Upscale any video of any resolution to 4K with AI. (Get started for free)
Numbers for Mac 132 Key Features and Compatibility with AI Video Upscaling Workflows
Numbers for Mac 132 Key Features and Compatibility with AI Video Upscaling Workflows - Spreadsheet Creation and Data Analysis Capabilities
Apple Numbers has significantly improved its spreadsheet creation and data analysis tools, making it a viable option for users of all skill levels. It's capable of complex calculations thanks to functions like XLOOKUP and regular expressions, and it offers a wide range of data analysis features, plus customizable templates for streamlining data management. Users can share spreadsheets seamlessly using iCloud Drive folders, promoting collaboration. Data visualization has also seen enhancements, including various chart types and the interesting feature of integrating audio descriptions through VoiceOver on Apple devices. These unique features might, however, present a learning curve for users accustomed to more established spreadsheet software. Despite this, Numbers continues to offer a compelling combination of functionality and a visually pleasing interface, making it a noteworthy alternative for spreadsheet needs.
Apple Numbers provides a surprisingly extensive set of tools for both basic and intricate spreadsheet tasks. It boasts a library of functions, including some less common ones like XLOOKUP and regular expression support, offering a degree of flexibility I found initially unexpected. While the chart creation options aren't revolutionary, they do offer a standard set of visuals, including column and donut charts, which can be useful for quick data visualization. It's worth noting that the audio integration feature through VoiceOver, while intriguing, seems mostly geared towards accessibility rather than data analysis itself.
The iCloud Drive integration for collaborative editing is a strong point, allowing seamless real-time collaboration on spreadsheets. However, it is still worth assessing the limitations when compared to more enterprise-grade platforms, especially if the user base will cross Apple device types. It's interesting how Numbers blends traditional spreadsheet capabilities with a focus on aspects that I think some spreadsheet programs have neglected. For instance, the built-in audio recording feature might be of limited interest to a majority of users, but for those seeking a way to add audio annotations to spreadsheets, this can be very novel. Similarly, the feature linking captions and titles to objects (like tables or videos) can contribute to smoother presentation, but it might be overlooked unless explicitly required.
I found the connection to AI spreadsheet assistants promising, though this needs more exploration to assess the true usefulness in specific workflows. The functionality appears broadly similar to that of Excel in its core, which should feel familiar to many users. What is unique about Numbers in my mind is its emphasis on visual presentation, making even complex spreadsheets appear polished and professional. Overall, while it may not have some of the more advanced or enterprise-focused features found in professional solutions, Numbers offers a strong combination of flexibility, user-friendliness, and a visual polish that can enhance the entire spreadsheet creation process. That combination can be especially valuable when the users are also creating presentation content for public or internal consumption.
Numbers for Mac 132 Key Features and Compatibility with AI Video Upscaling Workflows - Integration with AI Video Upscaling Tools
The landscape of video editing and content creation is rapidly changing with the integration of AI-powered video upscaling tools. These tools, which can significantly improve video quality by upscaling lower-resolution footage to higher resolutions like 4K or even 8K, are becoming increasingly common. Services like TensorPix and tools like VideoProc Converter AI demonstrate the power of these AI-driven solutions, offering features such as GPU acceleration for faster processing on various hardware. Moreover, the increasing availability of these tools, including options like HitPaw and AVCLabs, allows both experienced professionals and novices to access and leverage these capabilities. This accessibility, however, raises new considerations for users. It becomes important to think about how these AI-powered tools seamlessly integrate with existing software and workflows, like those associated with Numbers for Mac, while ensuring that the user interface and control are intuitive. The potential for enhanced visuals is undeniable, but careful consideration of the learning curves and integration processes is crucial for effective implementation into existing practices. Ultimately, the integration of AI video upscaling can elevate video quality, but it's important to recognize the associated complexities that users may face as they adapt their workflows to these advancements.
The potential synergy between Numbers and AI video upscaling tools is intriguing. Many AI upscaling tools employ sophisticated convolutional neural networks (CNNs), leading to remarkable quality improvements without excessively impacting processing time. This efficiency complements Numbers' focus on managing large datasets effectively.
One notable feature is the ability of these tools to reconstruct fine details and edges lost in lower-resolution videos. This detail preservation could be leveraged by Numbers' charting capabilities to generate richer, more informative visual representations of data.
Imagine directly exporting data from Numbers as higher-resolution images using AI upscaling, resulting in clearer charts and graphs for presentations. Furthermore, many upscalers support batch processing, potentially significantly streamlining workflows when dealing with large datasets within Numbers.
Some tools even allow for customizable upscaling parameters, like controlling noise reduction or detail levels, which could be an interesting parallel to the user-defined functions available in Numbers. Maintaining accurate colors is important for effective data visualization, and AI upscaling techniques are improving color fidelity in visual outputs, a benefit Numbers could utilize for improved charts and graphs.
The core strength of these AI tools often comes from their reliance on machine learning models trained on vast image datasets, potentially resulting in better output than simpler scaling methods found in other software. These upscalers are typically adaptable across various video formats, retaining quality regardless of resolution. This could help optimize workflows needing data presentations in multiple formats when used in conjunction with Numbers.
While some upscaling tools offer real-time processing, potentially enabling immediate feedback within a Numbers workflow, the interoperability of these AI tools with other software and platforms is also promising. Integrating with tools outside of Numbers could create more holistic workflows for creative and analytical tasks, but the extent of actual benefit for most use cases remains to be seen. Overall, it seems that if AI upscaling tools were tightly integrated with Numbers' features, there's a chance it could benefit from a quality boost, which might be worth investigating in further detail, especially for users creating presentations and reports with a high reliance on data visualizations.
This intersection of tools presents a promising direction for future development. As AI upscaling continues to evolve, exploring these potential integrations with Numbers might uncover novel ways to improve data visualization and enhance the overall user experience. However, there are currently limited options for direct integration or even a clear roadmap for it, leaving any immediate benefit unclear.
Numbers for Mac 132 Key Features and Compatibility with AI Video Upscaling Workflows - Selective Video Enhancement Options
The ability to selectively enhance parts of a video is a growing trend in video editing. Instead of applying the same improvements across an entire video, users can now focus on specific areas. This is valuable when you want to improve the quality of certain scenes, faces, or objects without affecting the rest of the video, helping to create a more polished and intentional final product. AI-powered video upscaling tools, such as AVCLabs Video Enhancer AI and Topaz Video Enhance AI, often provide these selective enhancements. Features like noise reduction, facial recognition, and motion tracking can refine a video in specific ways. However, it's worth noting that many of these advanced features often come with a learning curve. Integrating these tools smoothly into existing workflows, especially those involving software like Numbers for Mac, can also be challenging. The goal is to make selective enhancements both powerful and easy to use, but it's an area where more intuitive interfaces and better compatibility could be greatly beneficial.
AI-powered video enhancement tools offer increasingly sophisticated ways to refine video quality, going beyond uniform adjustments. We can now selectively enhance certain portions of a video, treating the foreground and background differently. This granular control potentially enhances presentation quality when using Numbers by enabling creators to focus improvements on areas critical to data representation, leading to a more polished appearance.
Many of these AI upscaling tools leverage optical flow algorithms to intelligently analyze frame-to-frame motion within a video. This is particularly helpful for videos with significant movement, as it produces smoother and more natural transitions. The improved visual fluidity could be a valuable addition to Numbers-based presentations that involve dynamic data visualizations.
Some of the most advanced AI-upscaling techniques make use of generative adversarial networks (GANs). GANs essentially learn to generate realistic video textures and details, effectively "filling in the gaps" in low-resolution footage to bring it closer to the visual fidelity of higher resolution content. This ability has the potential to transform the way we experience data visualizations in Numbers, especially when they are used in conjunction with motion graphics and dynamic charts.
We also find selective sharpening options within some of these tools, which could be useful for focusing attention on specific sections of video during a Numbers-based presentation. The ability to selectively highlight certain details in a presentation can greatly assist in communicating insights effectively. It would be interesting to see how this can help emphasize key data points or highlight interesting trends.
Intriguingly, some tools provide real-time synchronization with enhancements. This dynamic adaptation of video quality means that as a Numbers presentation unfolds, the video's visual elements can adjust automatically. This feature, if seamlessly integrated with Numbers, could improve the experience of live presentations. Imagine a chart or graph in the presentation being linked with dynamic footage, changing in tandem with the spoken narrative.
Certain tools offer real-time video enhancement, making the process of improving video quality more intuitive. Imagine making changes on-the-fly during a presentation, adding a layer of visual responsiveness that can add a unique dimension to the storytelling aspect. This also introduces the potential to address issues in visuals with a speed that can be vital in a live setting.
Furthermore, these tools are equipped with advanced color correction features. These features can refine the color accuracy within a video, which is especially relevant for data visualization in Numbers. If properly integrated, this could ensure that color-coded charts and graphs from Numbers maintain their intended visual impact throughout a presentation.
Targeted enhancement capabilities allow users to emphasize areas of interest within a video clip. By using these controls, presentations created using Numbers could dynamically shift the focus of a viewer's attention. This might improve the ease with which the audience follows the narrative within the presentation.
Selective video enhancement could also be a tool for optimizing file sizes. By employing intelligent compression techniques, it could be possible to preserve video quality while reducing file size. This could be very useful when presenting or sharing large video files within a data visualization project generated in Numbers, particularly in environments with limitations on network bandwidth.
The machine learning models used within selective video enhancements continually learn and adapt over time. This is particularly useful because as users refine their workflows, their specific preference can start to guide the outcome, leading to more streamlined video refinement for future projects. These learned outcomes can translate to more polished Numbers-based presentations as the user gains familiarity with their workflow, leading to less editing time over time.
It's still very early days for these selective enhancement techniques. There's a considerable potential here. If seamlessly incorporated into the workflows of applications like Numbers, the ability to target specific areas for video refinement could be a game changer for data visualization. As these technologies evolve, it's exciting to think about how they can enhance our ability to communicate insights through data. However, it is important to remain critically aware of any downsides. We must also remain mindful of the need for easy integration and effective controls for end users if the benefits are to truly be realized.
Numbers for Mac 132 Key Features and Compatibility with AI Video Upscaling Workflows - Performance Optimization for Apple Silicon Macs
Apple Silicon Macs, introduced with the M1 chip and followed by the M2, M3, and others, have significantly boosted performance and energy efficiency. This is largely due to the chip's unified architecture, featuring both performance and efficiency cores. This approach allows for faster processing speeds while also extending battery life. macOS and native applications, like GarageBand and Logic Pro, are built to capitalize on this architecture, resulting in notable performance improvements, especially in demanding tasks. For example, Final Cut Pro sees substantial speed increases when running on Apple Silicon. However, this optimized performance comes with a caveat. Developers need to individually test and tailor their applications to Apple Silicon since the architecture differs from Intel-based Macs. This means that software optimized for Intel might not run as efficiently on Apple Silicon, impacting the overall performance. It's a trade-off to consider when evaluating the suitability of Apple Silicon for various workloads.
Apple's shift to Apple Silicon, spearheaded by the M1 and later chips like the M2, has brought about significant improvements for Macs, particularly in terms of performance. This is achieved through a unified architecture that combines the CPU, GPU, and a Neural Engine onto a single chip. This design reduces the usual bottlenecks in communication between different components, which in turn contributes to quicker data processing and more fluid visual outputs within Numbers.
Besides performance improvements, the shift also has a considerable impact on power usage. Apple Silicon-based Macs can execute tasks demanding heavy processing at a significantly lower power consumption than similar Intel-based Macs, leading to extended battery life. This can be advantageous for researchers and professionals who frequently work on the go.
Apple has engineered macOS and applications like Numbers to fully exploit the Apple Silicon architecture, making the most of the ARM instruction set. This creates a tight link between the software and hardware, which results in faster computations and sharper data visualizations within spreadsheets compared to Intel-based systems. When working with larger datasets, you can notice a definite speed improvement.
The integration of the Metal framework with Apple Silicon allows Numbers to render graphics more quickly, producing visual representations like charts with less delay. This is noticeable when generating detailed or complex visualizations within a Numbers document.
Apple Silicon also offers a built-in Neural Engine dedicated to machine learning. This can lead to enhancements in Numbers' capabilities such as data analysis and insights generation. How effectively it can improve trend predictions and automate insights remains to be seen, but the tight integration with hardware allows for quick execution of these more sophisticated functions.
The shift to unified memory creates high memory bandwidth for the chip. This bandwidth allows for more efficient data management within Numbers, leading to quicker access to data points in a spreadsheet and smoother responsiveness, particularly when working with large datasets or multiple applications concurrently.
Although Rosetta 2 enables Intel apps to run on Apple Silicon, the performance has room for improvement. Apple has been working to update apps like Numbers to natively support Apple Silicon, which usually leads to better stability and a more responsive experience.
Apple Silicon’s architecture offers impressive parallel processing capabilities, letting Numbers handle computationally complex formulas faster than sequential processing. This can yield tangible results when a spreadsheet has several formulas linked or performing intensive tasks.
The latest chip architecture presents enhanced virtualization abilities, potentially leading to smoother experiences when users run virtualized environments like Windows alongside Numbers. While still needing to see this play out with more real-world examples, this could be beneficial for users who need a flexible multi-platform workflow.
One of the compelling long-term aspects of Apple Silicon is its longer support lifecycle compared to the Intel-based Macs. Apple's focus on this new architecture suggests continued support and performance enhancements in future iterations. Users can expect improved performance over time, making this a promising investment compared to the older Intel chips.
While many of these advantages are quite beneficial, the development process for apps that are optimized for Apple Silicon are still evolving. It's crucial to realize that not all the apps have reached their potential, and the effects of each optimization can vary considerably. It's an exciting time to see the evolution of this shift, but the results are not yet universal. This means you might encounter apps that show inconsistent performance gains, requiring developers to refine and optimize their code further. Overall, however, the outlook for Apple Silicon is very positive. The underlying architecture improvements appear quite robust, and if further refined, should make a real positive impact for users of apps like Numbers. It is a trend worth following closely.
Numbers for Mac 132 Key Features and Compatibility with AI Video Upscaling Workflows - Batch Video Import Functionality
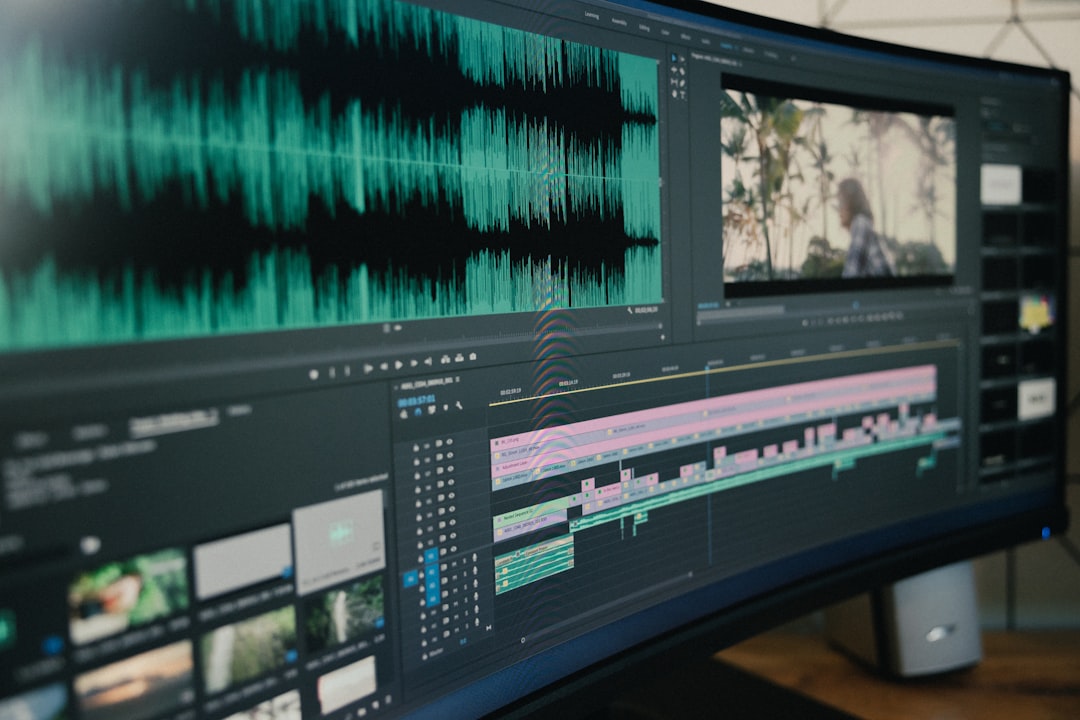
Numbers for Mac's integration with AI video upscaling workflows has taken a step forward with the introduction of batch video import functionality. This new feature lets users import multiple videos simultaneously, which can be a significant time-saver when working with a large number of video clips. The ability to import multiple videos at once is especially useful when working with AI video upscaling workflows. This is because it allows users to easily batch process a large number of videos and significantly speed up the entire process of improving video quality. The convenience of batch processing can be a game-changer for users managing many low-resolution videos, simplifying the organization and optimization of video assets.
However, the introduction of any new functionality can also bring complexities. It's important to note that integrating these tools smoothly into workflows that also involve other software, like Numbers for Mac, may require careful consideration and adjustments to how users currently work. It will be interesting to see how developers navigate this challenge. While batch import holds much promise, users will likely need to spend some time understanding how these workflows interact with existing habits, and that is not always a simple process. In the end, the overall impact of this functionality on how video assets are managed is significant, and if these challenges can be overcome, batch video import can truly improve video-related projects and workflows.
Numbers for Mac's ability to handle multiple video imports at once, often referred to as batch video import, is a hidden gem that's worth exploring further. It's intriguing how Numbers manages to bring in videos of various formats and seemingly converts them into a more spreadsheet-friendly format for use within charts or documents. This conversion step seems to tie into the spreadsheet's capabilities, creating a stronger connection between video and visual data.
One interesting aspect of this functionality is its built-in resolution management. I've noticed that when importing numerous videos, Numbers automatically scales them down if they exceed predefined system parameters. While this is understandable from a resource management perspective, it could be useful if users had more direct control over this behavior. It's also interesting to see Numbers automatically extract metadata from videos, such as frame rate, resolution, and duration. This automatic extraction is useful for creating informative tables and visual representations related to the videos.
Furthermore, this batch import process gets a significant boost on Apple Silicon Macs. The unified memory and the general performance improvements associated with the newer chips appear to translate into quicker uploads and faster rendering of video thumbnails during imports. The improvement here seems noteworthy, although it's unclear how much impact it truly has for various video formats and sizes.
One of the more compelling features related to batch import is the potential for tighter integration with data analysis within Numbers. Because it's possible to bring in a set of videos while also working on the numerical data within a spreadsheet, the potential arises to correlate them. This opens up a unique way to analyze and visualize data that relies on both numbers and visual elements.
Beyond direct interaction, Numbers also supports AppleScript for automation. This allows users to create custom scripts for specific batch imports, including criteria for file types and operations for video processing. It seems a bit unusual for a spreadsheet to offer such fine-grained control, but in this case, it does appear to be very effective for streamlining tasks. The capability to use APIs for batch processing within macOS is also intriguing, as it seems to open up the potential for higher-level functionality.
Once videos are in the spreadsheet, dynamic links can be created to connect video content and the other spreadsheet elements. It's interesting to think about how Numbers handles these updates, especially when modifications are made to the video files themselves. While not a core feature of spreadsheets, it could add a lot of value for presentations and visual storytelling using data.
From a collaboration point of view, the fact that batch video import and the associated links can be shared through Numbers' features makes it easier to manage and access video-related content for those working on projects together. It's noteworthy that Numbers offers this collaborative aspect beyond the typical spreadsheet features.
Finally, there's a lot of potential for integrating batch video import with analytical tools. It might be possible to apply statistical methods to analyze video-related metrics such as the average length of videos within a group or the occurrence of specific visual elements. These analytical insights could lead to unique discoveries from video data that would be hard to get from spreadsheets alone.
In conclusion, the batch video import functionality within Numbers for Mac offers some promising features, especially when combined with the recent improvements associated with Apple Silicon hardware. While the capability remains somewhat underutilized compared to features like charting and spreadsheet computations, the ability to incorporate videos into data analysis opens up potentially compelling avenues for the future of how data is represented and interpreted. However, we need to continue to investigate and see if this area will bear fruit.
Numbers for Mac 132 Key Features and Compatibility with AI Video Upscaling Workflows - Compatibility with Advanced AI Upscaling Algorithms
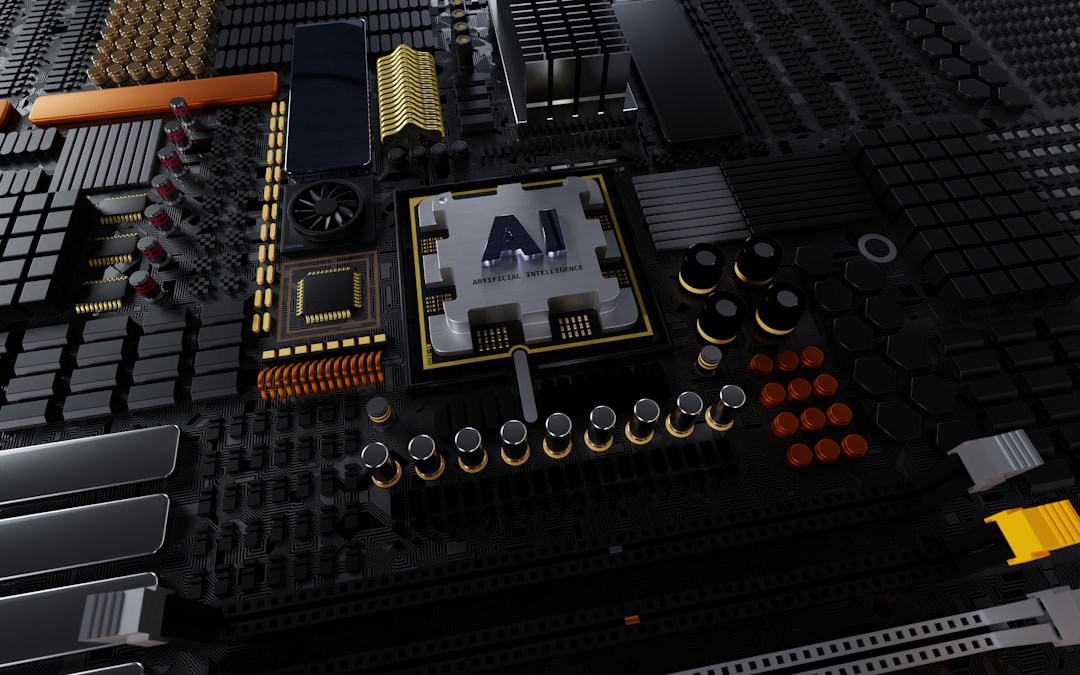
Compatibility with Advanced AI Upscaling Algorithms is becoming increasingly important as AI-powered video enhancements gain traction. These algorithms, often leveraging complex machine learning methods, can significantly improve the quality of lower-resolution video by enhancing details and clarity. However, the integration of such advanced AI upscaling features with tools like Numbers for Mac isn't always smooth. There can be a mismatch in how these algorithms work with the existing features within Numbers, causing issues with seamless integration. Even though some upscaling tools offer flexibility with settings and real-time adjustments, using them effectively might require users to adjust their existing workflows. This can present a challenge, particularly if the user aims to blend data visualizations with enhanced video within Numbers. There's clear potential for combining sharp data visualizations with higher quality video content within Numbers, but realizing that vision depends on whether the compatibility and usability hurdles can be overcome. It's an area that may need more attention in the future.
The realm of AI video upscaling is evolving rapidly, with advanced algorithms pushing the boundaries of what's possible in improving video quality. Many of these upscalers now rely on convolutional neural networks (CNNs), which are particularly adept at extracting key visual features from images. This allows them to reconstruct lost details with impressive precision, giving even older, lower-resolution videos a more modern look. It's fascinating to see how CNNs can make historical footage appear remarkably clear and detailed.
Some of the more advanced tools are now employing generative adversarial networks (GANs) for upscaling. These GANs are essentially able to predict and generate incredibly realistic textures, effectively filling in the gaps in lower-resolution content. When used within programs like Numbers, the visual quality boost is noticeable, particularly for charts and graphs that need to showcase visual details. The ability to predict visual detail is a step beyond just basic resolution enhancements and could prove to be very beneficial in specific use cases.
Maintaining the original color accuracy is crucial for effective data visualization. Thankfully, AI upscaling is becoming much better at preserving the color fidelity in videos. This is an important feature for applications like Numbers, where well-defined colors help users interpret complex data in charts and graphs. However, we still need to carefully watch these results to ensure that the color fidelity across multiple formats and resolutions remains accurate.
Another key advantage of these algorithms is their capability for batch processing, which is quite useful for those working with large numbers of videos. For example, if you need to enhance a collection of videos for a data presentation within Numbers, these algorithms can process them all efficiently, saving a significant amount of time compared to the older methods. It would be helpful to further investigate the various options for managing batches and determine how that process might be enhanced to better fit specific workflows.
Many upscaling algorithms utilize gradient-based optimization techniques to fine-tune the visual output. This offers a great deal of flexibility, allowing for real-time adjustments and refinements. You might notice this in the responsiveness of video features when used alongside Numbers presentations. It will be interesting to explore how we can leverage these real-time adjustments to ensure that the visuals stay consistent with the data.
AI upscaling algorithms are showing remarkable adaptability across diverse video formats. This is helpful because it reduces compatibility headaches for users who frequently work with multiple video types in their workflows. We should see this type of feature being increasingly adopted in both video editing tools and applications like Numbers to ensure consistent quality across various content types.
The incorporation of optical flow algorithms is a very interesting advancement in the field. These algorithms can detect and analyze motion within video frames, resulting in smoother video transitions and improved quality, especially when using dynamic data visualizations in Numbers. The ability to track and predict motion opens a path toward even more advanced capabilities in data visualization in the future.
Many of these tools offer clever resolution management. They dynamically adjust output resolution to optimize quality while conserving computational resources. For instance, in Numbers, this might mean that the software dynamically adapts video resolution to fit the current presentation settings, resulting in optimized visual displays. This type of intelligent scaling can reduce a considerable amount of user effort and improve overall performance, but we should be aware of possible bottlenecks.
A growing number of tools are introducing real-time synchronization features, ensuring that video quality stays aligned with a presentation as it unfolds. Imagine seamlessly integrating video content with a data visualization presentation in Numbers—the upscaled videos can adapt in real-time to enhance the viewing experience. This type of integration can make the entire presentation feel much more dynamic and engaging. While it is still early in the adoption of these real-time sync features, the potential for innovative use cases is remarkable.
Finally, the advancements in AI upscaling are influencing how people work collaboratively on video-based presentations. This means that teams of people are able to efficiently manage and edit video-based data within Numbers to achieve a desired visual output more efficiently. While the specific impacts still need to be seen across multiple workflows, the potential exists for more novel ways to communicate data in the future. The integration of AI into these collaborative workflows is worth investigating further.
In summary, AI video upscaling is rapidly improving, and its integration with applications like Numbers holds great potential for innovation. The algorithms are becoming increasingly sophisticated, offering a wider range of capabilities for those looking to create dynamic, engaging, and insightful presentations and reports. There are still some challenges to be addressed in terms of managing workflows, ensuring stability, and optimizing performance, but the outlook for this technology within the field of data visualization is positive. We can likely expect to see even more impactful applications of AI video upscaling in the years to come.
Upscale any video of any resolution to 4K with AI. (Get started for free)
More Posts from ai-videoupscale.com: