions like DaVinci Resolve provide color grading tools, allowing for intricate manipulation and a higher level of control over color appearance in the final export. But it's crucial to acknowledge the limitations inherent in each color space and device. An awareness of these limitations is necessary when aiming for maximum color accuracy in high-quality video exports. This is achieved through effective color management techniques that can bridge the gaps between different formats and devices, helping to minimize color information loss and preserving a richer visual experience.
Color spaces are essentially mathematical definitions of the range of colors a device can handle – capture, display, or reproduce. They are fundamental to understanding how video data is represented and processed. We commonly encounter RGB, YUV 444, YUV 422, and YUV 420 in video workflows. The selection of the right color space is critical for retaining color accuracy throughout the entire process, from initial capture to final output.
Within digital filmmaking, we see color spaces like sRGB, Adobe RGB, DCI-P3, and the increasingly important Rec. 2020. Each has its own unique color range and specific use cases. Understanding the nuances of each is vital for matching the desired output with the appropriate space. A related concept is a color gamut, which refers to the specific range of colors a device can accurately reproduce within a defined color space.
When delving into a particular color space, we need to pay attention to its RGB chromaticities, how it handles color components (often referred to as the gamma transfer function), and the white point definition. Software like DaVinci Resolve includes robust color management tools that empower us to finely tune how colors are represented in the final export, which helps maximize our creative potential.
Understanding color spaces involves a deep understanding of color theory in general. Color primaries, transfer functions, and how the system as a whole manages the colors are key parts of the puzzle. Color management workflows themselves are designed to ensure that colors are reproduced consistently across different file formats and devices, which is increasingly important with a wider array of platforms to consider. Failing to account for the limitations of each color space, including its range, can easily lead to loss of information and compromised visual fidelity during export. This is something to keep in mind, especially when producing high-fidelity video that requires the most accurate color representation possible.
Maximizing Color Information Retention A Guide to High-Fidelity Video Export in 2024 - Leveraging HDR and Wide Color Gamut Technologies
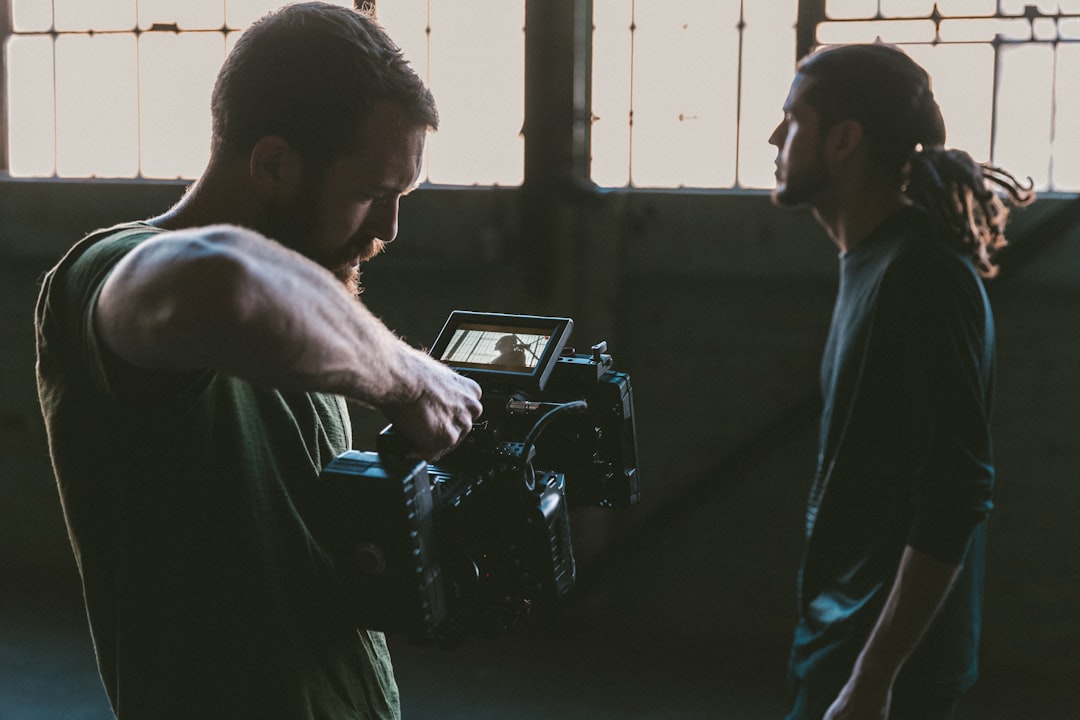
HDR and Wide Color Gamut (WCG) technologies are revolutionizing how we experience video by greatly expanding the range of colors and brightness that can be displayed. With HDR, we gain a much wider contrast range, allowing for a more realistic representation of bright and dark areas within a scene. WCG, working hand-in-hand with HDR, broadens the spectrum of colors that can be accurately reproduced, producing a more vibrant and immersive viewing experience. However, maximizing these benefits hinges on using these technologies correctly throughout the entire video production pipeline. Choosing the right color spaces, paying close attention to the specific needs of the intended display, and having the display correctly calibrated are all crucial for preventing a loss of the rich color information that HDR and WCG offer.
The landscape of display technology is in constant flux, with new advances in HDR and WCG constantly emerging. This evolution creates exciting possibilities for richer and more immersive video content. But, understanding how these technologies work, their limitations, and the best ways to incorporate them into our workflows is vital. As the industry increasingly adopts ultra-high-definition video, these technologies are becoming increasingly crucial for achieving truly high-quality video experiences, both for professional productions and for personal viewing. Without a clear understanding of their applications, we risk losing much of their potential, hindering the realization of a truly high-fidelity visual experience.
Over the last couple of decades, display technology has dramatically improved, not only in resolution but also in its ability to handle a broader range of brightness and color. High Dynamic Range (HDR) fundamentally changes how brightness is represented in video, enabling a much greater contrast between the darkest and brightest parts of an image. This opens the door for a much more realistic visual experience, especially when considering how our eyes perceive light in the real world.
Along with HDR, we've seen significant developments in Wide Color Gamut (WCG) technologies. Color spaces like DCI-P3 and Rec. 2020 can cover a far wider range of colors than the traditional sRGB space we're accustomed to. Some estimates suggest these newer color spaces can encompass as much as 25% more of the visible spectrum. This expanded palette is a key ingredient in creating a more visually engaging and accurate high-fidelity video.
The human visual system is much more sensitive to subtle differences in both color and brightness when viewing HDR content compared to standard dynamic range (SDR). This increased sensitivity is what allows HDR to deliver so much detail in both shadows and highlights, making those details more perceptible and visually impactful. A 10-bit or even higher color depth is often employed with HDR, which offers billions of color variations per frame. This is a big improvement over the 16.7 million colors of 8-bit content, which can sometimes lead to visible banding in smooth color transitions.
Of course, taking advantage of these features requires appropriate displays. Many existing screens haven't been designed to accommodate the expanded dynamic range and broader color gamuts of HDR and WCG. This means that creators need to be very mindful about the formats they are delivering their content in, as a mismatch can lead to significant quality loss when playing back the video.
HDR can complicate color grading as well. Working with the expanded dynamic range requires different tools and techniques to ensure the final output is visually appealing and true to the content creator's intent. The way metadata is used in the various HDR formats (like HDR10, Dolby Vision, and HLG) is an added layer of complexity, influencing how the color information is presented based on the capabilities of the display device.
The adoption of HDR and WCG means we're using different sets of chromaticities in our display technologies. For instance, Rec.2020 uses distinct chromaticities for red, green, and blue, compared to older standards. How these changes affect the overall color perception throughout the post-production process is something to watch.
There's an inseparable connection between HDR and WCG. HDR addresses the luminance range while WCG focuses on the color range. It's crucial that both are optimized during the video export process. Failing to do so can lead to situations where one aspect detracts from the other, impacting the overall fidelity of the video.
We are seeing a strong push in professional workflows to ensure content is mastered for both HDR and wide color gamuts. It's become increasingly important to ensure the video looks consistent across all playback devices, which can be a challenge with the varied capabilities of modern displays. It’s a reminder of the ongoing complexities in the color management process, particularly in the context of high-fidelity video export.
Maximizing Color Information Retention A Guide to High-Fidelity Video Export in 2024 - Implementing Advanced Video Coding Standards like HEVC
Implementing advanced video coding standards like HEVC (High Efficiency Video Coding), also known as H.265, offers a significant leap in video compression efficiency compared to older standards. This improved efficiency is especially important in the age of HDR and WCG content, where maintaining high visual quality becomes even more critical due to the greater amount of color and brightness information. HEVC's ability to handle these expanded color spaces and dynamic ranges allows for more vibrant and realistic video experiences.
Specifically, the Main 10 profile of HEVC employs 10-bit color depth and 4:2:0 chroma subsampling, which is beneficial for retaining color fidelity when working with HDR content. This enhanced color handling contributes to better quality at lower bitrates, an essential consideration for various online platforms and content distribution methods.
However, realizing the full potential of HEVC in maximizing color information retention requires a deeper understanding of its features and proper implementation. It's not a simple switch—creators must be aware of the encoding techniques and workflow nuances involved to ensure the best results. The interoperability of hardware and software, specifically compatible display technologies, is also vital to avoiding situations where the rich color information produced by HEVC gets lost during playback.
The continued evolution of video coding standards, with concepts like Next Generation Video Coding (NGVC) on the horizon, highlights the need for creators to keep abreast of the latest advancements. It’s a constant process of adaptation, particularly with the goal of achieving truly high-fidelity video, where color accuracy and visual detail are paramount.
Implementing advanced video coding standards like HEVC, also known as H.265, presents intriguing possibilities for compressing video while retaining high visual quality. HEVC's compression efficiency is roughly twice that of its predecessor, AVC, which translates to significantly smaller file sizes for comparable video quality. This is especially useful when dealing with high-resolution videos, where storage and bandwidth are often limited. However, there are some tradeoffs to consider.
HEVC's approach to chrominance and luminance encoding through subsampling can sometimes introduce color artifacts, particularly in intricate scenes. While the method can be very efficient, improper settings during encoding can lead to less-than-ideal results. Thankfully, the Main 10 profile of HEVC tackles this issue using a 10-bit per sample format which helps to maintain a broader range of color information. This increased color depth is essential for HDR content and ensures smoother transitions in gradations of light and shadow.
Another aspect of HEVC is its reliance on a perceptual-based encoding technique. This prioritizes the elements that are most perceptible to the human eye for encoding but can cause inconsistencies when not properly optimized for specific content. While this makes the encoding process more efficient, there's a risk that important information in less noticeable areas might get overlooked, leading to minor degradation in overall fidelity. This kind of compromise seems to be inherent in any lossy compression method.
HEVC's B-frame improvements also contribute to the higher compression ratios it can achieve. However, excessive use of B-frames can introduce latency during real-time editing or playback which could hinder live streaming or interactive applications.
HEVC's increased complexity adds to the computational burden of encoding and decoding. It demands more powerful hardware, which can be a major hurdle in less-advanced workflows. This increased complexity also influences color grading during post-production. Colorists need a nuanced understanding of how HEVC handles the compressed color information to avoid getting unexpected outcomes.
The growing adoption of wider color gamuts like Rec.2020 introduces challenges when working with HEVC. Managing the primary colors with broader color spaces becomes more critical, or there is the risk of losing the advantages of wider gamuts during the encoding process.
Despite its computational intensity, HEVC is well-suited for adaptive streaming applications. By adjusting video quality based on varying network conditions, it can ensure a smoother and more consistent viewing experience, regardless of bandwidth fluctuations.
It’s clear that HEVC brings significant benefits to video compression, but there are definite technical challenges and compromises involved. The future of video encoding seems to lean towards even more efficient compression methods referred to as Next Generation Video Coding (NGVC). As these new standards emerge, we'll likely see a continued push toward optimizing compression while maintaining visual quality. Research in this area will likely investigate new approaches to color handling and perceptual encoding methods, potentially leading to more optimal video compression workflows. The research into optimal video compression methods seems very active based on the amount of work published so far related to HEVC. In the meantime, a good understanding of HEVC's capabilities and limitations can help content creators make the best decisions for their projects in 2024.
Maximizing Color Information Retention A Guide to High-Fidelity Video Export in 2024 - Utilizing Confidence-Based Feature Reconstruction Methods
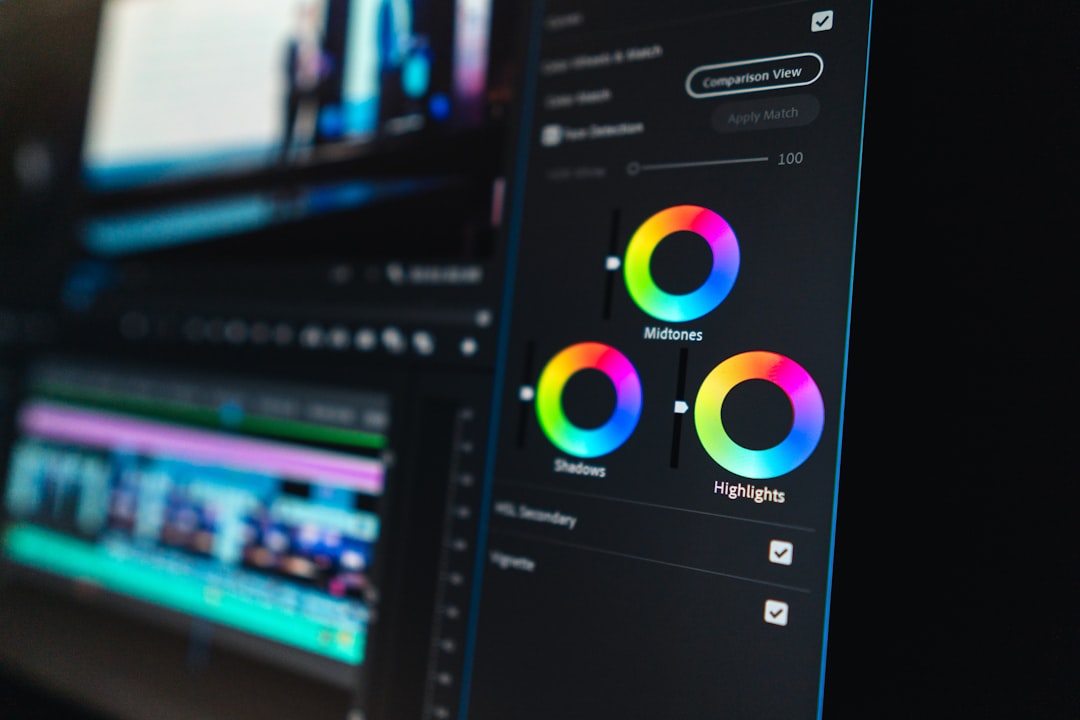
In the realm of video processing, especially within the context of compression, techniques that prioritize perceptual quality are becoming increasingly important. Confidence-based feature reconstruction methods address this need by enhancing the quality of newly appearing parts of a video during compression. These techniques are a response to the growing trend towards advanced, learned video compression that typically emphasizes objective metrics like PSNR. However, achieving a great viewing experience demands attention to how humans perceive quality, not just raw data quality metrics. By focusing on areas where there's uncertainty or change, confidence-based reconstruction methods can improve the efficiency of the compression process while producing more visually accurate results. As video production continues to move towards wider color gamuts and higher dynamic ranges, these advancements become even more crucial. This is because the goal of maintaining accurate and rich color information from source to output becomes a more difficult challenge. Methods like confidence-based reconstruction show a pathway beyond traditional video compression methods, helping to create workflows that simultaneously offer improvements in video quality and compression efficiency, a critical need for today's video content creators.
Okay, let's explore the idea of utilizing confidence-based feature reconstruction methods in the context of high-fidelity video export.
Essentially, confidence-based feature reconstruction (CBFR) relies on statistical approaches to intelligently fill in missing or degraded data in a video. It's like having a system that can "guess" what a missing piece of the picture should look like based on what's around it and how confident it is in those surrounding pixels. This approach can be incredibly useful in improving the quality of videos, particularly when dealing with lower-resolution content or situations where compression has introduced artifacts.
CBFR often works across both spatial and frequency domains. This dual approach allows it to more effectively capture and restore important color information during the reconstruction process. By focusing on essential visual details and reducing noise, it's been shown to help with the tricky issue of compression artifacts—those distortions that can really harm the perceived quality of color in compressed video files, particularly when utilizing advanced techniques like HEVC.
One of the really cool aspects of CBFR is its adaptability. It can be tweaked and customized to suit specific video types, which means it can handle different kinds of video content in the most appropriate way, leading to more consistent preservation of color detail.
CBFR algorithms can also use predictive modeling to infer the colors of missing pixels. This is done by examining the colors of surrounding pixels. This type of "intelligent interpolation" helps maintain the continuity of colors and textures throughout the video.
This gets particularly interesting in the video context because the algorithms can incorporate the concept of temporal coherence. This basically means it can learn from previous frames in the video to maintain color consistency over time. That's really important for maintaining the perceived quality of a video and ensuring the final export is as high-fidelity as possible.
We're also seeing CBFR being explored in real-time video applications thanks to increasing computing power. This could open the door to on-the-fly color enhancements for live broadcasts or streaming, which is very exciting from a research perspective.
A nice feature of CBFR is that its implementation can be standardized, making it potentially efficient on a wide range of video processing platforms. Regardless of the specific hardware or software used, the method could ensure that the color reconstruction is as efficient and effective as possible.
Modern machine learning approaches are being integrated into CBFR, allowing the creation of even more adaptive models that can learn color relationships from massive datasets. This has the potential to further improve the fidelity of reconstructed videos.
But, it's worth mentioning that the usefulness of CBFR will ultimately depend on the starting quality of the video. If the initial video content is already too heavily degraded, even the best reconstruction methods might not be able to fully restore color information to an acceptable standard without significant external intervention. This is something we'll need to be mindful of moving forward as we consider incorporating CBFR into our video pipelines.
Maximizing Color Information Retention A Guide to High-Fidelity Video Export in 2024 - Optimizing Video Processing for Telepresence Systems
Telepresence systems are pushing the boundaries of virtual communication, striving to deliver more immersive and engaging experiences. Recent advancements, exemplified by systems like Project Starline, highlight the potential of technologies such as 3D capture, real-time neural view synthesis, and spatial audio to drastically improve the feeling of being present in a remote location. These systems stand in sharp contrast to traditional 2D video conferencing, aiming to create a more realistic and natural interaction.
Successfully implementing these technologies requires a careful balance of innovation and established video processing principles. For example, the need for low latency and smooth interactions necessitate the effective use of multistream compression methods to efficiently manage video data while preserving visual quality, particularly color information. However, advanced features come at a cost, often requiring significant processing power and bandwidth. This necessitates a deep understanding of how to leverage video coding standards and optimize bandwidth usage to ensure telepresence systems perform optimally without sacrificing the fidelity of the video stream.
As telepresence technology advances, it will become increasingly important to bridge the gap between cutting-edge innovations and the core principles of efficient video processing. Only then can we truly achieve high-fidelity virtual experiences that foster a sense of genuine presence and enhance remote communication.
Telepresence systems are pushing the boundaries of video communication, demanding efficient and optimized video processing. The sheer volume of data involved, particularly with the increasing use of high-resolution 4K video, puts a strain on both hardware and software. Maintaining a smooth and responsive experience requires clever optimizations to reduce processing demands and ensure the system can decode and render multiple video streams in real time. We're increasingly seeing the use of specialized hardware to handle these high processing loads.
The impact of latency in these systems is crucial. Even minor delays can disrupt the flow of a conversation and diminish the sense of being present in the same space. Researchers are working to optimize video processing algorithms to minimize latency, thereby improving the natural back-and-forth of conversations. This can be a difficult engineering challenge due to network variability and other factors.
Bandwidth availability is a significant hurdle for telepresence, especially as video resolution and fidelity increase. Systems like Project Starline use multistream compression to improve video quality while dealing with bandwidth limitations. This approach to bandwidth management relies on adaptive bitrate streaming, which intelligently adapts the quality of the video in real time based on the available network connection. The goal is to minimize interruptions and maintain a constant flow of video, which is important in preserving the sense of a live interaction.
Network disruptions, like packet loss, are inevitable, especially when dealing with high-bandwidth streams. Systems are employing techniques like forward error correction (FEC) to address packet loss. FEC works by adding extra redundancy to the video stream. If parts of the stream are lost during transmission, FEC allows the receiver to reconstruct the lost packets without needing to request retransmission, minimizing any noticeable degradation in video quality.
It's becoming increasingly clear that understanding how people perceive video quality is a key part of optimizing these systems. Visual perception is not uniform, and some parts of a video stream might be more important than others for maintaining the sense of realism. We are seeing an increase in encoding strategies that focus on the elements people are most likely to notice during video. By prioritizing the encoding of these key elements, telepresence systems can provide a more satisfying experience, even under bandwidth constraints.
With recent advancements in AI and computer vision, video processing techniques like semantic segmentation are emerging as powerful tools for telepresence. Semantic segmentation can identify different objects or features within the video stream, such as faces, body language, or objects within a room. By recognizing and prioritizing the most important objects, systems can optimize encoding, allowing for a higher-quality representation of the most critical elements while reducing the data demands for the rest.
Color plays an important role in the perceived quality of video, and variations in lighting can often cause issues in these systems. To overcome this, we are seeing telepresence systems incorporate real-time color correction algorithms that can dynamically adjust brightness, contrast, and color balance. These adaptations help to minimize variations due to lighting conditions and maintain more consistent and accurate color rendition across a variety of environments.
The role of machine learning is increasingly important in optimizing the performance of telepresence systems. AI algorithms can analyze massive amounts of video data to identify patterns and develop strategies for reducing bandwidth use while preserving visual quality. These algorithms can also adapt to user behavior, tailoring the video stream and adjusting encoding parameters dynamically to ensure a smooth experience. It's likely we'll see a significant increase in this type of AI optimization in the coming years.
We are seeing a major shift towards more efficient video compression standards like HEVC and AV1. These new codecs offer better compression ratios, enabling higher-quality video streaming with less bandwidth. This is crucial for telepresence, as it can help improve the accessibility and overall performance of these systems across a broader range of network conditions. This trend reflects a steady increase in demand for higher-quality video and an effort to make telepresence accessible on a greater number of devices.
Beyond video processing, the optimization of 3D spatial audio is also a critical part of enhancing the sense of presence in a telepresence system. These advancements rely on synchronization between audio and video streams to create a more realistic experience for the user. The development of 3D audio creates a more compelling sense of "being there," alongside video processing optimizations, and provides a more natural interaction experience.
Maximizing Color Information Retention A Guide to High-Fidelity Video Export in 2024 - Balancing Performance and Graphics in Video Rendering Pipelines
The efficiency of video rendering hinges on a delicate equilibrium between performance and graphical fidelity. This balance relies on the collaborative work of the CPU and GPU, each contributing distinct roles to the rendering process. While GPUs traditionally handle graphics rendering, optimizing the CPU for data preparation and command issuance is equally important to ensure smooth, high-speed rendering. The nature of rendering operations themselves can impact performance, as color blending operations can vary wildly in speed depending on factors such as the number of texture combiners utilized.
Software solutions are continuously evolving to mirror or even exceed the rendering speeds seen in dedicated hardware. Custom-designed rendering pipelines, offered through frameworks like Unity's Scriptable Render Pipeline, offer a way to tailor graphics rendering to specific projects, further pushing the boundaries of performance. But selecting the right rendering path for your project is critical as it directly impacts how lighting and shading calculations are performed. This can create tradeoffs as you fine-tune the balance between a fast, lightweight path that sacrifices accuracy and more detailed paths that increase rendering times.
Improvements in graphics pipeline architecture are continuously improving speed, scalability, and robustness. As a result, we see better color information retention and, generally, improved visual fidelity in the end result. The push for increasingly detailed content, particularly with HDR and Wide Color Gamut formats, has spurred significant advances. These innovations demand more sophisticated rendering techniques to handle the greater detail present in these more modern formats. Understanding how to align these performance optimizations with the demands of high-fidelity exports is becoming more crucial as content creators strive to ensure the retention of color information and the overall visual quality of their projects.
The core of a video rendering pipeline involves the interplay between the CPU, which prepares data and issues commands, and the GPU, which executes those commands for visual output. While GPU optimization often takes center stage for graphical tasks, optimizing the CPU is also crucial for overall application speed. Interestingly, the efficiency of color blending during rendering can vary significantly. Simple texture combinations might be processed quickly using just a couple of instructions, whereas more complex blends can lead to notable performance drops.
Remarkably, software-based graphics pipelines have matured to the point where their rendering speeds can rival those of dedicated hardware. This has led to the development of advanced features in game engines like Unity. The High Definition Render Pipeline (HDRP) within Unity aims for high-fidelity visuals, especially on powerful PCs and consoles. Moreover, the Scriptable Render Pipeline (SRP) allows for creating custom rendering solutions tailored to specific project requirements, offering developers more flexibility in optimizing graphical performance.
How lighting and shading are handled can vary based on the specific rendering path selected within a pipeline. Choosing the right path is paramount for achieving performance goals on the intended platform. Research from NVIDIA, for instance, emphasizes the importance of striking a balance between rendering capabilities and visual quality for optimal performance in video rendering.
The conversion of scene data into visuals on-screen is a multi-stage process within the graphics pipeline. Each step is a potential bottleneck if not carefully optimized, especially in real-time applications. It's a constant reminder that efficient processing is essential for maintaining smooth, high-quality graphics.
Overall, advances in graphics pipeline architectures have led to improvements in both performance and stability, leading to better color retention and a richer overall visual experience. These improvements have implications for video export quality and are especially relevant as HDR, wide color gamuts, and high color depth become more commonplace. The interplay of performance and color fidelity is a continuing research topic as computational power and display technology both advance, pushing the boundaries of what we consider visually compelling video.
More Posts from ai-videoupscale.com: