How Windows 10's Video Upscaling Compares to AI-Powered Solutions in 2024
How Windows 10's Video Upscaling Compares to AI-Powered Solutions in 2024 - Windows 10's Traditional Interpolation vs AI's Machine Learning Approach
Windows 10's built-in video upscaling uses a traditional approach called interpolation. It works by employing mathematical formulas to guess the values of missing pixels based on the pixels around them. However, this method often struggles to deliver the same level of quality seen in newer AI-based upscalers. AI-powered solutions, utilizing machine learning, take a different route. These programs learn from vast amounts of visual data, allowing them to understand complex patterns and recreate them with more accuracy when upscaling videos. This results in clearer images and finer details.
Even though Windows now includes the ability to incorporate custom AI models through Windows Machine Learning, the core difference remains. Traditional methods still lack the sophistication of advanced AI processing, especially in achieving crispness and detail. The landscape is rapidly changing though. Windows is moving towards greater AI integration with features like potential Neural Processing Unit support. This future direction, along with the consistent improvements seen in AI upscaling, is solidifying AI's position as the preferred choice for professionals and anyone wanting the highest possible quality.
Windows 10's video upscaling relies on established interpolation methods. These techniques, like bilinear and bicubic, use mathematical calculations to guess the values of new pixels based on nearby ones. While this approach is straightforward, it can create visible artifacts, especially in scenes with rapid movement.
AI-powered upscaling solutions, often using machine learning, take a different tack. These methods examine massive collections of images to identify underlying patterns. This learned knowledge helps them generate new pixel information that better captures detail, texture, and edges during upscaling.
Traditional methods work adequately for simple enlargements. However, they struggle when dealing with complex visuals requiring texture and detail reconstruction, often leading to a softened or blurred appearance with loss of fine detail.
AI techniques frequently utilize convolutional neural networks (CNNs). These network designs excel at recognizing features within images. This enables them to produce more realistic results that retain depth and structure compared to the linear approach of interpolation.
Interestingly, traditional interpolation doesn't adapt its output to the video content. AI methods, on the other hand, can adjust their outputs for different video types. This means they can, potentially, provide better outcomes for animation compared to live-action footage.
Traditional interpolation is computationally much faster, making it suitable for real-time applications. Yet, with continuously improving GPUs, AI-based upscaling solutions are starting to catch up, and real-time AI processing is becoming more commonplace.
One drawback of machine learning methods is the possibility of overfitting. This means a model trained on specific types of data might not perform as well on previously unseen images. This emphasizes the importance of using diverse training data to minimize these shortcomings.
Both approaches necessitate careful optimization. However, refining an AI model can significantly boost its predictive accuracy, potentially outperforming the less flexible nature of traditional interpolation.
Generally, traditional methods create a smoother output from a distance. But, as you get closer to the screen, AI-upscaled content often displays significantly more detail and visual quality.
AI's rise hasn't made traditional techniques obsolete. Some applications still leverage the speed of interpolation to pre-process videos before applying more resource-intensive machine learning techniques.
How Windows 10's Video Upscaling Compares to AI-Powered Solutions in 2024 - Neural Networks Enhancing Resolution and Reducing Noise
Neural networks are playing a crucial role in improving video quality, particularly in the areas of resolution enhancement and noise reduction within the realm of AI-powered upscaling. These sophisticated networks learn from vast amounts of visual data, identifying the complex connections between low-resolution and high-resolution images. This learned relationship then allows them to effectively reconstruct missing details during upscaling, often exceeding the capabilities of older methods. Beyond simply enlarging images, these AI techniques can intelligently add details and textures that weren't originally present, resulting in a sharper and more visually compelling output. Moreover, many AI upscalers now incorporate noise reduction features, making them valuable tools for cleaning up grainy or noisy video footage. While traditional upscaling methods remain useful in some cases, the advancements in neural networks represent a significant leap forward in video enhancement technology, offering capabilities that were previously out of reach. Their ability to adapt and learn makes them particularly promising for both casual use and professional applications where high-quality video is paramount.
Neural networks are increasingly used to enhance video resolution and reduce noise, often through a technique called "super-resolution." This approach involves training networks to understand the relationship between low and high-resolution images, allowing them to generate plausible details that aren't present in the original. It's a far cry from traditional methods that simply guess pixel values. One popular architecture for this task is the Generative Adversarial Network (GAN). GANs use a competitive framework with two networks: one generating higher-resolution images and the other acting as a critic, assessing realism. This competition pushes the generation process to continuously improve, resulting in more convincing outputs.
The noise reduction capabilities of these networks aren't just a happy accident. They're specifically designed to recognize and suppress high-frequency components often linked to unwanted artifacts in videos. By filtering out these frequencies, neural networks can clean up images without sacrificing crucial detail. Convolutional Neural Networks (CNNs) are particularly useful here, as they can be tailored with specific layers to focus on various frequencies, effectively distinguishing noise from real content.
Further enhancing their efficacy is a concept called "residual learning." This technique allows the networks to focus on reconstructing only the differences between low and high-resolution images, rather than the entire content. This can lead to significant improvements in accuracy and efficiency. Moreover, some advanced methods extend beyond frame-by-frame analysis. They consider a sequence of frames to incorporate motion information, making them particularly well-suited for handling dynamic scenes, leading to smoother and more detailed motion.
However, this powerful approach isn't without its drawbacks. AI-powered upscaling often requires significant memory resources due to the size of the models and training datasets. This can be a hurdle for users with less powerful systems that may not have high-bandwidth GPUs. Neural networks also exhibit a fascinating adaptive behavior. They can adjust their learning rates based on the input data, making them more flexible compared to traditional upscaling methods that lack this adaptive ability.
A substantial portion of the training process involves exposure to vast quantities of data. To prevent overfitting and promote wider application, data augmentation techniques are frequently used. This creates diverse training examples for the neural networks, increasing their robustness and ability to handle a variety of video scenarios. Despite these advancements, neural networks can sometimes stumble. Their effectiveness can be limited, especially in cases involving extremely low bit-rate videos. The inherent limitations of the input footage itself can sometimes limit how much detail and noise reduction can be realistically achieved. While neural networks are undoubtedly pushing the boundaries of video enhancement, they are still evolving, and certain challenges remain.
How Windows 10's Video Upscaling Compares to AI-Powered Solutions in 2024 - Topaz Video Enhance AI Tackles Motion Blur and Artifacts
Topaz Video Enhance AI is making improvements in tackling motion blur and other video artifacts. The latest version of the software brings enhancements, including a new stabilization filter and a dedicated AI model called Themis, designed specifically to combat motion blur. This, combined with its ability to upscale videos to 8K resolution, can lead to sharper and clearer video with fewer motion-related imperfections.
The software also allows you to enhance multiple videos simultaneously, saving time and effort. You can preview the results before applying changes, ensuring you're happy with the outcome. While other, simpler methods for video enhancement often struggle with these types of issues, Topaz attempts to overcome these with its more sophisticated AI approach. This means that potentially, users can see significantly improved results over the techniques used in Windows 10's basic video enhancement features.
Topaz Video Enhance AI uses machine learning, particularly a type of neural network called a convolutional neural network (CNN). These networks are quite good at keeping the original video looking natural while reducing common video problems that come with upscaling. This is a major step up from older techniques.
The software can look at the kind of video being upscaled— whether it's animation, real-life footage, or even scenes with low light—and change how it processes the video accordingly. This approach, called content-awareness, lets it use specialized methods for different video styles.
One interesting feature of Topaz Video Enhance AI is how it uses information across multiple frames in a video. By analyzing a sequence of frames, it's able to create smoother movements and reduce the blur that traditional techniques struggle with, especially in fast-moving scenes.
Topaz Video Enhance AI can also do what's called "video frame interpolation." This basically means it can add new frames between existing ones, effectively increasing the frame rate of the output. This helps smooth out action scenes with dropped frames.
The software's ability to reduce artifacts comes from being trained on a massive collection of video data. This allows it to not only recover missing details but also intelligently get rid of unwanted noise without losing important features of the original video.
Unlike older upscaling techniques that treat everything the same, Topaz can focus on improving certain areas of a single frame, making sure the most important details are sharper without upsetting the rest of the video.
While it can achieve substantial improvements, Topaz still faces limitations with extremely low-resolution or highly compressed source videos. If the initial quality is very poor, there's only so much that can be reconstructed.
The processing speed of AI-based upscaling varies depending on the video's complexity and how in-depth the model is. Some users experience slower performance with high-resolution videos compared to faster traditional interpolation methods.
Topaz has a user-friendly interface that offers lots of control over settings like noise reduction. This is important for professionals who need to carefully manage visual characteristics while still improving their footage.
Despite using complex algorithms, users often find it easy to get good results. The software can automatically determine optimal settings for a broad range of video content, making it suitable for both beginners and experienced editors.
How Windows 10's Video Upscaling Compares to AI-Powered Solutions in 2024 - VideoProc Converter AI Pushes Boundaries with 8K Upscaling
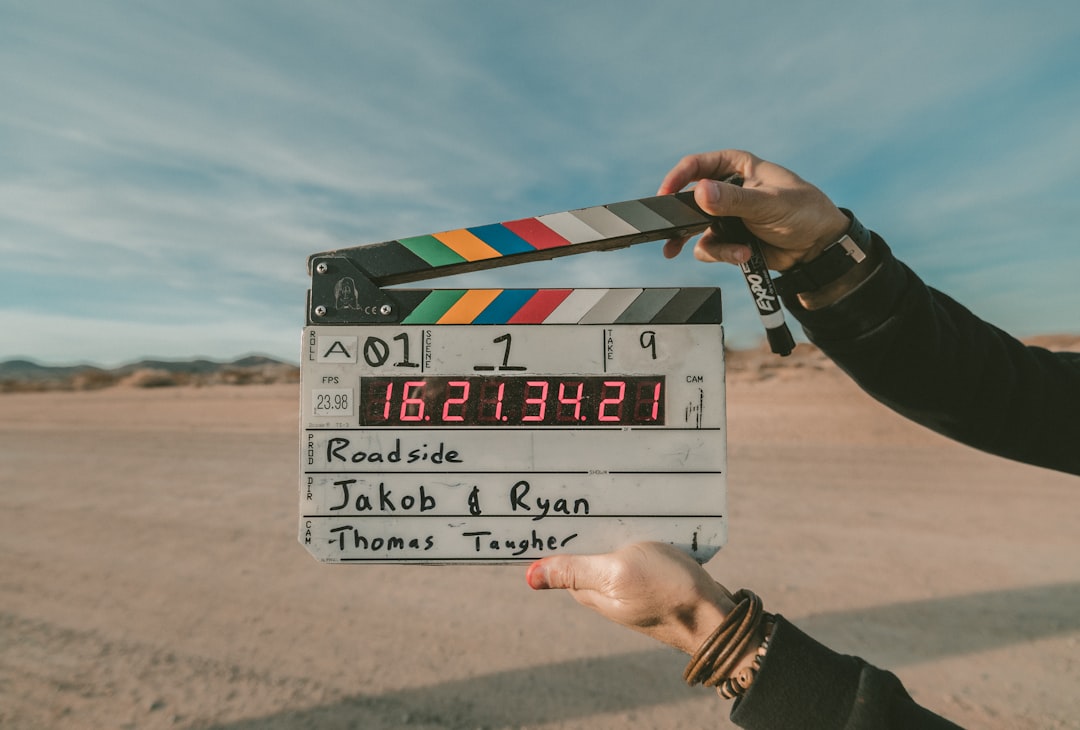
VideoProc Converter AI is making waves in video enhancement with its impressive ability to upscale videos to 8K resolution. It leverages AI technologies like Super Resolution to refine video quality, reducing noise and blur while retaining fine details. This newer software has introduced two distinct AI models, "Gen Detail" and "Real Smooth," potentially allowing for more nuanced control over the upscaling process depending on the video's content. Furthermore, its integration with NVIDIA TensorRT accelerates the AI processing, making it more efficient for users. While these features represent a step forward, it's essential to thoroughly evaluate its performance in various video scenarios and compare it to other AI upscaling options available. The field of AI video enhancement is constantly evolving, and solutions like VideoProc Converter AI will need to continuously adapt and improve to maintain a leading position.
The increasing prevalence of 8K content has driven the need for upscaling solutions capable of handling the higher pixel density and associated quality demands. This push has led to developments in AI algorithms specifically tailored to tackle these high-resolution challenges. AI models involved in video upscaling are often trained using a wide range of video sources, encompassing countless hours of footage from various genres. This breadth of training data fosters their ability to generalize, resulting in improved performance across a wide variety of video types and conditions.
Interestingly, some of the more advanced AI upscaling approaches don't just deal with individual frames in isolation. They can analyze and process multiple frames simultaneously, effectively incorporating temporal information. This approach allows for more context-aware enhancements, especially when it comes to reducing motion blur and ensuring a smoother representation of movement within the video. This is in contrast to the older methods which were primarily frame-by-frame techniques.
It's important to note that AI-powered solutions aren't solely focused on simply increasing resolution. They're adept at identifying and correcting various artifacts that often accompany video compression or low-quality sources. These artifacts can be problematic, and specialized neural networks have been designed to specifically deal with them. One aspect of this is the ability to preserve edges in images better than older methods. While traditional scaling techniques often lead to a softening of edges, AI methods can retain the fine detail and structure, particularly significant for high-resolution outputs.
However, there are tradeoffs. AI neural networks, due to their intricate structure, can be resource-intensive, demanding significant computational power and memory. This can place limitations on users with less powerful hardware. While this means greater performance, it also means there's a barrier to entry for certain users. Furthermore, while extensive training data improves performance, it can also lead to the model overfitting to the specific training data and underperforming on unfamiliar video material. This means the training data needs to be diverse and comprehensive for optimal performance in real-world scenarios.
Nevertheless, recent advancements are pushing toward real-time AI upscaling. While historically, AI processing speed has been a bottleneck, ongoing improvements in GPU technology are gradually closing that gap. As a result, it's now increasingly possible for users to enjoy high-quality video enhancements in real time.
Some AI upscaling tools are quite user-friendly. For example, VideoProc Converter uses features like automatic setting detection, making it accessible to users of varying skill levels. Those with less technical expertise can quickly and easily achieve good results without needing extensive understanding of the algorithms and their settings. Some AI systems even include frame interpolation techniques. This process adds synthetically generated frames between existing frames, which can increase the overall frame rate and create a smoother and more fluid viewing experience for motion-intensive videos. This is an area where the older upscaling methods often fall short.
How Windows 10's Video Upscaling Compares to AI-Powered Solutions in 2024 - TensorPix Offers Cloud-Based 4x Video Enlargement
TensorPix offers a cloud-based approach to video enhancement, relying on AI to improve image and video quality, including a 4x resolution boost. Besides upscaling, it boasts capabilities like restoring older videos, reducing noise, and even compressing videos. One interesting aspect is its cloud-based nature, which removes the need for local software and also enables it to process multiple videos concurrently thanks to GPU-powered cloud servers. While it positions itself as a competitor to other AI upscaling tools, its performance, pricing, and feature set need to be carefully examined to determine if it's a good fit for specific needs. In a field where tools like Topaz and VideoProc are making strides, TensorPix has its work cut out for it in proving itself a viable option.
TensorPix utilizes a cloud-based approach to video enhancement, relying on sophisticated neural networks to upscale video quality. These networks are designed to handle video sequences effectively, potentially reducing motion blur during action scenes while improving overall consistency. It's interesting how they've managed to leverage cloud computing to provide real-time upscaling. This is a significant benefit as complex AI models often introduce lag when processing.
One of the aspects that caught my attention is how TensorPix seems to be able to adapt its processing based on the video's nature, whether it's a live action film, animation, or a dimly lit scene. This adaptive behavior potentially leads to more nuanced enhancements. This approach, focusing on how the specific content is constructed, contrasts with some older upscaling tools which didn't take such factors into account.
The way TensorPix tries to reconstruct missing details and textures is particularly intriguing. It leverages what it's learned from a large dataset to improve upon the original. The reconstructed details, theoretically, should lead to a more realistic output.
The cloud-based nature of the system offers accessibility. Users can leverage powerful GPUs in the cloud, which are usually too expensive to obtain for most individuals or even small studios. This makes advanced upscaling tools available to a larger audience without the need for powerful computers locally.
In my initial observations, TensorPix seems to effectively manage noise reduction. This is an important aspect since a lot of older scaling methods tended to amplify noise during upscaling, which can degrade the overall quality.
TensorPix, in its promotional material, emphasizes the ability to upscale content up to 4x its original size. The industry seems to be moving towards higher resolutions like 4K and even 8K, and this aspect of TensorPix makes it potentially suitable for these new video standards.
TensorPix also aims to address common issues arising from compression. Compression artifacts like blockiness and banding can ruin the visual experience. TensorPix's approach claims to significantly reduce these artifacts.
Despite its complexity, TensorPix is being promoted as a user-friendly solution. Users with varying skill levels should be able to utilize the tool, even without deep technical expertise. It seems they've focused on providing a straightforward user interface, which is a plus.
A key aspect is how well it integrates with other workflows. Professionals who are already using a particular video editing software or pipeline can potentially use TensorPix without causing disruptions to their existing system. This could help it be integrated into professional settings more readily.
Of course, these are preliminary observations, and I believe more in-depth testing is needed to get a better understanding of how it performs in different situations and how it compares to other AI video enhancement tools. But, based on initial impressions, TensorPix seems to be a promising addition to the field of AI upscaling, particularly for individuals and studios seeking accessibility to advanced video enhancement techniques.
How Windows 10's Video Upscaling Compares to AI-Powered Solutions in 2024 - DaVinci Resolve Balances Free and Paid AI Upscaling Options
DaVinci Resolve provides a mix of free and paid AI upscaling tools, making it appealing to a diverse user base. The free version is a solid option, but those wanting higher-end features will need to upgrade to the Studio edition for a one-time fee of $295. This Studio edition offers the impressive Super Scale feature, which intelligently uses AI to upscale videos, particularly converting 1080p footage to a sharper 4K. Along with Super Scale, Resolve boasts features like Face Refinement and IntelliTrack that expand its usability for various video editing tasks. While other AI upscaling software like Topaz are popular, Resolve stands out because it seamlessly blends free and advanced paid capabilities. This approach could attract a wide audience, particularly users who start with the free edition and eventually discover the value of the Studio features.
DaVinci Resolve offers a compelling approach to AI video upscaling, providing both a free version and a paid Studio version for $295. The free version provides a taste of what AI-powered upscaling can do, while the paid version unlocks features like Super Scale, which utilizes sophisticated AI algorithms to upscale 1080p video to 4K and beyond, significantly improving image clarity. It's interesting to see how Resolve handles motion through its built-in optical flow, attempting to create a smoother upscale compared to the usual interpolation approaches which can lead to blur.
Furthermore, DaVinci Resolve offers plenty of control over the AI upscaling process, allowing users to adjust parameters and refine the output to best suit their projects. The fact it supports a range of resolutions, including 8K, also indicates a flexibility and future-proofing that's useful in today's content creation landscape. A key element of Resolve's AI approach is the smart generation of pixels, where it tries to predict missing information from what it's learned, rather than just using the surrounding pixels. This, in theory, should lead to sharper detail.
However, users should be aware that Resolve's more sophisticated AI upscaling features, found in the paid version, can demand a powerful system to function without lagging or producing slow rendering times. Also, despite the advancements, even the AI in Resolve has limitations with extremely low-resolution content, as there's only so much that can be reconstructed from a limited set of visual information. The paid Studio version benefits from continuous improvements through machine learning updates, though, suggesting the AI model within it will gradually improve over time.
Comparing Resolve's AI upscaling to traditional techniques, particularly Windows 10's interpolation methods, it's evident that AI excels at both image quality and versatility. Resolve can even adapt the upscaling to the style of the video (animation, live action, etc.) which demonstrates a level of flexibility beyond the limitations of Windows' methods. While AI upscaling is still evolving, Resolve, with its combination of free and paid options and its adaptability, presents a valuable tool for anyone interested in exploring the possibilities of AI video upscaling.
More Posts from ai-videoupscale.com: